TensorFlow モデルから OpenVINO™ への変換¶
この Jupyter ノートブックはオンラインで起動でき、ブラウザーのウィンドウで対話型環境を開きます。ローカルにインストールすることもできます。次のオプションのいずれかを選択します。
このチュートリアルでは、モデル変換 API を使用して、TensorFlow MobileNetV3 画像分類モデルを OpenVINO 中間表現 (OpenVINO IR) 形式に変換する方法を示します。OpenVINO IR を作成した後、OpenVINO ランタイムにモデルをロードし、サンプルイメージを使用して推論を実行します。
目次¶
# Install openvino package
%pip install -q "openvino>=2023.1.0"
Note: you may need to restart the kernel to use updated packages.
インポート¶
import time
from pathlib import Path
import cv2
import matplotlib.pyplot as plt
import numpy as np
import openvino as ov
import tensorflow as tf
# Fetch `notebook_utils` module
import urllib.request
urllib.request.urlretrieve(
url='https://raw.githubusercontent.com/openvinotoolkit/openvino_notebooks/main/notebooks/utils/notebook_utils.py',
filename='notebook_utils.py'
)
from notebook_utils import download_file
2024-02-09 22:34:07.759850: I tensorflow/core/util/port.cc:110] oneDNN custom operations are on. You may see slightly different numerical results due to floating-point round-off errors from different computation orders. To turn them off, set the environment variable TF_ENABLE_ONEDNN_OPTS=0. 2024-02-09 22:34:07.794264: I tensorflow/core/platform/cpu_feature_guard.cc:182] This TensorFlow binary is optimized to use available CPU instructions in performance-critical operations. To enable the following instructions: AVX2 AVX512F AVX512_VNNI FMA, in other operations, rebuild TensorFlow with the appropriate compiler flags.
2024-02-09 22:34:08.310440: W tensorflow/compiler/tf2tensorrt/utils/py_utils.cc:38] TF-TRT Warning: Could not find TensorRT
設定¶
# The paths of the source and converted models.
model_dir = Path("model")
model_dir.mkdir(exist_ok=True)
model_path = Path("model/v3-small_224_1.0_float")
ir_path = Path("model/v3-small_224_1.0_float.xml")
モデルのダウンロード¶
tf.keras.applications api を使用してモデルをロードし、ディスクに保存します。
model = tf.keras.applications.MobileNetV3Small()
model.save(model_path)
WARNING:tensorflow:input_shape is undefined or non-square, or rows is not 224. Weights for input shape (224, 224) will be loaded as the default.
2024-02-09 22:34:11.190073: E tensorflow/compiler/xla/stream_executor/cuda/cuda_driver.cc:266] failed call to cuInit: CUDA_ERROR_COMPAT_NOT_SUPPORTED_ON_DEVICE: forward compatibility was attempted on non supported HW
2024-02-09 22:34:11.190106: I tensorflow/compiler/xla/stream_executor/cuda/cuda_diagnostics.cc:168] retrieving CUDA diagnostic information for host: iotg-dev-workstation-07
2024-02-09 22:34:11.190111: I tensorflow/compiler/xla/stream_executor/cuda/cuda_diagnostics.cc:175] hostname: iotg-dev-workstation-07
2024-02-09 22:34:11.190249: I tensorflow/compiler/xla/stream_executor/cuda/cuda_diagnostics.cc:199] libcuda reported version is: 470.223.2
2024-02-09 22:34:11.190264: I tensorflow/compiler/xla/stream_executor/cuda/cuda_diagnostics.cc:203] kernel reported version is: 470.182.3
2024-02-09 22:34:11.190268: E tensorflow/compiler/xla/stream_executor/cuda/cuda_diagnostics.cc:312] kernel version 470.182.3 does not match DSO version 470.223.2 -- cannot find working devices in this configuration
WARNING:tensorflow:Compiled the loaded model, but the compiled metrics have yet to be built. model.compile_metrics will be empty until you train or evaluate the model.
2024-02-09 22:34:15.411337: I tensorflow/core/common_runtime/executor.cc:1197] [/device:CPU:0] (DEBUG INFO) Executor start aborting (this does not indicate an error and you can ignore this message): INVALID_ARGUMENT: You must feed a value for placeholder tensor 'inputs' with dtype float and shape [?,1,1,1024]
[[{{node inputs}}]]
2024-02-09 22:34:18.568762: I tensorflow/core/common_runtime/executor.cc:1197] [/device:CPU:0] (DEBUG INFO) Executor start aborting (this does not indicate an error and you can ignore this message): INVALID_ARGUMENT: You must feed a value for placeholder tensor 'inputs' with dtype float and shape [?,1,1,1024]
[[{{node inputs}}]]
WARNING:absl:Found untraced functions such as _jit_compiled_convolution_op, _jit_compiled_convolution_op, _jit_compiled_convolution_op, _jit_compiled_convolution_op, _jit_compiled_convolution_op while saving (showing 5 of 54). These functions will not be directly callable after loading.
