PaddleGAN と OpenVINO™ による超解像度化¶
この Jupyter ノートブックはオンラインで起動でき、ブラウザーのウィンドウで対話型環境を開きます。ローカルにインストールすることもできます。次のオプションのいずれかを選択します。
このノートブックは、RealSR (現実世界超解像度) モデルを PaddlePaddle/PaddleGAN から OpenVINO 中間表現 (OpenVINO IR) 形式に変換する方法を示し、PaddleGAN モデルと OpenVINO IR モデル両方の推論結果を示します。
PaddleGAN 超解像度モデルの詳細については、PaddleGAN のドキュメントを参照してください。RealSR の詳細については、CVPR 2020 の研究論文を参照してください。
このノートブックは、小さな画像 (解像度 800x600 まで) に最適です。
目次¶
インポート¶
%pip install -q "openvino>=2023.1.0"
%pip install -q "paddlepaddle>=2.5.1" "paddle2onnx>=0.6"
%pip install -q "imageio==2.9.0" "imageio-ffmpeg" "numba>=0.53.1" "easydict" "munch" "natsort"
%pip install -q "git+https://github.com/PaddlePaddle/PaddleGAN.git" --no-deps
%pip install -q scikit-image
Note: you may need to restart the kernel to use updated packages.
Note: you may need to restart the kernel to use updated packages.
Note: you may need to restart the kernel to use updated packages.
Note: you may need to restart the kernel to use updated packages.
ERROR: pip's dependency resolver does not currently take into account all the packages that are installed. This behaviour is the source of the following dependency conflicts.
ppgan 2.1.0 requires imageio==2.9.0, but you have imageio 2.33.1 which is incompatible.
ppgan 2.1.0 requires librosa==0.8.1, but you have librosa 0.10.1 which is incompatible.
ppgan 2.1.0 requires opencv-python<=4.6.0.66, but you have opencv-python 4.9.0.80 which is incompatible.
Note: you may need to restart the kernel to use updated packages.
import time
import warnings
from pathlib import Path
import cv2
import matplotlib.pyplot as plt
import numpy as np
import openvino as ov
import paddle
from IPython.display import HTML, FileLink, ProgressBar, clear_output, display
from IPython.display import Image as DisplayImage
from PIL import Image
from paddle.static import InputSpec
from ppgan.apps import RealSRPredictor
# Fetch `notebook_utils` module
import urllib.request
urllib.request.urlretrieve(
url='https://raw.githubusercontent.com/openvinotoolkit/openvino_notebooks/main/notebooks/utils/notebook_utils.py',
filename='notebook_utils.py'
)
from notebook_utils import NotebookAlert, download_file
設定¶
# The filenames of the downloaded and converted models.
MODEL_NAME = "paddlegan_sr"
MODEL_DIR = Path("model")
OUTPUT_DIR = Path("output")
OUTPUT_DIR.mkdir(exist_ok=True)
model_path = MODEL_DIR / MODEL_NAME
ir_path = model_path.with_suffix(".xml")
onnx_path = model_path.with_suffix(".onnx")
PaddlePaddle モデルの推論¶
PaddleGAN モデルの調査¶
PaddleGAN のドキュメントでは、sr.run()
メソッドを使用してモデルを実行する方法が説明されています。関数が何を行うのかを調べ、その関数から呼び出される関連する関数を確認します。追加 ??
メソッドには、ドックストリングとソースコードが表示されます。
# Running this cell will download the model weights if they have not been downloaded before.
# This may take a while.
sr = RealSRPredictor()
[02/09 23:42:09] ppgan INFO: Found /opt/home/k8sworker/.cache/ppgan/DF2K_JPEG.pdparams
sr.run??
sr.run_image??
sr.norm??
sr.denorm??
