NNCF を使用した DeepFloyd IF モデルのトレーニング後の量子化と重み圧縮¶
この Jupyter ノートブックは、ローカルへのインストール後にのみ起動できます。
このチュートリアルの目的は、NNCF (Neural Network Compression Framework) から 8 ビットのトレーニング後の量子化と重み圧縮を適用してモデルを高速化し、OpenVINO™ ツールキットによって最適化されたモデルを推論する方法をデモンストレーションすることです。
注: 最初に 238-deep-floyd-if-convert ノートブックを実行して、最適化に使用される OpenVINO IR モデルを生成する必要があります。
最適化プロセスには次の手順が含まれます。
- NNCF を使用して、ノートブックから変換された OpenVINO テキスト・エンコーダーの重みを圧縮します。
- NNCF を使用して、ノートブックから変換された stage_1 および stage_2 U-Net を量子化します。
- ノートブックから同じ入力データを使用してモデルの結果を確認します。
- 変換および最適化されたモデルのモデルサイズを比較します。
- 変換および最適化されたモデルのパフォーマンスを比較します。
目次¶
必要条件¶
%pip install -q datasets "nncf>=2.6.0"
import nncf
import torch
import openvino as ov
from diffusers import DiffusionPipeline
from diffusers.utils.pil_utils import pt_to_pil
from pathlib import Path
from typing import Any, List
from utils import TextEncoder, UnetFirstStage, UnetSecondStage
checkpoint_variant = 'fp16'
model_dtype = torch.float32
RANDOM_SEED = 42
N_DIFFUSION_STEPS = 50
UNET_2_STEPS = 20
core = ov.Core()
INFO:nncf:NNCF initialized successfully. Supported frameworks detected: torch, tensorflow, onnx, openvino
MODEL_DIR = Path('./models')
TEXT_ENCODER_IR_PATH = MODEL_DIR / "encoder_ir.xml"
UNET_I_IR_PATH = MODEL_DIR / "unet_ir_I.xml"
UNET_II_IR_PATH = MODEL_DIR / "unet_ir_II.xml"
if not (TEXT_ENCODER_IR_PATH.exists() and UNET_I_IR_PATH.exists() and UNET_II_IR_PATH.exists()):
raise RuntimeError('This notebook should be run after 238-deep-floyd-if notebook')
import ipywidgets as widgets
device = widgets.Dropdown(
options=core.available_devices + ["AUTO"],
value='AUTO',
description='Device:',
disabled=False,
)
device
Dropdown(description='Device:', index=2, options=('CPU', 'GPU', 'AUTO'), value='AUTO')
重みを圧縮¶
テキスト・エンコーダー・モデルは、最大 22 GB のディスク領域を消費します。メモリー不足を避けるために、量子化の代わりに 8 ビットの重み圧縮を使用することを推奨します。最適化されたモデルは、量子化されたモデルと比較して速度の向上は得られませんが、モデルのフットプリントは大幅に削減されます。
%%time
text_encoder = core.read_model(TEXT_ENCODER_IR_PATH)
text_encoder_optimized = nncf.compress_weights(text_encoder)
TEXT_ENCODER_INT8_IR_PATH = Path("_optimized.".join(TEXT_ENCODER_IR_PATH.as_posix().split(".")))
