PhotoMaker と OpenVINO を使用したテキストからの画像生成¶
この Jupyter ノートブックは、ローカルへのインストール後にのみ起動できます。
PhotoMaker は効率的なパーソナライズされたテキストから画像への生成方法であり、主に ID 情報を保存するため任意の数の入力 ID 画像をスタック ID 埋め込みにエンコードします。このような埋め込みは、統一 ID 表現として機能し、同じ入力 ID の特性を包括的にカプセル化するだけでなく、その後統合するため異なる ID の特性にも対応できます。これにより、より興味深い、実用的なアプリケーションへの道が開かれます。ユーザーは、テキストプロンプトとともに 1 つまたは数枚の顔写真を入力して、カスタマイズされた写真または絵画を受け取ることができます (トレーニングは必要ありません)。さらに、このモデルは、SDXL
に基づく任意の基本モデルに適合させたり、他の LoRA
モジュールと組み合わせて使用することができます。PhotoMaker の詳細については、技術レポートを参照してください。
このノートブックでは、OpenVINO を使用して PhotoMaker パイプラインを高速化する方法を検討します。
目次¶
PhotoMaker パイプラインの紹介¶
提案されている PhotoMaker では、最初にテキスト・エンコーダーと画像 (ID) エンコーダーからそれぞれテキスト埋め込みと画像埋め込みを取得します。次に、対応するクラスの埋め込み (男性と女性など) と各画像の埋め込みを結合することにより、融合された埋め込みを抽出します。次に、すべての融合された埋め込みを長さの次元に沿って連結して、スタックされた ID 埋め込みを形成します。最後に、diffusion model
内の ID コンテンツを適応的にマージするため、スタックされた ID 埋め込みをすべてのクロスアテンション・レイヤーにフィードします。トレーニング中に背景がマスクされた同じ ID の画像を使用しますが、推論中に背景の歪みなしで異なる ID の画像を直接入力し、新しい ID を作成できることに注意してください。
必要条件¶
PhotoMaker リポジトリーのクローンを作成します。
from pathlib import Path
if not Path("PhotoMaker").exists():
!git clone https://github.com/TencentARC/PhotoMaker.git
Cloning into 'PhotoMaker'...
remote: Enumerating objects: 212, done.[K
remote: Counting objects: 0% (1/121)[K
remote: Counting objects: 1% (2/121)[K
remote: Counting objects: 2% (3/121)[K
remote: Counting objects: 3% (4/121)[K
remote: Counting objects: 4% (5/121)[K
remote: Counting objects: 5% (7/121)[K
remote: Counting objects: 6% (8/121)[K
remote: Counting objects: 7% (9/121)[K
remote: Counting objects: 8% (10/121)[K
remote: Counting objects: 9% (11/121)[K
remote: Counting objects: 10% (13/121)[K
remote: Counting objects: 11% (14/121)[K
remote: Counting objects: 12% (15/121)[K
remote: Counting objects: 13% (16/121)[K
remote: Counting objects: 14% (17/121)[K
remote: Counting objects: 15% (19/121)[K
remote: Counting objects: 16% (20/121)[K
remote: Counting objects: 17% (21/121)[K
remote: Counting objects: 18% (22/121)[K
remote: Counting objects: 19% (23/121)[K
remote: Counting objects: 20% (25/121)[K
remote: Counting objects: 21% (26/121)[K
remote: Counting objects: 22% (27/121)[K
remote: Counting objects: 23% (28/121)[K
remote: Counting objects: 24% (30/121)[K
remote: Counting objects: 25% (31/121)[K
remote: Counting objects: 26% (32/121)[K
remote: Counting objects: 27% (33/121)[K
remote: Counting objects: 28% (34/121)[K
remote: Counting objects: 29% (36/121)[K
remote: Counting objects: 30% (37/121)[K
remote: Counting objects: 31% (38/121)[K
remote: Counting objects: 32% (39/121)[K
remote: Counting objects: 33% (40/121)[K
remote: Counting objects: 34% (42/121)[K
remote: Counting objects: 35% (43/121)[K
remote: Counting objects: 36% (44/121)[K
remote: Counting objects: 37% (45/121)[K
remote: Counting objects: 38% (46/121)[K
remote: Counting objects: 39% (48/121)[K
remote: Counting objects: 40% (49/121)[K
remote: Counting objects: 41% (50/121)[K
remote: Counting objects: 42% (51/121)[K
remote: Counting objects: 43% (53/121)[K
remote: Counting objects: 44% (54/121)[K
remote: Counting objects: 45% (55/121)[K
remote: Counting objects: 46% (56/121)[K
remote: Counting objects: 47% (57/121)[K
remote: Counting objects: 48% (59/121)[K
remote: Counting objects: 49% (60/121)[K
remote: Counting objects: 50% (61/121)[K
remote: Counting objects: 51% (62/121)[K
remote: Counting objects: 52% (63/121)[K
remote: Counting objects: 53% (65/121)[K
remote: Counting objects: 54% (66/121)[K
remote: Counting objects: 55% (67/121)[K
remote: Counting objects: 56% (68/121)[K
remote: Counting objects: 57% (69/121)[K
remote: Counting objects: 58% (71/121)[K
remote: Counting objects: 59% (72/121)[K
remote: Counting objects: 60% (73/121)[K
remote: Counting objects: 61% (74/121)[K
remote: Counting objects: 62% (76/121)[K
remote: Counting objects: 63% (77/121)[K
remote: Counting objects: 64% (78/121)[K
remote: Counting objects: 65% (79/121)[K
remote: Counting objects: 66% (80/121)[K
remote: Counting objects: 67% (82/121)[K
remote: Counting objects: 68% (83/121)[K
remote: Counting objects: 69% (84/121)[K
remote: Counting objects: 70% (85/121)[K
remote: Counting objects: 71% (86/121)[K
remote: Counting objects: 72% (88/121)[K
remote: Counting objects: 73% (89/121)[K
remote: Counting objects: 74% (90/121)[K
remote: Counting objects: 75% (91/121)[K
remote: Counting objects: 76% (92/121)[K
remote: Counting objects: 77% (94/121)[K
remote: Counting objects: 78% (95/121)[K
remote: Counting objects: 79% (96/121)[K
remote: Counting objects: 80% (97/121)[K
remote: Counting objects: 81% (99/121)[K
remote: Counting objects: 82% (100/121)[K
remote: Counting objects: 83% (101/121)[K
remote: Counting objects: 84% (102/121)[K
remote: Counting objects: 85% (103/121)[K
remote: Counting objects: 86% (105/121)[K
remote: Counting objects: 87% (106/121)[K
remote: Counting objects: 88% (107/121)[K
