OpenVINO™ による人間の行動認識¶
この Jupyter ノートブックはオンラインで起動でき、ブラウザーのウィンドウで対話型環境を開きます。ローカルにインストールすることもできます。次のオプションのいずれかを選択します。
このノートブックでは、Open Model Zoo の行動認識モデル、具体的にはエンコーダーとデコーダーを使用して、OpenVINO によるリアルタイムの人間の行動認識を示します。
どちらのモデルも、Kinetics-400データセットの人間の活動を識別するために、シーケンスツーシーケンス ("seq2seq"
) [1] システムを作成します。モデルは ResNet34 エンコーダー [2] を使用したビデオ・トランスフォーマーのアプローチを採用しています。ノートブックでは、次のパイプラインを作成する方法を示します。
このノートブックの最後では、ウェブカメラからのリアルタイムの推論結果が表示されます。 さらに、ビデオファイルをアップロードすることもできます。
注: ウェブカメラを使用するには、ウェブカメラを備えたコンピューター上でこの Jupyter ノートブックを実行する必要があります。サーバー上で実行すると、ウェブカメラは機能しなくなります。ただし、最終ステップではビデオに対して推論を行うことができます。
[1] seq2seq: 一連の項目を入力と出力に受け取るディープラーニング・モデル。この場合、入力: ビデオフレーム、出力: アクションシーケンス。この "seq2seq"
は、エンコーダーとデコーダーで構成されています。エンコーダーは、デコーダーによって分析される入力の "context"
をキャプチャーし、最終的に人間のアクションと信頼性を取得します。
[2] ビデオ・トランスフォーマーと ResNet34。
目次¶
%pip install -q "openvino-dev>=2023.1.0"
DEPRECATION: pytorch-lightning 1.6.5 has a non-standard dependency specifier torch>=1.8.*. pip 24.1 will enforce this behaviour change. A possible replacement is to upgrade to a newer version of pytorch-lightning or contact the author to suggest that they release a version with a conforming dependency specifiers. Discussion can be found at https://github.com/pypa/pip/issues/12063
Note: you may need to restart the kernel to use updated packages.
インポート¶
import collections
import os
import time
from typing import Tuple, List
from pathlib import Path
import cv2
import numpy as np
from IPython import display
import openvino as ov
from openvino.runtime.ie_api import CompiledModel
# Fetch `notebook_utils` module
import urllib.request
urllib.request.urlretrieve(
url='https://raw.githubusercontent.com/openvinotoolkit/openvino_notebooks/main/notebooks/utils/notebook_utils.py',
filename='notebook_utils.py'
)
import notebook_utils as utils
モデル¶
モデルのダウンロード¶
notebook_utils
ファイルの関数である download_ir_model
を使用します。ディレクトリー構造が自動的に作成され、選択したモデルがダウンロードされます。
この場合、モデル名として "action-recognition-0001"
を使用すると、システムは自動的に2つのモデル "action-recognition-0001-encoder"
と"action-recognition-0001-decoder"
をダウンロードします。
注:
"driver-action-recognition-adas-0002"
("driver-action-recognition-adas-0002-encoder"
+"driver-action-recognition-adas-0002-decoder"
) など別のモデルをダウンロードする場合は、以下のコードでモデル名を置き換えます。リスト外のモデルを使用する場合は、異なる前処理と後処理が必要になる場合があります。
# A directory where the model will be downloaded.
base_model_dir = "model"
# The name of the model from Open Model Zoo.
model_name = "action-recognition-0001"
