FastComposer: 局所的な注意によるチューニング不要の複数被写体の画像生成¶
この Jupyter ノートブックは、ローカルへのインストール後にのみ起動できます。
FastComposer は、画像エンコーダーで抽出された被写体の埋め込みを使用して、拡散モデルの一般的なテキスト条件付けを拡張し、フォワードパスのみで被写体の画像とテキスト指示に基づいてパーソナライズされた画像の生成を可能にします。さらに、次の 2 つの問題にも対処します。
アイデンティティーの混合問題。複数被写体生成の問題に対処するため、トレーニング中の交差注意定位監視を提案し、ターゲット画像内の正しい領域に定位した参照被写体の注意を強化します。
被写体の過剰適合。単純に被写体の埋め込みを条件付けると、被写体の過剰適合が発生します。FastComposer は、被写体主導の画像生成においてアイデンティティーと編集可能性の両方を維持するため、ノイズ除去ステップで被写体の調整を遅延させることを提案します。
FastComposer は、さまざまなスタイル、アクション、コンテキストを持つ、非可視な複数の人物のイメージを生成します。
注:
model.py
は fastcomposer リポジトリーのmodel.py
を変更したものです。主な変更点は 2 つあります。
- システムに CUDA ドライバーがない場合、エラーを回避するため、一部の未使用のコード行が削除されています
- トランスフォーマー 4.30.1 以上との互換性を持たせるための変更 (セキュリティーの脆弱性のため)
目次¶
このチュートリアルでは、1 枚の画像を生成するのに約 25~28GB の空きメモリーを必要とします。画像を 1 枚追加するごとに ~11GB の空きメモリーが必要です。
インストールの要件¶
必要なパッケージをインストールします。
%pip install -q --upgrade pip
%pip install -q --extra-index-url https://download.pytorch.org/whl/cpu torch torchvision
%pip install -q transformers huggingface-hub accelerate "diffusers>=0.16.1" gradio
%pip install -q "openvino>=2023.1.0"
Note: you may need to restart the kernel to use updated packages.
Note: you may need to restart the kernel to use updated packages.
Note: you may need to restart the kernel to use updated packages.
Note: you may need to restart the kernel to use updated packages.
GitHub から FastComposer プロジェクトをクローンします。
from pathlib import Path
# clone FastComposer repo
if not Path("fastcomposer").exists():
!git clone https://github.com/mit-han-lab/fastcomposer.git
else:
print("FastComposer repo already cloned")
FastComposer repo already cloned
事前トレーニング済みモデルをダウンロードします。
from huggingface_hub import hf_hub_download
model_path = hf_hub_download(repo_id='mit-han-lab/fastcomposer', filename='pytorch_model.bin')
モデルを OpenVINO 中間表現 (IR) 形式に変換¶
構成を定義し、FastComposerModel
のインスタンスを作成します。
from model import FastComposerModel
from dataclasses import dataclass
import torch
@dataclass()
class Config:
finetuned_model_path = str(model_path)
image_encoder_name_or_path = 'openai/clip-vit-large-patch14'
localization_layers = 5
mask_loss = False
mask_loss_prob = 0.5
non_ema_revision = None
object_localization = False
object_localization_weight = 0.01
object_resolution = 256
pretrained_model_name_or_path = 'runwayml/stable-diffusion-v1-5'
revision = None
config = Config()
model = FastComposerModel.from_pretrained(config)
model.load_state_dict(torch.load(config.finetuned_model_path, map_location="cpu"), strict=False)
text_config_dict is provided which will be used to initialize CLIPTextConfig. The value text_config["id2label"] will be overriden. text_config_dict is provided which will be used to initialize CLIPTextConfig. The value text_config["bos_token_id"] will be overriden. text_config_dict is provided which will be used to initialize CLIPTextConfig. The value text_config["eos_token_id"] will be overriden.
_IncompatibleKeys(missing_keys=['vae.encoder.mid_block.attentions.0.to_q.weight', 'vae.encoder.mid_block.attentions.0.to_q.bias', 'vae.encoder.mid_block.attentions.0.to_k.weight', 'vae.encoder.mid_block.attentions.0.to_k.bias', 'vae.encoder.mid_block.attentions.0.to_v.weight', 'vae.encoder.mid_block.attentions.0.to_v.bias', 'vae.encoder.mid_block.attentions.0.to_out.0.weight', 'vae.encoder.mid_block.attentions.0.to_out.0.bias', 'vae.decoder.mid_block.attentions.0.to_q.weight', 'vae.decoder.mid_block.attentions.0.to_q.bias', 'vae.decoder.mid_block.attentions.0.to_k.weight', 'vae.decoder.mid_block.attentions.0.to_k.bias', 'vae.decoder.mid_block.attentions.0.to_v.weight', 'vae.decoder.mid_block.attentions.0.to_v.bias', 'vae.decoder.mid_block.attentions.0.to_out.0.weight', 'vae.decoder.mid_block.attentions.0.to_out.0.bias'], unexpected_keys=['text_encoder.embeddings.position_ids', 'image_encoder.vision_model.embeddings.position_ids', 'vae.encoder.mid_block.attentions.0.query.weight', 'vae.encoder.mid_block.attentions.0.query.bias', 'vae.encoder.mid_block.attentions.0.key.weight', 'vae.encoder.mid_block.attentions.0.key.bias', 'vae.encoder.mid_block.attentions.0.value.weight', 'vae.encoder.mid_block.attentions.0.value.bias', 'vae.encoder.mid_block.attentions.0.proj_attn.weight', 'vae.encoder.mid_block.attentions.0.proj_attn.bias', 'vae.decoder.mid_block.attentions.0.query.weight', 'vae.decoder.mid_block.attentions.0.query.bias', 'vae.decoder.mid_block.attentions.0.key.weight', 'vae.decoder.mid_block.attentions.0.key.bias', 'vae.decoder.mid_block.attentions.0.value.weight', 'vae.decoder.mid_block.attentions.0.value.bias', 'vae.decoder.mid_block.attentions.0.proj_attn.weight', 'vae.decoder.mid_block.attentions.0.proj_attn.bias'])