remote: Counting objects: 89% (108/121)[K
remote: Counting objects: 90% (109/121)[K
remote: Counting objects: 91% (111/121)[K
remote: Counting objects: 92% (112/121)[K
remote: Counting objects: 93% (113/121)[K
remote: Counting objects: 94% (114/121)[K
remote: Counting objects: 95% (115/121)[K
remote: Counting objects: 96% (117/121)[K
remote: Counting objects: 97% (118/121)[K
remote: Counting objects: 98% (119/121)[K
remote: Counting objects: 99% (120/121)[K
remote: Counting objects: 100% (121/121)[K
remote: Counting objects: 100% (121/121), done.[K
remote: Compressing objects: 1% (1/75)[K
remote: Compressing objects: 2% (2/75)[K
remote: Compressing objects: 4% (3/75)[K
remote: Compressing objects: 5% (4/75)[K
remote: Compressing objects: 6% (5/75)[K
remote: Compressing objects: 8% (6/75)[K
remote: Compressing objects: 9% (7/75)[K
remote: Compressing objects: 10% (8/75)[K
remote: Compressing objects: 12% (9/75)[K
remote: Compressing objects: 13% (10/75)[K
remote: Compressing objects: 14% (11/75)[K
remote: Compressing objects: 16% (12/75)[K
remote: Compressing objects: 17% (13/75)[K
remote: Compressing objects: 18% (14/75)[K
remote: Compressing objects: 20% (15/75)[K
remote: Compressing objects: 21% (16/75)[K
remote: Compressing objects: 22% (17/75)[K
remote: Compressing objects: 24% (18/75)[K
remote: Compressing objects: 25% (19/75)[K
remote: Compressing objects: 26% (20/75)[K
remote: Compressing objects: 28% (21/75)[K
remote: Compressing objects: 29% (22/75)[K
remote: Compressing objects: 30% (23/75)[K
remote: Compressing objects: 32% (24/75)[K
remote: Compressing objects: 33% (25/75)[K
remote: Compressing objects: 34% (26/75)[K
remote: Compressing objects: 36% (27/75)[K
remote: Compressing objects: 37% (28/75)[K
remote: Compressing objects: 38% (29/75)[K
remote: Compressing objects: 40% (30/75)[K
remote: Compressing objects: 41% (31/75)[K
remote: Compressing objects: 42% (32/75)[K
remote: Compressing objects: 44% (33/75)[K
remote: Compressing objects: 45% (34/75)[K
remote: Compressing objects: 46% (35/75)[K
remote: Compressing objects: 48% (36/75)[K
remote: Compressing objects: 49% (37/75)[K
remote: Compressing objects: 50% (38/75)[K
remote: Compressing objects: 52% (39/75)[K
remote: Compressing objects: 53% (40/75)[K
remote: Compressing objects: 54% (41/75)[K
remote: Compressing objects: 56% (42/75)[K
remote: Compressing objects: 57% (43/75)[K
remote: Compressing objects: 58% (44/75)[K
remote: Compressing objects: 60% (45/75)[K
remote: Compressing objects: 61% (46/75)[K
remote: Compressing objects: 62% (47/75)[K
remote: Compressing objects: 64% (48/75)[K
remote: Compressing objects: 65% (49/75)[K
remote: Compressing objects: 66% (50/75)[K
remote: Compressing objects: 68% (51/75)[K
remote: Compressing objects: 69% (52/75)[K
remote: Compressing objects: 70% (53/75)[K
remote: Compressing objects: 72% (54/75)[K
remote: Compressing objects: 73% (55/75)[K
remote: Compressing objects: 74% (56/75)[K
remote: Compressing objects: 76% (57/75)[K
remote: Compressing objects: 77% (58/75)[K
remote: Compressing objects: 78% (59/75)[K
remote: Compressing objects: 80% (60/75)[K
remote: Compressing objects: 81% (61/75)[K
remote: Compressing objects: 82% (62/75)[K
remote: Compressing objects: 84% (63/75)[K
remote: Compressing objects: 85% (64/75)[K
remote: Compressing objects: 86% (65/75)[K
remote: Compressing objects: 88% (66/75)[K
remote: Compressing objects: 89% (67/75)[K
remote: Compressing objects: 90% (68/75)[K
remote: Compressing objects: 92% (69/75)[K
remote: Compressing objects: 93% (70/75)[K
remote: Compressing objects: 94% (71/75)[K
remote: Compressing objects: 96% (72/75)[K
remote: Compressing objects: 97% (73/75)[K
remote: Compressing objects: 98% (74/75)[K
remote: Compressing objects: 100% (75/75)[K
remote: Compressing objects: 100% (75/75), done.[K
Receiving objects: 0% (1/212)
Receiving objects: 1% (3/212)
Receiving objects: 2% (5/212), 1.57 MiB | 3.10 MiB/s
Receiving objects: 2% (6/212), 3.53 MiB | 3.44 MiB/s
Receiving objects: 3% (7/212), 3.53 MiB | 3.44 MiB/s
Receiving objects: 4% (9/212), 3.53 MiB | 3.44 MiB/s
Receiving objects: 5% (11/212), 3.53 MiB | 3.44 MiB/s
Receiving objects: 6% (13/212), 3.53 MiB | 3.44 MiB/s
Receiving objects: 7% (15/212), 3.53 MiB | 3.44 MiB/s
Receiving objects: 8% (17/212), 3.53 MiB | 3.44 MiB/s
Receiving objects: 9% (20/212), 3.53 MiB | 3.44 MiB/s
Receiving objects: 10% (22/212), 5.48 MiB | 3.55 MiB/s
Receiving objects: 11% (24/212), 5.48 MiB | 3.55 MiB/s
Receiving objects: 12% (26/212), 5.48 MiB | 3.55 MiB/s
Receiving objects: 13% (28/212), 5.48 MiB | 3.55 MiB/s
Receiving objects: 14% (30/212), 5.48 MiB | 3.55 MiB/s
Receiving objects: 15% (32/212), 5.48 MiB | 3.55 MiB/s
Receiving objects: 16% (34/212), 5.48 MiB | 3.55 MiB/s