ov.save_model(text_encoder_optimized, TEXT_ENCODER_INT8_IR_PATH)
2023-10-30 08:36:34.384792: I tensorflow/core/util/port.cc:110] oneDNN custom operations are on. You may see slightly different numerical results due to floating-point round-off errors from different computation orders. To turn them off, set the environment variable TF_ENABLE_ONEDNN_OPTS=0. 2023-10-30 08:36:34.423283: I tensorflow/core/platform/cpu_feature_guard.cc:182] This TensorFlow binary is optimized to use available CPU instructions in performance-critical operations. To enable the following instructions: AVX2 AVX512F AVX512_VNNI FMA, in other operations, rebuild TensorFlow with the appropriate compiler flags. 2023-10-30 08:36:35.184200: W tensorflow/compiler/tf2tensorrt/utils/py_utils.cc:38] TF-TRT Warning: Could not find TensorRT
CPU times: user 3min 16s, sys: 58 s, total: 4min 14s
Wall time: 4min 12s
量子化¶
データセットの準備¶
DeepFloyd IF は、第 1 ステージと第 2 ステージの U-Net モデルで構成されます。第 1 ステージの U-Net はテキストプロンプトに基づいて 64x64 ピクセルの画像を生成し、第 2 ステージの U-Net は前のステップの画像に基づいて 256x256 ピクセルの画像を生成します。Hugging Face のトレーニング LAION2B データセットの一部をキャリブレーション・データとして使用します。LAION2B は LAION5B データセットの英語のサブセットで、20 億を超えるオブジェクトが含まれています。
import numpy as np
from datasets import load_dataset
np.random.seed(RANDOM_SEED)
def get_negative_prompt():
negative_prompts = [
"amateur", "blurred", "deformed", "disfigured", "disgusting", "jpeg artifacts", "low contrast",
"low quality", "low saturation", "mangled", "morbid", "mutilated", "mutation",
"out of frame", "out of frame", "ugly", "uncentered", "underexposed", "unreal",
]
num_elements = np.random.randint(2, 6)
random_elements = np.random.choice(negative_prompts, num_elements)
return [" ".join(random_elements)]
def prepare_calibration_data(dataloader, stage_1):
"""
This function prepares calibration data from a dataloader for a specified number of initialization steps.
It iterates over the dataloader, fetching batches and storing the relevant data.
"""
data = []
for batch in dataloader:
prompt = batch["TEXT"]
negative_prompt = get_negative_prompt()
prompt_embeds, negative_embeds = stage_1.encode_prompt(prompt, negative_prompt=negative_prompt)
data.append((prompt_embeds, negative_embeds))
return data
def prepare_dataset(stage_1, opt_init_steps=300):
"""
Prepares a text dataset for quantization.
"""
dataset = load_dataset("laion/laion2B-en-aesthetic", streaming=True, split="train")
train_dataset = dataset.shuffle(seed=RANDOM_SEED, buffer_size=1000).take(opt_init_steps)
dataloader = torch.utils.data.DataLoader(train_dataset, batch_size=1)
calibration_data = prepare_calibration_data(dataloader, stage_1)
return calibration_data
%%time
generator = torch.manual_seed(RANDOM_SEED)
opt_init_steps = 300
selection_prob = 0.5
prompts_number = np.ceil(opt_init_steps // (min(N_DIFFUSION_STEPS, UNET_2_STEPS) * selection_prob))
stage_1 = DiffusionPipeline.from_pretrained(
"DeepFloyd/IF-I-M-v1.0",
variant=checkpoint_variant,
torch_dtype=model_dtype
)
encoded_prompts = prepare_dataset(stage_1, int(prompts_number))
safety_checker/model.safetensors not found
A mixture of fp16 and non-fp16 filenames will be loaded.
Loaded fp16 filenames:
[text_encoder/pytorch_model.fp16-00001-of-00002.bin, unet/diffusion_pytorch_model.fp16.bin, text_encoder/pytorch_model.fp16-00002-of-00002.bin]
Loaded non-fp16 filenames:
[safety_checker/pytorch_model.bin, watermarker/diffusion_pytorch_model.bin
If this behavior is not expected, please check your folder structure.
Cannot initialize model with low cpu memory usage because accelerate was not found in the environment. Defaulting to low_cpu_mem_usage=False. It is strongly recommended to install accelerate for faster and less memory-intense model loading. You can do so with:
`
pip install accelerate
`
.
