Kosmos-2: マルチモーダル大規模言語モデルと OpenVINO¶
この Jupyter ノートブックは、ローカルへのインストール後にのみ起動できます。
KOSMOS-2 は、マルチモーダルなグラウンディングと参照の新しい機能を備えたマルチモーダル大規模言語モデル (MLLM) です。KOSMOS-2 は、マルチモーダル入力を理解し、指示に従い、オブジェクトの説明 (境界ボックスなど) を理解し、視覚世界に対するグラウンド言語を認識できます。
マルチモーダル大規模言語モデル (MLLM) は、言語、視覚、視覚言語タスクなど、幅広いタスクにわたって汎用インターフェイスとしての役割を果たします。MLLM は、テキスト、画像、音声などの一般的なモダリティーを認識し、ゼロショットおよびフューショット設定で自由形式のテキストを使用して応答を生成できます。
この研究では、著者はマルチモーダルな大規模言語モデルの基礎となる機能を解放します。グラウンディング機能によって、視覚言語タスクはより便利で効率的な人間と AI の対話が可能になります。これにより、詳細なテキストの説明を入力して参照するのではなく、画像内のオブジェクトまたは領域を直接ポイントできるようになり、モデルはその画像領域をその空間的位置とともに理解できるようになります。また、グラウンディング機能により、モデルは視覚的な回答 (つまり、境界ボックス) で応答できるようになり、参照表現の理解など、さらに多くの視覚言語タスクをサポートできます。視覚的な回答は、テキストのみの回答と比べてより正確であり、相互参照の曖昧さが解決されます。さらに、グラウンディング機能により、生成された自由形式のテキスト応答の名詞句と参照表現を画像領域にリンクできるため、より正確で情報量の多い、包括的な回答が得られます。
画像¶
目次¶
要件をインストール¶
%pip install --upgrade pip
%pip uninstall -q -y openvino-dev openvino openvino-nightly
%pip install -q "openvino-nightly"
%pip install -q "transformers>=4.35" Pillow gradio opencv-python
%pip install -q --extra-index-url https://download.pytorch.org/whl/cpu torch torchvision
Requirement already satisfied: pip in /opt/home/k8sworker/ci-ai/cibuilds/ov-notebook/OVNotebookOps-609/.workspace/scm/ov-notebook/.venv/lib/python3.8/site-packages (24.0)
DEPRECATION: pytorch-lightning 1.6.5 has a non-standard dependency specifier torch>=1.8.*. pip 24.1 will enforce this behaviour change. A possible replacement is to upgrade to a newer version of pytorch-lightning or contact the author to suggest that they release a version with a conforming dependency specifiers. Discussion can be found at https://github.com/pypa/pip/issues/12063
Note: you may need to restart the kernel to use updated packages.
Note: you may need to restart the kernel to use updated packages.
DEPRECATION: pytorch-lightning 1.6.5 has a non-standard dependency specifier torch>=1.8.*. pip 24.1 will enforce this behaviour change. A possible replacement is to upgrade to a newer version of pytorch-lightning or contact the author to suggest that they release a version with a conforming dependency specifiers. Discussion can be found at https://github.com/pypa/pip/issues/12063
Note: you may need to restart the kernel to use updated packages.
DEPRECATION: pytorch-lightning 1.6.5 has a non-standard dependency specifier torch>=1.8.*. pip 24.1 will enforce this behaviour change. A possible replacement is to upgrade to a newer version of pytorch-lightning or contact the author to suggest that they release a version with a conforming dependency specifiers. Discussion can be found at https://github.com/pypa/pip/issues/12063
Note: you may need to restart the kernel to use updated packages.
DEPRECATION: pytorch-lightning 1.6.5 has a non-standard dependency specifier torch>=1.8.*. pip 24.1 will enforce this behaviour change. A possible replacement is to upgrade to a newer version of pytorch-lightning or contact the author to suggest that they release a version with a conforming dependency specifiers. Discussion can be found at https://github.com/pypa/pip/issues/12063
Note: you may need to restart the kernel to use updated packages.
元のモデルの推論¶
元の例を見てみましょう。
import requests
from PIL import Image
from transformers import AutoProcessor, AutoModelForVision2Seq
model = AutoModelForVision2Seq.from_pretrained("microsoft/kosmos-2-patch14-224")
processor = AutoProcessor.from_pretrained("microsoft/kosmos-2-patch14-224")
prompt = "<grounding>An image of" # <grounding> is used to prompt the model to generate locations tokens
url = "https://huggingface.co/microsoft/kosmos-2-patch14-224/resolve/main/snowman.png"
image = Image.open(requests.get(url, stream=True).raw)
# The original Kosmos-2 demo saves the image first then reload it. For some images, this will give slightly different image input and change the generation outputs.