Receiving objects: 17% (37/212), 5.48 MiB | 3.55 MiB/s
Receiving objects: 18% (39/212), 5.48 MiB | 3.55 MiB/s
Receiving objects: 19% (41/212), 5.48 MiB | 3.55 MiB/s
Receiving objects: 20% (43/212), 5.48 MiB | 3.55 MiB/s
Receiving objects: 21% (45/212), 5.48 MiB | 3.55 MiB/s
Receiving objects: 22% (47/212), 5.48 MiB | 3.55 MiB/s
Receiving objects: 23% (49/212), 5.48 MiB | 3.55 MiB/s
Receiving objects: 24% (51/212), 5.48 MiB | 3.55 MiB/s
Receiving objects: 25% (53/212), 5.48 MiB | 3.55 MiB/s
Receiving objects: 26% (56/212), 5.48 MiB | 3.55 MiB/s
Receiving objects: 27% (58/212), 5.48 MiB | 3.55 MiB/s
Receiving objects: 28% (60/212), 5.48 MiB | 3.55 MiB/s
Receiving objects: 29% (62/212), 7.41 MiB | 3.63 MiB/s
Receiving objects: 30% (64/212), 7.41 MiB | 3.63 MiB/s
Receiving objects: 31% (66/212), 7.41 MiB | 3.63 MiB/s
Receiving objects: 32% (68/212), 7.41 MiB | 3.63 MiB/s
Receiving objects: 33% (70/212), 7.41 MiB | 3.63 MiB/s
Receiving objects: 34% (73/212), 7.41 MiB | 3.63 MiB/s
Receiving objects: 35% (75/212), 7.41 MiB | 3.63 MiB/s
Receiving objects: 36% (77/212), 7.41 MiB | 3.63 MiB/s
Receiving objects: 37% (79/212), 7.41 MiB | 3.63 MiB/s
Receiving objects: 38% (81/212), 7.41 MiB | 3.63 MiB/s
Receiving objects: 39% (83/212), 7.41 MiB | 3.63 MiB/s
Receiving objects: 40% (85/212), 9.27 MiB | 3.64 MiB/s
Receiving objects: 41% (87/212), 9.27 MiB | 3.64 MiB/s
Receiving objects: 42% (90/212), 9.27 MiB | 3.64 MiB/s
Receiving objects: 43% (92/212), 9.27 MiB | 3.64 MiB/s
Receiving objects: 44% (94/212), 9.27 MiB | 3.64 MiB/s
Receiving objects: 45% (96/212), 9.27 MiB | 3.64 MiB/s
Receiving objects: 46% (98/212), 9.27 MiB | 3.64 MiB/s
Receiving objects: 47% (100/212), 9.27 MiB | 3.64 MiB/s
Receiving objects: 48% (102/212), 9.27 MiB | 3.64 MiB/s
Receiving objects: 49% (104/212), 9.27 MiB | 3.64 MiB/s
Receiving objects: 50% (106/212), 9.27 MiB | 3.64 MiB/s
Receiving objects: 51% (109/212), 9.27 MiB | 3.64 MiB/s
Receiving objects: 52% (111/212), 9.27 MiB | 3.64 MiB/s
Receiving objects: 53% (113/212), 9.27 MiB | 3.64 MiB/s
Receiving objects: 54% (115/212), 9.27 MiB | 3.64 MiB/s
Receiving objects: 55% (117/212), 9.27 MiB | 3.64 MiB/s
Receiving objects: 56% (119/212), 9.27 MiB | 3.64 MiB/s
Receiving objects: 57% (121/212), 9.27 MiB | 3.64 MiB/s
Receiving objects: 58% (123/212), 9.27 MiB | 3.64 MiB/s
Receiving objects: 59% (126/212), 9.27 MiB | 3.64 MiB/s
Receiving objects: 60% (128/212), 9.27 MiB | 3.64 MiB/s
Receiving objects: 61% (130/212), 9.27 MiB | 3.64 MiB/s
Receiving objects: 62% (132/212), 9.27 MiB | 3.64 MiB/s
Receiving objects: 63% (134/212), 9.27 MiB | 3.64 MiB/s
Receiving objects: 64% (136/212), 9.27 MiB | 3.64 MiB/s
Receiving objects: 65% (138/212), 9.27 MiB | 3.64 MiB/s
Receiving objects: 66% (140/212), 9.27 MiB | 3.64 MiB/s
Receiving objects: 67% (143/212), 9.27 MiB | 3.64 MiB/s
Receiving objects: 68% (145/212), 9.27 MiB | 3.64 MiB/s
Receiving objects: 69% (147/212), 9.27 MiB | 3.64 MiB/s
Receiving objects: 70% (149/212), 9.27 MiB | 3.64 MiB/s
Receiving objects: 71% (151/212), 9.27 MiB | 3.64 MiB/s
Receiving objects: 72% (153/212), 9.27 MiB | 3.64 MiB/s
Receiving objects: 73% (155/212), 9.27 MiB | 3.64 MiB/s
Receiving objects: 74% (157/212), 9.27 MiB | 3.64 MiB/s
Receiving objects: 75% (159/212), 9.27 MiB | 3.64 MiB/s
Receiving objects: 76% (162/212), 9.27 MiB | 3.64 MiB/s
remote: Total 212 (delta 98), reused 56 (delta 46), pack-reused 91[K
Receiving objects: 77% (164/212), 9.27 MiB | 3.64 MiB/s
Receiving objects: 78% (166/212), 9.27 MiB | 3.64 MiB/s
Receiving objects: 79% (168/212), 9.27 MiB | 3.64 MiB/s
Receiving objects: 80% (170/212), 9.27 MiB | 3.64 MiB/s
Receiving objects: 81% (172/212), 9.27 MiB | 3.64 MiB/s
Receiving objects: 82% (174/212), 9.27 MiB | 3.64 MiB/s
Receiving objects: 83% (176/212), 9.27 MiB | 3.64 MiB/s
Receiving objects: 84% (179/212), 9.27 MiB | 3.64 MiB/s
Receiving objects: 85% (181/212), 9.27 MiB | 3.64 MiB/s
Receiving objects: 86% (183/212), 9.27 MiB | 3.64 MiB/s
Receiving objects: 87% (185/212), 9.27 MiB | 3.64 MiB/s
Receiving objects: 88% (187/212), 9.27 MiB | 3.64 MiB/s
Receiving objects: 89% (189/212), 9.27 MiB | 3.64 MiB/s
Receiving objects: 90% (191/212), 9.27 MiB | 3.64 MiB/s
Receiving objects: 91% (193/212), 9.27 MiB | 3.64 MiB/s
Receiving objects: 92% (196/212), 9.27 MiB | 3.64 MiB/s
Receiving objects: 93% (198/212), 9.27 MiB | 3.64 MiB/s
Receiving objects: 94% (200/212), 9.27 MiB | 3.64 MiB/s
Receiving objects: 95% (202/212), 9.27 MiB | 3.64 MiB/s
Receiving objects: 96% (204/212), 9.27 MiB | 3.64 MiB/s
Receiving objects: 97% (206/212), 9.27 MiB | 3.64 MiB/s
Receiving objects: 98% (208/212), 9.27 MiB | 3.64 MiB/s
Receiving objects: 99% (210/212), 9.27 MiB | 3.64 MiB/s
Receiving objects: 100% (212/212), 9.27 MiB | 3.64 MiB/s
Receiving objects: 100% (212/212), 9.31 MiB | 3.64 MiB/s, done.
