FILM と OpenVINO を使用したフレーム補間¶
この Jupyter ノートブックは、ローカルへのインストール後にのみ起動できます。
フレーム補間は、指定された一連の画像から中間画像を合成するプロセスです。この技術は、ビデオのリフレッシュ・レートを高めたり、スローモーション効果を作成するため時間的アップサンプリングによく使用されます。最近では、デジタルカメラやスマートフォンを使用して、高品質の写真を撮影するため数秒以内に何枚も写真を撮ることがあります。これらの “ほぼ複製” 写真間を補間すると、シーンの動きを明らかにする魅力的なビデオが作成され、多くの場合、元の写真よりもさらによい瞬間の感覚が得られます。
ECCV 2022 で公開された “FILM: Frame Interpolation for Large Motion” では、類似した写真から高品質のスローモーション・ビデオを作成する方法が紹介されています。FILM は、小さな動きを適切に処理しながら、大きな動きで最先端の結果を達成する新しいニューラル・ネットワーク・アーキテクチャーです。
FILM モデルは 2 つのイメージを入力として受け取り、中央のイメージを出力します。推論時にモデルが再帰的に呼び出され、画像間の画像が出力されます。FILM には 3 つのコンポーネントがあります。
- 各入力画像を深いマルチスケール (ピラミッド) 特徴で要約する特徴抽出機能。
- 各ピラミッドレベルでピクセル単位の動き (つまり、フロー) を計算する双方向動き推定器。
- 最終的な補間画像を出力する Fusion モジュール。
FILM は通常のビデオフレーム 3 つでトレーニングされ、中央のフレームが監視のためのグラウンドトゥルースとして機能します。
このチュートリアルでは、TensorFlow Hub をモデルソースとして使用します。
注: このチュートリアルを実行するには、システムに VP9 ビデオ・エンコーダーが必要です。Ubuntu* にはプリインストールされていますが、Windows* では手動でインストールする必要があります。
目次¶
必要条件¶
%pip install -q tensorflow tensorflow_hub numpy "opencv-python" tqdm matplotlib gradio Pillow "openvino>=2023.2.0"
[notice] A new release of pip is available: 23.2.1 -> 23.3.1
[notice] To update, run: pip install --upgrade pip
Note: you may need to restart the kernel to use updated packages.
WARNING: Skipping openvino as it is not installed.
Note: you may need to restart the kernel to use updated packages.
[notice] A new release of pip is available: 23.2.1 -> 23.3.1
[notice] To update, run: pip install --upgrade pip
Note: you may need to restart the kernel to use updated packages.
from pathlib import Path
from urllib.request import urlretrieve
from typing import Optional, Generator
from datetime import datetime
import gc
import tensorflow_hub as hub
import tensorflow as tf
import openvino as ov
import ipywidgets
import numpy as np
import cv2
import matplotlib.pyplot as plt
from tqdm.auto import tqdm
import gradio as gr
import PIL
import IPython
