OpenVINO™ による人物追跡¶
この Jupyter ノートブックはオンラインで起動でき、ブラウザーのウィンドウで対話型環境を開きます。ローカルにインストールすることもできます。次のオプションのいずれかを選択します。
このノートブックは、OpenVINO を使用したリアルタイムの人物追跡のデモンストレーションを行います。入力ビデオシーケンスからフレームを読み取り、フレーム内の人物を検出し、各人物を一意に識別して、フレームから出るまで全員を追跡します。SORT (Simple Online and Realtime Tracking) の拡張である Deep SORT アルゴリズムを使用してオブジェクト追跡を実行します。
検出と追跡¶
オブジェクト検出では、フレーム内のオブジェクトを検出し、その周囲に境界ボックスまたはマスクを配置して、オブジェクトを分類します。検出器のジョブはここで終了することに注意してください。各フレームを個別に処理し、その特定のフレーム内の多数のオブジェクトを識別します。
一方、オブジェクト・トラッカーは、ビデオ全体にわたって特定のオブジェクトを追跡する必要があります。検出器がフレーム内で 3 台の車を検出した場合、オブジェクト・トラッカーは 3 つの個別の検出を識別し、後続のフレームにわたってそれを追跡する必要があります (一意の ID で)。
ディープソート¶
Deep SORT は、オブジェクトの速度と動きだけでなく、オブジェクトの外観に基づいてオブジェクトを追跡するアルゴリズムとして定義できます。これは次の 3 つの主要コンポーネントで構成されています。
-
検出
これは追跡モジュールの最初のステップです。このステップでは、ディープラーニング・モデルを使用して、フレーム内の追跡対象となるオブジェクトを検出します。これらの検出は次のステップに渡されます。
-
予測
このステップでは、カルマンフィルター[1] フレームワークを使用して、次のフレームの各追跡オブジェクトのターゲット境界ボックスを予測します。予測出力には、確認済みと未確認の 2 つの状態があります。新しいトラックはデフォルトで未確認の状態になっていますが、この新しいトラックと一定数の連続検出が一致すると、確認済みにすることができます。一方、一致したトラックが一定時間以上再生されなかった場合、そのトラックも削除されます。
-
データの関連付けと更新
ここで、ターゲット境界ボックスを検出された境界ボックスと一致させ、トラック ID を更新する必要があります。予測されたカルマン状態と新たに到達した測定値との関連を解決する従来の方法は、ハンガリー・アルゴリズム[2] を使用して割り当て問題を構築することです。この問題の定式化では、2 つの適切なメトリックの組み合わせを通じて、動きと外観の情報を統合します。最初のマッチングステップで使用されるコストは、マハラノビス距離とコサイン距離の組み合わせとして設定されます。マハラノビス距離は動き情報を組み込むために使用され、コサイン距離は 2 つのオブジェクト間の類似性を計算するために使用されます。コサイン距離は、長期間の遮蔽や動きの推定が失敗した場合にトラッカーが ID を回復するのに役立つメトリックです。この目的のため、再識別モデルが実装され、オブジェクトの外観を表す高次元空間のベクトルが生成されます。これら単純なものを使用することで、トラッカーはさらに強力かつ正確になります。
2 番目のマッチングステージでは、前のステップで未確認かつ一致しなかったトラックのセットに対して、オリジナルの SORT アルゴリズム[3] で提案された交差和集合 (IOU) 関連付けを実行します。検出とターゲットの IOU が
IOUmin
と呼ばれるしきい値より小さい場合、その割り当ては拒否されます。これにより、静的なシーン・ジオメトリーによる部分的な遮蔽などによる突然の外観の変化を考慮し、エラーに対する堅牢性を高めることができます。検出結果がターゲットに関連付けられると、検出された境界ボックスを使用してターゲットの状態が更新されます。
[1] R. Kalman, “A New Approach to Linear Filtering and Prediction Problems”, Journal of Basic Engineering, vol. 82, no. Series D, pp. 35-45, 1960.
[2] H. W. Kuhn, “The Hungarian method for the assignment problem”, Naval Research Logistics Quarterly, vol. 2, pp. 83-97, 1955.
[3] A. Bewley, G. Zongyuan, F. Ramos, and B. Upcroft, “Simple online and realtime tracking,” in ICIP, 2016, pp. 3464–3468.
目次¶
%pip install -q "openvino-dev>=2023.1.0"
%pip install -q opencv-python matplotlib requests scipy
DEPRECATION: pytorch-lightning 1.6.5 has a non-standard dependency specifier torch>=1.8.*. pip 24.1 will enforce this behaviour change. A possible replacement is to upgrade to a newer version of pytorch-lightning or contact the author to suggest that they release a version with a conforming dependency specifiers. Discussion can be found at https://github.com/pypa/pip/issues/12063
Note: you may need to restart the kernel to use updated packages.
DEPRECATION: pytorch-lightning 1.6.5 has a non-standard dependency specifier torch>=1.8.*. pip 24.1 will enforce this behaviour change. A possible replacement is to upgrade to a newer version of pytorch-lightning or contact the author to suggest that they release a version with a conforming dependency specifiers. Discussion can be found at https://github.com/pypa/pip/issues/12063
Note: you may need to restart the kernel to use updated packages.