image.save("new_image.jpg")
image = Image.open("new_image.jpg")
inputs = processor(text=prompt, images=image, return_tensors="pt")
generated_ids = model.generate(
pixel_values=inputs["pixel_values"],
input_ids=inputs["input_ids"],
attention_mask=inputs["attention_mask"],
image_embeds=None,
image_embeds_position_mask=inputs["image_embeds_position_mask"],
use_cache=True,
max_new_tokens=128,
)
generated_text = processor.batch_decode(generated_ids, skip_special_tokens=True)[0]
# Specify `cleanup_and_extract=False` in order to see the raw model generation.
processed_text = processor.post_process_generation(generated_text, cleanup_and_extract=False)
print(f'Raw model generation: {processed_text}')
# `<grounding> An image of<phrase> a snowman</phrase><object><patch_index_0044><patch_index_0863></object> warming himself by<phrase> a fire</phrase><object><patch_index_0005><patch_index_0911></object>.`
# By default, the generated text is cleanup and the entities are extracted.
processed_text, entities = processor.post_process_generation(generated_text)
print(f'Cleaned up generated text: {processed_text=}')
# `An image of a snowman warming himself by a fire.`
print(f'Extracted entities: {entities}')
# `[('a snowman', (12, 21), [(0.390625, 0.046875, 0.984375, 0.828125)]), ('a fire', (41, 47), [(0.171875, 0.015625, 0.484375, 0.890625)])]`
2024-02-10 00:50:06.197575: I tensorflow/core/util/port.cc:110] oneDNN custom operations are on. You may see slightly different numerical results due to floating-point round-off errors from different computation orders. To turn them off, set the environment variable TF_ENABLE_ONEDNN_OPTS=0. 2024-02-10 00:50:06.231091: I tensorflow/core/platform/cpu_feature_guard.cc:182] This TensorFlow binary is optimized to use available CPU instructions in performance-critical operations. To enable the following instructions: AVX2 AVX512F AVX512_VNNI FMA, in other operations, rebuild TensorFlow with the appropriate compiler flags.
2024-02-10 00:50:06.822623: W tensorflow/compiler/tf2tensorrt/utils/py_utils.cc:38] TF-TRT Warning: Could not find TensorRT
Special tokens have been added in the vocabulary, make sure the associated word embeddings are fine-tuned or trained.
Raw model generation: <grounding> An image of<phrase> a snowman</phrase><object><patch_index_0044><patch_index_0863></object> warming himself by<phrase> a fire</phrase><object><patch_index_0005><patch_index_0911></object>.
Cleaned up generated text: processed_text='An image of a snowman warming himself by a fire.'
Extracted entities: [('a snowman', (12, 21), [(0.390625, 0.046875, 0.984375, 0.828125)]), ('a fire', (41, 47), [(0.171875, 0.015625, 0.484375, 0.890625)])]
エンティティーを取得したら、次のヘルパー関数を使用して、その境界 B ボックスを画像上に描画できます。
import cv2
import numpy as np
from PIL import Image
def is_overlapping(rect1, rect2):
x1, y1, x2, y2 = rect1
x3, y3, x4, y4 = rect2
return not (x2 < x3 or x1 > x4 or y2 < y3 or y1 > y4)
def draw_entity_boxes_on_image(image, entities):
"""_summary_
Args:
image (_type_): image or image path
collect_entity_location (_type_): _description_
"""
if isinstance(image, Image.Image):
image_h = image.height
image_w = image.width
image = np.array(image)[:, :, [2, 1, 0]]
else:
raise ValueError(f"invaild image format, {type(image)} for {image}")
if len(entities) == 0:
return image
new_image = image.copy()
previous_bboxes = []
# size of text
text_size = 1
# thickness of text
text_line = 1 # int(max(1 * min(image_h, image_w) / 512, 1))
box_line = 3
(c_width, text_height), _ = cv2.getTextSize("F", cv2.FONT_HERSHEY_COMPLEX, text_size, text_line)
base_height = int(text_height * 0.675)
text_offset_original = text_height - base_height
text_spaces = 3
for entity_name, (start, end), bboxes in entities:
for (x1_norm, y1_norm, x2_norm, y2_norm) in bboxes:
orig_x1, orig_y1, orig_x2, orig_y2 = int(x1_norm * image_w), int(y1_norm * image_h), int(x2_norm * image_w), int(y2_norm * image_h)
# draw bbox
# random color
color = tuple(np.random.randint(0, 255, size=3).tolist())
new_image = cv2.rectangle(new_image, (orig_x1, orig_y1), (orig_x2, orig_y2), color, box_line)
l_o, r_o = box_line // 2 + box_line % 2, box_line // 2 + box_line % 2 + 1
x1 = orig_x1 - l_o
y1 = orig_y1 - l_o
if y1 < text_height + text_offset_original + 2 * text_spaces:
y1 = orig_y1 + r_o + text_height + text_offset_original + 2 * text_spaces
x1 = orig_x1 + r_o
# add text background
(text_width, text_height), _ = cv2.getTextSize(f" {entity_name}", cv2.FONT_HERSHEY_COMPLEX, text_size, text_line)
text_bg_x1, text_bg_y1, text_bg_x2, text_bg_y2 = x1, y1 - (text_height + text_offset_original + 2 * text_spaces), x1 + text_width, y1
for prev_bbox in previous_bboxes:
while is_overlapping((text_bg_x1, text_bg_y1, text_bg_x2, text_bg_y2), prev_bbox):
text_bg_y1 += (text_height + text_offset_original + 2 * text_spaces)
text_bg_y2 += (text_height + text_offset_original + 2 * text_spaces)
y1 += (text_height + text_offset_original + 2 * text_spaces)
if text_bg_y2 >= image_h:
text_bg_y1 = max(0, image_h - (text_height + text_offset_original + 2 * text_spaces))
text_bg_y2 = image_h
y1 = image_h
break
alpha = 0.5
for i in range(text_bg_y1, text_bg_y2):
for j in range(text_bg_x1, text_bg_x2):
if i < image_h and j < image_w:
if j < text_bg_x1 + 1.35 * c_width:
# original color
bg_color = color
else:
# white
bg_color = [255, 255, 255]
new_image[i, j] = (alpha * new_image[i, j] + (1 - alpha) * np.array(bg_color)).astype(np.uint8)
cv2.putText(
new_image, f" {entity_name}", (x1, y1 - text_offset_original - 1 * text_spaces), cv2.FONT_HERSHEY_COMPLEX, text_size, (0, 0, 0), text_line, cv2.LINE_AA
)
# previous_locations.append((x1, y1))
previous_bboxes.append((text_bg_x1, text_bg_y1, text_bg_x2, text_bg_y2))
pil_image = Image.fromarray(new_image[:, :, [2, 1, 0]])
return pil_image
# Draw the bounding bboxes
new_image = draw_entity_boxes_on_image(image, entities)
display(new_image)
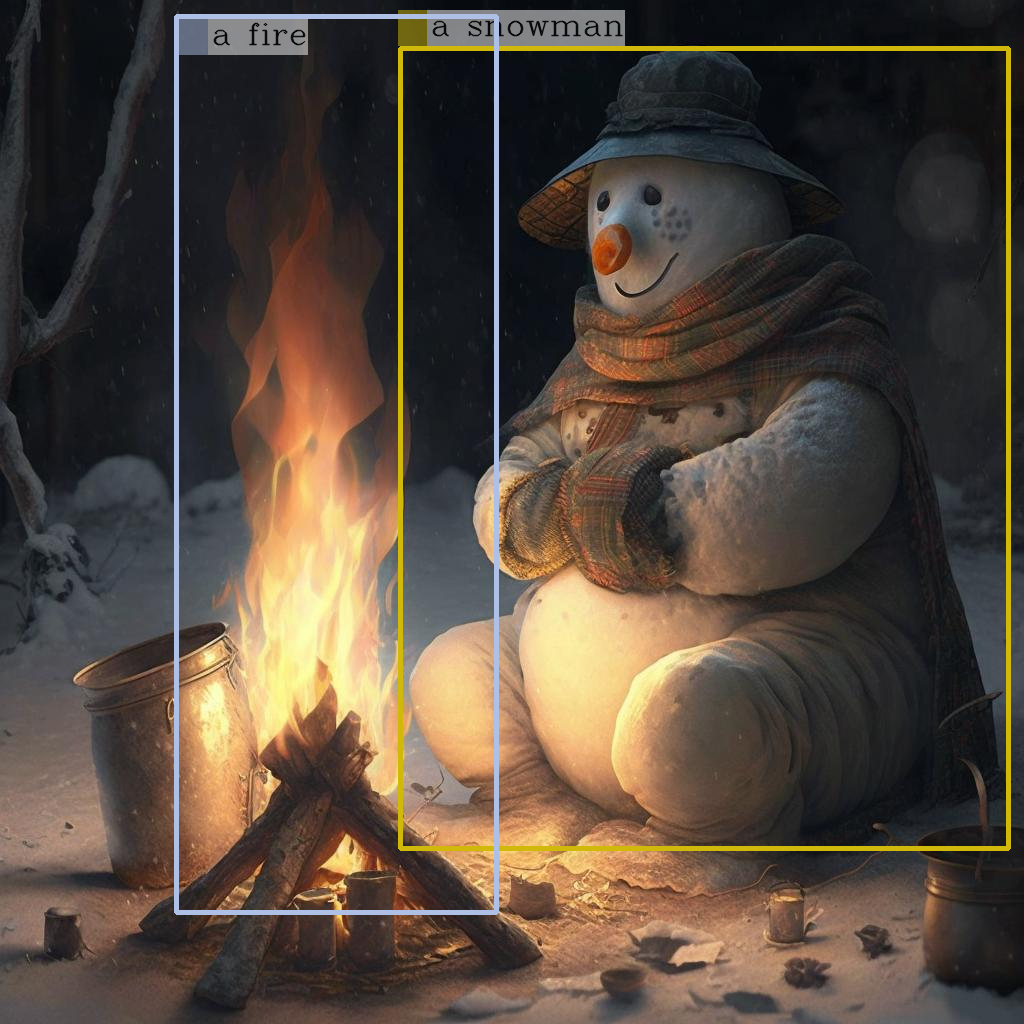
モデルを OpenVINO 中間表現 (IR) 形式に変換¶
元のモデルには、ビジョンモデル Kosmos2VisionModel
、画像モデルの出力をテキストモデルの入力の一部 (つまり、画像特徴) に変換するレイヤーである Kosmos2ImageToTextProjection
、およびトランスフォーマー・ベースのテキストモデル Kosmos2TextForCausalLM
の 3 つのモデルが含まれています。すべて変換してから元のモデルと置き換えます。
変換されたモデルのパスを定義します。
from pathlib import Path
models_base_folder = Path("models")
VISION_MODEL_IR_PATH = models_base_folder / "vision_model.xml"
IMAGE_TO_TEXT_PROJECTION_MODEL_IR_PATH = models_base_folder / "image_to_text_projection_model.xml"
FIRST_STAGE_MODEL_PATH = models_base_folder / "kosmos_input_embed.xml"
SECOND_STAGE_MODEL_PATH = models_base_folder / "kosmos_with_past.xml"
PyTorch モジュールの変換関数を定義します。ov.convert_model
関数を使用して OpenVINO 中間表現オブジェクトを取得し、ov.save_model
関数でそれを XML ファイルとして保存します。
import gc
import torch
import openvino as ov
def cleanup_torchscript_cache():
# cleanup memory
torch._C._jit_clear_class_registry()
torch.jit._recursive.concrete_type_store = torch.jit._recursive.ConcreteTypeStore()
torch.jit._state._clear_class_state()
gc.collect()
def convert(model: torch.nn.Module, xml_path: str, example_input):
xml_path = Path(xml_path)
if not xml_path.exists():
xml_path.parent.mkdir(parents=True, exist_ok=True)
with torch.no_grad():
converted_model = ov.convert_model(model, example_input=example_input)
ov.save_model(converted_model, xml_path, compress_to_fp16=False)
cleanup_torchscript_cache()
ビジョンモデルを変換¶
ビジョンモデルは、pixel_values
を受け入れ、image_embeds
を返します。
convert(model.vision_model, VISION_MODEL_IR_PATH, inputs["pixel_values"])
WARNING:tensorflow:Please fix your imports. Module tensorflow.python.training.tracking.base has been moved to tensorflow.python.trackable.base. The old module will be deleted in version 2.11.