# Selected precision (FP32, FP16, FP16-INT8).
precision = "FP16"
model_path_decoder = (
f"model/intel/{model_name}/{model_name}-decoder/{precision}/{model_name}-decoder.xml"
)
model_path_encoder = (
f"model/intel/{model_name}/{model_name}-encoder/{precision}/{model_name}-encoder.xml"
)
encoder_url = f"https://storage.openvinotoolkit.org/repositories/open_model_zoo/temp/{model_name}/{model_name}-encoder/{precision}/{model_name}-encoder.xml"
decoder_url = f"https://storage.openvinotoolkit.org/repositories/open_model_zoo/temp/{model_name}/{model_name}-decoder/{precision}/{model_name}-decoder.xml"
if not os.path.exists(model_path_decoder):
utils.download_ir_model(decoder_url, Path(model_path_decoder).parent)
if not os.path.exists(model_path_encoder):
utils.download_ir_model(encoder_url, Path(model_path_encoder).parent)
model/intel/action-recognition-0001/action-recognition-0001-decoder/FP16/action-recognition-0001-decoder.bin: …
model/intel/action-recognition-0001/action-recognition-0001-encoder/FP16/action-recognition-0001-encoder.bin: …
ラベルの読み込み¶
このチュートリアルでは Kinetics-400 データセットを使用し、このノートブックに埋め込まれたテキストファイルも提供します。
注:
"driver-action-recognition-adas-0002"
モデルを実行する場合は、kinetics.txt
ファイルをdriver_actions.txt
に置き換えます。
# Download the text from the openvino_notebooks storage
vocab_file_path = utils.download_file(
"https://storage.openvinotoolkit.org/repositories/openvino_notebooks/data/data/text/kinetics.txt",
directory="data"
)
with vocab_file_path.open(mode='r') as f:
labels = [line.strip() for line in f]
print(labels[0:9], np.shape(labels))
data/kinetics.txt: 0%| | 0.00/5.82k [00:00<?, ?B/s]
['abseiling', 'air drumming', 'answering questions', 'applauding', 'applying cream', 'archery', 'arm wrestling', 'arranging flowers', 'assembling computer'] (400,)
モデルのロード¶
特定のアーキテクチャーの 2 つのモデル (エンコーダーとデコーダー) をロードします。ダウンロードされたモデルは、ベンダー、モデル名、精度を示す固定構造に配置されます。
OpenVINO ランタイムを初期化します。
*.bin
と*.xml
ファイルからネットワークを読み取ります (重みとアーキテクチャー)。指定されたデバイス用にモデルをコンパイルします。
ノードの入力名と出力名を取得します。
モデルを実行するには、数行のコードで済みます。
OpenVINO を使用して推論を実行するデバイスをドロップダウン・リストから選択します。
import ipywidgets as widgets
core = ov.Core()
device = widgets.Dropdown(
options=core.available_devices + ["AUTO"],
value='AUTO',
description='Device:',
disabled=False,
)
device
Dropdown(description='Device:', index=1, options=('CPU', 'AUTO'), value='AUTO')
モデルの初期化関数¶
# Initialize OpenVINO Runtime.
core = ov.Core()
def model_init(model_path: str, device: str) -> Tuple:
"""
Read the network and weights from a file, load the
model on CPU and get input and output names of nodes
:param:
model: model architecture path *.xml
device: inference device
:retuns:
compiled_model: Compiled model
input_key: Input node for model
output_key: Output node for model
"""
# Read the network and corresponding weights from a file.
model = core.read_model(model=model_path)
# Compile the model for specified device.
compiled_model = core.compile_model(model=model, device_name=device)
# Get input and output names of nodes.
input_keys = compiled_model.input(0)
output_keys = compiled_model.output(0)
return input_keys, output_keys, compiled_model
エンコーダとデコーダの初期化¶
# Encoder initialization
input_key_en, output_keys_en, compiled_model_en = model_init(model_path_encoder, device.value)
# Decoder initialization
input_key_de, output_keys_de, compiled_model_de = model_init(model_path_decoder, device.value)
# Get input size - Encoder.
height_en, width_en = list(input_key_en.shape)[2:]
# Get input size - Decoder.
frames2decode = list(input_key_de.shape)[0:][1]
フレームの前処理と後処理には、次のヘルパー関数を使用します。
-
エンコーダー・モデルを実行する前に入力画像を前処理します (
center_crop
とadaptative_resize
)。 上位 3 つの確率をラベル名にデコードします (
decode_output
)。-
ビデオの関心領域 (ROI) (
rec_frame_display
)。 -
ビデオにラベル名を表示するフレームを準備します (
display_text_fnc
)。
def center_crop(frame: np.ndarray) -> np.ndarray:
"""
Center crop squared the original frame to standardize the input image to the encoder model
:param frame: input frame
:returns: center-crop-squared frame
"""