インポート¶
import collections
from pathlib import Path
import sys
import time
import numpy as np
import cv2
from IPython import display
import matplotlib.pyplot as plt
import openvino as ov
# Import local modules
utils_file_path = Path('../utils/notebook_utils.py')
notebook_directory_path = Path('.')
if not utils_file_path.exists():
!git clone --depth 1 https://github.com/igor-davidyuk/openvino_notebooks.git -b moving_data_to_cloud openvino_notebooks
utils_file_path = Path('./openvino_notebooks/notebooks/utils/notebook_utils.py')
notebook_directory_path = Path('./openvino_notebooks/notebooks/407-person-tracking-webcam/')
sys.path.append(str(utils_file_path.parent))
sys.path.append(str(notebook_directory_path))
import notebook_utils as utils
from deepsort_utils.tracker import Tracker
from deepsort_utils.nn_matching import NearestNeighborDistanceMetric
from deepsort_utils.detection import Detection, compute_color_for_labels, xywh_to_xyxy, xywh_to_tlwh, tlwh_to_xyxy
モデルのダウンロード¶
テストを開始するには、OpenVINO のOpen Model Zoo の事前トレーニング済みモデルを使用します。
ここでは、openvino-dev
パッケージのコマンドライン・ツールである omz_downloader
を使用します。ディレクトリー構造が自動的に作成され、選択したモデルがダウンロードされます。モデルがすでにダウンロードされている場合、この手順はスキップされます。選択されたモデルはパブリック・ディレクトリーから取得されます。つまり、OpenVINO 中間表現 (OpenVINO IR) に変換する必要があります。
注: リスト外のモデルを使用する場合は、異なる前処理と後処理が必要になる場合があります。
この場合、人物検出モデルを展開してビデオの各フレーム内の人物を検出し、再識別モデルを使用して、コサイン距離によって人物の画像のペアを一致させる埋め込みベクトルを出力します。
別のモデル (オブジェクト検出モデルリストからの person-detection-xxx
、再識別モデルリストからの person-reidentification-retail-xxx
) をダウンロードする場合は、以下のコードでモデル名を置き換えます。
# A directory where the model will be downloaded.
base_model_dir = "model"
precision = "FP16"
# The name of the model from Open Model Zoo
detection_model_name = "person-detection-0202"
download_command = f"omz_downloader " \
f"--name {detection_model_name} " \
f"--precisions {precision} " \
f"--output_dir {base_model_dir} " \
f"--cache_dir {base_model_dir}"
! $download_command
detection_model_path = f"model/intel/{detection_model_name}/{precision}/{detection_model_name}.xml"
reidentification_model_name = "person-reidentification-retail-0287"
download_command = f"omz_downloader " \
f"--name {reidentification_model_name} " \
f"--precisions {precision} " \
f"--output_dir {base_model_dir} " \
f"--cache_dir {base_model_dir}"
! $download_command
reidentification_model_path = f"model/intel/{reidentification_model_name}/{precision}/{reidentification_model_name}.xml"
################|| Downloading person-detection-0202 ||################
========== Downloading model/intel/person-detection-0202/FP16/person-detection-0202.xml
... 12%, 32 KB, 1342 KB/s, 0 seconds passed
... 25%, 64 KB, 1352 KB/s, 0 seconds passed
… 38%, 96 KB, 1176 KB/s, 0 seconds passed
... 51%, 128 KB, 1385 KB/s, 0 seconds passed
… 64%, 160 KB, 1372 KB/s, 0 seconds passed … 77%, 192 KB, 1513 KB/s, 0 seconds passed … 89%, 224 KB, 1759 KB/s, 0 seconds passed … 100%, 248 KB, 1949 KB/s, 0 seconds passed
========== Downloading model/intel/person-detection-0202/FP16/person-detection-0202.bin
... 0%, 32 KB, 1296 KB/s, 0 seconds passed
… 1%, 64 KB, 1293 KB/s, 0 seconds passed … 2%, 96 KB, 1907 KB/s, 0 seconds passed
... 3%, 128 KB, 1703 KB/s, 0 seconds passed
… 4%, 160 KB, 2107 KB/s, 0 seconds passed … 5%, 192 KB, 2285 KB/s, 0 seconds passed … 6%, 224 KB, 2641 KB/s, 0 seconds passed
... 7%, 256 KB, 2336 KB/s, 0 seconds passed
… 8%, 288 KB, 2606 KB/s, 0 seconds passed … 9%, 320 KB, 2703 KB/s, 0 seconds passed … 9%, 352 KB, 2953 KB/s, 0 seconds passed … 10%, 384 KB, 2663 KB/s, 0 seconds passed … 11%, 416 KB, 2873 KB/s, 0 seconds passed … 12%, 448 KB, 2930 KB/s, 0 seconds passed … 13%, 480 KB, 3123 KB/s, 0 seconds passed
... 14%, 512 KB, 2867 KB/s, 0 seconds passed