実行では、入力が画像であるかビデオであるかチェックされます。画像の場合、RGB
画像として読み込み、正規化して Paddle テンソルに変換します。これは、self.model()
メソッドを呼び出すことによってネットワークに伝播され、その後非正規化されます。正規化関数は、すべての画像値を単純に 255 で除算します。これは、0 ~ 255 の範囲の整数値を含むイメージを、0 ~ 1 の範囲の浮動小数点値を含むイメージに変換します。非正規化関数は、出力を (C、H、W) ネットワーク形状から (H、W、C) 画像形状に変換します。次に、0 ~ 255 の間の画像値をクリップし、その画像を 0 ~ 255 の範囲の整数値を持つ標準 RGB
イメージに変換します。
モデルがどのように見えるか詳細情報を取得するには、sr.model??
コマンドを使用します。
# sr.model??
推論の実行¶
PaddlePaddle モデルの推論を表示するには、下のセルで PADDLEGAN_INFERENCE
を True
に設定します。推論の実行には時間がかかることがあることに注意してください。
# Load the image from openvino storage
IMAGE_PATH = download_file(
"https://storage.openvinotoolkit.org/repositories/openvino_notebooks/data/data/image/coco_tulips.jpg",
directory="data"
)
data/coco_tulips.jpg: 0%| | 0.00/63.6k [00:00<?, ?B/s]
# Set PADDLEGAN_INFERENCE to True to show inference on the PaddlePaddle model.
# This may take a long time, especially for larger images.
#
PADDLEGAN_INFERENCE = False
if PADDLEGAN_INFERENCE:
# Load the input image and convert to a tensor with the input shape.
image = cv2.cvtColor(cv2.imread(str(IMAGE_PATH)), cv2.COLOR_BGR2RGB)
input_image = image.transpose(2, 0, 1)[None, :, :, :] / 255
input_tensor = paddle.to_tensor(input_image.astype(np.float32))
if max(image.shape) > 400:
NotebookAlert(
f"This image has {image.shape} shape. Doing inference will be slow "
"and the notebook may stop responding. Set PADDLEGAN_INFERENCE to False "
"to skip doing inference on the PaddlePaddle model.",
"warning",
)
if PADDLEGAN_INFERENCE:
# Do inference and measure how long it takes.
print(f"Start superresolution inference for {IMAGE_PATH.name} with shape {image.shape}...")
start_time = time.perf_counter()
sr.model.eval()
with paddle.no_grad():
result = sr.model(input_tensor)
end_time = time.perf_counter()
duration = end_time - start_time
result_image = (
(result.numpy().squeeze() * 255).clip(0, 255).astype("uint8").transpose((1, 2, 0))
)
print(f"Superresolution image shape: {result_image.shape}")
print(f"Inference duration: {duration:.2f} seconds")
plt.imshow(result_image);
PaddleGAN モデルを ONNX および OpenVINO IR に変換¶
PaddlePaddle モデルを OpenVINO IR に変換するには、まずモデルを ONNX に変換し、次に ONNX モデルを OpenVINO IR 形式に変換します。
PaddlePaddle モデルを ONNX に変換¶
# Ignore PaddlePaddle warnings:
# The behavior of expression A + B has been unified with elementwise_add(X, Y, axis=-1).
warnings.filterwarnings("ignore")
sr.model.eval()
# ONNX export requires an input shape in this format as a parameter.
# Both OpenVINO and Paddle support `-1` placeholder for marking flexible dimensions
input_shape = [-1, 3, -1, -1]
x_spec = InputSpec(input_shape, "float32", "x")
paddle.onnx.export(sr.model, str(model_path), input_spec=[x_spec], opset_version=13)
2024-02-09 23:42:16 [INFO] Static PaddlePaddle model saved in model/paddle_model_static_onnx_temp_dir.
I0209 23:42:16.006111 2843987 program_interpreter.cc:212] New Executor is Running.
[Paddle2ONNX] Start to parse PaddlePaddle model...