img_h, img_w, _ = frame.shape
min_dim = min(img_h, img_w)
start_x = int((img_w - min_dim) / 2.0)
start_y = int((img_h - min_dim) / 2.0)
roi = [start_y, (start_y + min_dim), start_x, (start_x + min_dim)]
return frame[start_y : (start_y + min_dim), start_x : (start_x + min_dim), ...], roi
def adaptive_resize(frame: np.ndarray, size: int) -> np.ndarray:
"""
The frame going to be resized to have a height of size or a width of size
:param frame: input frame
:param size: input size to encoder model
:returns: resized frame, np.array type
"""
h, w, _ = frame.shape
scale = size / min(h, w)
w_scaled, h_scaled = int(w * scale), int(h * scale)
if w_scaled == w and h_scaled == h:
return frame
return cv2.resize(frame, (w_scaled, h_scaled))
def decode_output(probs: np.ndarray, labels: np.ndarray, top_k: int = 3) -> np.ndarray:
"""
Decodes top probabilities into corresponding label names
:param probs: confidence vector for 400 actions
:param labels: list of actions
:param top_k: The k most probable positions in the list of labels
:returns: decoded_labels: The k most probable actions from the labels list
decoded_top_probs: confidence for the k most probable actions
"""
top_ind = np.argsort(-1 * probs)[:top_k]
out_label = np.array(labels)[top_ind.astype(int)]
decoded_labels = [out_label[0][0], out_label[0][1], out_label[0][2]]
top_probs = np.array(probs)[0][top_ind.astype(int)]
decoded_top_probs = [top_probs[0][0], top_probs[0][1], top_probs[0][2]]
return decoded_labels, decoded_top_probs
def rec_frame_display(frame: np.ndarray, roi) -> np.ndarray:
"""
Draw a rec frame over actual frame
:param frame: input frame
:param roi: Region of interest, image section processed by the Encoder
:returns: frame with drawed shape
"""
cv2.line(frame, (roi[2] + 3, roi[0] + 3), (roi[2] + 3, roi[0] + 100), (0, 200, 0), 2)
cv2.line(frame, (roi[2] + 3, roi[0] + 3), (roi[2] + 100, roi[0] + 3), (0, 200, 0), 2)
cv2.line(frame, (roi[3] - 3, roi[1] - 3), (roi[3] - 3, roi[1] - 100), (0, 200, 0), 2)
cv2.line(frame, (roi[3] - 3, roi[1] - 3), (roi[3] - 100, roi[1] - 3), (0, 200, 0), 2)
cv2.line(frame, (roi[3] - 3, roi[0] + 3), (roi[3] - 3, roi[0] + 100), (0, 200, 0), 2)
cv2.line(frame, (roi[3] - 3, roi[0] + 3), (roi[3] - 100, roi[0] + 3), (0, 200, 0), 2)
cv2.line(frame, (roi[2] + 3, roi[1] - 3), (roi[2] + 3, roi[1] - 100), (0, 200, 0), 2)
cv2.line(frame, (roi[2] + 3, roi[1] - 3), (roi[2] + 100, roi[1] - 3), (0, 200, 0), 2)
# Write ROI over actual frame
FONT_STYLE = cv2.FONT_HERSHEY_SIMPLEX
org = (roi[2] + 3, roi[1] - 3)
org2 = (roi[2] + 2, roi[1] - 2)
FONT_SIZE = 0.5
FONT_COLOR = (0, 200, 0)
FONT_COLOR2 = (0, 0, 0)
cv2.putText(frame, "ROI", org2, FONT_STYLE, FONT_SIZE, FONT_COLOR2)
cv2.putText(frame, "ROI", org, FONT_STYLE, FONT_SIZE, FONT_COLOR)
return frame
def display_text_fnc(frame: np.ndarray, display_text: str, index: int):
"""
Include a text on the analyzed frame
:param frame: input frame
:param display_text: text to add on the frame
:param index: index line dor adding text
"""
# Configuration for displaying images with text.
FONT_COLOR = (255, 255, 255)
FONT_COLOR2 = (0, 0, 0)
FONT_STYLE = cv2.FONT_HERSHEY_DUPLEX
FONT_SIZE = 0.7
TEXT_VERTICAL_INTERVAL = 25
TEXT_LEFT_MARGIN = 15
# ROI over actual frame
(processed, roi) = center_crop(frame)
# Draw a ROI over actual frame.
frame = rec_frame_display(frame, roi)
# Put a text over actual frame.
text_loc = (TEXT_LEFT_MARGIN, TEXT_VERTICAL_INTERVAL * (index + 1))
text_loc2 = (TEXT_LEFT_MARGIN + 1, TEXT_VERTICAL_INTERVAL * (index + 1) + 1)
cv2.putText(frame, display_text, text_loc2, FONT_STYLE, FONT_SIZE, FONT_COLOR2)
cv2.putText(frame, display_text, text_loc, FONT_STYLE, FONT_SIZE, FONT_COLOR)
上記のパイプラインに従って、次の関数を使用して操作を行います。
エンコーダーを実行する前にフレームを前処理します (
preprocessing
)。フレームごとのエンコーダー推論 (
encoder
)。フレームセットごとのデコーダー推論 (
decoder
)。デコーダー出力を正規化して、行動認識ラベルごとに信頼値を取得します (
softmax
)。
def preprocessing(frame: np.ndarray, size: int) -> np.ndarray:
"""
Preparing frame before Encoder.
