OpenVINO™ を使用したリアルタイムの推論と CT スキャンデータのベンチマーク¶
この Jupyter ノートブックはオンラインで起動でき、ブラウザーのウィンドウで対話型環境を開きます。ローカルにインストールすることもできます。次のオプションのいずれかを選択します。
PyTorch Lightning と OpenVINO™ による腎臓のセグメント化 - パート 4¶
このチュートリアルは、医療セグメント化モデルのトレーニング、最適化、量子化、リアルタイムの推論の表示方法に関するシリーズの一部です。目標は、腎臓のセグメント化モデルの推論を加速することです。UNet モデルは最初からトレーニングされており、データは Kits19 から取得されています。
このチュートリアルでは、OpenVINO で非同期 API と MULTI プラグインを使用してモデルのパフォーマンスをベンチマークし、リアルタイムの推論を表示する方法を説明します。
このノートブックには、量子化された OpenVINO IR モデルと、2D 画像に変換された KiTS-19 データセットの画像が必要です。(モデルがどのように量子化されるかについては、UNet モデルの変換と量子化、およびリアルタイムの推論の表示チュートリアルを参照してください。)
このノートブックは、完全な KiTS-19 フレーム・データセットを使用して 20 エポックだけトレーニングされた事前トレーニング済みモデルを提供します。このモデルの検証セットの F1 スコアは 0.9 です。トレーニング・コードは、PyTorch MONAI トレーニングのノートブックで入手できます。
デモンストレーションの目的で、このチュートリアルでは推論に使用する変換された CT スキャンを 1 つダウンロードします。
目次¶
%pip install -q "openvino>=2023.1.0" "monai>=0.9.1,<1.0.0" "nncf>=2.5.0"
Note: you may need to restart the kernel to use updated packages.
インポート¶
import os
import sys
import zipfile
from pathlib import Path
import numpy as np
from monai.transforms import LoadImage
import openvino as ov
from custom_segmentation import SegmentationModel
sys.path.append("../utils")
from notebook_utils import download_file
2024-02-09 22:50:38.323593: I tensorflow/core/util/port.cc:110] oneDNN custom operations are on. You may see slightly different numerical results due to floating-point round-off errors from different computation orders. To turn them off, set the environment variable TF_ENABLE_ONEDNN_OPTS=0. 2024-02-09 22:50:38.357752: I tensorflow/core/platform/cpu_feature_guard.cc:182] This TensorFlow binary is optimized to use available CPU instructions in performance-critical operations. To enable the following instructions: AVX2 AVX512F AVX512_VNNI FMA, in other operations, rebuild TensorFlow with the appropriate compiler flags.
2024-02-09 22:50:38.922511: W tensorflow/compiler/tf2tensorrt/utils/py_utils.cc:38] TF-TRT Warning: Could not find TensorRT
設定¶
事前トレーニング済みモデルを使用するには、IR_PATH
を "pretrained_model/unet44.xml"
に設定し、COMPRESSED_MODEL_PATH
を "pretrained_model/quantized_unet44.xml"
に設定します。自身でトレーニングまたは最適化したモデルを使用するには、モデルパスを調整します。
# The directory that contains the IR model (xml and bin) files.
models_dir = Path('pretrained_model')
ir_model_url = 'https://storage.openvinotoolkit.org/repositories/openvino_notebooks/models/kidney-segmentation-kits19/FP16-INT8/'
ir_model_name_xml = 'quantized_unet_kits19.xml'
ir_model_name_bin = 'quantized_unet_kits19.bin'
download_file(ir_model_url + ir_model_name_xml, filename=ir_model_name_xml, directory=models_dir)
download_file(ir_model_url + ir_model_name_bin, filename=ir_model_name_bin, directory=models_dir)