2023-11-02 11:23:42.519606: I tensorflow/core/util/port.cc:111] oneDNN custom operations are on. You may see slightly different numerical results due to floating-point round-off errors from different computation orders. To turn them off, set the environment variable TF_ENABLE_ONEDNN_OPTS=0. 2023-11-02 11:23:42.521340: I tensorflow/tsl/cuda/cudart_stub.cc:28] Could not find cuda drivers on your machine, GPU will not be used. 2023-11-02 11:23:42.549839: E tensorflow/compiler/xla/stream_executor/cuda/cuda_dnn.cc:9342] Unable to register cuDNN factory: Attempting to register factory for plugin cuDNN when one has already been registered 2023-11-02 11:23:42.549860: E tensorflow/compiler/xla/stream_executor/cuda/cuda_fft.cc:609] Unable to register cuFFT factory: Attempting to register factory for plugin cuFFT when one has already been registered 2023-11-02 11:23:42.549882: E tensorflow/compiler/xla/stream_executor/cuda/cuda_blas.cc:1518] Unable to register cuBLAS factory: Attempting to register factory for plugin cuBLAS when one has already been registered 2023-11-02 11:23:42.555392: I tensorflow/tsl/cuda/cudart_stub.cc:28] Could not find cuda drivers on your machine, GPU will not be used. 2023-11-02 11:23:42.556206: I tensorflow/core/platform/cpu_feature_guard.cc:182] This TensorFlow binary is optimized to use available CPU instructions in performance-critical operations. To enable the following instructions: AVX2 AVX512F AVX512_VNNI FMA, in other operations, rebuild TensorFlow with the appropriate compiler flags. 2023-11-02 11:23:43.247021: W tensorflow/compiler/tf2tensorrt/utils/py_utils.cc:38] TF-TRT Warning: Could not find TensorRT
MODEL_PATH = Path("models/model.xml")
DATA_PATH = Path("data")
IMAGES = {
"https://raw.githubusercontent.com/google-research/frame-interpolation/main/photos/one.png": Path("data/one.png"),
"https://raw.githubusercontent.com/google-research/frame-interpolation/main/photos/two.png": Path("data/two.png")
}
OUTPUT_VIDEO_PATH = DATA_PATH / "output.webm"
OV_OUTPUT_VIDEO_PATH = DATA_PATH / "ov_output.webm"
TIMES_TO_INTERPOLATE = 5
DATA_PATH.mkdir(parents=True, exist_ok=True)
PIL.ImageFile.LOAD_TRUNCATED_IMAGES = True # allows Gradio to read PNG images with large metadata
画像を準備¶
画像をダウンロードして NumPy 配列にキャストし、モデル入力として提供します。
def preprocess_np_frame(frame):
result = frame.astype(np.float32) / 255 # normalize to [0, 1]
result = result[np.newaxis, ...] # add batch dim
return result
def prepare_input(img_url: str):
if not IMAGES[img_url].exists():