Resolving deltas: 0% (0/104)
Resolving deltas: 3% (4/104)
Resolving deltas: 41% (43/104)
Resolving deltas: 46% (48/104)
Resolving deltas: 50% (52/104)
Resolving deltas: 86% (90/104)
Resolving deltas: 89% (93/104)
Resolving deltas: 95% (99/104)
Resolving deltas: 97% (101/104)
Resolving deltas: 98% (102/104)
Resolving deltas: 99% (103/104)
Resolving deltas: 100% (104/104)
Resolving deltas: 100% (104/104), done.
必要なパッケージをインストール
%pip uninstall -q -y openvino-dev openvino openvino-nightly
%pip install -q --extra-index-url https://download.pytorch.org/whl/cpu\
transformers diffusers gradio openvino-nightly torchvision
WARNING: Skipping openvino-dev as it is not installed.
Note: you may need to restart the kernel to use updated packages.
DEPRECATION: pytorch-lightning 1.6.5 has a non-standard dependency specifier torch>=1.8.*. pip 24.1 will enforce this behaviour change. A possible replacement is to upgrade to a newer version of pytorch-lightning or contact the author to suggest that they release a version with a conforming dependency specifiers. Discussion can be found at https://github.com/pypa/pip/issues/12063
Note: you may need to restart the kernel to use updated packages.
PyTorch モデルの準備
adapter_id = "TencentARC/PhotoMaker"
base_model_id = "SG161222/RealVisXL_V3.0"
TEXT_ENCODER_OV_PATH = Path("model/text_encoder.xml")
TEXT_ENCODER_2_OV_PATH = Path("model/text_encoder_2.xml")
UNET_OV_PATH = Path("model/unet.xml")
ID_ENCODER_OV_PATH = Path("model/id_encoder.xml")
VAE_DECODER_OV_PATH = Path("model/vae_decoder.xml")
元のパイプラインをロードし、変換用のモデルを準備¶
各 PyTorch モデルをエクスポートするには、Hugging Face Hub から ID エンコーダーの重み、LoRa
の重みをダウンロードし、PhotoMaker のリポジトリーから PhotoMakerStableDiffusionXLPipeline
オブジェクトを使用して、元の PhotoMaker パイプラインを生成します。
import torch
import numpy as np
import os
from PIL import Image
from pathlib import Path
from PhotoMaker.photomaker.model import PhotoMakerIDEncoder
from PhotoMaker.photomaker.pipeline import PhotoMakerStableDiffusionXLPipeline
from diffusers import EulerDiscreteScheduler
import gc
trigger_word = "img"
def load_original_pytorch_pipeline_components(photomaker_path: str, base_model_id: str):
# Load base model
pipe = PhotoMakerStableDiffusionXLPipeline.from_pretrained(
base_model_id, use_safetensors=True
).to("cpu")
# Load PhotoMaker checkpoint
pipe.load_photomaker_adapter(
os.path.dirname(photomaker_path),
subfolder="",
weight_name=os.path.basename(photomaker_path),
trigger_word=trigger_word,
)
pipe.scheduler = EulerDiscreteScheduler.from_config(pipe.scheduler.config)
pipe.fuse_lora()
gc.collect()
return pipe
2024-02-10 01:01:11.344416: I tensorflow/core/util/port.cc:110] oneDNN custom operations are on. You may see slightly different numerical results due to floating-point round-off errors from different computation orders. To turn them off, set the environment variable TF_ENABLE_ONEDNN_OPTS=0. 2024-02-10 01:01:11.379661: I tensorflow/core/platform/cpu_feature_guard.cc:182] This TensorFlow binary is optimized to use available CPU instructions in performance-critical operations. To enable the following instructions: AVX2 AVX512F AVX512_VNNI FMA, in other operations, rebuild TensorFlow with the appropriate compiler flags.
2024-02-10 01:01:12.029266: W tensorflow/compiler/tf2tensorrt/utils/py_utils.cc:38] TF-TRT Warning: Could not find TensorRT
/opt/home/k8sworker/ci-ai/cibuilds/ov-notebook/OVNotebookOps-609/.workspace/scm/ov-notebook/.venv/lib/python3.8/site-packages/diffusers/utils/outputs.py:63: UserWarning: torch.utils._pytree._register_pytree_node is deprecated. Please use torch.utils._pytree.register_pytree_node instead.
torch.utils._pytree._register_pytree_node(
from huggingface_hub import hf_hub_download
photomaker_path = hf_hub_download(
repo_id=adapter_id, filename="photomaker-v1.bin", repo_type="model"
)
pipe = load_original_pytorch_pipeline_components(
photomaker_path, base_model_id
)
Loading pipeline components...: 0%| | 0/7 [00:00<?, ?it/s]
Loading PhotoMaker components [1] id_encoder from [/opt/home/k8sworker/.cache/huggingface/hub/models--TencentARC--PhotoMaker/snapshots/3602d02ba7cc99ce8886e24063ed10e4f2510c84]...