パイプラインは、Unet
、TextEncoder
、ImageEncoder
、PostfuseModule
(MLP)、object_transforms
のモデルで構成されます。
推論パイプライン¶
モデルを OpenVINO IR 形式に変換します。
テキスト・エンコーダーを変換¶
モデル・コンポーネントは PyTorch モジュールであり、openvino.convert_model 関数を使用して直接変換できます。また、変換結果をシリアル化するため openvino.save_model 関数も使用します。ヘルパー関数を作成します。
import gc
import openvino
def convert(model: torch.nn.Module, xml_path: str, example_input):
xml_path = Path(xml_path)
if not xml_path.exists():
xml_path.parent.mkdir(parents=True, exist_ok=True)
with torch.no_grad():
converted_model = openvino.convert_model(model, example_input=example_input)
openvino.save_model(converted_model, xml_path)
# cleanup memory
torch._C._jit_clear_class_registry()
torch.jit._recursive.concrete_type_store = torch.jit._recursive.ConcreteTypeStore()
torch.jit._state._clear_class_state()
テキスト・エンコーダーは、入力プロンプトを次のステージの U-Net に送ることができる埋め込みスペースに変換する役割を担います。通常、これは、入力トークンのシーケンスをテキスト埋め込みのシーケンスにマッピングするトランスフォーマー・ベースのエンコーダーです。
テキスト・エンコーダーの入力は、トークナイザーによって処理され、モデルが受け入れる最大長にパディングされたテキストのトークン・インデックスを含むテンソル input_ids
で構成されます。
text_encoder_ir_xml_path = Path('models/text_encoder_ir.xml')
example_input = torch.zeros((1, 77), dtype=torch.int64)
model.text_encoder.eval()
convert(model.text_encoder, text_encoder_ir_xml_path, example_input)
del model.text_encoder
gc.collect();
/home/ea/work/my_optimum_intel/optimum_env/lib/python3.8/site-packages/transformers/models/clip/modeling_clip.py:273: TracerWarning: Converting a tensor to a Python boolean might cause the trace to be incorrect. We can't record the data flow of Python values, so this value will be treated as a constant in the future. This means that the trace might not generalize to other inputs!
if attn_weights.size() != (bsz * self.num_heads, tgt_len, src_len):
/home/ea/work/my_optimum_intel/optimum_env/lib/python3.8/site-packages/transformers/models/clip/modeling_clip.py:281: TracerWarning: Converting a tensor to a Python boolean might cause the trace to be incorrect. We can't record the data flow of Python values, so this value will be treated as a constant in the future. This means that the trace might not generalize to other inputs!
if causal_attention_mask.size() != (bsz, 1, tgt_len, src_len):
/home/ea/work/my_optimum_intel/optimum_env/lib/python3.8/site-packages/transformers/models/clip/modeling_clip.py:313: TracerWarning: Converting a tensor to a Python boolean might cause the trace to be incorrect. We can't record the data flow of Python values, so this value will be treated as a constant in the future. This means that the trace might not generalize to other inputs!
if attn_output.size() != (bsz * self.num_heads, tgt_len, self.head_dim):
オブジェクト変換¶
受け取ったユーザー画像をパディングして正方形にし、サイズを変更します。入力はサイズ [3、高さ、幅] のテンソルです。
from collections import OrderedDict
from torchvision import transforms as T
from fastcomposer.fastcomposer.transforms import PadToSquare
object_transforms = torch.nn.Sequential(
OrderedDict(
[
("pad_to_square", PadToSquare(fill=0, padding_mode="constant")),
(
"resize",
T.Resize(
(config.object_resolution, config.object_resolution),
interpolation=T.InterpolationMode.BILINEAR,
antialias=True,
),
),
("convert_to_float", T.ConvertImageDtype(torch.float32)),
]
)
)
object_transforms_ir_xml_path = Path('models/object_transforms_ir.xml')
example_input = torch.zeros([3, 1500, 1453], dtype=torch.uint8)
object_transforms.eval()
convert(object_transforms, object_transforms_ir_xml_path, example_input)
del object_transforms
gc.collect();
/home/ea/work/openvino_notebooks/notebooks/252-fastcomposer-image-generation/fastcomposer/fastcomposer/transforms.py:35: TracerWarning: Converting a tensor to a Python boolean might cause the trace to be incorrect. We can't record the data flow of Python values, so this value will be treated as a constant in the future. This means that the trace might not generalize to other inputs!
if h == w:
/home/ea/work/openvino_notebooks/notebooks/252-fastcomposer-image-generation/fastcomposer/fastcomposer/transforms.py:37: TracerWarning: Converting a tensor to a Python boolean might cause the trace to be incorrect. We can't record the data flow of Python values, so this value will be treated as a constant in the future. This means that the trace might not generalize to other inputs!