Loading pipeline components...: 0%| | 0/7 [00:00<?, ?it/s]
You are using the default legacy behaviour of the <class 'transformers.models.t5.tokenization_t5.T5Tokenizer'>. This is expected, and simply means that the legacy (previous) behavior will be used so nothing changes for you. If you want to use the new behaviour, set legacy=False. This should only be set if you understand what it means, and thouroughly read the reason why this was added as explained in https://github.com/huggingface/transformers/pull/24565 /home/ea/work/ov_venv/lib/python3.8/site-packages/torch/cuda/__init__.py:138: UserWarning: CUDA initialization: The NVIDIA driver on your system is too old (found version 11080). Please update your GPU driver by downloading and installing a new version from the URL: http://www.nvidia.com/Download/index.aspx Alternatively, go to: https://pytorch.org to install a PyTorch version that has been compiled with your version of the CUDA driver. (Triggered internally at ../c10/cuda/CUDAFunctions.cpp:108.) return torch._C._cuda_getDeviceCount() > 0
Loading checkpoint shards: 0%| | 0/2 [00:00<?, ?it/s]
Downloading readme: 0%| | 0.00/56.0 [00:00<?, ?B/s]
Resolving data files: 0%| | 0/128 [00:00<?, ?it/s]
CPU times: user 18min 16s, sys: 1min 2s, total: 19min 18s
Wall time: 2min 5s
キャリブレーション用の中間モデル入力を収集するには、CompiledModel
をカスタマイズする必要があります。
class CompiledModelDecorator(ov.CompiledModel):
def __init__(self, compiled_model, prob: float, data_cache: List[Any] = []):
super().__init__(compiled_model)
self.data_cache = data_cache
self.prob = np.clip(prob, 0, 1)
def __call__(self, *args, **kwargs):
if np.random.rand() >= self.prob:
self.data_cache.append(*args)
return super().__call__(*args, **kwargs)
stage_1.unet = UnetFirstStage(
UNET_I_IR_PATH,
stage_1.unet.config,
dtype=model_dtype,
device=device.value
)
stage_1.set_progress_bar_config(disable=True)
stage_1_data_cache = []
stage_1.unet.unet_openvino = CompiledModelDecorator(stage_1.unet.unet_openvino, prob=selection_prob, data_cache=stage_1_data_cache)
generator = torch.manual_seed(RANDOM_SEED)
stage_2_inputs = [] # to speed up dataset preparation for stage 2 U-Net we can collect several images below
for data in encoded_prompts:
prompt_embeds, negative_embeds = data
image = stage_1(prompt_embeds=prompt_embeds, negative_prompt_embeds=negative_embeds,
generator=generator, output_type="pt", num_inference_steps=N_DIFFUSION_STEPS).images
stage_2_inputs.append((image, prompt_embeds, negative_embeds))
if len(stage_1_data_cache) >= opt_init_steps:
break
量子化第 1 ステージ U-Net¶
%%time
ov_model = core.read_model(UNET_I_IR_PATH)
stage_1_calibration_dataset = nncf.Dataset(stage_1_data_cache, lambda x: x)
quantized_model = nncf.quantize(
model=ov_model,
calibration_dataset=stage_1_calibration_dataset,
model_type=nncf.ModelType.TRANSFORMER,
advanced_parameters=nncf.AdvancedQuantizationParameters(smooth_quant_alpha=0.25)
)
UNET_I_INT8_PATH = "_optimized.".join(UNET_I_IR_PATH.as_posix().split("."))
ov.save_model(quantized_model, UNET_I_INT8_PATH)
Statistics collection: 100%|████████████████████████████████████████████████████████████████████████████████████████████████████████████████████████████████████████████████| 300/300 [01:35<00:00, 3.14it/s]
Applying Smooth Quant: 100%|██████████████████████████████████████████████████████████████████████████████████████████████████████████████████████████████████████████████████| 73/73 [00:04<00:00, 17.55it/s]
Statistics collection: 100%|████████████████████████████████████████████████████████████████████████████████████████████████████████████████████████████████████████████████| 300/300 [05:44<00:00, 1.15s/it]
Applying Fast Bias correction: 100%|████████████████████████████████████████████████████████████████████████████████████████████████████████████████████████████████████████| 268/268 [00:35<00:00, 7.50it/s]
CPU times: user 1h 8min 46s, sys: 1min 22s, total: 1h 10min 8s
Wall time: 9min 46s
%%time
from tqdm.notebook import tqdm
start = len(stage_2_inputs)
for i, data in tqdm(enumerate(encoded_prompts[start:])):
prompt_embeds, negative_embeds = data
image = stage_1(prompt_embeds=prompt_embeds, negative_prompt_embeds=negative_embeds,
generator=generator, output_type="pt", num_inference_steps=N_DIFFUSION_STEPS).images
stage_2_inputs.append((image, prompt_embeds, negative_embeds))
0it [00:00, ?it/s]
CPU times: user 1h 17min 46s, sys: 44.9 s, total: 1h 18min 31s
Wall time: 4min 46s
%%time
generator = torch.manual_seed(RANDOM_SEED)
opt_init_steps = 300
stage_2 = DiffusionPipeline.from_pretrained(
"DeepFloyd/IF-II-M-v1.0",
text_encoder=None,
variant=checkpoint_variant,
torch_dtype=model_dtype
)
stage_2.set_progress_bar_config(disable=True)
stage_2.unet = UnetSecondStage(
UNET_II_IR_PATH,
stage_2.unet.config,
dtype=model_dtype,
device=device.value
)
stage_2_data_cache = []
stage_2.unet.unet_openvino = CompiledModelDecorator(stage_2.unet.unet_openvino, prob=selection_prob, data_cache=stage_2_data_cache)
for data in tqdm(stage_2_inputs):
image, prompt_embeds, negative_embeds = data
image = stage_2(image=image, prompt_embeds=prompt_embeds, negative_prompt_embeds=negative_embeds,
generator=generator, output_type="pt", num_inference_steps=UNET_2_STEPS).images
if len(stage_2_data_cache) >= opt_init_steps:
break
A mixture of fp16 and non-fp16 filenames will be loaded.