INFO:tensorflow:Assets written to: model/v3-small_224_1.0_float/assets
INFO:tensorflow:Assets written to: model/v3-small_224_1.0_float/assets
モデルを OpenVINO IR 形式に変換¶
TensorFlow モデルを OpenVINO IR 形式に変換¶
モデル変換 Python API を使用して、TensorFlow モデルを OpenVINO IR に変換します。ov.convert_model
関数は、保存されたモデルのディレクトリーへのパスを受け入れ、このモデルを表す OpenVINO Model クラスのインスタンスを返します。取得したモデルはすぐに使用でき、ov.compile_model
を使用してデバイスにロードするか、ov.save_model
関数でディスクに保存できます。TensorFlow モデルでのモデル変換 API の使用の詳細は、チュートリアルを参照してください。
# Run model conversion API if the IR model file does not exist
if not ir_path.exists():
print("Exporting TensorFlow model to IR... This may take a few minutes.")
ov_model = ov.convert_model(model_path, input=[[1, 224, 224, 3]])
ov.save_model(ov_model, ir_path)
else:
print(f"IR model {ir_path} already exists.")
Exporting TensorFlow model to IR... This may take a few minutes.
推論デバイスの選択¶
OpenVINO を使用して推論を実行するためにドロップダウン・リストからデバイスを選択します。
import ipywidgets as widgets
device = widgets.Dropdown(
options=core.available_devices + ["AUTO"],
value='AUTO',
description='Device:',
disabled=False,
)
device
Dropdown(description='Device:', index=1, options=('CPU', 'AUTO'), value='AUTO')
compiled_model = core.compile_model(model=model, device_name=device.value)
モデルの情報を取得¶
input_key = compiled_model.input(0)
output_key = compiled_model.output(0)
network_input_shape = input_key.shape
画像のロード¶
画像をロードし、サイズを変更し、ネットワークの入力形状に変換します。
# Download the image from the openvino_notebooks storage
image_filename = download_file(
"https://storage.openvinotoolkit.org/repositories/openvino_notebooks/data/data/image/coco.jpg",
directory="data"
)
# The MobileNet network expects images in RGB format.
image = cv2.cvtColor(cv2.imread(filename=str(image_filename)), code=cv2.COLOR_BGR2RGB)
# Resize the image to the network input shape.
resized_image = cv2.resize(src=image, dsize=(224, 224))
# Transpose the image to the network input shape.
input_image = np.expand_dims(resized_image, 0)
plt.imshow(image);
data/coco.jpg: 0%| | 0.00/202k [00:00<?, ?B/s]
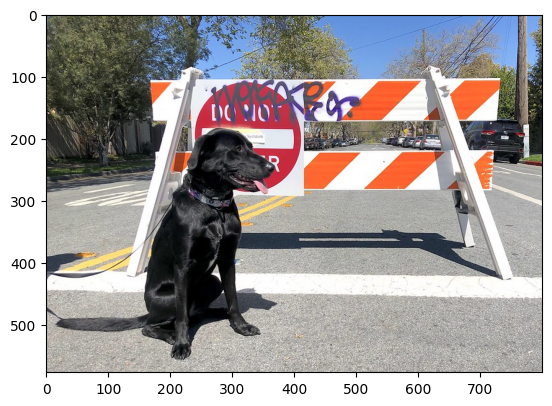
推論の実行¶
result = compiled_model(input_image)[output_key]
result_index = np.argmax(result)
# Download the datasets from the openvino_notebooks storage
image_filename = download_file(
"https://storage.openvinotoolkit.org/repositories/openvino_notebooks/data/data/datasets/imagenet/imagenet_2012.txt",
directory="data"
)
# Convert the inference result to a class name.
imagenet_classes = image_filename.read_text().splitlines()
imagenet_classes[result_index]
data/imagenet_2012.txt: 0%| | 0.00/30.9k [00:00<?, ?B/s]
'n02099267 flat-coated retriever'
タイミング¶
1,000 枚の画像の推論にかかる時間を測定します。これはパフォーマンスの指標となります。より正確なベンチマークを行うには、OpenVINO のベンチマーク・ツールを使用します。パフォーマンスを向上するには多くの最適化が可能であることに注意してください。
num_images = 1000
start = time.perf_counter()
for _ in range(num_images):
compiled_model([input_image])
end = time.perf_counter()
time_ir = end - start
print(
f"IR model in OpenVINO Runtime/CPU: {time_ir/num_images:.4f} "
f"seconds per image, FPS: {num_images/time_ir:.2f}"
)
IR model in OpenVINO Runtime/CPU: 0.0011 seconds per image, FPS: 926.34