elif h > w:
0
画像エンコーダー¶
画像エンコーダーは、CLIP (Contrastive Language-Image Pretraining) の画像エンコーダーです。前のステップで変換された画像を受け取り、高次元のベクトルまたは埋め込みに変換します。
image_encoder_ir_xml_path = Path('models/image_encoder_ir.xml')
example_input = torch.zeros((1, 2, 3, 256, 256), dtype=torch.float32)
model.image_encoder.eval()
convert(model.image_encoder, image_encoder_ir_xml_path, example_input)
del model.image_encoder
gc.collect();
/home/ea/work/openvino_notebooks/notebooks/252-fastcomposer-image-generation/model.py:108: TracerWarning: Converting a tensor to a Python boolean might cause the trace to be incorrect. We can't record the data flow of Python values, so this value will be treated as a constant in the future. This means that the trace might not generalize to other inputs!
if h != self.image_size or w != self.image_size:
ポストフューズ・モジュール¶
このステップでは、マルチレイヤー・パーセプトロン (MLP) を使用して、参照対象から抽出された視覚的特徴でテキスト埋め込みを拡張します。Postfuse モジュールは、単語の埋め込みと視覚的特徴を連結し、拡張された埋め込みを MLP に送ります。
postfuse_module_ir_xml_path = Path('models/postfuse_module_ir.xml')
example_input = [
torch.zeros((1, 77, 768), dtype=torch.float32),
torch.zeros((1, 2, 1, 768), dtype=torch.float32),
torch.zeros((1, 77), dtype=torch.bool),
torch.zeros((1,), dtype=torch.int64)
]
model.postfuse_module.eval()
convert(model.postfuse_module, postfuse_module_ir_xml_path, example_input)
del model.postfuse_module
gc.collect();
Unet を変換¶
U-Net モデルは、テキスト・エンコーダーの隠れ状態に基づいて、潜在画像表現のノイズを段階的に除去します。
unet_ir_xml_path = Path('models/unet_ir.xml')
example_input = [
torch.zeros((8, 4, 64, 64), dtype=torch.float32),
torch.zeros((), dtype=torch.int64),
torch.zeros((8, 77, 768), dtype=torch.float32)
]
model.unet.eval()
convert(model.unet, unet_ir_xml_path, example_input)
del model
del example_input
gc.collect()
/home/ea/work/my_optimum_intel/optimum_env/lib/python3.8/site-packages/diffusers/models/unet_2d_condition.py:915: TracerWarning: Converting a tensor to a Python boolean might cause the trace to be incorrect. We can't record the data flow of Python values, so this value will be treated as a constant in the future. This means that the trace might not generalize to other inputs!
if dim % default_overall_up_factor != 0:
/home/ea/work/my_optimum_intel/optimum_env/lib/python3.8/site-packages/peft/tuners/loha/layer.py:303: TracerWarning: torch.tensor results are registered as constants in the trace. You can safely ignore this warning if you use this function to create tensors out of constant variables that would be the same every time you call this function. In any other case, this might cause the trace to be incorrect.
def forward(ctx, w1a, w1b, w2a, w2b, scale=torch.tensor(1)):
/home/ea/work/my_optimum_intel/optimum_env/lib/python3.8/site-packages/peft/tuners/loha/layer.py:326: TracerWarning: torch.tensor results are registered as constants in the trace. You can safely ignore this warning if you use this function to create tensors out of constant variables that would be the same every time you call this function. In any other case, this might cause the trace to be incorrect.
def forward(ctx, t1, w1a, w1b, t2, w2a, w2b, scale=torch.tensor(1)):
/home/ea/work/my_optimum_intel/optimum_env/lib/python3.8/site-packages/diffusers/models/downsampling.py:135: TracerWarning: Converting a tensor to a Python boolean might cause the trace to be incorrect. We can't record the data flow of Python values, so this value will be treated as a constant in the future. This means that the trace might not generalize to other inputs!
assert hidden_states.shape[1] == self.channels
/home/ea/work/my_optimum_intel/optimum_env/lib/python3.8/site-packages/diffusers/models/downsampling.py:144: TracerWarning: Converting a tensor to a Python boolean might cause the trace to be incorrect. We can't record the data flow of Python values, so this value will be treated as a constant in the future. This means that the trace might not generalize to other inputs!
assert hidden_states.shape[1] == self.channels
/home/ea/work/my_optimum_intel/optimum_env/lib/python3.8/site-packages/diffusers/models/upsampling.py:149: TracerWarning: Converting a tensor to a Python boolean might cause the trace to be incorrect. We can't record the data flow of Python values, so this value will be treated as a constant in the future. This means that the trace might not generalize to other inputs!
assert hidden_states.shape[1] == self.channels
/home/ea/work/my_optimum_intel/optimum_env/lib/python3.8/site-packages/diffusers/models/upsampling.py:165: TracerWarning: Converting a tensor to a Python boolean might cause the trace to be incorrect. We can't record the data flow of Python values, so this value will be treated as a constant in the future. This means that the trace might not generalize to other inputs!
if hidden_states.shape[0] >= 64:
16724
パイプラインを再構築¶
また、OpenVINO モデルを使用するには、内部 FastComposer エンティティーをいくつか変更する必要があります。最初に、どうやって結果を出すか。例えば、出力を numpy から torch タイプに変換します。
import numpy as np
from diffusers.pipelines.stable_diffusion import StableDiffusionPipelineOutput
from diffusers.pipelines.stable_diffusion import StableDiffusionPipeline
from diffusers.loaders import TextualInversionLoaderMixin
from typing import Any, Callable, Dict, List, Optional, Union
from PIL import Image
class StableDiffusionFastCompposerPipeline(StableDiffusionPipeline):
r"""
Pipeline for text-to-image generation using FastComposer (https://arxiv.org/abs/2305.10431).
This model inherits from [`StableDiffusionPipeline`]. Check the superclass documentation for the generic methods the
library implements for all the pipelines (such as downloading or saving, running on a particular device, etc.)