[Paddle2ONNX] Model file path: model/paddle_model_static_onnx_temp_dir/model.pdmodel
[Paddle2ONNX] Paramters file path: model/paddle_model_static_onnx_temp_dir/model.pdiparams
[Paddle2ONNX] Start to parsing Paddle model...
[Paddle2ONNX] Use opset_version = 13 for ONNX export.
[Paddle2ONNX] PaddlePaddle model is exported as ONNX format now.
2024-02-09 23:42:19 [INFO] ONNX model saved in model/paddlegan_sr.onnx.
モデル変換 Python API を使用して ONNX モデルを OpenVINO IR に変換¶
print("Exporting ONNX model to OpenVINO IR... This may take a few minutes.")
model = ov.convert_model(
onnx_path,
input=input_shape
)
# Serialize model in IR format
ov.save_model(model, str(ir_path))
Exporting ONNX model to OpenVINO IR... This may take a few minutes.
OpenVINO IR モデルで推論を行う¶
# Read the network and get input and output names.
core = ov.Core()
# Alternatively, the model obtained from `ov.convert_model()` may be used here
model = core.read_model(model=ir_path)
input_layer = model.input(0)
推論デバイスの選択¶
OpenVINO を使用して推論を実行するためにドロップダウン・リストからデバイスを選択します。
import ipywidgets as widgets
device = widgets.Dropdown(
options=core.available_devices + ["AUTO"],
value='AUTO',
description='Device:',
disabled=False,
)
device
Dropdown(description='Device:', index=1, options=('CPU', 'AUTO'), value='AUTO')
image = cv2.cvtColor(cv2.imread(str(IMAGE_PATH)), cv2.COLOR_BGR2RGB)
if max(image.shape) > 800:
NotebookAlert(
f"This image has shape {image.shape}. The notebook works best with images with "
"a maximum side of 800x600. Larger images may work well, but inference may "
"be slow",
"warning",
)
plt.imshow(image)
<matplotlib.image.AxesImage at 0x7fe9ebed32e0>
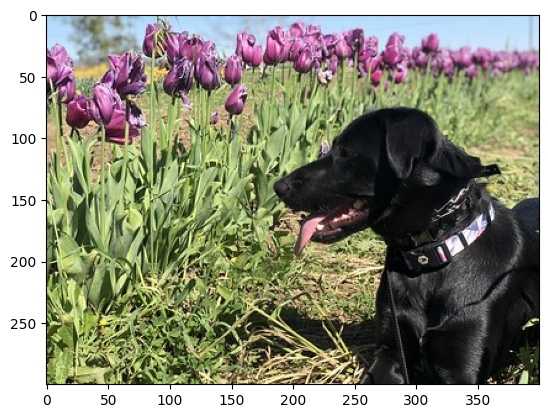
# Load the network to the CPU device (this may take a few seconds).
compiled_model = core.compile_model(model=model, device_name=device.value)
output_layer = compiled_model.output(0)
# Convert the image to the network input shape and divide pixel values by 255.
# See the "Investigate PaddleGAN model" section.
input_image = image.transpose(2, 0, 1)[None, :, :, :] / 255
start_time = time.perf_counter()
# Do inference.
ir_result = compiled_model([input_image])[output_layer]
end_time = time.perf_counter()
duration = end_time - start_time
print(f"Inference duration: {duration:.2f} seconds")
Inference duration: 3.27 seconds
# Get the result array in CHW format.
result_array = ir_result.squeeze()