… 15%, 544 KB, 2911 KB/s, 0 seconds passed … 16%, 576 KB, 3076 KB/s, 0 seconds passed … 17%, 608 KB, 3233 KB/s, 0 seconds passed
... 18%, 640 KB, 3007 KB/s, 0 seconds passed
… 18%, 672 KB, 3146 KB/s, 0 seconds passed … 19%, 704 KB, 3173 KB/s, 0 seconds passed … 20%, 736 KB, 3306 KB/s, 0 seconds passed … 21%, 768 KB, 3105 KB/s, 0 seconds passed … 22%, 800 KB, 3224 KB/s, 0 seconds passed … 23%, 832 KB, 3245 KB/s, 0 seconds passed … 24%, 864 KB, 3362 KB/s, 0 seconds passed
... 25%, 896 KB, 3181 KB/s, 0 seconds passed
… 26%, 928 KB, 3286 KB/s, 0 seconds passed … 27%, 960 KB, 3303 KB/s, 0 seconds passed … 27%, 992 KB, 3404 KB/s, 0 seconds passed
... 28%, 1024 KB, 3237 KB/s, 0 seconds passed
… 29%, 1056 KB, 3331 KB/s, 0 seconds passed … 30%, 1088 KB, 3345 KB/s, 0 seconds passed … 31%, 1120 KB, 3436 KB/s, 0 seconds passed … 32%, 1152 KB, 3287 KB/s, 0 seconds passed … 33%, 1184 KB, 3371 KB/s, 0 seconds passed … 34%, 1216 KB, 3384 KB/s, 0 seconds passed … 35%, 1248 KB, 3464 KB/s, 0 seconds passed
... 36%, 1280 KB, 3323 KB/s, 0 seconds passed
… 36%, 1312 KB, 3335 KB/s, 0 seconds passed … 37%, 1344 KB, 3410 KB/s, 0 seconds passed … 38%, 1376 KB, 3484 KB/s, 0 seconds passed
... 39%, 1408 KB, 3355 KB/s, 0 seconds passed
… 40%, 1440 KB, 3428 KB/s, 0 seconds passed … 41%, 1472 KB, 3436 KB/s, 0 seconds passed … 42%, 1504 KB, 3505 KB/s, 0 seconds passed … 43%, 1536 KB, 3383 KB/s, 0 seconds passed … 44%, 1568 KB, 3442 KB/s, 0 seconds passed … 45%, 1600 KB, 3457 KB/s, 0 seconds passed … 45%, 1632 KB, 3518 KB/s, 0 seconds passed
... 46%, 1664 KB, 3404 KB/s, 0 seconds passed
… 47%, 1696 KB, 3462 KB/s, 0 seconds passed … 48%, 1728 KB, 3475 KB/s, 0 seconds passed … 49%, 1760 KB, 3532 KB/s, 0 seconds passed
... 50%, 1792 KB, 3427 KB/s, 0 seconds passed
… 51%, 1824 KB, 3480 KB/s, 0 seconds passed … 52%, 1856 KB, 3491 KB/s, 0 seconds passed … 53%, 1888 KB, 3545 KB/s, 0 seconds passed … 54%, 1920 KB, 3444 KB/s, 0 seconds passed … 54%, 1952 KB, 3493 KB/s, 0 seconds passed … 55%, 1984 KB, 3505 KB/s, 0 seconds passed … 56%, 2016 KB, 3555 KB/s, 0 seconds passed
... 57%, 2048 KB, 3457 KB/s, 0 seconds passed
… 58%, 2080 KB, 3466 KB/s, 0 seconds passed … 59%, 2112 KB, 3515 KB/s, 0 seconds passed … 60%, 2144 KB, 3562 KB/s, 0 seconds passed
... 61%, 2176 KB, 3471 KB/s, 0 seconds passed
… 62%, 2208 KB, 3480 KB/s, 0 seconds passed … 63%, 2240 KB, 3525 KB/s, 0 seconds passed … 64%, 2272 KB, 3570 KB/s, 0 seconds passed … 64%, 2304 KB, 3484 KB/s, 0 seconds passed … 65%, 2336 KB, 3492 KB/s, 0 seconds passed … 66%, 2368 KB, 3533 KB/s, 0 seconds passed … 67%, 2400 KB, 3577 KB/s, 0 seconds passed
... 68%, 2432 KB, 3494 KB/s, 0 seconds passed
… 69%, 2464 KB, 3501 KB/s, 0 seconds passed … 70%, 2496 KB, 3543 KB/s, 0 seconds passed … 71%, 2528 KB, 3585 KB/s, 0 seconds passed
... 72%, 2560 KB, 3503 KB/s, 0 seconds passed
… 73%, 2592 KB, 3509 KB/s, 0 seconds passed … 73%, 2624 KB, 3549 KB/s, 0 seconds passed … 74%, 2656 KB, 3589 KB/s, 0 seconds passed … 75%, 2688 KB, 3512 KB/s, 0 seconds passed … 76%, 2720 KB, 3518 KB/s, 0 seconds passed … 77%, 2752 KB, 3557 KB/s, 0 seconds passed
... 78%, 2784 KB, 3596 KB/s, 0 seconds passed
… 79%, 2816 KB, 3525 KB/s, 0 seconds passed … 80%, 2848 KB, 3531 KB/s, 0 seconds passed … 81%, 2880 KB, 3564 KB/s, 0 seconds passed … 82%, 2912 KB, 3601 KB/s, 0 seconds passed
... 82%, 2944 KB, 3529 KB/s, 0 seconds passed
… 83%, 2976 KB, 3534 KB/s, 0 seconds passed … 84%, 3008 KB, 3569 KB/s, 0 seconds passed … 85%, 3040 KB, 3508 KB/s, 0 seconds passed … 86%, 3072 KB, 3536 KB/s, 0 seconds passed
... 87%, 3104 KB, 3539 KB/s, 0 seconds passed