MODEL_PATH = models_dir / ir_model_name_xml
# Uncomment the next line to use the FP16 model instead of the quantized model.
# MODEL_PATH = "pretrained_model/unet_kits19.xml"
pretrained_model/quantized_unet_kits19.xml: 0%| | 0.00/280k [00:00<?, ?B/s]
pretrained_model/quantized_unet_kits19.bin: 0%| | 0.00/1.90M [00:00<?, ?B/s]
モデル・パフォーマンスのベンチマーク¶
IR モデルの推論パフォーマンスを測定するには、OpenVINO の推論パフォーマンス測定ツールであるベンチマーク・ツールを使用します。ベンチマーク・ツールは、! benchmark_app
または %sx benchmark_app
コマンドを使用してノートブックで実行できるコマンドライン・アプリケーションです。
注:
benchmark_app
ツールは、OpenVINO 中間表現 (OpenVINO IR) モデルのパフォーマンスのみを測定できます。より正確なパフォーマンスを得るには、他のアプリケーションを閉じて、ターミナル/コマンドプロンプトでbenchmark_app
を実行します。benchmark_app -m model.xml -d CPU
を実行して、CPU で非同期推論のベンチマークを 1 分間実行します。GPU でベンチマークを行うには、CPU
をGPU
に変更します。benchmark_app --help
を実行すると、すべてのコマンドライン・オプションの概要が表示されます。
core = ov.Core()
# By default, benchmark on MULTI:CPU,GPU if a GPU is available, otherwise on CPU.
device_list = ["MULTI:CPU,GPU" if "GPU" in core.available_devices else "AUTO"]
import ipywidgets as widgets
device = widgets.Dropdown(
options=core.available_devices + device_list,
value=device_list[0],
description='Device:',
disabled=False,
)
device
Dropdown(description='Device:', index=1, options=('CPU', 'AUTO'), value='AUTO')
# Benchmark model
! benchmark_app -m $MODEL_PATH -d $device.value -t 15 -api sync
[Step 1/11] Parsing and validating input arguments
[ INFO ] Parsing input parameters
[Step 2/11] Loading OpenVINO Runtime
[ INFO ] OpenVINO:
[ INFO ] Build ................................. 2023.3.0-13775-ceeafaf64f3-releases/2023/3
[ INFO ]
[ INFO ] Device info:
[ INFO ] AUTO
[ INFO ] Build ................................. 2023.3.0-13775-ceeafaf64f3-releases/2023/3
[ INFO ]
[ INFO ]
[Step 3/11] Setting device configuration
[ WARNING ] Performance hint was not explicitly specified in command line. Device(AUTO) performance hint will be set to PerformanceMode.LATENCY.
[Step 4/11] Reading model files
[ INFO ] Loading model files
[ INFO ] Read model took 13.02 ms
[ INFO ] Original model I/O parameters:
[ INFO ] Model inputs:
[ INFO ] input.1 (node: input.1) : f32 / [...] / [1,1,512,512]
[ INFO ] Model outputs:
[ INFO ] 153 (node: 153) : f32 / [...] / [1,1,512,512]
[Step 5/11] Resizing model to match image sizes and given batch
[ INFO ] Model batch size: 1
[Step 6/11] Configuring input of the model
[ INFO ] Model inputs:
[ INFO ] input.1 (node: input.1) : f32 / [N,C,H,W] / [1,1,512,512]
[ INFO ] Model outputs:
[ INFO ] 153 (node: 153) : f32 / [...] / [1,1,512,512]
[Step 7/11] Loading the model to the device
[ INFO ] Compile model took 232.64 ms
[Step 8/11] Querying optimal runtime parameters
[ INFO ] Model:
[ INFO ] NETWORK_NAME: pretrained_unet_kits19
[ INFO ] EXECUTION_DEVICES: ['CPU']
[ INFO ] PERFORMANCE_HINT: PerformanceMode.LATENCY
[ INFO ] OPTIMAL_NUMBER_OF_INFER_REQUESTS: 1
[ INFO ] MULTI_DEVICE_PRIORITIES: CPU
[ INFO ] CPU:
[ INFO ] AFFINITY: Affinity.CORE
[ INFO ] CPU_DENORMALS_OPTIMIZATION: False
[ INFO ] CPU_SPARSE_WEIGHTS_DECOMPRESSION_RATE: 1.0
[ INFO ] ENABLE_CPU_PINNING: True
[ INFO ] ENABLE_HYPER_THREADING: False
[ INFO ] EXECUTION_DEVICES: ['CPU']
[ INFO ] EXECUTION_MODE_HINT: ExecutionMode.PERFORMANCE
[ INFO ] INFERENCE_NUM_THREADS: 12
[ INFO ] INFERENCE_PRECISION_HINT: <Type: 'float32'>
[ INFO ] NETWORK_NAME: pretrained_unet_kits19
[ INFO ] NUM_STREAMS: 1
[ INFO ] OPTIMAL_NUMBER_OF_INFER_REQUESTS: 1
[ INFO ] PERFORMANCE_HINT: LATENCY
[ INFO ] PERFORMANCE_HINT_NUM_REQUESTS: 0
[ INFO ] PERF_COUNT: NO
[ INFO ] SCHEDULING_CORE_TYPE: SchedulingCoreType.ANY_CORE
[ INFO ] MODEL_PRIORITY: Priority.MEDIUM
[ INFO ] LOADED_FROM_CACHE: False
[Step 9/11] Creating infer requests and preparing input tensors
[ WARNING ] No input files were given for input 'input.1'!. This input will be filled with random values!