# Convert the array to an image with the same method as PaddleGAN:
# Multiply by 255, clip values between 0 and 255, convert to a HWC INT8 image.
# See the "Investigate PaddleGAN model" section.
image_super = (result_array * 255).clip(0, 255).astype("uint8").transpose((1, 2, 0))
# Resize the image with bicubic upsampling for comparison.
image_bicubic = cv2.resize(image, tuple(image_super.shape[:2][::-1]), interpolation=cv2.INTER_CUBIC)
plt.imshow(image_super)
<matplotlib.image.AxesImage at 0x7fe9b40e3580>
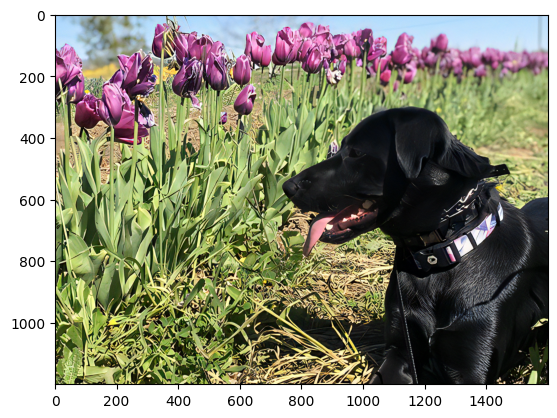
アニメーション GIF を表示¶
バイキュービック・イメージと超解像度イメージの違いを視覚化するには、両方のバージョンを切り替えるアニメーション GIF イメージを作成します。
result_pil = Image.fromarray(image_super)
bicubic_pil = Image.fromarray(image_bicubic)
gif_image_path = OUTPUT_DIR / Path(IMAGE_PATH.stem + "_comparison.gif")
final_image_path = OUTPUT_DIR / Path(IMAGE_PATH.stem + "_super.png")
result_pil.save(
fp=str(gif_image_path),
format="GIF",
append_images=[bicubic_pil],
save_all=True,
duration=1000,
loop=0,
)
result_pil.save(fp=str(final_image_path), format="png")
DisplayImage(open(gif_image_path, "rb").read(), width=1920 // 2)
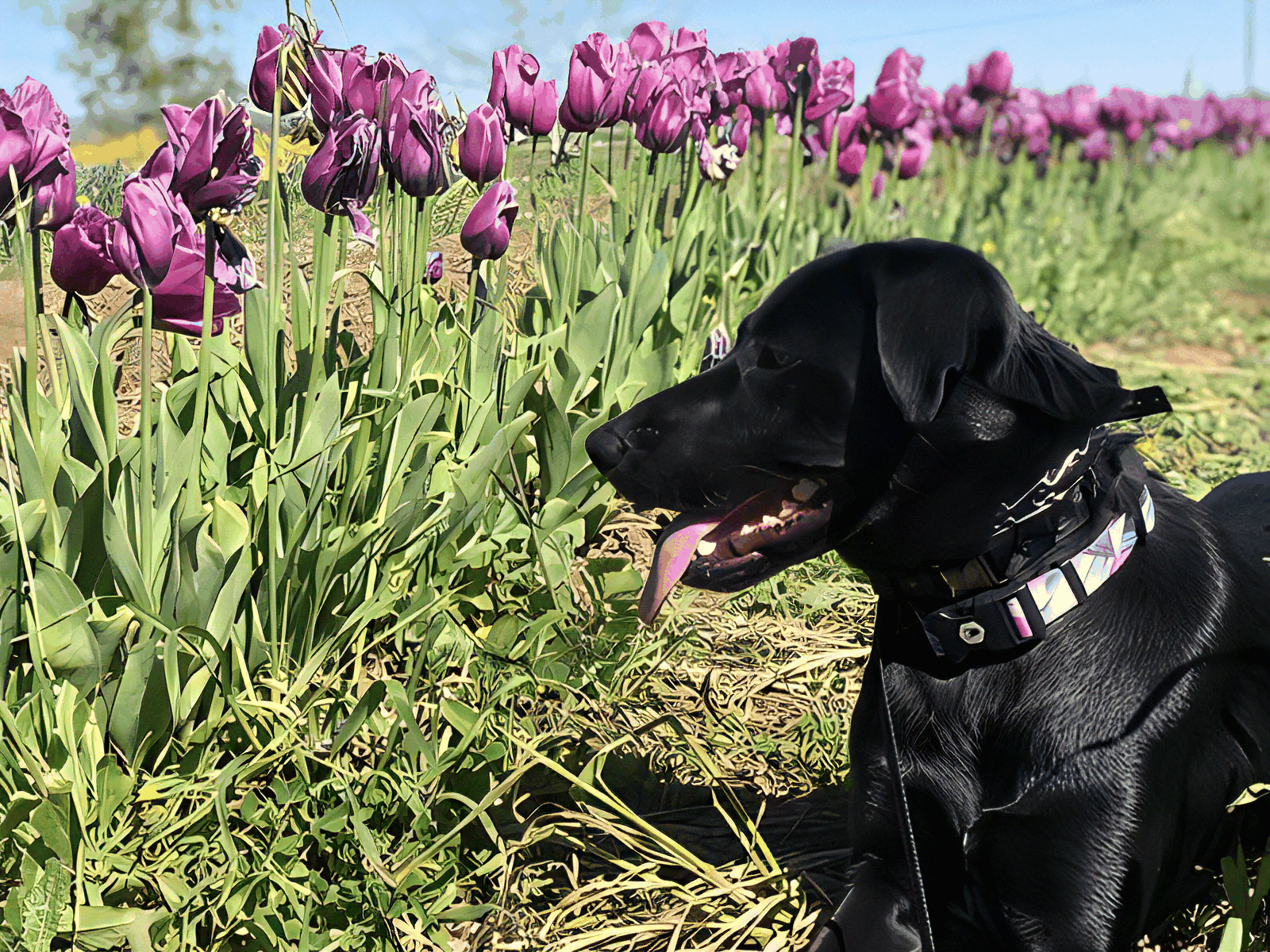
比較ビデオを作成¶
“スライダー” を使用してビデオを作成し、右側にバイキュービック画像、左側に超解像度画像を表示します。
ビデオの場合、超解像度およびバイキュービック・イメージは、処理速度を向上させるため、元の幅と高さの半分にサイズ変更されます。これは超解像効果の指標となります。ビデオは .avi
ビデオファイルとして保存されます。リンクをクリックしてビデオをダウンロードすることも、画像ディレクトリーから直接開いてローカルで再生することもできます。
FOURCC = cv2.VideoWriter_fourcc(*"MJPG")
result_video_path = OUTPUT_DIR / Path(f"{IMAGE_PATH.stem}_comparison_paddlegan.avi")