Loaded fp16 filenames:
[text_encoder/model.fp16-00001-of-00002.safetensors, unet/diffusion_pytorch_model.fp16.safetensors, text_encoder/model.fp16-00002-of-00002.safetensors, safety_checker/model.fp16.safetensors]
Loaded non-fp16 filenames:
[watermarker/diffusion_pytorch_model.safetensors
If this behavior is not expected, please check your folder structure.
Cannot initialize model with low cpu memory usage because accelerate was not found in the environment. Defaulting to low_cpu_mem_usage=False. It is strongly recommended to install accelerate for faster and less memory-intense model loading. You can do so with:
`
pip install accelerate
`
.
Loading pipeline components...: 0%| | 0/7 [00:00<?, ?it/s]
CPU times: user 6h 28min 3s, sys: 2min 11s, total: 6h 30min 15s
Wall time: 24min 32s
量子化第 2 ステージ U-Net¶
%%time
ov_model = core.read_model(UNET_II_IR_PATH)
calibration_dataset = nncf.Dataset(stage_2_data_cache, lambda x: x)
quantized_model = nncf.quantize(
model=ov_model,
calibration_dataset=calibration_dataset,
model_type=nncf.ModelType.TRANSFORMER,
)
UNET_II_INT8_PATH = "_optimized.".join(UNET_II_IR_PATH.as_posix().split("."))
ov.save_model(quantized_model, UNET_II_INT8_PATH)
Statistics collection: 100%|████████████████████████████████████████████████████████████████████████████████████████████████████████████████████████████████████████████████| 300/300 [12:02<00:00, 2.41s/it]
Applying Smooth Quant: 100%|██████████████████████████████████████████████████████████████████████████████████████████████████████████████████████████████████████████████████| 54/54 [00:03<00:00, 15.80it/s]
Statistics collection: 100%|████████████████████████████████████████████████████████████████████████████████████████████████████████████████████████████████████████████████| 300/300 [34:51<00:00, 6.97s/it]
Applying Fast Bias correction: 100%|████████████████████████████████████████████████████████████████████████████████████████████████████████████████████████████████████████| 245/245 [00:39<00:00, 6.17it/s]
CPU times: user 7h 57min 5s, sys: 6min 43s, total: 8h 3min 49s
Wall time: 49min 24s
最適化された OpenVINO モデルを実行¶
1 番目のノートブックから同じ入力データを使用して、最適化された OpenVINO DeepFloyd IF モデルの結果で予測を確認してみます。
prompt = 'ultra close color photo portrait of rainbow owl with deer horns in the woods'
negative_prompt = 'blurred unreal uncentered occluded'
%%time
stage_1 = DiffusionPipeline.from_pretrained(
"DeepFloyd/IF-I-M-v1.0",
variant=checkpoint_variant,
torch_dtype=model_dtype
)
# Initialize the First Stage U-Net wrapper class
stage_1.unet = UnetFirstStage(
UNET_I_INT8_PATH,
stage_1.unet.config,
dtype=model_dtype,
device=device.value
)
stage_1.text_encoder = TextEncoder(TEXT_ENCODER_INT8_IR_PATH, dtype=model_dtype, device=device.value)
print('The model has been loaded')
# Generate text embeddings
prompt_embeds, negative_embeds = stage_1.encode_prompt(prompt, negative_prompt=negative_prompt)
# Fix PRNG seed
generator = torch.manual_seed(RANDOM_SEED)
# Inference
image = stage_1(prompt_embeds=prompt_embeds, negative_prompt_embeds=negative_embeds,
generator=generator, output_type="pt", num_inference_steps=N_DIFFUSION_STEPS).images
# Show the image
pt_to_pil(image)[0]
safety_checker/model.safetensors not found
A mixture of fp16 and non-fp16 filenames will be loaded.