[ INFO ] Fill input 'input.1' with random values
[Step 10/11] Measuring performance (Start inference synchronously, limits: 15000 ms duration)
[ INFO ] Benchmarking in inference only mode (inputs filling are not included in measurement loop).
[ INFO ] First inference took 24.68 ms
[Step 11/11] Dumping statistics report
[ INFO ] Execution Devices:['CPU']
[ INFO ] Count: 1366 iterations
[ INFO ] Duration: 15004.33 ms
[ INFO ] Latency:
[ INFO ] Median: 10.75 ms
[ INFO ] Average: 10.80 ms
[ INFO ] Min: 10.53 ms
[ INFO ] Max: 12.59 ms
[ INFO ] Throughput: 91.04 FPS
データのダウンロードと準備¶
リアルタイムの推論用の検証ビデオを 1 つダウンロードします。
このチュートリアルでは、後にリリースされるトレーニングおよび量子化ノートブックでも使用された KitsDataset
クラスを再利用します。
データは BASEDIR
内に存在する必要があります。BASEDIR
ディレクトリーには、case_00000
から case_00299
までのサブディレクトリーが含まれている必要があります。上記で指定したケースのデータが存在しない場合、ダウンロードされ、次のセルに抽出されます。
# Directory that contains the CT scan data. This directory should contain subdirectories
# case_00XXX where XXX is between 000 and 299.
BASEDIR = Path("kits19_frames_1")
# The CT scan case number. For example: 16 for data from the case_00016 directory.
# Currently only 117 is supported.
CASE = 117
case_path = BASEDIR / f"case_{CASE:05d}"
if not case_path.exists():
filename = download_file(
f"https://storage.openvinotoolkit.org/data/test_data/openvino_notebooks/kits19/case_{CASE:05d}.zip"
)
with zipfile.ZipFile(filename, "r") as zip_ref:
zip_ref.extractall(path=BASEDIR)
os.remove(filename) # remove zipfile
print(f"Downloaded and extracted data for case_{CASE:05d}")
else:
print(f"Data for case_{CASE:05d} exists")
case_00117.zip: 0%| | 0.00/5.48M [00:00<?, ?B/s]
Downloaded and extracted data for case_00117
リアルタイムの推論を表示¶
ノートブック内のモデルでリアルタイムの推論を表示するには、OpenVINO ランタイム の非同期処理機能を使用します。
device="GPU"
または device="MULTI:CPU,GPU"
を指定して GPU デバイスを使用し、統合グラフィック・カードで推論を行う場合、コードを初めて実行するとモデルの読み込みが遅くなります。モデルはキャッシュされるため、初回以降のモデルの読み込みは高速になります。モデルキャッシュを含む OpenVINO ランタイムの詳細については、OpenVINO API チュートリアルを参照してください。
非同期推論を実行するには、AsyncInferQueue を使用します。コンパイルされたモデルと複数のジョブ (並列実行スレッド) を使用してインスタンス化できます 。ジョブ数を渡さないか、0
を渡した場合、OpenVINO はデバイスとヒューリスティックに基づいて最適な数を選択します。推論キューを取得したら、次の 2 つの作業を実行する必要があります。
データを前処理し、推論キューにプッシュします。前処理のステップは同じままです
推論が完了した後にモデル出力をどう処理するか推論キューに指示します。これは、推論結果と、準備された入力データとともに推論キューに渡されたデータを受け取るコールバック Python関数によって表されます。
その他すべては AsyncInferQueue
インスタンスによって処理されます。
Open Model Zoo のモデル API に基づいて、SegmentationModel
を使用してセグメント化モデルを OpenVINO ランタイムにロードします。このモデルの実装には、モデルの前処理と後処理が含まれます。SegmentationModel
の場合、これには元の画像/フレームにセグメント化マスクのオーバーレイを作成するコードが含まれます。実装を確認するには、次のセルのコメントを解除します。
core = ov.Core()
segmentation_model = SegmentationModel(
ie=core, model_path=Path(MODEL_PATH), sigmoid=True, rotate_and_flip=True
)
image_paths = sorted(case_path.glob("imaging_frames/*jpg"))
print(f"{case_path.name}, {len(image_paths)} images")
