InstructPix2Pix と OpenVINO を使用した画像編集¶
この Jupyter ノートブックは、ローカルへのインストール後にのみ起動できます。
InstructPix2Pix は、ユーザーが提供した指示に基づいて画像を編集する条件付き拡散モデルです。従来の生成画像編集モデルは、スタイルの転送や画像ドメイン間の変換などの単一の編集タスクを対象としています。テキストガイダンスにより、単一のモデルで複数のタスクを解決する可能性があります。InstructPix2Pix メソッドは、テキストラベル、キャプション、または入力/出力画像の説明を使用する代わりに、モデルに実行するアクションを指示することで編集を可能にするという点で、既存のテキストベースの画像編集とは異なります。編集指示に従うことの利点は、ユーザーが自然な文章でモデルに正確に何をすべきかを指示できる点です。入力画像と出力画像間で一定であるサンプル画像やビジュアルコンテンツの説明などの追加情報をユーザーが提供する必要はありません。このアプローチの詳細については、この論文とリポジトリーを参照してください。
このノートブックでは、OpenVINO を使用して InstructPix2Pix モデルを変換および実行する方法を説明します。
これには次の手順が含まれます。
モデル変換 API を使用して、PyTorch モデルを OpenVINO IR 形式に変換します。
OpenVINO を使用して InstructPix2Pix パイプラインを実行します。
NNCF 量子化を使用して InstructPix2Pix パイプラインを最適化します。
元のパイプラインと最適化されたパイプラインの結果を比較します。
目次¶
必要条件¶
必要なパッケージのインストール
%pip install -q "transformers>=4.25.1" accelerate gradio datasets diffusers --extra-index-url https://download.pytorch.org/whl/cpu
%pip install -q "openvino>=2023.1.0"
Pytorch モデル・パイプラインを作成¶
StableDiffusionInstructPix2PixPipeline
は、diffusers ライブラリーの一部として提供されるわずか数行のコードでテキスト指示から画像を編集するのに使用できるエンドツーエンドの推論パイプラインです。
まず、モデルのすべてのコンポーネントの事前トレーニング済みの重みを読み込みます。
注: 最初は、重みをダウンロードするため、モデルの読み込みに時間がかかる場合があります。また、ダウンロード速度はインターネットの接続環境によって異なります。
import torch
from diffusers import StableDiffusionInstructPix2PixPipeline, EulerAncestralDiscreteScheduler
model_id = "timbrooks/instruct-pix2pix"
pipe = StableDiffusionInstructPix2PixPipeline.from_pretrained(model_id, torch_dtype=torch.float32, safety_checker=None)
scheduler_config = pipe.scheduler.config
text_encoder = pipe.text_encoder
text_encoder.eval()
unet = pipe.unet
unet.eval()
vae = pipe.vae
vae.eval()
del pipe
モデルを OpenVINO IR に変換¶
OpenVINO は、モデル変換 API を使用して PyTorch モデルをサポートし、モデルを IR 形式に変換します。 ov.convert_model
関数は、PyTorch モデル・オブジェクトとサンプル入力を受け取り、それをデバイスにロードするのにすぐに使用できる ov.Model
クラス・インスタンスに変換したり、ov.save_model
を使用してディスクに保存したりできます。
InstructPix2Pix モデルは、大規模テキストから画像への潜在拡散モデルである Stable Diffusion に基づいています。OpenVINO を使用して Stable Diffusion を実行し、テキストから画像を生成する方法の詳細については、別のチュートリアルを参照してください。
このモデルは 3 つの重要な部分で構成されています。
テキスト・エンコーダー - テキストプロンプトから条件を作成します。
Unet - 潜在画像表現のノイズを段階的に除去します。
オートエンコーダー (VAE) - ノイズ除去プロセスを開始するために初期画像を潜在空間にエンコードし、ノイズ除去が完了したら潜在空間を画像にデコードします。
各パーツを変換してみましょう。
テキスト・エンコーダー¶
テキスト・エンコーダーは、入力プロンプト (例えば、“馬に乗った宇宙飛行士の写真”) を、UNet が理解できる埋め込みスペースに変換する役割を果たします。これは通常、入力トークンのシーケンスを潜在テキスト埋め込みのシーケンスにマッピングする単純なトランスフォーマー・ベースのエンコーダーです。
テキスト・エンコーダーの入力はテンソル input_ids
です。これには、トークナイザーによって処理され、モデルによって受け入れられる最大長までパディングされたテキストからのトークン・インデックスが含まれます。モデルの出力は 2 つのテンソルです。
last_hidden_state
- モデル内の最後の MultiHeadtention レイヤーからの非表示状態pooler_out
- モデル全体の非表示状態のプールされた出力
from pathlib import Path
import openvino as ov
import gc
core = ov.Core()
TEXT_ENCODER_OV_PATH = Path("text_encoder.xml")
def cleanup_torchscript_cache():
"""
Helper for removing cached model representation
"""
torch._C._jit_clear_class_registry()
torch.jit._recursive.concrete_type_store = torch.jit._recursive.ConcreteTypeStore()
torch.jit._state._clear_class_state()
def convert_encoder(text_encoder: torch.nn.Module, ir_path:Path):
"""
Convert Text Encoder mode.