"""
@torch.no_grad()
def _tokenize_and_mask_noun_phrases_ends(self, caption):
input_ids = self.special_tokenizer.encode(caption)
noun_phrase_end_mask = [False for _ in input_ids]
clean_input_ids = []
clean_index = 0
for i, id in enumerate(input_ids):
if id == self.image_token_id:
noun_phrase_end_mask[clean_index - 1] = True
else:
clean_input_ids.append(id)
clean_index += 1
max_len = self.special_tokenizer.model_max_length
if len(clean_input_ids) > max_len:
clean_input_ids = clean_input_ids[:max_len]
else:
clean_input_ids = clean_input_ids + [self.tokenizer.pad_token_id] * (
max_len - len(clean_input_ids)
)
if len(noun_phrase_end_mask) > max_len:
noun_phrase_end_mask = noun_phrase_end_mask[:max_len]
else:
noun_phrase_end_mask = noun_phrase_end_mask + [False] * (
max_len - len(noun_phrase_end_mask)
)
clean_input_ids = torch.tensor(clean_input_ids, dtype=torch.long)
noun_phrase_end_mask = torch.tensor(noun_phrase_end_mask, dtype=torch.bool)
return clean_input_ids.unsqueeze(0), noun_phrase_end_mask.unsqueeze(0)
@torch.no_grad()
def _encode_augmented_prompt(self, prompt: str, reference_images: List[Image.Image], device: torch.device, weight_dtype: torch.dtype):
# TODO: check this
# encode reference images
object_pixel_values = []
for image in reference_images:
image_tensor = torch.from_numpy(np.array(image.convert("RGB"))).permute(2, 0, 1)
image = torch.from_numpy((self.object_transforms(image_tensor)[0]))
object_pixel_values.append(image)
object_pixel_values = torch.stack(object_pixel_values, dim=0).to(memory_format=torch.contiguous_format).float()
object_pixel_values = object_pixel_values.unsqueeze(0).to(dtype=torch.float32, device=device)
object_embeds = self.image_encoder(object_pixel_values)[0]
object_embeds = torch.from_numpy(object_embeds)
# augment the text embedding
input_ids, image_token_mask = self._tokenize_and_mask_noun_phrases_ends(prompt)
input_ids, image_token_mask = input_ids.to(device), image_token_mask.to(device)
num_objects = image_token_mask.sum(dim=1)
text_embeds = torch.from_numpy(self.text_encoder(input_ids)[0])
augmented_prompt_embeds = self.postfuse_module([
text_embeds,
object_embeds,
image_token_mask,
num_objects
])[0]
return torch.from_numpy(augmented_prompt_embeds)
def _encode_prompt(
self,
prompt,
device,
num_images_per_prompt,
do_classifier_free_guidance,
negative_prompt=None
):
r"""
Encodes the prompt into text encoder hidden states.
Args:
prompt (`str` or `List[str]`, *optional*):
prompt to be encoded
device: (`torch.device`):
torch device
num_images_per_prompt (`int`):
number of images that should be generated per prompt
do_classifier_free_guidance (`bool`):
whether to use classifier free guidance or not
negative_prompt (`str` or `List[str]`, *optional*):
The prompt or prompts not to guide the image generation. If not defined, one has to pass
`negative_prompt_embeds` instead. Ignored when not using guidance (i.e., ignored if `guidance_scale` is
less than `1`).
"""
if isinstance(prompt, str):
batch_size = 1
elif isinstance(prompt, list):
batch_size = len(prompt)
# textual inversion: procecss multi-vector tokens if necessary
if isinstance(self, TextualInversionLoaderMixin):
prompt = self.maybe_convert_prompt(prompt, self.tokenizer)
text_inputs = self.tokenizer(
prompt,
padding="max_length",
max_length=self.tokenizer.model_max_length,
truncation=True,
return_tensors="pt",
)
text_input_ids = text_inputs.input_ids
untruncated_ids = self.tokenizer(prompt, padding="longest", return_tensors="pt").input_ids
if untruncated_ids.shape[-1] >= text_input_ids.shape[-1] and not torch.equal(
text_input_ids, untruncated_ids
):
removed_text = self.tokenizer.batch_decode(
untruncated_ids[:, self.tokenizer.model_max_length - 1 : -1]
)
print(
"The following part of your input was truncated because CLIP can only handle sequences up to"
f" {self.tokenizer.model_max_length} tokens: {removed_text}"
)
prompt_embeds = self.text_encoder(text_input_ids.to(device))[0]
prompt_embeds = torch.from_numpy(prompt_embeds)
bs_embed, seq_len, _ = prompt_embeds.shape
# duplicate text embeddings for each generation per prompt, using mps friendly method
prompt_embeds = prompt_embeds.repeat(1, num_images_per_prompt, 1)
prompt_embeds = prompt_embeds.view(bs_embed * num_images_per_prompt, seq_len, -1)
# get unconditional embeddings for classifier free guidance
if do_classifier_free_guidance:
uncond_tokens: List[str]
if negative_prompt is None:
uncond_tokens = [""] * batch_size
elif type(prompt) is not type(negative_prompt):
raise TypeError(
f"`negative_prompt` should be the same type to `prompt`, but got {type(negative_prompt)} !="
f" {type(prompt)}."
)
elif isinstance(negative_prompt, str):
uncond_tokens = [negative_prompt]
elif batch_size != len(negative_prompt):
raise ValueError(
f"`negative_prompt`: {negative_prompt} has batch size {len(negative_prompt)}, but `prompt`:"
f" {prompt} has batch size {batch_size}. Please make sure that passed `negative_prompt` matches"
" the batch size of `prompt`."