[ WARNING ] Please fix your imports. Module %s has been moved to %s. The old module will be deleted in version %s.
/opt/home/k8sworker/ci-ai/cibuilds/ov-notebook/OVNotebookOps-609/.workspace/scm/ov-notebook/.venv/lib/python3.8/site-packages/transformers/models/kosmos2/modeling_kosmos2.py:471: TracerWarning: Converting a tensor to a Python boolean might cause the trace to be incorrect. We can't record the data flow of Python values, so this value will be treated as a constant in the future. This means that the trace might not generalize to other inputs!
if attn_weights.size() != (bsz * self.num_heads, tgt_len, src_len):
/opt/home/k8sworker/ci-ai/cibuilds/ov-notebook/OVNotebookOps-609/.workspace/scm/ov-notebook/.venv/lib/python3.8/site-packages/transformers/models/kosmos2/modeling_kosmos2.py:511: TracerWarning: Converting a tensor to a Python boolean might cause the trace to be incorrect. We can't record the data flow of Python values, so this value will be treated as a constant in the future. This means that the trace might not generalize to other inputs!
if attn_output.size() != (bsz * self.num_heads, tgt_len, self.head_dim):
画像をテキストに変換する投影モデル¶
from torch import nn
def get_image_embeds(pixel_values):
vision_model_output = model.vision_model(pixel_values)
image_embeds = model.vision_model.model.post_layernorm(vision_model_output[0])
image_embeds = nn.functional.normalize(image_embeds, dim=-1)
return image_embeds
image_embeds = get_image_embeds(inputs["pixel_values"])
convert(model.image_to_text_projection, IMAGE_TO_TEXT_PROJECTION_MODEL_IR_PATH, image_embeds)
/opt/home/k8sworker/ci-ai/cibuilds/ov-notebook/OVNotebookOps-609/.workspace/scm/ov-notebook/.venv/lib/python3.8/site-packages/torch/jit/_trace.py:165: UserWarning: The .grad attribute of a Tensor that is not a leaf Tensor is being accessed. Its .grad attribute won't be populated during autograd.backward(). If you indeed want the .grad field to be populated for a non-leaf Tensor, use .retain_grad() on the non-leaf Tensor. If you access the non-leaf Tensor by mistake, make sure you access the leaf Tensor instead. See github.com/pytorch/pytorch/pull/30531 for more informations. (Triggered internally at aten/src/ATen/core/TensorBody.h:489.)