Loaded fp16 filenames:
[text_encoder/pytorch_model.fp16-00001-of-00002.bin, unet/diffusion_pytorch_model.fp16.bin, text_encoder/pytorch_model.fp16-00002-of-00002.bin]
Loaded non-fp16 filenames:
[safety_checker/pytorch_model.bin, watermarker/diffusion_pytorch_model.bin
If this behavior is not expected, please check your folder structure.
Cannot initialize model with low cpu memory usage because accelerate was not found in the environment. Defaulting to low_cpu_mem_usage=False. It is strongly recommended to install accelerate for faster and less memory-intense model loading. You can do so with:
`
pip install accelerate
`
.
Loading pipeline components...: 0%| | 0/7 [00:00<?, ?it/s]
Loading checkpoint shards: 0%| | 0/2 [00:00<?, ?it/s]
The model has been loaded
0%| | 0/50 [00:00<?, ?it/s]
CPU times: user 3min 39s, sys: 21 s, total: 4min
Wall time: 58.7 s

%%time
stage_2 = DiffusionPipeline.from_pretrained(
"DeepFloyd/IF-II-M-v1.0",
text_encoder=None,
variant=checkpoint_variant,
torch_dtype=model_dtype
)
# Initialize the Second Stage U-Net wrapper class
stage_2.unet = UnetSecondStage(
UNET_II_INT8_PATH,
stage_2.unet.config,
dtype=model_dtype,
device=device.value
)
print('The model has been loaded')
image = stage_2(
image=image, prompt_embeds=prompt_embeds, negative_prompt_embeds=negative_embeds,
generator=generator, output_type="pt", num_inference_steps=UNET_2_STEPS).images
# Show the image
pil_image = pt_to_pil(image)[0]
pil_image
A mixture of fp16 and non-fp16 filenames will be loaded.
Loaded fp16 filenames:
[text_encoder/model.fp16-00001-of-00002.safetensors, unet/diffusion_pytorch_model.fp16.safetensors, text_encoder/model.fp16-00002-of-00002.safetensors, safety_checker/model.fp16.safetensors]
Loaded non-fp16 filenames:
[watermarker/diffusion_pytorch_model.safetensors
If this behavior is not expected, please check your folder structure.
Cannot initialize model with low cpu memory usage because accelerate was not found in the environment. Defaulting to low_cpu_mem_usage=False. It is strongly recommended to install accelerate for faster and less memory-intense model loading. You can do so with:
`
pip install accelerate
`
.
Loading pipeline components...: 0%| | 0/7 [00:00<?, ?it/s]
The model has been loaded
0%| | 0/20 [00:00<?, ?it/s]
CPU times: user 6min 20s, sys: 6.78 s, total: 6min 27s
Wall time: 32.1 s
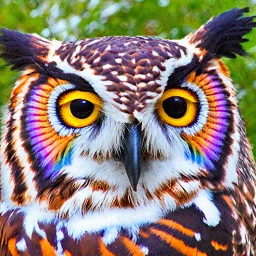
import cv2
import numpy as np
from utils import convert_result_to_image, download_omz_model
# 1032: 4x superresolution, 1033: 3x superresolution
model_name = 'single-image-super-resolution-1032'
download_omz_model(model_name, MODEL_DIR)
sr_model_xml_path = MODEL_DIR / f'{model_name}.xml'
model = core.read_model(model=sr_model_xml_path)
model.reshape({
0: [1, 3, 256, 256],
1: [1, 3, 1024, 1024]
})
compiled_sr_model = core.compile_model(model=model, device_name=device.value)
original_image = np.array(pil_image)
bicubic_image = cv2.resize(
src=original_image, dsize=(1024, 1024), interpolation=cv2.INTER_CUBIC
)