)
else:
uncond_tokens = negative_prompt
# textual inversion: procecss multi-vector tokens if necessary
if isinstance(self, TextualInversionLoaderMixin):
uncond_tokens = self.maybe_convert_prompt(uncond_tokens, self.tokenizer)
max_length = prompt_embeds.shape[1]
uncond_input = self.tokenizer(
uncond_tokens,
padding="max_length",
max_length=max_length,
truncation=True,
return_tensors="pt",
)
negative_prompt_embeds = self.text_encoder(uncond_input.input_ids.to(device))[0]
negative_prompt_embeds = torch.from_numpy(negative_prompt_embeds)
if do_classifier_free_guidance:
# duplicate unconditional embeddings for each generation per prompt, using mps friendly method
seq_len = negative_prompt_embeds.shape[1]
negative_prompt_embeds = negative_prompt_embeds.to(dtype=torch.float32, device=device)
negative_prompt_embeds = negative_prompt_embeds.repeat(1, num_images_per_prompt, 1)
negative_prompt_embeds = negative_prompt_embeds.view(batch_size * num_images_per_prompt, seq_len, -1)
# For classifier free guidance, we need to do two forward passes.
# Here we concatenate the unconditional and text embeddings into a single batch
# to avoid doing two forward passes
prompt_embeds = torch.cat([negative_prompt_embeds, prompt_embeds])
return prompt_embeds
@torch.no_grad()
def __call__(
self,
prompt: Union[str, List[str]] = None,
height: Optional[int] = None,
width: Optional[int] = None,
num_inference_steps: int = 50,
guidance_scale: float = 7.5,
negative_prompt: Optional[Union[str, List[str]]] = None,
num_images_per_prompt: Optional[int] = 1,
eta: float = 0.0,
generator: Optional[Union[torch.Generator, List[torch.Generator]]] = None,
latents: Optional[torch.FloatTensor] = None,
prompt_embeds: Optional[torch.FloatTensor] = None,
negative_prompt_embeds: Optional[torch.FloatTensor] = None,
output_type: Optional[str] = "pil",
return_dict: bool = True,
callback: Optional[Callable[[int, int, torch.FloatTensor], None]] = None,
callback_steps: int = 1,
cross_attention_kwargs: Optional[Dict[str, Any]] = None,
alpha_: float = 0.7,
reference_subject_images: List[Image.Image] = None,
augmented_prompt_embeds: Optional[torch.FloatTensor] = None
):
r"""
Function invoked when calling the pipeline for generation.
Args:
prompt (`str` or `List[str]`, *optional*):
The prompt or prompts to guide the image generation. If not defined, one has to pass `prompt_embeds`.
instead.
height (`int`, *optional*, defaults to self.unet.config.sample_size * self.vae_scale_factor):
The height in pixels of the generated image.
width (`int`, *optional*, defaults to self.unet.config.sample_size * self.vae_scale_factor):
The width in pixels of the generated image.
num_inference_steps (`int`, *optional*, defaults to 50):
The number of denoising steps. More denoising steps usually lead to a higher quality image at the
expense of slower inference.
guidance_scale (`float`, *optional*, defaults to 7.5):
Guidance scale as defined in [Classifier-Free Diffusion Guidance](https://arxiv.org/abs/2207.12598).
`guidance_scale` is defined as `w` of equation 2. of [Imagen
Paper](https://arxiv.org/pdf/2205.11487.pdf). Guidance scale is enabled by setting `guidance_scale >
1`. Higher guidance scale encourages to generate images that are closely linked to the text `prompt`,
usually at the expense of lower image quality.
negative_prompt (`str` or `List[str]`, *optional*):
The prompt or prompts not to guide the image generation. If not defined, one has to pass
`negative_prompt_embeds` instead. Ignored when not using guidance (i.e., ignored if `guidance_scale` is
less than `1`).
num_images_per_prompt (`int`, *optional*, defaults to 1):_unwrap_model
The number of images to generate per prompt.
eta (`float`, *optional*, defaults to 0.0):
Corresponds to parameter eta (η) in the DDIM paper: https://arxiv.org/abs/2010.02502. Only applies to
[`schedulers.DDIMScheduler`], will be ignored for others.
generator (`torch.Generator` or `List[torch.Generator]`, *optional*):
One or a list of [torch generator(s)](https://pytorch.org/docs/stable/generated/torch.Generator.html)
to make generation deterministic.
latents (`torch.FloatTensor`, *optional*):
Pre-generated noisy latents, sampled from a Gaussian distribution, to be used as inputs for image
generation. Can be used to tweak the same generation with different prompts. If not provided, a latents
tensor will ge generated by sampling using the supplied random `generator`.
prompt_embeds (`torch.FloatTensor`, *optional*):
Pre-generated text embeddings. Can be used to easily tweak text inputs, *e.g.* prompt weighting. If not
provided, text embeddings will be generated from `prompt` input argument.
negative_prompt_embeds (`torch.FloatTensor`, *optional*):
Pre-generated negative text embeddings. Can be used to easily tweak text inputs, *e.g.* prompt
weighting. If not provided, negative_prompt_embeds will be generated from `negative_prompt` input
argument.
output_type (`str`, *optional*, defaults to `"pil"`):
The output format of the generate image. Choose between
[PIL](https://pillow.readthedocs.io/en/stable/): `PIL.Image.Image` or `np.array`.
return_dict (`bool`, *optional*, defaults to `True`):
Whether or not to return a [`~pipelines.stable_diffusion.StableDiffusionPipelineOutput`] instead of a
plain tuple.