video_target_height, video_target_width = (
image_super.shape[0] // 2,
image_super.shape[1] // 2,
)
out_video = cv2.VideoWriter(
str(result_video_path),
FOURCC,
90,
(video_target_width, video_target_height),
)
resized_result_image = cv2.resize(image_super, (video_target_width, video_target_height))[
:, :, (2, 1, 0)
]
resized_bicubic_image = cv2.resize(image_bicubic, (video_target_width, video_target_height))[
:, :, (2, 1, 0)
]
progress_bar = ProgressBar(total=video_target_width)
progress_bar.display()
for i in range(2, video_target_width):
# Create a frame where the left part (until i pixels width) contains the
# superresolution image, and the right part (from i pixels width) contains
# the bicubic image.
comparison_frame = np.hstack(
(
resized_result_image[:, :i, :],
resized_bicubic_image[:, i:, :],
)
)
# Create a small black border line between the superresolution
# and bicubic part of the image.
comparison_frame[:, i - 1 : i + 1, :] = 0
out_video.write(comparison_frame)
progress_bar.progress = i
progress_bar.update()
out_video.release()
clear_output()
ビデオをダウンロード¶
下のリンクをクリックしてビデオをダウンロードするか、Google Colab を使用している場合はセルを実行してください
if 'google.colab' in str(get_ipython()):
# Save a file
from google.colab import files
# Save the file to the local file system
with open(result_video_path, 'r') as f:
files.download(result_video_path)
else:
video_link = FileLink(result_video_path)
video_link.html_link_str = "<a href='%s' download>%s</a>"
display(HTML(f"The video has been saved to {video_link._repr_html_()}"))