if a.grad is not None:
テキストモデルを変換¶
テキストモデルは生成パイプラインで実行されますが、2 つのステージに分けることができます。最初のステージで、モデルは image_embeds
を 2 番目のステージの出力に変換します。第 2 ステージでは、モデルは数回の実行中にトークンを生成します。トークンは、AutoProcessor
によって生のモデル生成テキストに変換できます。
from typing import Optional, List
from transformers.models.kosmos2.modeling_kosmos2 import create_position_ids_from_input_ids
def get_projecton_image_embeds(pixel_values):
vision_model_output = model.vision_model(pixel_values)
image_embeds = model.vision_model.model.post_layernorm(vision_model_output[0])
image_embeds = nn.functional.normalize(image_embeds, dim=-1)
image_embeds, _ = model.image_to_text_projection(image_embeds)
return image_embeds
def flattenize_inputs(inputs):
"""
Helper function for making nested inputs flattens
"""
flatten_inputs = []
for input_data in inputs:
if input_data is None:
continue
if isinstance(input_data, (list, tuple)):
flatten_inputs.extend(flattenize_inputs(input_data))
else:
flatten_inputs.append(input_data)
return flatten_inputs
def postprocess_converted_model(ov_model, example_input=None, input_names=None, output_names=None, dynamic_shapes=None):
"""
Helper function for appling postprocessing on converted model with updating input names, shapes and output names
acording to requested specification
"""
flatten_example_inputs = flattenize_inputs(example_input) if example_input else []
if input_names:
for inp_name, m_input, input_data in zip(input_names, ov_model.inputs, flatten_example_inputs):
m_input.get_tensor().set_names({inp_name})
if output_names:
for out, out_name in zip(ov_model.outputs, output_names):
out.get_tensor().set_names({out_name})
return ov_model
def convert_text_model():
model.text_model.model.config.torchscript = True
model.text_model.config.torchscript = True
image_embeds = get_projecton_image_embeds(inputs["pixel_values"])
conv_inputs = {
'input_ids': inputs["input_ids"],
'attention_mask': inputs["attention_mask"],
'image_embeds': image_embeds,
'image_embeds_position_mask': inputs["image_embeds_position_mask"],
}
outs = model.text_model.model(**conv_inputs)
inputs_ = ["input_ids", 'attention_mask']
outputs = ["logits"]
dynamic_shapes = {"input_ids": {1: "seq_len"}, "attention_mask": {1: "seq_len"}, "position_ids": {0: "seq_len"}}
for idx in range(len(outs[1])):
inputs_.extend([f"past_key_values.{idx}.key", f"past_key_values.{idx}.value"])
dynamic_shapes[inputs_[-1]] = {2: "past_sequence + sequence"}
dynamic_shapes[inputs_[-2]] = {2: "past_sequence + sequence"}
outputs.extend([f"present.{idx}.key", f"present.{idx}.value"])
if not FIRST_STAGE_MODEL_PATH.exists():
ov_model = ov.convert_model(model.text_model.model, example_input=conv_inputs)
ov_model = postprocess_converted_model(ov_model, output_names=outputs)
ov.save_model(ov_model, FIRST_STAGE_MODEL_PATH)
del ov_model
cleanup_torchscript_cache()
if not SECOND_STAGE_MODEL_PATH.exists():
position_ids = create_position_ids_from_input_ids(
inputs["input_ids"],
padding_idx=model.text_model.config.pad_token_id,
past_key_values_length=0,
)[:, -1:]
example_input_second_stage = {
"input_ids": inputs["input_ids"][:, -1:],
"attention_mask": inputs["input_ids"].new_ones(1, inputs["input_ids"].shape[1] + 1),
'position_ids': position_ids,
"past_key_values": outs[1],
}
ov_model = ov.convert_model(model.text_model.model, example_input=example_input_second_stage)
ov_model = postprocess_converted_model(
ov_model,
example_input=example_input_second_stage.values(),
input_names=inputs_,
output_names=outputs,
dynamic_shapes=dynamic_shapes
)
ov.save_model(ov_model, SECOND_STAGE_MODEL_PATH)
del ov_model
cleanup_torchscript_cache()
convert_text_model()
/opt/home/k8sworker/ci-ai/cibuilds/ov-notebook/OVNotebookOps-609/.workspace/scm/ov-notebook/.venv/lib/python3.8/site-packages/transformers/models/kosmos2/modeling_kosmos2.py:810: TracerWarning: Converting a tensor to a Python boolean might cause the trace to be incorrect. We can't record the data flow of Python values, so this value will be treated as a constant in the future. This means that the trace might not generalize to other inputs!
if max_pos > self.weights.size(0):
/opt/home/k8sworker/ci-ai/cibuilds/ov-notebook/OVNotebookOps-609/.workspace/scm/ov-notebook/.venv/lib/python3.8/site-packages/transformers/models/kosmos2/modeling_kosmos2.py:1119: TracerWarning: Converting a tensor to a Python boolean might cause the trace to be incorrect. We can't record the data flow of Python values, so this value will be treated as a constant in the future. This means that the trace might not generalize to other inputs!
if input_shape[-1] > 1:
/opt/home/k8sworker/ci-ai/cibuilds/ov-notebook/OVNotebookOps-609/.workspace/scm/ov-notebook/.venv/lib/python3.8/site-packages/transformers/models/kosmos2/modeling_kosmos2.py:926: TracerWarning: Converting a tensor to a Python boolean might cause the trace to be incorrect. We can't record the data flow of Python values, so this value will be treated as a constant in the future. This means that the trace might not generalize to other inputs!
if attention_mask.size() != (batch_size, 1, seq_length, src_len):
/opt/home/k8sworker/ci-ai/cibuilds/ov-notebook/OVNotebookOps-609/.workspace/scm/ov-notebook/.venv/lib/python3.8/site-packages/transformers/models/kosmos2/modeling_kosmos2.py:1212: TracerWarning: Converting a tensor to a Python boolean might cause the trace to be incorrect. We can't record the data flow of Python values, so this value will be treated as a constant in the future. This means that the trace might not generalize to other inputs!