# Reshape the images from (H,W,C) to (N,C,H,W) as expected by the model.
input_image_original = np.expand_dims(original_image.transpose(2, 0, 1), axis=0)
input_image_bicubic = np.expand_dims(bicubic_image.transpose(2, 0, 1), axis=0)
# Model Inference
result = compiled_sr_model(
[input_image_original, input_image_bicubic]
)[compiled_sr_model.output(0)]
img = convert_result_to_image(result)
img
single-image-super-resolution-1032 already downloaded to models
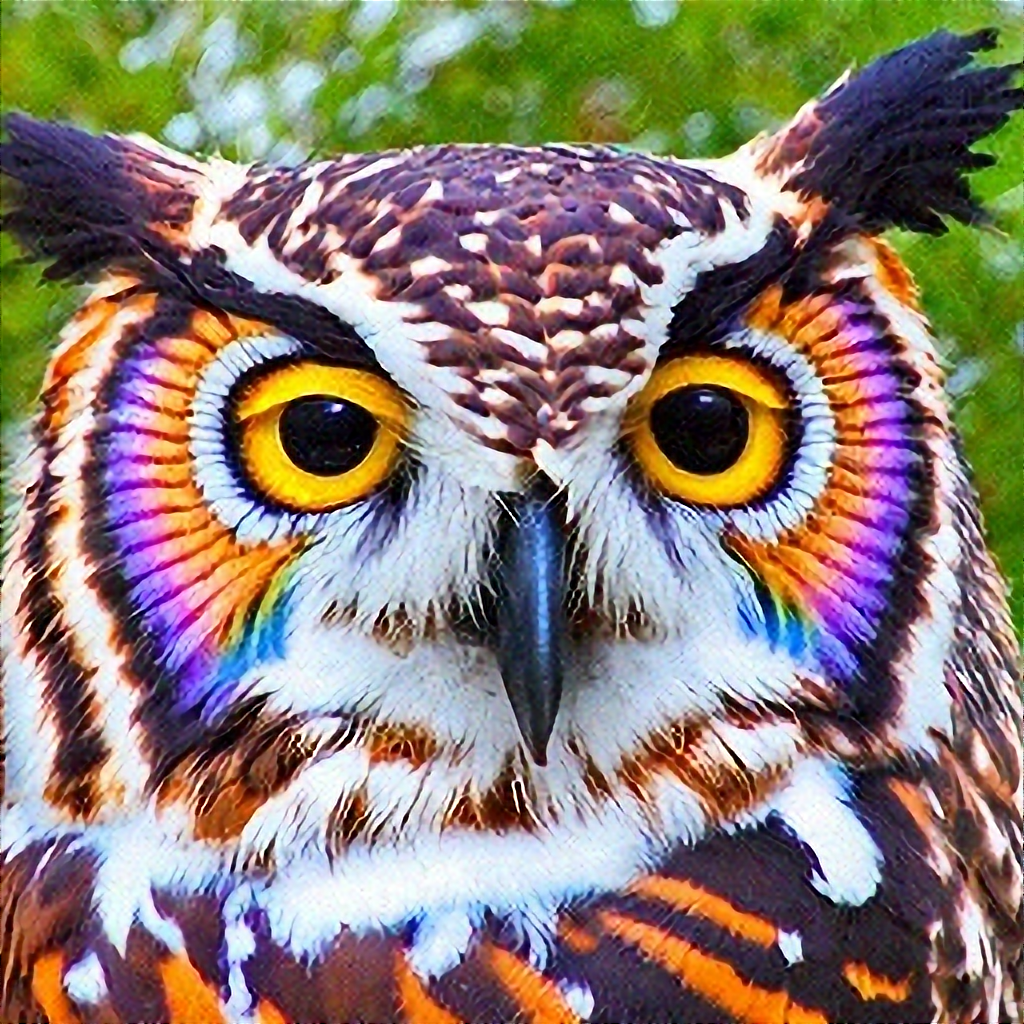
注: 量子化モデルの精度は、通常、キャリブレーション・データセットのサイズを増やすことで改善できます。U-Net モデルでは、selection_prob
値を小さくすると、多様なデータセットを収集できますが、データセットの収集時間が長くなります。
ファイルサイズを比較¶
FP16 OpenVINO モデルのファイルサイズに対する最適化された IR ファイルサイズの圧縮率を計算してみます。
def calculate_compression_rate(ov_model_path):
fp16_ir_model_size = Path(ov_model_path).with_suffix(".bin").stat().st_size / 1024 / 1024
int8_model_path = "_optimized.".join(ov_model_path.as_posix().split("."))