Loading PhotoMaker components [2] lora_weights from [/opt/home/k8sworker/.cache/huggingface/hub/models--TencentARC--PhotoMaker/snapshots/3602d02ba7cc99ce8886e24063ed10e4f2510c84]
/opt/home/k8sworker/ci-ai/cibuilds/ov-notebook/OVNotebookOps-609/.workspace/scm/ov-notebook/.venv/lib/python3.8/site-packages/diffusers/loaders/lora.py:1078: FutureWarning: fuse_text_encoder_lora is deprecated and will be removed in version 0.27. You are using an old version of LoRA backend. This will be deprecated in the next releases in favor of PEFT make sure to install the latest PEFT and transformers packages in the future. deprecate("fuse_text_encoder_lora", "0.27", LORA_DEPRECATION_MESSAGE)
モデルを OpenVINO 中間表現 (IR) 形式に変換¶
2023.0 リリース以降、OpenVINO は PyTorch モデルの変換を直接サポートするようになりました。OpenVINO ov.Model
オブジェクト・インスタンスを取得するには、モデル・オブジェクト、モデルトレース用の入力データを ov.convert_model
関数に提供する必要があります。ov.save_model
関数を使用して、次回のデプロイのためにモデルをディスクに保存できます。
パイプラインは 5 つの重要なパーツで構成されます。
画像のアノテーションによって条件付けする画像埋め込みを生成する ID エンコーダー。
テキスト・エンコーダーは、テキストプロンプトから画像を生成するテキスト埋め込みを作成します。
段階的にノイズを除去する潜像表現のための Unet。
潜在空間を画像にデコードするオートエンコーダー (VAE)。
import openvino as ov
def flattenize_inputs(inputs):
"""
Helper function for resolve nested input structure (e.g. lists or tuples of tensors)
"""
flatten_inputs = []
for input_data in inputs:
if input_data is None:
continue
if isinstance(input_data, (list, tuple)):
flatten_inputs.extend(flattenize_inputs(input_data))
else:
flatten_inputs.append(input_data)
return flatten_inputs
dtype_mapping = {
torch.float32: ov.Type.f32,
torch.float64: ov.Type.f64,
torch.int32: ov.Type.i32,
torch.int64: ov.Type.i64,
torch.bool: ov.Type.boolean,
}
def prepare_input_info(input_dict):
"""
Helper function for preparing input info (shapes and data types) for conversion based on example inputs
"""
flatten_inputs = flattenize_inputs(input_dict.values())
input_info = []
for input_data in flatten_inputs:
updated_shape = list(input_data.shape)
if input_data.ndim == 5:
updated_shape[1] = -1
input_info.append((dtype_mapping[input_data.dtype], updated_shape))
return input_info
def convert(model: torch.nn.Module, xml_path: str, example_input, input_info):
"""
Helper function for converting PyTorch model to OpenVINO IR
"""
xml_path = Path(xml_path)
if not xml_path.exists():
xml_path.parent.mkdir(parents=True, exist_ok=True)
with torch.no_grad():
ov_model = ov.convert_model(
model, example_input=example_input, input=input_info
)
ov.save_model(ov_model, xml_path)
del ov_model
torch._C._jit_clear_class_registry()
torch.jit._recursive.concrete_type_store = torch.jit._recursive.ConcreteTypeStore()
torch.jit._state._clear_class_state()
ID エンコーダー¶
PhotoMaker は、画像エンコーダとフューズモジュールを統合して ID エンコーダーを作成しました。これは、U-Net モデルの入力となるテキスト・エンコーダーの出力 (テキスト埋め込み) を更新する画像埋め込みを生成するのに使用されます。
id_encoder = pipe.id_encoder
id_encoder.eval()
def create_bool_tensor(*size):
new_tensor = torch.zeros((size), dtype=torch.bool)
return new_tensor
inputs = {
"id_pixel_values": torch.randn((1, 1, 3, 224, 224)),
"prompt_embeds": torch.randn((1, 77, 2048)),
"class_tokens_mask": create_bool_tensor(1, 77),
}
input_info = prepare_input_info(inputs)
convert(id_encoder, ID_ENCODER_OV_PATH, inputs, input_info)
del id_encoder
gc.collect()
WARNING:tensorflow:Please fix your imports. Module tensorflow.python.training.tracking.base has been moved to tensorflow.python.trackable.base. The old module will be deleted in version 2.11.
[ WARNING ] Please fix your imports. Module %s has been moved to %s. The old module will be deleted in version %s.
/opt/home/k8sworker/ci-ai/cibuilds/ov-notebook/OVNotebookOps-609/.workspace/scm/ov-notebook/.venv/lib/python3.8/site-packages/transformers/models/clip/modeling_clip.py:273: TracerWarning: Converting a tensor to a Python boolean might cause the trace to be incorrect. We can't record the data flow of Python values, so this value will be treated as a constant in the future. This means that the trace might not generalize to other inputs!
if attn_weights.size() != (bsz * self.num_heads, tgt_len, src_len):
/opt/home/k8sworker/ci-ai/cibuilds/ov-notebook/OVNotebookOps-609/.workspace/scm/ov-notebook/.venv/lib/python3.8/site-packages/transformers/models/clip/modeling_clip.py:313: TracerWarning: Converting a tensor to a Python boolean might cause the trace to be incorrect. We can't record the data flow of Python values, so this value will be treated as a constant in the future. This means that the trace might not generalize to other inputs!