… 88%, 3136 KB, 3573 KB/s, 0 seconds passed … 89%, 3168 KB, 3608 KB/s, 0 seconds passed … 90%, 3200 KB, 3544 KB/s, 0 seconds passed … 91%, 3232 KB, 3549 KB/s, 0 seconds passed … 91%, 3264 KB, 3578 KB/s, 0 seconds passed … 92%, 3296 KB, 3612 KB/s, 0 seconds passed
... 93%, 3328 KB, 3553 KB/s, 0 seconds passed
… 94%, 3360 KB, 3555 KB/s, 0 seconds passed … 95%, 3392 KB, 3583 KB/s, 0 seconds passed … 96%, 3424 KB, 3529 KB/s, 0 seconds passed … 97%, 3456 KB, 3554 KB/s, 0 seconds passed
... 98%, 3488 KB, 3558 KB/s, 0 seconds passed
… 99%, 3520 KB, 3588 KB/s, 0 seconds passed … 100%, 3549 KB, 3616 KB/s, 0 seconds passed
################|| Downloading person-reidentification-retail-0287 ||################
========== Downloading model/intel/person-reidentification-retail-0287/person-reidentification-retail-0267.onnx
... 0%, 32 KB, 1173 KB/s, 0 seconds passed
... 1%, 64 KB, 1062 KB/s, 0 seconds passed
… 2%, 96 KB, 1556 KB/s, 0 seconds passed … 3%, 128 KB, 1333 KB/s, 0 seconds passed … 4%, 160 KB, 1577 KB/s, 0 seconds passed
... 5%, 192 KB, 1482 KB/s, 0 seconds passed
… 6%, 224 KB, 1719 KB/s, 0 seconds passed … 7%, 256 KB, 1959 KB/s, 0 seconds passed … 8%, 288 KB, 2199 KB/s, 0 seconds passed
... 9%, 320 KB, 1952 KB/s, 0 seconds passed
… 10%, 352 KB, 2136 KB/s, 0 seconds passed … 11%, 384 KB, 2325 KB/s, 0 seconds passed … 11%, 416 KB, 2445 KB/s, 0 seconds passed … 12%, 448 KB, 2257 KB/s, 0 seconds passed … 13%, 480 KB, 2408 KB/s, 0 seconds passed … 14%, 512 KB, 2564 KB/s, 0 seconds passed
... 15%, 544 KB, 2428 KB/s, 0 seconds passed
… 16%, 576 KB, 2473 KB/s, 0 seconds passed … 17%, 608 KB, 2602 KB/s, 0 seconds passed … 18%, 640 KB, 2734 KB/s, 0 seconds passed … 19%, 672 KB, 2867 KB/s, 0 seconds passed
... 20%, 704 KB, 2632 KB/s, 0 seconds passed
… 21%, 736 KB, 2745 KB/s, 0 seconds passed … 22%, 768 KB, 2859 KB/s, 0 seconds passed … 22%, 800 KB, 2974 KB/s, 0 seconds passed … 23%, 832 KB, 2755 KB/s, 0 seconds passed … 24%, 864 KB, 2856 KB/s, 0 seconds passed … 25%, 896 KB, 2957 KB/s, 0 seconds passed … 26%, 928 KB, 3058 KB/s, 0 seconds passed
... 27%, 960 KB, 2853 KB/s, 0 seconds passed
… 28%, 992 KB, 2943 KB/s, 0 seconds passed … 29%, 1024 KB, 3034 KB/s, 0 seconds passed … 30%, 1056 KB, 3125 KB/s, 0 seconds passed
... 31%, 1088 KB, 2933 KB/s, 0 seconds passed
… 32%, 1120 KB, 3012 KB/s, 0 seconds passed … 33%, 1152 KB, 3095 KB/s, 0 seconds passed … 33%, 1184 KB, 3142 KB/s, 0 seconds passed … 34%, 1216 KB, 2999 KB/s, 0 seconds passed … 35%, 1248 KB, 3074 KB/s, 0 seconds passed … 36%, 1280 KB, 3148 KB/s, 0 seconds passed … 37%, 1312 KB, 3190 KB/s, 0 seconds passed
... 38%, 1344 KB, 3055 KB/s, 0 seconds passed
… 39%, 1376 KB, 3124 KB/s, 0 seconds passed … 40%, 1408 KB, 3191 KB/s, 0 seconds passed … 41%, 1440 KB, 3100 KB/s, 0 seconds passed
... 42%, 1472 KB, 3102 KB/s, 0 seconds passed
… 43%, 1504 KB, 3165 KB/s, 0 seconds passed … 44%, 1536 KB, 3228 KB/s, 0 seconds passed … 44%, 1568 KB, 3143 KB/s, 0 seconds passed … 45%, 1600 KB, 3144 KB/s, 0 seconds passed … 46%, 1632 KB, 3201 KB/s, 0 seconds passed … 47%, 1664 KB, 3260 KB/s, 0 seconds passed
... 48%, 1696 KB, 3180 KB/s, 0 seconds passed
… 49%, 1728 KB, 3180 KB/s, 0 seconds passed … 50%, 1760 KB, 3234 KB/s, 0 seconds passed … 51%, 1792 KB, 3289 KB/s, 0 seconds passed … 52%, 1824 KB, 3212 KB/s, 0 seconds passed
... 53%, 1856 KB, 3210 KB/s, 0 seconds passed
… 54%, 1888 KB, 3262 KB/s, 0 seconds passed … 55%, 1920 KB, 3314 KB/s, 0 seconds passed … 55%, 1952 KB, 3194 KB/s, 0 seconds passed … 56%, 1984 KB, 3241 KB/s, 0 seconds passed … 57%, 2016 KB, 3288 KB/s, 0 seconds passed … 58%, 2048 KB, 3337 KB/s, 0 seconds passed
... 59%, 2080 KB, 3266 KB/s, 0 seconds passed
… 60%, 2112 KB, 3266 KB/s, 0 seconds passed … 61%, 2144 KB, 3310 KB/s, 0 seconds passed … 62%, 2176 KB, 3357 KB/s, 0 seconds passed … 63%, 2208 KB, 3385 KB/s, 0 seconds passed