quantized_model_size = Path(int8_model_path).with_suffix(".bin").stat().st_size / 1024 / 1024
print(f'{ov_model_path.as_posix().split(".")[0]}')
print(f" * FP16 IR model size: {fp16_ir_model_size:.2f} MB")
print(f" * INT8 model size: {quantized_model_size:.2f} MB")
print(f" * Model compression rate: {fp16_ir_model_size / quantized_model_size:.3f}")
for model_path in [TEXT_ENCODER_IR_PATH, UNET_I_IR_PATH, UNET_II_IR_PATH]:
calculate_compression_rate(model_path)
models/encoder_ir
* FP16 IR model size: 22006.77 MB
* INT8 model size: 4546.70 MB
* Model compression rate: 4.840
models/unet_ir_I
* FP16 IR model size: 1417.56 MB
* INT8 model size: 355.16 MB
* Model compression rate: 3.991
models/unet_ir_II
* FP16 IR model size: 1758.82 MB
* INT8 model size: 440.49 MB
* Model compression rate: 3.993
変換および最適化されたモデルのパフォーマンス時間を比較¶
OpenVINO FP16 および INT8 モデルの推論パフォーマンスを測定するには、Benchmark ツールを使用します。
注: より正確なパフォーマンスを得るには、他のアプリケーションを閉じて、ターミナル/コマンドプロンプトで
benchmark_app
を実行します。benchmark_app --help
を実行すると、すべてのコマンドライン・オプションの概要が表示されます。
import re
def get_fps(benchmark_output: str):
parsed_output = [line for line in benchmark_output if 'Throughput:' in line]
fps = re.findall(r"\d+\.\d+", parsed_output[0])[0]
return fps
テキスト・エンコーダー
benchmark_output = !benchmark_app -m $TEXT_ENCODER_IR_PATH -d $device.value -api async
original_fps = get_fps(benchmark_output)
print(f"FP16 Text Encoder Throughput: {original_fps} FPS")
benchmark_output = !benchmark_app -m $TEXT_ENCODER_INT8_IR_PATH -d $device.value -api async
optimized_fps = get_fps(benchmark_output)
print(f"INT8 Text Encoder Throughput: {optimized_fps} FPS")
print(f"Text encoder speed up: {float(optimized_fps) / float(original_fps)}")
FP16 Text Encoder Throughput: 0.99 FPS
INT8 Text Encoder Throughput: 2.47 FPS
Text encoder speed up: 2.4949494949494953
第 1 ステージ U-Net
benchmark_output = !benchmark_app -m $UNET_I_IR_PATH -d $device.value -api async
original_fps = get_fps(benchmark_output)
print(f"FP16 1 stage U-Net Throughput: {original_fps} FPS")
benchmark_output = !benchmark_app -m $UNET_I_INT8_PATH -d $device.value -api async
optimized_fps = get_fps(benchmark_output)
print(f"INT8 1 stage U-Net Throughput: {optimized_fps} FPS")
print(f"1 stage U-Net speed up: {float(optimized_fps) / float(original_fps)}")
FP16 1 stage U-Net Throughput: 4.65 FPS
INT8 1 stage U-Net Throughput: 12.06 FPS
1 stage U-Net speed up: 2.593548387096774
第 2 ステージ U-Net
benchmark_output = !benchmark_app -m $UNET_II_IR_PATH -d $device.value -api async
original_fps = get_fps(benchmark_output)
print(f"FP16 2 stage U-Net Throughput: {original_fps} FPS")
benchmark_output = !benchmark_app -m $UNET_II_INT8_PATH -d $device.value -api async
optimized_fps = get_fps(benchmark_output)
print(f"INT8 2 stage U-Net Throughput: {optimized_fps} FPS")
print(f"2 stage U-Net speed up: {float(optimized_fps) / float(original_fps)}")
FP16 2 stage U-Net Throughput: 0.28 FPS
INT8 2 stage U-Net Throughput: 0.92 FPS
2 stage U-Net speed up: 3.2857142857142856