urlretrieve(img_url, IMAGES[img_url])
filename = str(IMAGES[img_url])
img = cv2.imread(filename)
img = cv2.cvtColor(img, cv2.COLOR_BGR2RGB)
img = np.array(img)
img = preprocess_np_frame(img)
return img
input_images = [prepare_input(url) for url in IMAGES]
input = {
"x0": input_images[0],
"x1": input_images[1],
"time": np.array([[0.5]], dtype=np.float32)
}
plt.figure(figsize=(16, 8), layout="tight")
plt.subplot(1, 2, 1)
plt.imshow(input_images[0][0])
plt.axis("off")
plt.subplot(1, 2, 2)
plt.imshow(input_images[1][0])
plt.axis("off");
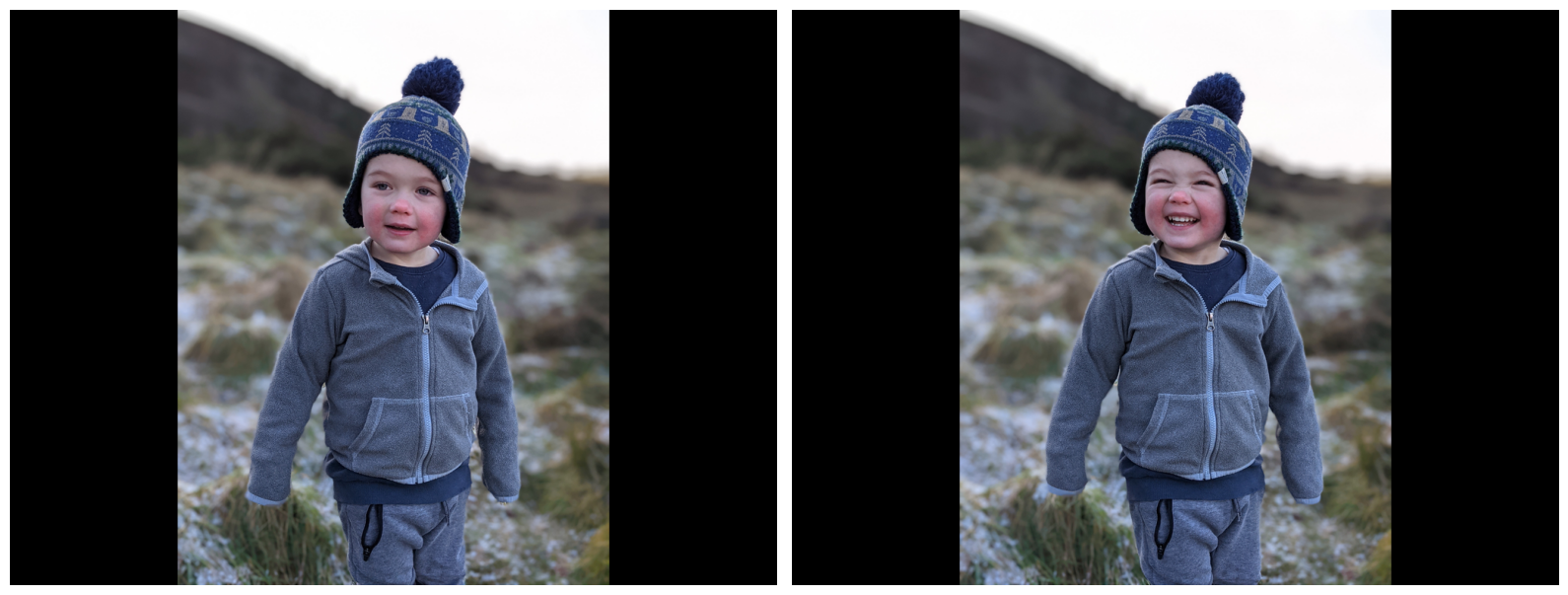
モデルのロード¶
モデルは tensorflow_hub.KerasLayer
関数を使用してロードされます。次に、入力テンソルの形状を指定して、ロードされたオブジェクトを tf.keras.Model
クラスにキャストします。
入力テンソルは次のとおりです。
time
- 生成された画像がどこにあるべきかを示す \([0,1]\) の間の値。\(0.5\) は入力画像の中間にあります。x0
- 初期フレーム。x1
- 最終フレーム。
詳細については、TensorFlow Hub のモデルページを参照してください。
inputs = dict(
x0=tf.keras.layers.Input(shape=(None, None, 3)),
x1=tf.keras.layers.Input(shape=(None, None, 3)),
time=tf.keras.layers.Input(shape=(1)),
)
model_url = "https://www.kaggle.com/models/google/film/frameworks/tensorFlow2/variations/film/versions/1"
film_layer = hub.KerasLayer(model_url)(inputs)
film_model = tf.keras.Model(inputs=inputs, outputs=film_layer)
モデルを推測¶
単一中間フレーム補間¶
output = film_model(input)
interpolated_image = output["image"][0]
interpolated_image = np.clip(interpolated_image, 0, 1)
def draw(img1, mid_img, img2):
title2img = {"First frame": img1, "Interpolated frame": mid_img, "Last frame": img2}
plt.figure(figsize=(16,8), layout="tight")
for i, (title, img) in enumerate(title2img.items()):
ax = plt.subplot(1, 3, i + 1)
ax.set_title(title)
plt.imshow(img)
plt.axis("off")
draw(input_images[0][0], interpolated_image, input_images[1][0])
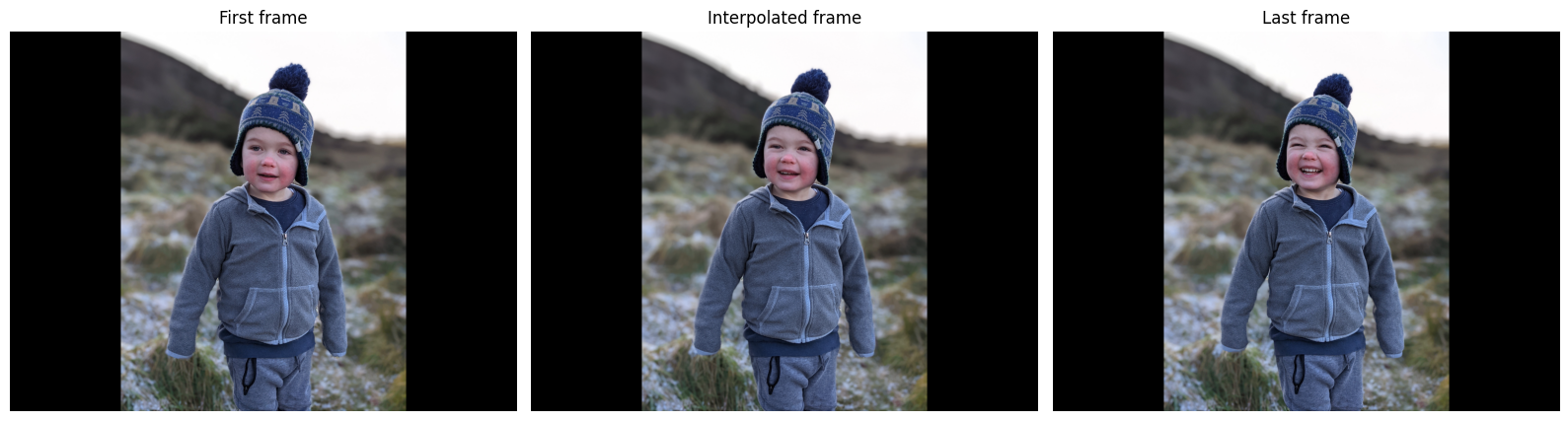
再帰的なフレーム生成¶
このプロセスでは、2 つの元のフレーム (最初と最後) を入力として受け取り、中間フレームを生成します。次に、 “最初 - 中間点”、“中間点 - 最後” のペアに対してこの処理が繰り返され、それらに中間点が提供されます。再帰は \(t=\) times_to_interpolate
回実行され、 \(2^t-1\) 画像が生成されます。
class Interpolator:
def __init__(self, model):
self._model = model
def _recursive_generator(
self,
frame1: np.ndarray,
frame2: np.ndarray,
num_recursions: int,
bar: Optional[tqdm] = None,
) -> Generator[np.ndarray, None, None]:
"""Splits halfway to repeatedly generate more frames.