... 64%, 2240 KB, 3289 KB/s, 0 seconds passed
… 65%, 2272 KB, 3330 KB/s, 0 seconds passed … 66%, 2304 KB, 3373 KB/s, 0 seconds passed … 66%, 2336 KB, 3267 KB/s, 0 seconds passed … 67%, 2368 KB, 3306 KB/s, 0 seconds passed … 68%, 2400 KB, 3347 KB/s, 0 seconds passed … 69%, 2432 KB, 3389 KB/s, 0 seconds passed
... 70%, 2464 KB, 3290 KB/s, 0 seconds passed
… 71%, 2496 KB, 3325 KB/s, 0 seconds passed … 72%, 2528 KB, 3364 KB/s, 0 seconds passed … 73%, 2560 KB, 3405 KB/s, 0 seconds passed
... 74%, 2592 KB, 3308 KB/s, 0 seconds passed
… 75%, 2624 KB, 3341 KB/s, 0 seconds passed … 76%, 2656 KB, 3378 KB/s, 0 seconds passed … 77%, 2688 KB, 3417 KB/s, 0 seconds passed … 77%, 2720 KB, 3323 KB/s, 0 seconds passed … 78%, 2752 KB, 3357 KB/s, 0 seconds passed … 79%, 2784 KB, 3391 KB/s, 0 seconds passed … 80%, 2816 KB, 3429 KB/s, 0 seconds passed
... 81%, 2848 KB, 3339 KB/s, 0 seconds passed
… 82%, 2880 KB, 3370 KB/s, 0 seconds passed … 83%, 2912 KB, 3404 KB/s, 0 seconds passed … 84%, 2944 KB, 3440 KB/s, 0 seconds passed
... 85%, 2976 KB, 3354 KB/s, 0 seconds passed
… 86%, 3008 KB, 3385 KB/s, 0 seconds passed … 87%, 3040 KB, 3417 KB/s, 0 seconds passed … 88%, 3072 KB, 3450 KB/s, 0 seconds passed … 88%, 3104 KB, 3366 KB/s, 0 seconds passed … 89%, 3136 KB, 3396 KB/s, 0 seconds passed
... 90%, 3168 KB, 3406 KB/s, 0 seconds passed
… 91%, 3200 KB, 3439 KB/s, 0 seconds passed … 92%, 3232 KB, 3378 KB/s, 0 seconds passed … 93%, 3264 KB, 3408 KB/s, 0 seconds passed … 94%, 3296 KB, 3417 KB/s, 0 seconds passed … 95%, 3328 KB, 3448 KB/s, 0 seconds passed
... 96%, 3360 KB, 3390 KB/s, 0 seconds passed
… 97%, 3392 KB, 3395 KB/s, 0 seconds passed … 98%, 3424 KB, 3426 KB/s, 0 seconds passed … 99%, 3456 KB, 3456 KB/s, 0 seconds passed … 100%, 3487 KB, 3432 KB/s, 1 seconds passed
========== Downloading model/intel/person-reidentification-retail-0287/FP16/person-reidentification-retail-0287.xml
... 5%, 32 KB, 1388 KB/s, 0 seconds passed
… 10%, 64 KB, 1393 KB/s, 0 seconds passed … 15%, 96 KB, 2032 KB/s, 0 seconds passed
... 21%, 128 KB, 1852 KB/s, 0 seconds passed
… 26%, 160 KB, 2275 KB/s, 0 seconds passed … 31%, 192 KB, 2375 KB/s, 0 seconds passed … 37%, 224 KB, 2717 KB/s, 0 seconds passed … 42%, 256 KB, 2459 KB/s, 0 seconds passed
... 47%, 288 KB, 2508 KB/s, 0 seconds passed
… 53%, 320 KB, 2763 KB/s, 0 seconds passed … 58%, 352 KB, 3008 KB/s, 0 seconds passed … 63%, 384 KB, 2783 KB/s, 0 seconds passed … 69%, 416 KB, 2988 KB/s, 0 seconds passed … 74%, 448 KB, 2990 KB/s, 0 seconds passed … 79%, 480 KB, 3160 KB/s, 0 seconds passed
... 85%, 512 KB, 2953 KB/s, 0 seconds passed
… 90%, 544 KB, 2958 KB/s, 0 seconds passed … 95%, 576 KB, 3112 KB/s, 0 seconds passed … 100%, 600 KB, 3237 KB/s, 0 seconds passed
========== Downloading model/intel/person-reidentification-retail-0287/FP16/person-reidentification-retail-0287.bin
... 2%, 32 KB, 1362 KB/s, 0 seconds passed
… 5%, 64 KB, 1347 KB/s, 0 seconds passed … 8%, 96 KB, 1945 KB/s, 0 seconds passed
... 11%, 128 KB, 1797 KB/s, 0 seconds passed
… 13%, 160 KB, 2222 KB/s, 0 seconds passed … 16%, 192 KB, 2330 KB/s, 0 seconds passed … 19%, 224 KB, 2685 KB/s, 0 seconds passed … 22%, 256 KB, 2414 KB/s, 0 seconds passed … 24%, 288 KB, 2704 KB/s, 0 seconds passed
... 27%, 320 KB, 2743 KB/s, 0 seconds passed
… 30%, 352 KB, 2995 KB/s, 0 seconds passed … 33%, 384 KB, 2739 KB/s, 0 seconds passed … 36%, 416 KB, 2949 KB/s, 0 seconds passed … 38%, 448 KB, 2958 KB/s, 0 seconds passed … 41%, 480 KB, 3144 KB/s, 0 seconds passed
... 44%, 512 KB, 2920 KB/s, 0 seconds passed
… 47%, 544 KB, 2936 KB/s, 0 seconds passed … 49%, 576 KB, 3093 KB/s, 0 seconds passed … 52%, 608 KB, 3259 KB/s, 0 seconds passed … 55%, 640 KB, 3060 KB/s, 0 seconds passed … 58%, 672 KB, 3195 KB/s, 0 seconds passed