Function accepts text encoder model, and prepares example inputs for conversion,
Parameters:
text_encoder (torch.nn.Module): text_encoder model from Stable Diffusion pipeline
ir_path (Path): File for storing model
Returns:
None
"""
input_ids = torch.ones((1, 77), dtype=torch.long)
# switch model to inference mode
text_encoder.eval()
# disable gradients calculation for reducing memory consumption
with torch.no_grad():
# Export model to IR format
ov_model = ov.convert_model(text_encoder, example_input=input_ids, input=[(1,77),])
ov.save_model(ov_model, ir_path)
del ov_model
cleanup_torchscript_cache()
print(f'Text Encoder successfully converted to IR and saved to {ir_path}')
if not TEXT_ENCODER_OV_PATH.exists():
convert_encoder(text_encoder, TEXT_ENCODER_OV_PATH)
else:
print(f"Text encoder will be loaded from {TEXT_ENCODER_OV_PATH}")
del text_encoder
gc.collect()
Text encoder will be loaded from text_encoder.xml
32
VAE¶
VAE モデルは、エンコーダーとデコーダーの 2 つの部分で構成されます。
エンコーダーは、画像を低次元の潜在表現に変換するのに使用され、これが UNet モデルの入力となります。
逆に、デコーダーは潜在表現を変換して画像に戻します。
VAE がデコードにのみ使用されるテキストから画像への推論パイプラインと比較すると、パイプラインには元の画像のエンコードも含まれます。2 つの部分はパイプラインの異なるステップで個別に使用され、互いに依存しないため、2 つの独立したモデルに変換する必要があります。
VAE_ENCODER_OV_PATH = Path("vae_encoder.xml")
def convert_vae_encoder(vae: torch.nn.Module, ir_path: Path):
"""
Convert VAE model for encoding to IR format.
Function accepts vae model, creates wrapper class for export only necessary for inference part,
prepares example inputs for conversion,
Parameters:
vae (torch.nn.Module): VAE model from StableDiffusio pipeline
ir_path (Path): File for storing model
Returns:
None
"""
class VAEEncoderWrapper(torch.nn.Module):
def __init__(self, vae):
super().__init__()
self.vae = vae
def forward(self, image):
return self.vae.encode(x=image)["latent_dist"].sample()
vae_encoder = VAEEncoderWrapper(vae)
vae_encoder.eval()
image = torch.zeros((1, 3, 512, 512))
with torch.no_grad():
ov_model = ov.convert_model(vae_encoder, example_input=image, input=[((1,3,512,512),)])
ov.save_model(ov_model, ir_path)
del ov_model
cleanup_torchscript_cache()
print(f'VAE encoder successfully converted to IR and saved to {ir_path}')
if not VAE_ENCODER_OV_PATH.exists():
convert_vae_encoder(vae, VAE_ENCODER_OV_PATH)
else:
print(f"VAE encoder will be loaded from {VAE_ENCODER_OV_PATH}")
VAE_DECODER_OV_PATH = Path('vae_decoder.xml')
def convert_vae_decoder(vae: torch.nn.Module, ir_path: Path):
"""
Convert VAE model for decoding to IR format.