if past_key_values_length > 0:
モデルをコンパイルしてパイプラインを準備¶
OpenVINO を使用してモデル推論に使用されるデバイスをドロップダウン・リストから選択します。
import ipywidgets as widgets
core = ov.Core()
DEVICE = widgets.Dropdown(
options=core.available_devices + ["AUTO"],
value='AUTO',
description='Device:',
disabled=False,
)
DEVICE
Dropdown(description='Device:', index=1, options=('CPU', 'AUTO'), value='AUTO')
元のパイプラインとの対話を可能にするため、コンパイルされたモデルの呼び出し可能なラッパークラスを作成します。すべてのラッパークラスは、np.array
ではなく torch.Tensor
を返すことに注意してください。
class WraperInternalVisionModel:
post_layernorm = model.vision_model.model.post_layernorm
class VisionModelWrapper(torch.nn.Module):
def __init__(self, model_ir_path):
super().__init__()
self.model = WraperInternalVisionModel()
self.vision_model = core.compile_model(model_ir_path, DEVICE.value)
def forward(self, pixel_values, **kwargs):
vision_model_output = self.vision_model(pixel_values)[0]
return [torch.from_numpy(vision_model_output)]
class ImageToTextProjectionModelWrapper(torch.nn.Module):
def __init__(self, model_ir_path):
super().__init__()
self.image_to_text_projection = core.compile_model(model_ir_path, DEVICE.value)
def forward(self, image_embeds):
output = self.image_to_text_projection(image_embeds)
image_embeds = output[0]
projection_attentions = output[1]
return image_embeds, projection_attentions
from transformers.generation import GenerationConfig, GenerationMixin
from transformers.models.kosmos2.modeling_kosmos2 import Kosmos2ForConditionalGenerationModelOutput
class KosmosForCausalLMWrapper(GenerationMixin):
def __init__(self, first_stage_model_path, second_stage_model_path, device):
self.model_stage_1 = core.compile_model(first_stage_model_path, DEVICE.value)
self.model_stage_2 = core.read_model(second_stage_model_path)
self.input_names = {
key.get_any_name(): idx for idx, key in enumerate(self.model_stage_2.inputs)
}
self.output_names = {
key.get_any_name(): idx for idx, key in enumerate(self.model_stage_2.outputs)
}
self.key_value_input_names = [
key for key in self.input_names if "key_values" in key
]
self.key_value_output_names = [
key for key in self.output_names if "present" in key
]
self.model_stage_2 = core.compile_model(self.model_stage_2, DEVICE.value)
self.request = self.model_stage_2.create_infer_request()
self.config = model.config
self.generation_config = GenerationConfig.from_model_config(model.config)
self.main_input_name = "input_ids"
self.device = torch.device("cpu")
self.num_pkv = 2
self.lm_head = nn.Linear(in_features=model.text_model.config.embed_dim, out_features=model.text_model.config.vocab_size, bias=False)
def get_input_embeddings(self) -> nn.Module:
return self.model.embed_tokens
def set_input_embeddings(self, value):
self.model.embed_tokens = value
def get_output_embeddings(self) -> nn.Module:
return self.lm_head
def set_output_embeddings(self, new_embeddings):
self.lm_head = new_embeddings
def can_generate(self):
"""Returns True to validate the check that the model using `GenerationMixin.generate()` can indeed generate."""
return True
def __call__(
self,
input_ids,
attention_mask: Optional[torch.Tensor] = None,
image_embeds: Optional[torch.Tensor] = None,
image_embeds_position_mask: Optional[torch.Tensor] = None,
position_ids=None,
past_key_values: Optional[List[torch.FloatTensor]] = None,
**kwargs,
):
return self.forward(
input_ids, attention_mask, image_embeds, image_embeds_position_mask, position_ids, past_key_values
)
def forward(
self,
input_ids,
attention_mask: Optional[torch.Tensor] = None,
image_embeds: Optional[torch.Tensor] = None,
image_embeds_position_mask: Optional[torch.Tensor] = None,
position_ids=None,
past_key_values: Optional[List[torch.FloatTensor]] = None,
**kwargs
):
if past_key_values is None:
outs = self.model_stage_1(
{
'input_ids': input_ids,
'attention_mask': attention_mask,
'image_embeds': image_embeds,
'image_embeds_position_mask': image_embeds_position_mask,
}
)
lm_logits = model.text_model.lm_head(torch.from_numpy(outs[0]))
pkv = list(outs.values())[1:]
pkv = tuple(pkv[i : i + 2] for i in range(0, len(pkv), 2))
return Kosmos2ForConditionalGenerationModelOutput(logits=lm_logits, past_key_values=pkv)
if past_key_values is not None:
past_key_values = tuple(
past_key_value
for pkv_per_layer in past_key_values
for past_key_value in pkv_per_layer
)
inputs_ = {
"input_ids": input_ids[:, -1].unsqueeze(-1),
"attention_mask": attention_mask,
'position_ids': position_ids
}
inputs_.update(dict(zip(self.key_value_input_names, past_key_values)))
# Run inference
self.request.start_async(inputs_, share_inputs=True)
self.request.wait()
logits = torch.from_numpy(self.request.get_tensor("logits").data)
logits = model.text_model.lm_head(logits)
# Tuple of length equal to : number of layer * number of past_key_value per decoder layer (2 corresponds to the self-attention layer)
past_key_values = tuple(