... 61%, 704 KB, 3192 KB/s, 0 seconds passed
… 63%, 736 KB, 3325 KB/s, 0 seconds passed … 66%, 768 KB, 3148 KB/s, 0 seconds passed … 69%, 800 KB, 3148 KB/s, 0 seconds passed … 72%, 832 KB, 3258 KB/s, 0 seconds passed … 74%, 864 KB, 3372 KB/s, 0 seconds passed
... 77%, 896 KB, 3219 KB/s, 0 seconds passed
… 80%, 928 KB, 3322 KB/s, 0 seconds passed … 83%, 960 KB, 3318 KB/s, 0 seconds passed … 86%, 992 KB, 3417 KB/s, 0 seconds passed … 88%, 1024 KB, 3269 KB/s, 0 seconds passed … 91%, 1056 KB, 3366 KB/s, 0 seconds passed
... 94%, 1088 KB, 3360 KB/s, 0 seconds passed
… 97%, 1120 KB, 3450 KB/s, 0 seconds passed … 99%, 1152 KB, 3319 KB/s, 0 seconds passed … 100%, 1153 KB, 3319 KB/s, 0 seconds passed
モデルのロード¶
モデルの読み込みと予測のための共通クラスを定義します。
OpenVINO モデルの初期化には主に 4 つのステップがあり、推論ループの前に 1 回だけ実行する必要があります。
- OpenVINO ランタイムを初期化します。
*.bin
および*.xml
ファイル (重みとアーキテクチャー) からネットワークを読み取ります。- デバイスのモデルをコンパイルします。
- ノードの入力名と出力名を取得します。
この場合、これらすべてをクラス・コンストラクター関数に配置できます。
OpenVINO が推論に最適なデバイスを自動的に選択するようにするには、AUTO
を使用します。ほとんどの場合、最適なデバイスは GPU
です (パフォーマンスは向上しますが、起動時間がわずかに長くなります)。
core = ov.Core()
class Model:
"""
This class represents a OpenVINO model object.
"""
def __init__(self, model_path, batchsize=1, device="AUTO"):
"""
Initialize the model object
Parameters
----------
model_path: path of inference model
batchsize: batch size of input data
device: device used to run inference
"""
self.model = core.read_model(model=model_path)
self.input_layer = self.model.input(0)
self.input_shape = self.input_layer.shape
self.height = self.input_shape[2]
self.width = self.input_shape[3]
for layer in self.model.inputs:
input_shape = layer.partial_shape
input_shape[0] = batchsize
self.model.reshape({layer: input_shape})
self.compiled_model = core.compile_model(model=self.model, device_name=device)
self.output_layer = self.compiled_model.output(0)
def predict(self, input):
"""
Run inference
Parameters
----------
input: array of input data
"""
result = self.compiled_model(input)[self.output_layer]
return result
OpenVINO を使用して推論を実行するためにドロップダウン・リストからデバイスを選択します。
import ipywidgets as widgets
device = widgets.Dropdown(
options=core.available_devices + ["AUTO"],
value='AUTO',
description='Device:',
disabled=False,
)
device
Dropdown(description='Device:', index=1, options=('CPU', 'AUTO'), value='AUTO')
detector = Model(detection_model_path, device=device.value)
# since the number of detection object is uncertain, the input batch size of reid model should be dynamic
extractor = Model(reidentification_model_path, -1, device.value)
データ処理¶
データ処理には、データの前処理機能と後処理機能が含まれます。
- データ前処理機能は、ネットワーク入力形式の要件に応じて、入力データのレイアウトと形状を変更するために使用されます。
- データ後処理機能は、ネットワークの元の出力から有用な情報を抽出し、視覚化するために使用されます。
def preprocess(frame, height, width):
"""
Preprocess a single image
Parameters
----------
frame: input frame
height: height of model input data
width: width of model input data
"""
resized_image = cv2.resize(frame, (width, height))
resized_image = resized_image.transpose((2, 0, 1))
input_image = np.expand_dims(resized_image, axis=0).astype(np.float32)
return input_image
def batch_preprocess(img_crops, height, width):
"""
Preprocess batched images
Parameters
----------
img_crops: batched input images
height: height of model input data
width: width of model input data
"""
img_batch = np.concatenate([
preprocess(img, height, width)
for img in img_crops
], axis=0)
return img_batch
def process_results(h, w, results, thresh=0.5):
"""
postprocess detection results
Parameters
----------
h, w: original height and width of input image
results: raw detection network output
thresh: threshold for low confidence filtering
"""