if attn_output.size() != (bsz * self.num_heads, tgt_len, self.head_dim):
/opt/home/k8sworker/ci-ai/cibuilds/ov-notebook/OVNotebookOps-609/.workspace/scm/ov-notebook/notebooks/283-photo-maker/PhotoMaker/photomaker/model.py:84: TracerWarning: Converting a tensor to a Python boolean might cause the trace to be incorrect. We can't record the data flow of Python values, so this value will be treated as a constant in the future. This means that the trace might not generalize to other inputs!
assert class_tokens_mask.sum() == stacked_id_embeds.shape[0], f"{class_tokens_mask.sum()} != {stacked_id_embeds.shape[0]}"
1919
テキスト・エンコーダー¶
テキスト・エンコーダーは、入力プロンプト (例えば、“馬に乗った宇宙飛行士の写真”) を、U-Net が理解できる埋め込みスペースに変換する役割を果たします。これは通常、入力トークンのシーケンスを潜在テキスト埋め込みのシーケンスにマッピングする単純なトランスフォーマー・ベースのエンコーダーです。
text_encoder = pipe.text_encoder
text_encoder.eval()
text_encoder_2 = pipe.text_encoder_2
text_encoder_2.eval()
text_encoder.config.output_hidden_states = True
text_encoder.config.return_dict = False
text_encoder_2.config.output_hidden_states = True
text_encoder_2.config.return_dict = False
inputs = {
"input_ids": torch.ones((1, 77), dtype=torch.long)
}
input_info = prepare_input_info(inputs)
convert(text_encoder, TEXT_ENCODER_OV_PATH, inputs, input_info)
convert(text_encoder_2, TEXT_ENCODER_2_OV_PATH, inputs, input_info)
del text_encoder
del text_encoder_2
gc.collect()
/opt/home/k8sworker/ci-ai/cibuilds/ov-notebook/OVNotebookOps-609/.workspace/scm/ov-notebook/.venv/lib/python3.8/site-packages/transformers/modeling_attn_mask_utils.py:86: TracerWarning: Converting a tensor to a Python boolean might cause the trace to be incorrect. We can't record the data flow of Python values, so this value will be treated as a constant in the future. This means that the trace might not generalize to other inputs!
if input_shape[-1] > 1 or self.sliding_window is not None:
/opt/home/k8sworker/ci-ai/cibuilds/ov-notebook/OVNotebookOps-609/.workspace/scm/ov-notebook/.venv/lib/python3.8/site-packages/transformers/modeling_attn_mask_utils.py:162: TracerWarning: Converting a tensor to a Python boolean might cause the trace to be incorrect. We can't record the data flow of Python values, so this value will be treated as a constant in the future. This means that the trace might not generalize to other inputs!
if past_key_values_length > 0:
/opt/home/k8sworker/ci-ai/cibuilds/ov-notebook/OVNotebookOps-609/.workspace/scm/ov-notebook/.venv/lib/python3.8/site-packages/transformers/models/clip/modeling_clip.py:281: TracerWarning: Converting a tensor to a Python boolean might cause the trace to be incorrect. We can't record the data flow of Python values, so this value will be treated as a constant in the future. This means that the trace might not generalize to other inputs!
if causal_attention_mask.size() != (bsz, 1, tgt_len, src_len):
3376
U-Net¶
U-Net モデル変換のプロセスは、元の Stable Diffusion XL モデルと同様です。
unet = pipe.unet
unet.eval()
class UnetWrapper(torch.nn.Module):
def __init__(self, unet):
super().__init__()
self.unet = unet
def forward(
self,
sample=None,
timestep=None,
encoder_hidden_states=None,
text_embeds=None,
time_ids=None,
):
return self.unet.forward(
sample,
timestep,
encoder_hidden_states,
added_cond_kwargs={"text_embeds": text_embeds, "time_ids": time_ids},
)
inputs = {
"sample": torch.rand([2, 4, 128, 128], dtype=torch.float32),
"timestep": torch.from_numpy(np.array(1, dtype=float)),
"encoder_hidden_states": torch.rand([2, 77, 2048], dtype=torch.float32),
"text_embeds": torch.rand([2, 1280], dtype=torch.float32),
"time_ids": torch.rand([2, 6], dtype=torch.float32),
}
input_info = prepare_input_info(inputs)
w_unet = UnetWrapper(unet)
convert(w_unet, UNET_OV_PATH, inputs, input_info)
del w_unet, unet
gc.collect()
/opt/home/k8sworker/ci-ai/cibuilds/ov-notebook/OVNotebookOps-609/.workspace/scm/ov-notebook/.venv/lib/python3.8/site-packages/diffusers/models/unets/unet_2d_condition.py:924: TracerWarning: Converting a tensor to a Python boolean might cause the trace to be incorrect. We can't record the data flow of Python values, so this value will be treated as a constant in the future. This means that the trace might not generalize to other inputs!
if dim % default_overall_up_factor != 0:
/opt/home/k8sworker/ci-ai/cibuilds/ov-notebook/OVNotebookOps-609/.workspace/scm/ov-notebook/.venv/lib/python3.8/site-packages/diffusers/models/downsampling.py:135: TracerWarning: Converting a tensor to a Python boolean might cause the trace to be incorrect. We can't record the data flow of Python values, so this value will be treated as a constant in the future. This means that the trace might not generalize to other inputs!
assert hidden_states.shape[1] == self.channels
/opt/home/k8sworker/ci-ai/cibuilds/ov-notebook/OVNotebookOps-609/.workspace/scm/ov-notebook/.venv/lib/python3.8/site-packages/diffusers/models/downsampling.py:144: TracerWarning: Converting a tensor to a Python boolean might cause the trace to be incorrect. We can't record the data flow of Python values, so this value will be treated as a constant in the future. This means that the trace might not generalize to other inputs!
assert hidden_states.shape[1] == self.channels
/opt/home/k8sworker/ci-ai/cibuilds/ov-notebook/OVNotebookOps-609/.workspace/scm/ov-notebook/.venv/lib/python3.8/site-packages/diffusers/models/upsampling.py:149: TracerWarning: Converting a tensor to a Python boolean might cause the trace to be incorrect. We can't record the data flow of Python values, so this value will be treated as a constant in the future. This means that the trace might not generalize to other inputs!