The image should be scaled to its shortest dimension at "size"
and cropped, centered, and squared so that both width and
height have lengths "size". The frame must be transposed from
Height-Width-Channels (HWC) to Channels-Height-Width (CHW).
:param frame: input frame
:param size: input size to encoder model
:returns: resized and cropped frame
"""
# Adaptative resize
preprocessed = adaptive_resize(frame, size)
# Center_crop
(preprocessed, roi) = center_crop(preprocessed)
# Transpose frame HWC -> CHW
preprocessed = preprocessed.transpose((2, 0, 1))[None,] # HWC -> CHW
return preprocessed, roi
def encoder(
preprocessed: np.ndarray,
compiled_model: CompiledModel
) -> List:
"""
Encoder Inference per frame. This function calls the network previously
configured for the encoder model (compiled_model), extracts the data
from the output node, and appends it in an array to be used by the decoder.
:param: preprocessed: preprocessing frame
:param: compiled_model: Encoder model network
:returns: encoder_output: embedding layer that is appended with each arriving frame
"""
output_key_en = compiled_model.output(0)
# Get results on action-recognition-0001-encoder model
infer_result_encoder = compiled_model([preprocessed])[output_key_en]
return infer_result_encoder
def decoder(encoder_output: List, compiled_model_de: CompiledModel) -> List:
"""
Decoder inference per set of frames. This function concatenates the embedding layer
froms the encoder output, transpose the array to match with the decoder input size.
Calls the network previously configured for the decoder model (compiled_model_de), extracts
the logits and normalize those to get confidence values along specified axis.
Decodes top probabilities into corresponding label names
:param: encoder_output: embedding layer for 16 frames
:param: compiled_model_de: Decoder model network
:returns: decoded_labels: The k most probable actions from the labels list
decoded_top_probs: confidence for the k most probable actions
"""
# Concatenate sample_duration frames in just one array
decoder_input = np.concatenate(encoder_output, axis=0)
# Organize input shape vector to the Decoder (shape: [1x16x512]]
decoder_input = decoder_input.transpose((2, 0, 1, 3))
decoder_input = np.squeeze(decoder_input, axis=3)
output_key_de = compiled_model_de.output(0)
# Get results on action-recognition-0001-decoder model
result_de = compiled_model_de([decoder_input])[output_key_de]
# Normalize logits to get confidence values along specified axis
probs = softmax(result_de - np.max(result_de))
# Decodes top probabilities into corresponding label names
decoded_labels, decoded_top_probs = decode_output(probs, labels, top_k=3)
return decoded_labels, decoded_top_probs
def softmax(x: np.ndarray) -> np.ndarray:
"""
Normalizes logits to get confidence values along specified axis
x: np.array, axis=None
"""
exp = np.exp(x)
return exp / np.sum(exp, axis=None)
実行中の行動認識機能は、ウェブカメラまたはビデオファイルのいずれか異なる操作で実行されます。以下の手順のリストを参照してください。
ターゲット fps で再生するビデオプレーヤーを作成します (
utils.VideoPlayer
)。エンコード/デコードするフレームのセットを準備します。
AI 関数を実行します。
結果を視覚化します。
def run_action_recognition(
source: str = "0",
flip: bool = True,
use_popup: bool = False,
compiled_model_en: CompiledModel = compiled_model_en,
compiled_model_de: CompiledModel = compiled_model_de,
skip_first_frames: int = 0,
):
"""
Use the "source" webcam or video file to run the complete pipeline for action-recognition problem
1. Create a video player to play with target fps
2. Prepare a set of frames to be encoded-decoded
3. Preprocess frame before Encoder
4. Encoder Inference per frame
5. Decoder inference per set of frames
6. Visualize the results
:param: source: webcam "0" or video path
:param: flip: to be used by VideoPlayer function for flipping capture image
:param: use_popup: False for showing encoded frames over this notebook, True for creating a popup window.
:param: skip_first_frames: Number of frames to skip at the beginning of the video.