# The 'results' variable is a [1, 1, N, 7] tensor.
detections = results.reshape(-1, 7)
boxes = []
labels = []
scores = []
for i, detection in enumerate(detections):
_, label, score, xmin, ymin, xmax, ymax = detection
# Filter detected objects.
if score > thresh:
# Create a box with pixels coordinates from the box with normalized coordinates [0,1].
boxes.append(
[(xmin + xmax) / 2 * w, (ymin + ymax) / 2 * h, (xmax - xmin) * w, (ymax - ymin) * h]
)
labels.append(int(label))
scores.append(float(score))
if len(boxes) == 0:
boxes = np.array([]).reshape(0, 4)
scores = np.array([])
labels = np.array([])
return np.array(boxes), np.array(scores), np.array(labels)
def draw_boxes(img, bbox, identities=None):
"""
Draw bounding box in original image
Parameters
----------
img: original image
bbox: coordinate of bounding box
identities: identities IDs
"""
for i, box in enumerate(bbox):
x1, y1, x2, y2 = [int(i) for i in box]
# box text and bar
id = int(identities[i]) if identities is not None else 0
color = compute_color_for_labels(id)
label = '{}{:d}'.format("", id)
t_size = cv2.getTextSize(label, cv2.FONT_HERSHEY_PLAIN, 2, 2)[0]
cv2.rectangle(img, (x1, y1), (x2, y2), color, 2)
cv2.rectangle(
img, (x1, y1), (x1 + t_size[0] + 3, y1 + t_size[1] + 4), color, -1)
cv2.putText(
img,
label,
(x1, y1 + t_size[1] + 4),
cv2.FONT_HERSHEY_PLAIN,
1.6,
[255, 255, 255],
2
)
return img
def cosin_metric(x1, x2):
"""
Calculate the consin distance of two vector
Parameters
----------
x1, x2: input vectors
"""
return np.dot(x1, x2) / (np.linalg.norm(x1) * np.linalg.norm(x2))
人物再識別モデルのテスト¶
再識別ネットワークは、reid_embedding
という名前の (1, 256)
形状のブロブを出力します。これは、コサイン距離を使用して他の記述子と比較できます。
base_file_link = 'https://storage.openvinotoolkit.org/repositories/openvino_notebooks/data/data/image/person_'
image_indices = ['1_1.png', '1_2.png', '2_1.png']
image_paths = [utils.download_file(base_file_link + image_index, directory='data') for image_index in image_indices]
image1, image2, image3 = [cv2.cvtColor(cv2.imread(str(image_path)), cv2.COLOR_BGR2RGB) for image_path in image_paths]
# Define titles with images.
data = {"Person 1": image1, "Person 2": image2, "Person 3": image3}
# Create a subplot to visualize images.
fig, axs = plt.subplots(1, len(data.items()), figsize=(5, 5))
# Fill the subplot.
for ax, (name, image) in zip(axs, data.items()):
ax.axis('off')
ax.set_title(name)
ax.imshow(image)
# Display an image.
plt.show(fig)
data/person_1_1.png: 0%| | 0.00/68.3k [00:00<?, ?B/s]
data/person_1_2.png: 0%| | 0.00/68.9k [00:00<?, ?B/s]
data/person_2_1.png: 0%| | 0.00/70.3k [00:00<?, ?B/s]
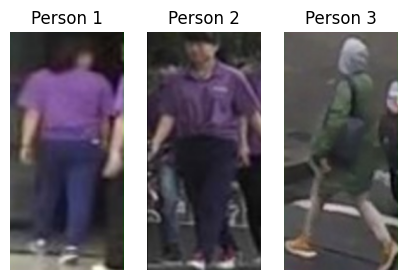
# Metric parameters
MAX_COSINE_DISTANCE = 0.6 # threshold of matching object
input_data = [image2, image3]
img_batch = batch_preprocess(input_data, extractor.height, extractor.width)
features = extractor.predict(img_batch)
sim = cosin_metric(features[0], features[1])
if sim >= 1 - MAX_COSINE_DISTANCE:
print(f'Same person (confidence: {sim})')
else:
print(f'Different person (confidence: {sim})')
Different person (confidence: 0.02726624347269535)
メイン処理関数¶
指定されたソースで人物追跡を実行します。ウェブカメラのフィードまたはビデオファイルのいずれか。
# Main processing function to run person tracking.
def run_person_tracking(source=0, flip=False, use_popup=False, skip_first_frames=0):
"""
Main function to run the person tracking:
1. Create a video player to play with target fps (utils.VideoPlayer).
2. Prepare a set of frames for person tracking.
3. Run AI inference for person tracking.
4. Visualize the results.
Parameters:
----------
source: The webcam number to feed the video stream with primary webcam set to "0", or the video path.
flip: To be used by VideoPlayer function for flipping capture image.
use_popup: False for showing encoded frames over this notebook, True for creating a popup window.
skip_first_frames: Number of frames to skip at the beginning of the video.