assert hidden_states.shape[1] == self.channels
/opt/home/k8sworker/ci-ai/cibuilds/ov-notebook/OVNotebookOps-609/.workspace/scm/ov-notebook/.venv/lib/python3.8/site-packages/diffusers/models/upsampling.py:165: TracerWarning: Converting a tensor to a Python boolean might cause the trace to be incorrect. We can't record the data flow of Python values, so this value will be treated as a constant in the future. This means that the trace might not generalize to other inputs!
if hidden_states.shape[0] >= 64:
11629
VAE デコーダー¶
VAE モデルには、エンコーダーとデコーダーの 2 つのパーツがあります。エンコーダーは、画像を低次元の潜在表現に変換するのに使用され、これが U-Net モデルの入力となります。逆に、デコーダーは潜在表現を変換して画像に戻します。
Text-to-Image パイプラインを実行すると、VAE デコーダーのみが必要であることが分かります。
vae_decoder = pipe.vae
vae_decoder.eval()
class VAEDecoderWrapper(torch.nn.Module):
def __init__(self, vae_decoder):
super().__init__()
self.vae = vae_decoder
def forward(self, latents):
return self.vae.decode(latents)
w_vae_decoder = VAEDecoderWrapper(vae_decoder)
inputs = torch.zeros((1, 4, 128, 128))
convert(w_vae_decoder, VAE_DECODER_OV_PATH, inputs, input_info=[1, 4, 128, 128])
del w_vae_decoder, vae_decoder
gc.collect()
1534
推論パイプラインの準備¶
この例では、PhotoMakerStableDiffusionXLPipeline
パイプラインを再利用して OpenVINO で画像を生成するため、このパイプライン内の各モデルのオブジェクトを新しい OpenVINO モデル・オブジェクトに置き換える必要があります。
Stable Diffusion パイプライン用の推論デバイスの選択¶
import ipywidgets as widgets
core = ov.Core()
device = widgets.Dropdown(
options=core.available_devices + ["AUTO"],
value="CPU",
description="Device:",
disabled=False,
)
device
Dropdown(description='Device:', options=('CPU', 'AUTO'), value='CPU')
モデルをコンパイルして推論用のラッパーを作成¶
元の PhotoMaker ワークフローにアクセスするには、OpenVINO コンパイル済みモデルごとに新しいラッパーを作成する必要があります。元のパイプラインと一致させるには、OpenVINO モデルラッパーの属性の一部を元のモデル・オブジェクトから再利用し、推論出力を numpy から torch.tensor
に変換する必要があります。
compiled_id_encoder = core.compile_model(ID_ENCODER_OV_PATH, device.value)
compiled_unet = core.compile_model(UNET_OV_PATH, device.value)
compiled_text_encoder = core.compile_model(TEXT_ENCODER_OV_PATH, device.value)
compiled_text_encoder_2 = core.compile_model(TEXT_ENCODER_2_OV_PATH, device.value)
compiled_vae_decoder = core.compile_model(VAE_DECODER_OV_PATH, device.value)
from collections import namedtuple
class OVIDEncoderWrapper(PhotoMakerIDEncoder):
dtype = torch.float32 # accessed in the original workflow
def __init__(self, id_encoder, orig_id_encoder):
super().__init__()
self.id_encoder = id_encoder
self.modules = orig_id_encoder.modules # accessed in the original workflow
self.config = orig_id_encoder.config # accessed in the original workflow
def __call__(
self,
*args,
):
id_pixel_values, prompt_embeds, class_tokens_mask = args
inputs = {
"id_pixel_values": id_pixel_values,
"prompt_embeds": prompt_embeds,
"class_tokens_mask": class_tokens_mask,
}
output = self.id_encoder(inputs)[0]
return torch.from_numpy(output)
class OVTextEncoderWrapper:
dtype = torch.float32 # accessed in the original workflow
def __init__(self, text_encoder, orig_text_encoder):
self.text_encoder = text_encoder
self.modules = orig_text_encoder.modules # accessed in the original workflow
self.config = orig_text_encoder.config # accessed in the original workflow
def __call__(self, input_ids, **kwargs):
inputs = {"input_ids": input_ids}
output = self.text_encoder(inputs)
hidden_states = []
hidden_states_len = len(output)
for i in range(1, hidden_states_len):
hidden_states.append(torch.from_numpy(output[i]))
BaseModelOutputWithPooling = namedtuple(
"BaseModelOutputWithPooling", "last_hidden_state hidden_states"
)
output = BaseModelOutputWithPooling(torch.from_numpy(output[0]), hidden_states)
return output
class OVUnetWrapper:
def __init__(self, unet, unet_orig):
self.unet = unet
self.config = unet_orig.config # accessed in the original workflow
self.add_embedding = (
unet_orig.add_embedding
) # accessed in the original workflow
def __call__(self, *args, **kwargs):
latent_model_input, t = args
inputs = {
"sample": latent_model_input,
"timestep": t,
"encoder_hidden_states": kwargs["encoder_hidden_states"],
"text_embeds": kwargs["added_cond_kwargs"]["text_embeds"],
"time_ids": kwargs["added_cond_kwargs"]["time_ids"],
}
output = self.unet(inputs)
return [torch.from_numpy(output[0])]
class OVVAEDecoderWrapper:
dtype = torch.float32 # accessed in the original workflow
def __init__(self, vae, vae_orig):
self.vae = vae
self.config = vae_orig.config # accessed in the original workflow
def decode(self, latents, return_dict=False):
output = self.vae(latents)[0]
output = torch.from_numpy(output)
return [output]
元のパイプラインの PyTorch モデル・オブジェクトを OpenVINO モデルに置き換えます。
pipe.id_encoder = OVIDEncoderWrapper(compiled_id_encoder, pipe.id_encoder)
pipe.unet = OVUnetWrapper(compiled_unet, pipe.unet)
pipe.text_encoder = OVTextEncoderWrapper(compiled_text_encoder, pipe.text_encoder)
pipe.text_encoder_2 = OVTextEncoderWrapper(compiled_text_encoder_2, pipe.text_encoder_2)
pipe.vae = OVVAEDecoderWrapper(compiled_vae_decoder, pipe.vae)