self.request.get_tensor(key).data for key in self.key_value_output_names
)
# Tuple of tuple of length `n_layers`, with each tuple of length equal to 2 (k/v of self-attention)
past_key_values = tuple(
past_key_values[i : i + self.num_pkv]
for i in range(0, len(past_key_values), self.num_pkv)
)
return Kosmos2ForConditionalGenerationModelOutput(logits=logits, past_key_values=past_key_values)
def prepare_inputs_for_generation(
self,
input_ids,
image_embeds=None,
image_embeds_position_mask=None,
past_key_values=None,
attention_mask=None,
use_cache=None,
**kwargs,
):
input_shape = input_ids.shape
# if model is used as a decoder in encoder-decoder model, the decoder attention mask is created on the fly
if attention_mask is None:
attention_mask = input_ids.new_ones(input_shape)
position_ids = None
# cut input_ids if past_key_values is used
if past_key_values is not None:
position_ids = create_position_ids_from_input_ids(
input_ids,
padding_idx=model.text_model.config.pad_token_id,
past_key_values_length=0,
)[:, -1:]
input_ids = input_ids[:, -1:]
image_embeds = None
image_embeds_position_mask = None
elif image_embeds_position_mask is not None:
batch_size, seq_len = input_ids.size()
mask_len = image_embeds_position_mask.size()[-1]
image_embeds_position_mask = torch.cat(
(
image_embeds_position_mask,
torch.zeros(size=(batch_size, seq_len - mask_len), dtype=torch.bool, device=input_ids.device),
),
dim=1,
)
return {
"input_ids": input_ids,
"image_embeds": image_embeds,
"image_embeds_position_mask": image_embeds_position_mask,
'position_ids': position_ids,
"past_key_values": past_key_values,
"attention_mask": attention_mask,
}
@staticmethod
# Copied from transformers.models.umt5.modeling_umt5.UMT5ForConditionalGeneration._reorder_cache
def _reorder_cache(past_key_values, beam_idx):
reordered_past = ()
for layer_past in past_key_values:
reordered_past += (
tuple(past_state.index_select(0, beam_idx.to(past_state.device)) for past_state in layer_past),
)
return reordered_past
class Kosmos2ForConditionalGenerationWrapper:
def __init__(self, vision_model_path, image_to_text_projection_model_path, first_stage_model_path, second_stage_model_path, device):
self.vision_model = VisionModelWrapper(vision_model_path)
self.image_to_text_projection = ImageToTextProjectionModelWrapper(image_to_text_projection_model_path)
self.text_model = KosmosForCausalLMWrapper(first_stage_model_path, second_stage_model_path, device)
def generate(
self,
pixel_values=None,
image_embeds_position_mask=None,
input_ids=None,
attention_mask=None,
image_embeds=None,
**kwargs,
):
vision_model_output = self.vision_model(pixel_values)
image_embeds = model.vision_model.model.post_layernorm(vision_model_output[0])
# normalized features
image_embeds = nn.functional.normalize(image_embeds, dim=-1)
image_embeds, projection_attentions = self.image_to_text_projection(image_embeds.detach().numpy())
output = self.text_model.generate(
input_ids,
attention_mask=attention_mask,
image_embeds=image_embeds,
image_embeds_position_mask=image_embeds_position_mask,
**kwargs,
)
return output
ov_model = Kosmos2ForConditionalGenerationWrapper(VISION_MODEL_IR_PATH, IMAGE_TO_TEXT_PROJECTION_MODEL_IR_PATH, FIRST_STAGE_MODEL_PATH, SECOND_STAGE_MODEL_PATH, DEVICE)
推論¶
generated_ids_ = ov_model.generate(
pixel_values=inputs["pixel_values"],
input_ids=inputs["input_ids"],
attention_mask=inputs["attention_mask"],
image_embeds=None,
image_embeds_position_mask=inputs["image_embeds_position_mask"],
max_new_tokens=128,
)
generated_text = processor.batch_decode(generated_ids, skip_special_tokens=True)[0]
# Specify `cleanup_and_extract=False` in order to see the raw model generation.
processed_text = processor.post_process_generation(generated_text, cleanup_and_extract=False)
print(f'Raw model generation: {processed_text}')
# `<grounding> An image of<phrase> a snowman</phrase><object><patch_index_0044><patch_index_0863></object> warming himself by<phrase> a fire</phrase><object><patch_index_0005><patch_index_0911></object>.`
# By default, the generated text is cleanup and the entities are extracted.
processed_text, entities = processor.post_process_generation(generated_text)
print(f'Cleaned up generated text: {processed_text=}')
# `An image of a snowman warming himself by a fire.`
print(f'Extracted entities: {entities}')
# `[('a snowman', (12, 21), [(0.390625, 0.046875, 0.984375, 0.828125)]), ('a fire', (41, 47), [(0.171875, 0.015625, 0.484375, 0.890625)])]`
Raw model generation: <grounding> An image of<phrase> a snowman</phrase><object><patch_index_0044><patch_index_0863></object> warming himself by<phrase> a fire</phrase><object><patch_index_0005><patch_index_0911></object>.
Cleaned up generated text: processed_text='An image of a snowman warming himself by a fire.'