"""
player = None
try:
# Create a video player to play with target fps.
player = utils.VideoPlayer(
source=source, size=(700, 450), flip=flip, fps=24, skip_first_frames=skip_first_frames
)
# Start capturing.
player.start()
if use_popup:
title = "Press ESC to Exit"
cv2.namedWindow(
winname=title, flags=cv2.WINDOW_GUI_NORMAL | cv2.WINDOW_AUTOSIZE
)
processing_times = collections.deque()
while True:
# Grab the frame.
frame = player.next()
if frame is None:
print("Source ended")
break
# If the frame is larger than full HD, reduce size to improve the performance.
# Resize the image and change dims to fit neural network input.
h, w = frame.shape[:2]
input_image = preprocess(frame, detector.height, detector.width)
# Measure processing time.
start_time = time.time()
# Get the results.
output = detector.predict(input_image)
stop_time = time.time()
processing_times.append(stop_time - start_time)
if len(processing_times) > 200:
processing_times.popleft()
_, f_width = frame.shape[:2]
# Mean processing time [ms].
processing_time = np.mean(processing_times) * 1100
fps = 1000 / processing_time
# Get poses from detection results.
bbox_xywh, score, label = process_results(h, w, results=output)
img_crops = []
for box in bbox_xywh:
x1, y1, x2, y2 = xywh_to_xyxy(box, h, w)
img = frame[y1:y2, x1:x2]
img_crops.append(img)
# Get reidentification feature of each person.
if img_crops:
# preprocess
img_batch = batch_preprocess(img_crops, extractor.height, extractor.width)
features = extractor.predict(img_batch)
else:
features = np.array([])
# Wrap the detection and reidentification results together
bbox_tlwh = xywh_to_tlwh(bbox_xywh)
detections = [
Detection(bbox_tlwh[i], features[i])
for i in range(features.shape[0])
]
# predict the position of tracking target
tracker.predict()
# update tracker
tracker.update(detections)
# update bbox identities
outputs = []
for track in tracker.tracks:
if not track.is_confirmed() or track.time_since_update > 1:
continue
box = track.to_tlwh()
x1, y1, x2, y2 = tlwh_to_xyxy(box, h, w)
track_id = track.track_id
outputs.append(np.array([x1, y1, x2, y2, track_id], dtype=np.int32))
if len(outputs) > 0:
outputs = np.stack(outputs, axis=0)
# draw box for visualization
if len(outputs) > 0:
bbox_tlwh = []
bbox_xyxy = outputs[:, :4]
identities = outputs[:, -1]
frame = draw_boxes(frame, bbox_xyxy, identities)
cv2.putText(
img=frame,
text=f"Inference time: {processing_time:.1f}ms ({fps:.1f} FPS)",
org=(20, 40),
fontFace=cv2.FONT_HERSHEY_COMPLEX,
fontScale=f_width / 1000,
color=(0, 0, 255),
thickness=1,
lineType=cv2.LINE_AA,
)
if use_popup:
cv2.imshow(winname=title, mat=frame)
key = cv2.waitKey(1)
# escape = 27
if key == 27:
break
else:
# Encode numpy array to jpg.
_, encoded_img = cv2.imencode(
ext=".jpg", img=frame, params=[cv2.IMWRITE_JPEG_QUALITY, 100]
)
# Create an IPython image.
i = display.Image(data=encoded_img)
# Display the image in this notebook.
display.clear_output(wait=True)
display.display(i)
# ctrl-c
except KeyboardInterrupt:
print("Interrupted")
# any different error
except RuntimeError as e:
print(e)
finally:
if player is not None:
# Stop capturing.
player.stop()
if use_popup:
cv2.destroyAllWindows()
実行¶
新しい追跡タスクを実行する前に、トラッカー・オブジェクトを再初期化する必要があります。
NN_BUDGET = 100
MAX_COSINE_DISTANCE = 0.6 # threshold of matching object
metric = NearestNeighborDistanceMetric(
"cosine", MAX_COSINE_DISTANCE, NN_BUDGET
)
tracker = Tracker(
metric,
max_iou_distance=0.7,
max_age=70,
n_init=3
)
ウェブカメラをビデオ入力として使用します。デフォルトでは、プライマリー・ウェブカメラは source=0
に設定されます。複数のウェブカメラがある場合、0 から始まる連続した番号が割り当てられます。前面カメラを使用する場合は、flip=True
を設定します。一部のウェブブラウザー、特に Mozilla Firefox ではちらつきが発生する場合があります。ちらつきが発生する場合は、use_popup=True
を設定してください。
ウェブカメラがない場合でも、ビデオファイルを使用してこのデモを実行できます。OpenCV でサポートされている形式であればどれでも機能します。
USE_WEBCAM = False
cam_id = 0
video_file = 'https://storage.openvinotoolkit.org/repositories/openvino_notebooks/data/data/video/people.mp4'
source = cam_id if USE_WEBCAM else video_file
run_person_tracking(source=source, flip=USE_WEBCAM, use_popup=False)
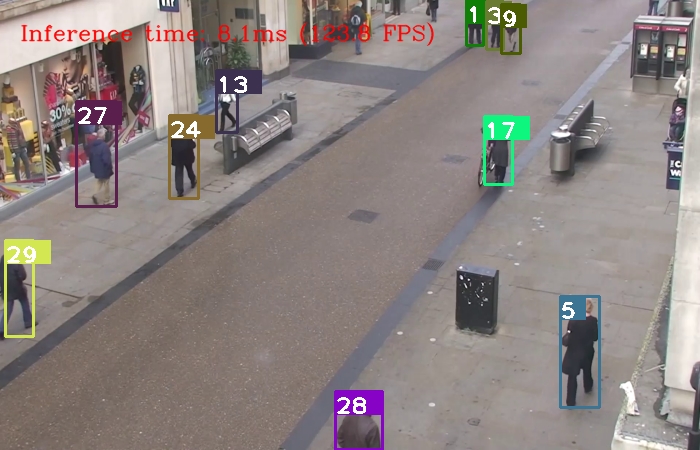
Source ended