Args:
frame1: Input image 1.
frame2: Input image 2.
num_recursions: How many times to interpolate the consecutive image pairs.
Yields:
The interpolated frames, including the first frame (frame1), but excluding
the final frame2.
"""
if num_recursions == 0:
yield frame1
else:
time = np.array([[0.5]], dtype=np.float32)
mid_frame = self._model({"x0": frame1, "x1": frame2, "time": time})["image"]
if bar is not None:
bar.update(1)
yield from self._recursive_generator(frame1, mid_frame, num_recursions - 1, bar)
yield from self._recursive_generator(mid_frame, frame2, num_recursions - 1, bar)
def interpolate_recursively(
self, frame1: np.ndarray, frame2: np.ndarray, times_to_interpolate: int
) -> Generator[np.ndarray, None, None]:
"""Generates interpolated frames by repeatedly interpolating the midpoint.
Args:
frame1: Input image 1.
frame2: Input image 2.
times_to_interpolate: Number of times to do recursive midpoint
interpolation.
Yields:
The interpolated frames (including the inputs).
"""
num_frames = 2 ** (times_to_interpolate) - 1
bar = tqdm(total=num_frames)
yield from self._recursive_generator(frame1, frame2, times_to_interpolate, bar)
# Separately yield the final frame.
yield frame2
def save_as_video(frames: Generator[np.ndarray, None, None], width: int, height: int, filename: Path):
out = cv2.VideoWriter(str(filename), cv2.VideoWriter_fourcc(*'VP90'), 30, (width, height))
for frame in frames:
img = frame[0]
img = np.clip(img, 0, 1)
rgb_img = img * 255
rgb_img = rgb_img.astype(np.uint8)
bgr_img = cv2.cvtColor(rgb_img, cv2.COLOR_RGB2BGR)
out.write(bgr_img)
out.release()
height, width = input_images[0][0].shape[:2]
interpolator = Interpolator(film_model)
frames = interpolator.interpolate_recursively(input_images[0], input_images[1], TIMES_TO_INTERPOLATE)
save_as_video(frames, width, height, OUTPUT_VIDEO_PATH)
OpenCV: FFMPEG: tag 0x30395056/'VP90' is not supported with codec id 167 and format 'webm / WebM'
0%| | 0/31 [00:00<?, ?it/s]
IPython.display.Video(filename=OUTPUT_VIDEO_PATH, embed=True)
モデルを OpenVINO IR に変換¶
TensorFlow Keras モデルを OpenVINO 中間表現 (IR) に変換するには、openvino.convert_model()
関数を呼び出し、モデルを引数として渡します。その後、openvino.save_model()
関数を使用して、モデル・オブジェクトをディスクにシリアル化できます。
if not MODEL_PATH.exists():
converted_model = ov.convert_model(film_model)
ov.save_model(converted_model, MODEL_PATH)