Extracted entities: [('a snowman', (12, 21), [(0.390625, 0.046875, 0.984375, 0.828125)]), ('a fire', (41, 47), [(0.171875, 0.015625, 0.484375, 0.890625)])]
new_image = draw_entity_boxes_on_image(image, entities)
display(new_image)
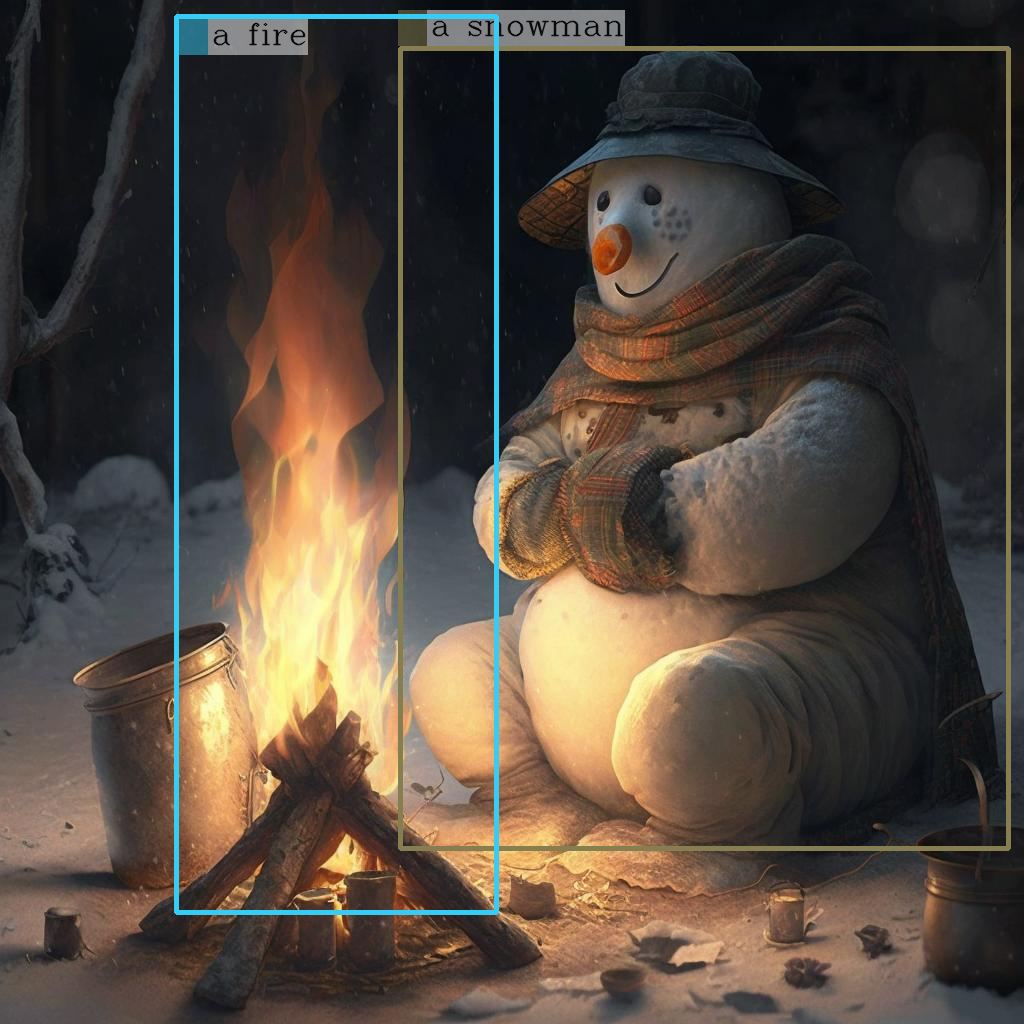
インタラクティブな推論¶
import gradio as gr
images = {
"snowman.png": "https://huggingface.co/microsoft/kosmos-2-patch14-224/resolve/main/snowman.png",
"two_dogs.jpg": "https://huggingface.co/microsoft/kosmos-2-patch14-224/resolve/main/two_dogs.jpg",
"six_planes.png": "https://ydshieh-kosmos-2.hf.space/file=/home/user/app/images/six_planes.png"
}
for image_name, url in images.items():
image = Image.open(requests.get(url, stream=True).raw)
image.save(image_name)
def generate(image, prompt, use_bbox, _=gr.Progress(track_tqdm=True)):
if use_bbox:
prompt = "<grounding> " + prompt
inputs = processor(text=prompt, images=image, return_tensors="pt")
generated_ids_ = ov_model.generate(
pixel_values=inputs["pixel_values"],
input_ids=inputs["input_ids"],
attention_mask=inputs["attention_mask"],
image_embeds=None,
image_embeds_position_mask=inputs["image_embeds_position_mask"],
max_new_tokens=128,
)
generated_text = processor.batch_decode(generated_ids_, skip_special_tokens=True)[0]
processed_text, entities = processor.post_process_generation(generated_text)
new_image = draw_entity_boxes_on_image(Image.fromarray(image), entities)
return new_image, processed_text
demo = gr.Interface(
generate,
[
gr.Image(label="Input image"),
gr.Textbox(label="Prompt"),
gr.Checkbox(label="Show bounding boxes", value=True)
],
["image", "text"],
examples=[
["snowman.png", "An image of"],
["two_dogs.jpg", "Describe this image in detail:"],
["six_planes.png", "What is going on?"]
],
allow_flagging="never",
)
try:
demo.queue().launch(debug=False)
except Exception:
demo.queue().launch(debug=False, share=True)
# if you are launching remotely, specify server_name and server_port
# demo.launch(server_name='your server name', server_port='server port in int')
# Read more in the docs: https://gradio.app/docs/
Running on local URL: http://127.0.0.1:7860 To create a public link, set share=True in launch().