callback (`Callable`, *optional*):
A function that will be called every `callback_steps` steps during inference. The function will be
called with the following arguments: `callback(step: int, timestep: int, latents: torch.FloatTensor)`.
callback_steps (`int`, *optional*, defaults to 1):
The frequency at which the `callback` function will be called. If not specified, the callback will be
called at every step.
cross_attention_kwargs (`dict`, *optional*):
A kwargs dictionary that if specified is passed along to the `AttentionProcessor` as defined under
`self.processor` in
[diffusers.cross_attention](https://github.com/huggingface/diffusers/blob/main/src/diffusers/models/cross_attention.py).
alpha_ (`float`, defaults to 0.7):
The ratio of subject conditioning. If `alpha_` is 0.7, the beginning 30% of denoising steps use text prompts, while the
last 70% utilize image-augmented prompts. Increase alpha for identity preservation, decrease it for prompt consistency.
reference_subject_images (`List[PIL.Image.Image]`):
a list of PIL images that are used as reference subjects. The number of images should be equal to the number of augmented
tokens in the prompts.
augmented_prompt_embeds: (`torch.FloatTensor`, *optional*):
Pre-generated image augmented text embeddings. If not provided, embeddings will be generated from `prompt` and
`reference_subject_images`.
Examples:
Returns:
[`~pipelines.stable_diffusion.StableDiffusionPipelineOutput`] or `tuple`:
[`~pipelines.stable_diffusion.StableDiffusionPipelineOutput`] if `return_dict` is True, otherwise a `tuple.
When returning a tuple, the first element is a list with the generated images, and the second element is a
list of `bool`s denoting whether the corresponding generated image likely represents "not-safe-for-work"
(nsfw) content, according to the `safety_checker`.
"""
# 0. Default height and width to unet
height = height or self.unet.config.sample_size * self.vae_scale_factor
width = width or self.unet.config.sample_size * self.vae_scale_factor
# 1. Check inputs. Raise error if not correct
self.check_inputs(
prompt,
height,
width,
callback_steps,
negative_prompt,
prompt_embeds,
negative_prompt_embeds,
)
assert (prompt is not None and reference_subject_images is not None) or (prompt_embeds is not None and augmented_prompt_embeds is not None), \
"Prompt and reference subject images or prompt_embeds and augmented_prompt_embeds must be provided."
# 2. Define call parameters
if prompt is not None and isinstance(prompt, str):
batch_size = 1
elif prompt is not None and isinstance(prompt, list):
batch_size = len(prompt)
else:
batch_size = prompt_embeds.shape[0]
device = self._execution_device
# here `guidance_scale` is defined analog to the guidance weight `w` of equation (2)
# of the Imagen paper: https://arxiv.org/pdf/2205.11487.pdf . `guidance_scale = 1`
# corresponds to doing no classifier free guidance.
do_classifier_free_guidance = guidance_scale > 1.0
assert do_classifier_free_guidance
# 3. Encode input prompt
prompt_text_only = prompt.replace("<image>", "")
prompt_embeds = self._encode_prompt(
prompt_text_only,
device,
num_images_per_prompt,
do_classifier_free_guidance,
negative_prompt,
)
if augmented_prompt_embeds is None:
augmented_prompt_embeds = self._encode_augmented_prompt(prompt, reference_subject_images, device, prompt_embeds.dtype)
augmented_prompt_embeds = augmented_prompt_embeds.repeat(num_images_per_prompt, 1, 1)
prompt_embeds = torch.cat([prompt_embeds, augmented_prompt_embeds], dim=0)
# 4. Prepare timesteps
self.scheduler.set_timesteps(num_inference_steps, device=device)
timesteps = self.scheduler.timesteps
# 5. Prepare latent variables
# num_channels_latents = self.unet.in_channels
num_channels_latents = 4
latents = self.prepare_latents(
batch_size * num_images_per_prompt,
num_channels_latents,
height,
width,
prompt_embeds.dtype,
device,
generator,
latents,
)
start_subject_conditioning_step = (1 - alpha_) * num_inference_steps
extra_step_kwargs = self.prepare_extra_step_kwargs(generator, eta)
(
null_prompt_embeds,
text_prompt_embeds,
augmented_prompt_embeds
) = prompt_embeds.chunk(3)
# 7. Denoising loop
num_warmup_steps = len(timesteps) - num_inference_steps * self.scheduler.order
with self.progress_bar(total=num_inference_steps) as progress_bar:
for i, t in enumerate(timesteps):
latent_model_input = (
torch.cat([latents] * 2) if do_classifier_free_guidance else latents
)
latent_model_input = self.scheduler.scale_model_input(latent_model_input, t)
if i <= start_subject_conditioning_step:
current_prompt_embeds = torch.cat(
[null_prompt_embeds, text_prompt_embeds], dim=0
)
else:
current_prompt_embeds = torch.cat(
[null_prompt_embeds, augmented_prompt_embeds], dim=0
)
# predict the noise residual
noise_pred = self.unet([
latent_model_input,
t,
current_prompt_embeds,
# cross_attention_kwargs
],
)[0]
noise_pred = torch.from_numpy(noise_pred)
# perform guidance
if do_classifier_free_guidance:
noise_pred_uncond, noise_pred_text = noise_pred.chunk(2)
noise_pred = noise_pred_uncond + guidance_scale * (
noise_pred_text - noise_pred_uncond
)
else:
assert 0, "Not Implemented"
# compute the previous noisy sample x_t -> x_t-1
latents = self.scheduler.step(
noise_pred, t, latents, **extra_step_kwargs
).prev_sample