:returns: display video over the notebook or in a popup window
"""
size = height_en # Endoder input size - From Cell 5_9
sample_duration = frames2decode # Decoder input size - From Cell 5_7
# Select frames per second of your source.
fps = 30
player = None
try:
# Create a video player.
player = utils.VideoPlayer(source, flip=flip, fps=fps, skip_first_frames=skip_first_frames)
# Start capturing.
player.start()
if use_popup:
title = "Press ESC to Exit"
cv2.namedWindow(title, cv2.WINDOW_GUI_NORMAL | cv2.WINDOW_AUTOSIZE)
processing_times = collections.deque()
processing_time = 0
encoder_output = []
decoded_labels = [0, 0, 0]
decoded_top_probs = [0, 0, 0]
counter = 0
# Create a text template to show inference results over video.
text_inference_template = "Infer Time:{Time:.1f}ms,{fps:.1f}FPS"
text_template = "{label},{conf:.2f}%"
while True:
counter = counter + 1
# Read a frame from the video stream.
frame = player.next()
if frame is None:
print("Source ended")
break
scale = 1280 / max(frame.shape)
# Adaptative resize for visualization.
if scale < 1:
frame = cv2.resize(frame, None, fx=scale, fy=scale, interpolation=cv2.INTER_AREA)
# Select one frame every two for processing through the encoder.
# After 16 frames are processed, the decoder will find the action,
# and the label will be printed over the frames.
if counter % 2 == 0:
# Preprocess frame before Encoder.
(preprocessed, _) = preprocessing(frame, size)
# Measure processing time.
start_time = time.time()
# Encoder Inference per frame
encoder_output.append(encoder(preprocessed, compiled_model_en))
# Decoder inference per set of frames
# Wait for sample duration to work with decoder model.
if len(encoder_output) == sample_duration:
decoded_labels, decoded_top_probs = decoder(encoder_output, compiled_model_de)
encoder_output = []
# Inference has finished. Display the results.
stop_time = time.time()
# Calculate processing time.
processing_times.append(stop_time - start_time)
# Use processing times from last 200 frames.
if len(processing_times) > 200:
processing_times.popleft()
# Mean processing time [ms]
processing_time = np.mean(processing_times) * 1000
fps = 1000 / processing_time
# Visualize the results.
for i in range(0, 3):
display_text = text_template.format(
label=decoded_labels[i],
conf=decoded_top_probs[i] * 100,
)
display_text_fnc(frame, display_text, i)
display_text = text_inference_template.format(Time=processing_time, fps=fps)
display_text_fnc(frame, display_text, 3)
# Use this workaround if you experience flickering.
if use_popup:
cv2.imshow(title, frame)
key = cv2.waitKey(1)
# escape = 27
if key == 27:
break
else:
# Encode numpy array to jpg.
_, encoded_img = cv2.imencode(".jpg", frame, params=[cv2.IMWRITE_JPEG_QUALITY, 90])
# Create an IPython image.
i = display.Image(data=encoded_img)
# Display the image in this notebook.
display.clear_output(wait=True)
display.display(i)
# ctrl-c
except KeyboardInterrupt:
print("Interrupted")
# Any different error
except RuntimeError as e:
print(e)
finally:
if player is not None:
# Stop capturing.
player.stop()
if use_popup:
cv2.destroyAllWindows()
ビデオファイルでモデルがどのように動作するか確認します。OpenCV でサポートされている形式であればどれでも動作します。ビデオファイルの実行中はいつでも停止ボタンを押すことができ、次のステップのためウェブカメラが起動します。
注: フレームが破損していると、ビデオが途切れる場合があります。その場合、変換することができます。ビデオに問題が発生した場合は、HandBrake を使用して MPEG 形式を選択してください。
デモの入力ソースとしてウェブカメラを使用する場合、USE_WEBCAM
変数の値を True に変更し、cam_id
を指定してください (デフォルト値は 0 ですが、マルチカメラ・システムでは異なる場合があります)。
USE_WEBCAM = False
cam_id = 0
video_file = "https://archive.org/serve/ISSVideoResourceLifeOnStation720p/ISS%20Video%20Resource_LifeOnStation_720p.mp4"
source = cam_id if USE_WEBCAM else video_file
additional_options = {"skip_first_frames": 600, "flip": False} if not USE_WEBCAM else {"flip": True}
run_action_recognition(source=source, use_popup=False, **additional_options)
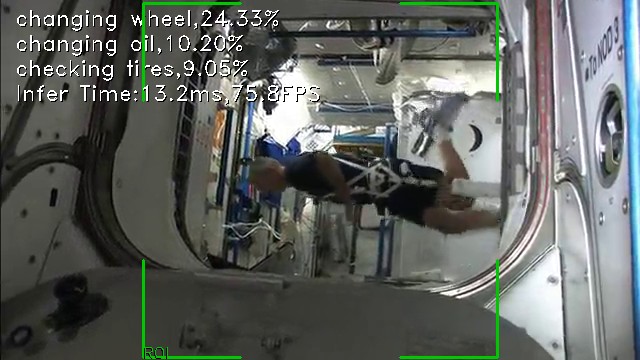
Source ended