OpenVINO を使用してテキストから画像への生成を実行¶
from diffusers.utils import load_image
prompt = "sci-fi, closeup portrait photo of a man img in Iron man suit, face"
negative_prompt = "(asymmetry, worst quality, low quality, illustration, 3d, 2d, painting, cartoons, sketch), open mouth"
generator = torch.Generator("cpu").manual_seed(42)
input_id_images = []
original_image = load_image("./PhotoMaker/examples/newton_man/newton_0.jpg")
input_id_images.append(original_image)
## Parameter setting
num_steps = 20
style_strength_ratio = 20
start_merge_step = int(float(style_strength_ratio) / 100 * num_steps)
if start_merge_step > 30:
start_merge_step = 30
images = pipe(
prompt=prompt,
input_id_images=input_id_images,
negative_prompt=negative_prompt,
num_images_per_prompt=1,
num_inference_steps=num_steps,
start_merge_step=start_merge_step,
generator=generator,
).images
0%| | 0/20 [00:00<?, ?it/s]
import matplotlib.pyplot as plt
def visualize_results(orig_img: Image.Image, output_img: Image.Image):
"""
Helper function for pose estimationresults visualization
Parameters:
orig_img (Image.Image): original image
output_img (Image.Image): processed image with PhotoMaker
Returns:
fig (matplotlib.pyplot.Figure): matplotlib generated figure
"""
orig_img = orig_img.resize(output_img.size)
orig_title = "Original image"
output_title = "Output image"
im_w, im_h = orig_img.size
is_horizontal = im_h < im_w
fig, axs = plt.subplots(
2 if is_horizontal else 1,
1 if is_horizontal else 2,
sharex="all",
sharey="all",
)
fig.suptitle(f"Prompt: '{prompt}'", fontweight="bold")
fig.patch.set_facecolor("white")
list_axes = list(axs.flat)
for a in list_axes:
a.set_xticklabels([])
a.set_yticklabels([])
a.get_xaxis().set_visible(False)
a.get_yaxis().set_visible(False)
a.grid(False)
list_axes[0].imshow(np.array(orig_img))
list_axes[1].imshow(np.array(output_img))
list_axes[0].set_title(orig_title, fontsize=15)
list_axes[1].set_title(output_title, fontsize=15)
fig.subplots_adjust(
wspace=0.01 if is_horizontal else 0.00, hspace=0.01 if is_horizontal else 0.1
)
fig.tight_layout()
return fig
fig = visualize_results(original_image, images[0])
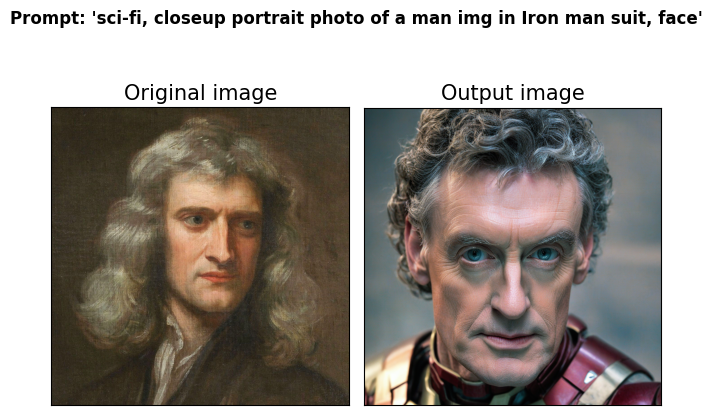
インタラクティブなデモ¶
import gradio as gr
def generate_from_text(
text_promt, input_image, neg_prompt, seed, num_steps, style_strength_ratio
):
"""
Helper function for generating result image from prompt text
Parameters:
text_promt (String): positive prompt
input_image (Image.Image): original image
neg_prompt (String): negative prompt
seed (Int): seed for random generator state initialization
num_steps (Int): number of sampling steps
style_strength_ratio (Int): the percentage of step when merging the ID embedding to text embedding
Returns:
result (Image.Image): generation result
"""
start_merge_step = int(float(style_strength_ratio) / 100 * num_steps)
if start_merge_step > 30:
start_merge_step = 30
result = pipe(
text_promt,
input_id_images=input_image,
negative_prompt=neg_prompt,
num_inference_steps=num_steps,
num_images_per_prompt=1,
start_merge_step=start_merge_step,
generator=torch.Generator().manual_seed(seed),
height=1024,
width=1024,
).images[0]
return result
with gr.Blocks() as demo:
with gr.Column():
with gr.Row():
input_image = gr.Image(label="Your image", sources=[
"upload"], type="pil")
output_image = gr.Image(label="Generated Images", type="pil")
positive_input = gr.Textbox(
label=f"Text prompt, Trigger words is '{trigger_word}'")
neg_input = gr.Textbox(label="Negative prompt")
with gr.Row():
seed_input = gr.Slider(0, 10_000_000, value=42, label="Seed")
steps_input = gr.Slider(
label="Steps", value=10, minimum=5, maximum=50, step=1
)
style_strength_ratio_input = gr.Slider(
label="Style strength ratio", value=20, minimum=5, maximum=100, step=5
)
btn = gr.Button()
btn.click(
generate_from_text,
[
positive_input,
input_image,
neg_input,
seed_input,
steps_input,
style_strength_ratio_input,
],
output_image,
)
gr.Examples(
[
[prompt, negative_prompt],
[
"A woman img wearing a Christmas hat",
negative_prompt,
],
[
"A man img in a helmet and vest riding a motorcycle",
negative_prompt,
],
[
"photo of a middle-aged man img sitting on a plush leather couch, and watching television show",
negative_prompt,
],
[
"photo of a skilled doctor img in a pristine white lab coat enjoying a delicious meal in a sophisticated dining room",
negative_prompt,
],
[
"photo of superman img flying through a vibrant sunset sky, with his cape billowing in the wind",
negative_prompt,
],
],
[positive_input, neg_input],
)
demo.queue().launch()
# if you are launching remotely, specify server_name and server_port
# demo.launch(server_name='your server name', server_port='server port in int')
# Read more in the docs: https://gradio.app/docs/
Running on local URL: http://127.0.0.1:7860 To create a public link, set share=True in launch().
demo.close()
Closing server running on port: 7860