# call the callback, if provided
if i == len(timesteps) - 1 or (
(i + 1) > num_warmup_steps and (i + 1) % self.scheduler.order == 0
):
progress_bar.update()
if callback is not None and i % callback_steps == 0:
callback(i, t, latents)
if output_type == "latent":
image = latents
has_nsfw_concept = None
elif output_type == "pil":
# 8. Post-processing
image = self.decode_latents(latents)
# 9. Run safety checker
image, has_nsfw_concept = self.run_safety_checker(
image, device, prompt_embeds.dtype
)
# 10. Convert to PIL
image = self.numpy_to_pil(image)
else:
# 8. Post-processing
image = self.decode_latents(latents)
# 9. Run safety checker
image, has_nsfw_concept = self.run_safety_checker(
image, device, prompt_embeds.dtype
)
# Offload last model to CPU
if hasattr(self, "final_offload_hook") and self.final_offload_hook is not None:
self.final_offload_hook.offload()
if not return_dict:
return (image, has_nsfw_concept)
return StableDiffusionPipelineOutput(
images=image, nsfw_content_detected=has_nsfw_concept
)
そして、パイプライン内のすべてのモデルを変換されたモデルに置き換えます。
import PIL
from transformers import CLIPTokenizer
def create_pipeline(
args,
*,
text_encoder,
image_encoder,
unet,
object_transforms,
postfuse_module,
device
):
weight_dtype = torch.float32
tokenizer = CLIPTokenizer.from_pretrained(
args.pretrained_model_name_or_path,
subfolder="tokenizer",
revision=args.revision,
)
tokenizer.add_tokens(["img"], special_tokens=True)
image_token_id = tokenizer.convert_tokens_to_ids("img")
pipe = StableDiffusionFastCompposerPipeline.from_pretrained(
args.pretrained_model_name_or_path, torch_dtype=weight_dtype
).to(device)
pipe.object_transforms = object_transforms
pipe.unet = unet
pipe.text_encoder = text_encoder
pipe.postfuse_module = postfuse_module
pipe.image_encoder = image_encoder
pipe.image_token_id = image_token_id
pipe.special_tokenizer = tokenizer
return pipe
class ModelWrapper:
def __init__(self, model):
super().__init__()
self.model = model
def inference(
self,
image1: PIL.Image.Image,
image2: PIL.Image.Image,
prompt: str,
negative_prompt: str,
seed: int,
guidance_scale: float,
alpha_: float,
num_steps: int,
num_images: int,
):
print("Running model inference...")
image = []
if image1 is not None:
image.append(image1)
if image2 is not None:
image.append(image2)
if len(image) == 0:
return [], "You need to upload at least one image."
num_subject_in_text = (
np.array(self.model.special_tokenizer.encode(prompt))
== self.model.image_token_id
).sum()
if num_subject_in_text != len(image):
return (
[],
f"Number of subjects in the text description doesn't match the number of reference images, #text subjects: {num_subject_in_text} #reference image: {len(image)}",
)
if seed == -1:
seed = np.random.randint(0, 1000000)
generator = torch.manual_seed(seed)
return (
self.model(
prompt=prompt,
negative_prompt=negative_prompt,
height=512,
width=512,
num_inference_steps=num_steps,
guidance_scale=guidance_scale,
num_images_per_prompt=num_images,
generator=generator,
alpha_=alpha_,
reference_subject_images=image,
).images,
"run successfully",
)
core = openvino.Core()
compiled_unet = core.compile_model(unet_ir_xml_path)
compiled_text_encoder = core.compile_model(text_encoder_ir_xml_path)
compiled_image_encoder = core.compile_model(image_encoder_ir_xml_path)
compiled_postfuse_module = core.compile_model(postfuse_module_ir_xml_path)
compiled_object_transforms = core.compile_model(object_transforms_ir_xml_path)
wrapped_model = ModelWrapper(
create_pipeline(
config,
text_encoder=compiled_text_encoder,
image_encoder=compiled_image_encoder,
unet=compiled_unet,
object_transforms=compiled_object_transforms,
postfuse_module=compiled_postfuse_module,
device='cpu'
)
)
Loading pipeline components...: 0%| | 0/7 [00:00<?, ?it/s]
text_config_dict is provided which will be used to initialize CLIPTextConfig. The value text_config["id2label"] will be overriden. text_config_dict is provided which will be used to initialize CLIPTextConfig. The value text_config["bos_token_id"] will be overriden. text_config_dict is provided which will be used to initialize CLIPTextConfig. The value text_config["eos_token_id"] will be overriden.
推論¶
推論が可能になりました。1 枚または 2 枚の画像 (image1
と image2
) を提供できます。推論で画像を 1 つだけ渡したい場合は、画像ではなく None
を渡します。 prompt
ユーザー画像からどのようなオブジェクトが生成されるかコンテキストを説明します。単語 img
は入力画像と相関するトークンです。
image1 = Image.open('fastcomposer/data/newton_einstein/einstein/0.png')
image2 = Image.open('fastcomposer/data/newton_einstein/newton/0.png')
prompt = 'A man img and a man img sitting in a park'
negative_prompt = '((((ugly)))), (((duplicate))), ((morbid)), ((mutilated)), [out of frame], extra fingers, mutated hands, ((poorly drawn hands)), ((poorly drawn face)), (((mutation))), (((deformed))), ((ugly)), blurry, ((bad anatomy)), (((bad proportions))), ((extra limbs)), cloned face, (((disfigured))). out of frame, ugly, extra limbs, (bad anatomy), gross proportions, (malformed limbs), ((missing arms)), ((missing legs)), (((extra arms))), (((extra legs))), mutated hands, (fused fingers), (too many fingers), (((long neck)))'
alpha_ = 0.7
num_images = 1 # each extra image requires ~11GB of free memory
num_steps = 50
guidance_scale = 5
seed = -1
result = wrapped_model.inference(
image1,
image2,
prompt,
negative_prompt,
seed,
guidance_scale,
alpha_,
num_steps,
num_images
)
Running model inference...