case_00117, 69 images
トレーニング・チュートリアルと同じ方法で、reader = LoadImage()
関数を使用して画像を読み取ります。
framebuf = []
next_frame_id = 0
reader = LoadImage(image_only=True, dtype=np.uint8)
while next_frame_id < len(image_paths) - 1:
image_path = image_paths[next_frame_id]
image = reader(str(image_path))
framebuf.append(image)
next_frame_id += 1
device
Dropdown(description='Device:', index=1, options=('CPU', 'AUTO'), value='AUTO')
コールバックが設定されている場合、推論を終了するジョブはすべて Python 関数を呼び出します。コールバック関数には 2 つの引数が必要です。1 つはコールバックを呼び出す要求で、InferRequest
を提供します。もう 1 つは userdata
と呼ばれ、ランタイム値を渡す可能性を提供します。
コールバック関数は推論の結果を表示します。
import cv2
import copy
from IPython import display
from typing import Dict, Any
# Define a callback function that runs every time the asynchronous pipeline completes inference on a frame
def completion_callback(infer_request: ov.InferRequest, user_data: Dict[str, Any],) -> None:
preprocess_meta = user_data['preprocess_meta']
raw_outputs = {out.any_name: copy.deepcopy(res.data) for out, res in zip(infer_request.model_outputs, infer_request.output_tensors)}
frame = segmentation_model.postprocess(raw_outputs, preprocess_meta)
_, encoded_img = cv2.imencode(".jpg", frame, params=[cv2.IMWRITE_JPEG_QUALITY, 90])
# Create IPython image
i = display.Image(data=encoded_img)
# Display the image in this notebook
display.clear_output(wait=True)
display.display(i)
import time
load_start_time = time.perf_counter()
compiled_model = core.compile_model(segmentation_model.net, device.value)
# Create asynchronous inference queue with optimal number of infer requests
infer_queue = ov.AsyncInferQueue(compiled_model)
infer_queue.set_callback(completion_callback)
load_end_time = time.perf_counter()
results = [None] * len(framebuf)
frame_number = 0
# Perform inference on every frame in the framebuffer
start_time = time.time()
for i, input_frame in enumerate(framebuf):
inputs, preprocessing_meta = segmentation_model.preprocess({segmentation_model.net.input(0): input_frame})
infer_queue.start_async(inputs, {'preprocess_meta': preprocessing_meta})
# Wait until all inference requests in the AsyncInferQueue are completed
infer_queue.wait_all()
stop_time = time.time()
# Calculate total inference time and FPS
total_time = stop_time - start_time
fps = len(framebuf) / total_time
time_per_frame = 1 / fps
print(f"Loaded model to {device} in {load_end_time-load_start_time:.2f} seconds.")
print(f'Total time to infer all frames: {total_time:.3f}s')
print(f'Time per frame: {time_per_frame:.6f}s ({fps:.3f} FPS)')
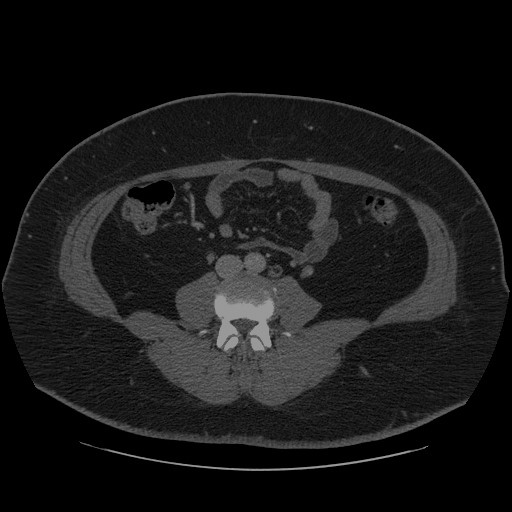
Loaded model to Dropdown(description='Device:', index=1, options=('CPU', 'AUTO'), value='AUTO') in 0.23 seconds.
Total time to infer all frames: 2.588s
Time per frame: 0.038061s (26.274 FPS)