del converted_model
del film_model
gc.collect()
100834
推論¶
推論デバイスの選択¶
OpenVINO を使用して推論を実行するためにドロップダウン・リストからデバイスを選択します。
core = ov.Core()
device = ipywidgets.Dropdown(
options=core.available_devices + ["AUTO"],
value='AUTO',
description='Device:',
disabled=False,
)
device
Dropdown(description='Device:', index=4, options=('CPU', 'GPU.0', 'GPU.1', 'GPU.2', 'AUTO'), value='AUTO')
compiled_model = core.compile_model(MODEL_PATH, device.value)
単一中間フレーム補間¶
モデル出力には補助推論データを含む複数のテンソルが含まれます。メインの出力テンソル (補間されたイメージ) は、 “image” キーに保存されます。
result = compiled_model(input)["image"]
image = result[0]
image = np.clip(image, 0, 1)
モデルは中間画像を返しました。確認します。
draw(input_images[0][0], image, input_images[1][0])
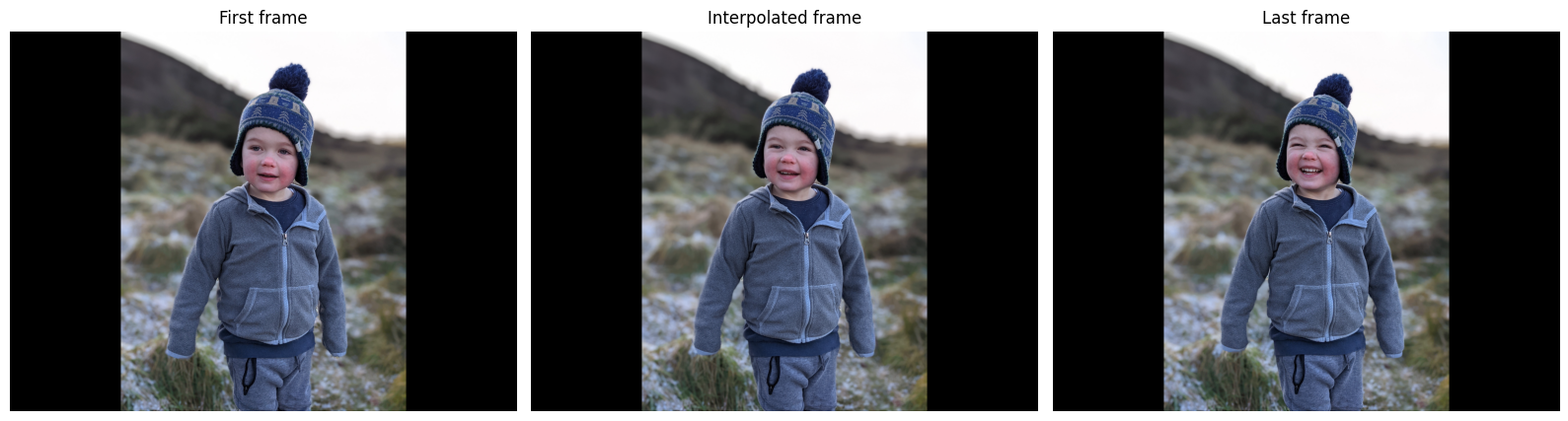
再帰的なフレーム生成¶
次に、最初の画像、中間画像、最後の画像の間でフレームを再帰的に生成して、滑らかなビデオを作成します。
height, width = input_images[0][0].shape[:2]
ov_interpolator = Interpolator(compiled_model)
frames = ov_interpolator.interpolate_recursively(input_images[0], input_images[1], TIMES_TO_INTERPOLATE)
save_as_video(frames, width, height, OV_OUTPUT_VIDEO_PATH)
OpenCV: FFMPEG: tag 0x30395056/'VP90' is not supported with codec id 167 and format 'webm / WebM'
0%| | 0/31 [00:00<?, ?it/s]
IPython.display.Video(filename=OV_OUTPUT_VIDEO_PATH, embed=True)
インタラクティブな推論¶
def generate(frame1, frame2, times_to_interpolate, _=gr.Progress(track_tqdm=True)):
x0, x1 = [preprocess_np_frame(frame) for frame in [frame1, frame2]]
frames = ov_interpolator.interpolate_recursively(x0, x1, times_to_interpolate)
height, width = frame1.shape[:2]
filename = DATA_PATH / f"output_{datetime.now().isoformat()}.webm"
save_as_video(frames, width, height, filename)
return filename
demo = gr.Interface(
generate,
[
gr.Image(label="First image"),
gr.Image(label="Last image"),
gr.Slider(1, 8, step=1, label="Times to interpolate", info="""Controls the number of times the frame interpolator is invoked.
The output will be the interpolation video with (2^value + 1) frames, fps of 30.""")
],
gr.Video(),
examples=[[*IMAGES.values(), 5]],
allow_flagging="never"
)
try:
demo.queue().launch(debug=False)
except Exception:
demo.queue().launch(share=True, debug=False)
# if you are launching remotely, specify server_name and server_port
# demo.launch(server_name='your server name', server_port='server port in int')
# Read more in the docs: https://gradio.app/docs/