0%| | 0/50 [00:00<?, ?it/s]
/home/ea/work/my_optimum_intel/optimum_env/lib/python3.8/site-packages/diffusers/pipelines/stable_diffusion/pipeline_stable_diffusion.py:533: FutureWarning: The decode_latents method is deprecated and will be removed in 1.0.0. Please use VaeImageProcessor.postprocess(...) instead
deprecate("decode_latents", "1.0.0", deprecation_message, standard_warn=False)
結果は複数の (num_images
) 画像で構成されており、それらを表示できるようになりました。
display(result[0][0])
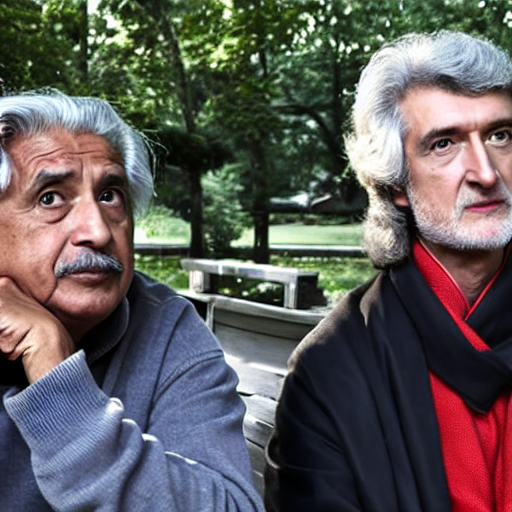
Gradio を実行¶
また、Gradio で実行することもできます。
import gradio as gr
def create_demo():
TITLE = "# [FastComposer Demo](https://github.com/mit-han-lab/fastcomposer) with OpenVINO"
DESCRIPTION = """To run the demo, you should:
1. Upload your images. The order of image1 and image2 needs to match the order of the subects in the prompt. You only need 1 image for single subject generation.
2. Input proper text prompts, such as "A woman img and a man img in the snow" or "A painting of a man img in the style of Van Gogh", where "img" specifies the token you want to augment and comes after the word.
3. Click the Run button. You can also adjust the hyperparameters to improve the results. Look at the job status to see if there are any errors with your input.
As a result, pictures with person or persons from input images will be generated in accordance with the description in the prompt.
"""
with gr.Blocks() as demo:
gr.Markdown(TITLE)
gr.Markdown(DESCRIPTION)
with gr.Row():
with gr.Column():
with gr.Group():
image1 = gr.Image(label="Image 1", type="pil")
gr.Examples(
examples=["fastcomposer/data/newton.jpeg"],
inputs=image1,
)
image2 = gr.Image(label="Image 2", type="pil")
gr.Examples(
examples=["fastcomposer/data/einstein.jpeg"],
inputs=image2,
)
gr.Markdown("Upload the image for your subject")
prompt = gr.Text(
value="A man img and a man img sitting in a park",
label="Prompt",
placeholder='e.g. "A woman img and a man img in the snow", "A painting of a man img in the style of Van Gogh"',
info='Use "img" to specify the word you want to augment.',
)
negative_prompt = gr.Text(
value="((((ugly)))), (((duplicate))), ((morbid)), ((mutilated)), [out of frame], extra fingers, mutated hands, ((poorly drawn hands)), ((poorly drawn face)), (((mutation))), (((deformed))), ((ugly)), blurry, ((bad anatomy)), (((bad proportions))), ((extra limbs)), cloned face, (((disfigured))). out of frame, ugly, extra limbs, (bad anatomy), gross proportions, (malformed limbs), ((missing arms)), ((missing legs)), (((extra arms))), (((extra legs))), mutated hands, (fused fingers), (too many fingers), (((long neck)))",
label="Negative Prompt",
info='Features that you want to avoid.',
)
alpha_ = gr.Slider(
label="alpha",
minimum=0,
maximum=1,
step=0.05,
value=0.75,
info="A smaller alpha aligns images with text better, but may deviate from the subject image. Increase alpha to improve identity preservation, decrease it for prompt consistency.",
)
num_images = gr.Slider(
label="Number of generated images",
minimum=1,
maximum=8,
step=1,
value=1,
info="Each extra image requires ~11GB of free memory.",
)
run_button = gr.Button("Run")
with gr.Accordion(label="Advanced options", open=False):
seed = gr.Slider(
label="Seed",
minimum=-1,
maximum=1000000,
step=1,
value=-1,
info="If set to -1, a different seed will be used each time.",
)
guidance_scale = gr.Slider(
label="Guidance scale",
minimum=1,
maximum=10,
step=1,
value=5,
)
num_steps = gr.Slider(
label="Steps",
minimum=1,
maximum=300,
step=1,
value=50,
)
with gr.Column():
result = gr.Gallery(label="Generated Images", columns=[2])
error_message = gr.Text(label="Job Status")
inputs = [
image1,
image2,
prompt,
negative_prompt,
seed,
guidance_scale,
alpha_,
num_steps,
num_images,
]
run_button.click(
fn=wrapped_model.inference, inputs=inputs, outputs=[result, error_message]
)
return demo
demo = create_demo()
if __name__ == "__main__":
try:
demo.launch(debug=False)
except Exception:
demo.launch(share=True, debug=False)
# if you are launching remotely, specify server_name and server_port
# demo.launch(server_name='your server name', server_port='server port in int')
# Read more in the docs: https://gradio.app/docs/