Function accepts vae model, creates wrapper class for export only necessary for inference part,
prepares example inputs for conversion,
Parameters:
vae (torch.nn.Module): VAE model frm StableDiffusion pipeline
ir_path (Path): File for storing model
Returns:
None
"""
class VAEDecoderWrapper(torch.nn.Module):
def __init__(self, vae):
super().__init__()
self.vae = vae
def forward(self, latents):
return self.vae.decode(latents)
vae_decoder = VAEDecoderWrapper(vae)
latents = torch.zeros((1, 4, 64, 64))
vae_decoder.eval()
with torch.no_grad():
ov_model = ov.convert_model(vae_decoder, example_input=latents, input=[((1,4,64,64),)])
ov.save_model(ov_model, ir_path)
del ov_model
cleanup_torchscript_cache()
print(f'VAE decoder successfully converted to IR and saved to {ir_path}')
if not VAE_DECODER_OV_PATH.exists():
convert_vae_decoder(vae, VAE_DECODER_OV_PATH)
else:
print(f"VAE decoder will be loaded from {VAE_DECODER_OV_PATH}")
del vae
gc.collect()
VAE encoder will be loaded from vae_encoder.xml
VAE decoder will be loaded from vae_decoder.xml
0
Unet¶
Unet モデルには 3 つの入力があります。
scaled_latent_model_input
- 前のステップからの潜在画像サンプル。生成プロセスはまだ開始されていないため、ランダムノイズを使用します。timestep
- 現在のスケジューラー・ステップ。text_embeddings
- テキスト・エンコーダーの非表示状態。
モデルは次のステップのサンプルの状態を予測します。
import numpy as np
UNET_OV_PATH = Path("unet.xml")
dtype_mapping = {
torch.float32: ov.Type.f32,
torch.float64: ov.Type.f64
}
def convert_unet(unet:torch.nn.Module, ir_path:Path):
"""
Convert U-net model to IR format.
Function accepts unet model, prepares example inputs for conversion,
Parameters:
unet (StableDiffusionPipeline): unet from Stable Diffusion pipeline
ir_path (Path): File for storing model
Returns:
None
"""
# prepare inputs
encoder_hidden_state = torch.ones((3, 77, 768))
latents_shape = (3, 8, 512 // 8, 512 // 8)
latents = torch.randn(latents_shape)
t = torch.from_numpy(np.array(1, dtype=float))
dummy_inputs = (latents, t, encoder_hidden_state)
input_info = []
for input_tensor in dummy_inputs:
shape = ov.PartialShape(tuple(input_tensor.shape))
element_type = dtype_mapping[input_tensor.dtype]
input_info.append((shape, element_type))
unet.eval()
with torch.no_grad():
ov_model = ov.convert_model(unet, example_input=dummy_inputs, input=input_info)
ov.save_model(ov_model, ir_path)
del ov_model
cleanup_torchscript_cache()
print(f'Unet successfully converted to IR and saved to {ir_path}')
if not UNET_OV_PATH.exists():
convert_unet(unet, UNET_OV_PATH)
gc.collect()
else:
print(f"Unet will be loaded from {UNET_OV_PATH}")
del unet
gc.collect()
Unet will be loaded from unet.xml
0
推論パイプラインの準備¶
すべてをまとめて論理フローを図解することで、モデル推論がどのように機能するかを詳しく見てみましょう。
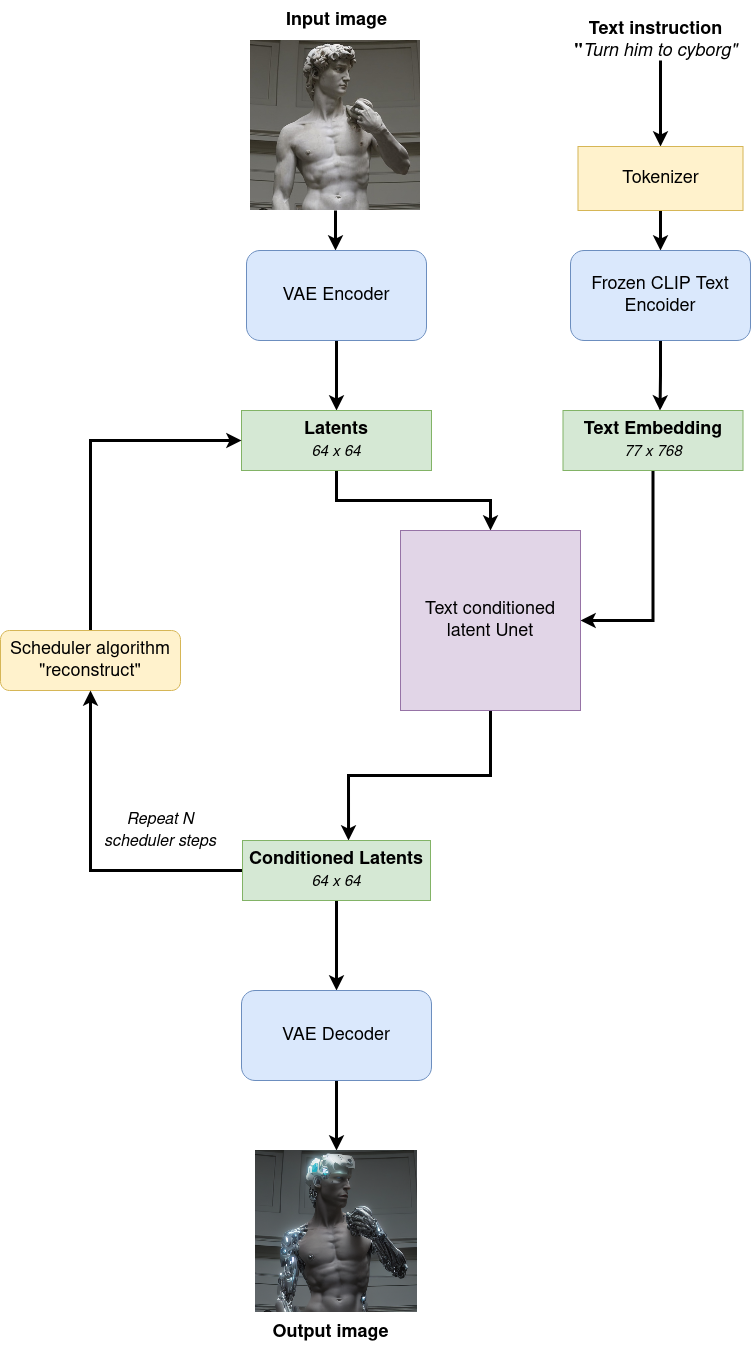
diagram¶
InstructPix2Pix モデルは、画像とテキストプロンプトの両方を入力として受け取ります。画像は変分オートエンコーダーのエンコーダ部分を使用してサイズ \(64 \times 64\) の潜在画像表現に変換され、テキストプロンプトは CLIP のテキストエンコーダを介してサイズ \(77 \times 768\) のテキスト埋め込みに変換されます。
次に、UNet モデルは、テキスト埋め込みを条件として、ランダムな潜在画像表現を繰り返しノイズ除去します。UNet の出力はノイズ残差であり、スケジューラー・アルゴリズムを介してノイズ除去された潜在画像表現を計算するために使用されます。
ノイズ除去プロセスは、指定された回数 (デフォルトでは 100 回) 繰り返され、段階的に潜在画像表現の改善が図られます。完了すると、潜在画像表現は変分オートエンコーダーのデコーダー部によってデコードされます。
from diffusers import DiffusionPipeline
from transformers import CLIPTokenizer
from typing import Union, List, Optional, Tuple
import PIL
import cv2
def scale_fit_to_window(dst_width:int, dst_height:int, image_width:int, image_height:int):
"""
Preprocessing helper function for calculating image size for resize with peserving original aspect ratio
and fitting image to specific window size
Parameters:
dst_width (int): destination window width
dst_height (int): destination window height
image_width (int): source image width
image_height (int): source image height
Returns:
result_width (int): calculated width for resize
result_height (int): calculated height for resize
"""
im_scale = min(dst_height / image_height, dst_width / image_width)
return int(im_scale * image_width), int(im_scale * image_height)
def preprocess(image: PIL.Image.Image):
"""
Image preprocessing function. Takes image in PIL.Image format, resizes it to keep aspect ration and fits to model input window 512x512,
then converts it to np.ndarray and adds padding with zeros on right or bottom side of image (depends from aspect ratio), after that
converts data to float32 data type and change range of values from [0, 255] to [-1, 1], finally, converts data layout from planar NHWC to NCHW.
The function returns preprocessed input tensor and padding size, which can be used in postprocessing.
Parameters:
image (PIL.Image.Image): input image
Returns:
image (np.ndarray): preprocessed image tensor
pad (Tuple[int]): pading size for each dimension for restoring image size in postprocessing
"""
src_width, src_height = image.size
dst_width, dst_height = scale_fit_to_window(
512, 512, src_width, src_height)
image = np.array(image.resize((dst_width, dst_height),
resample=PIL.Image.Resampling.LANCZOS))[None, :]
pad_width = 512 - dst_width
pad_height = 512 - dst_height
pad = ((0, 0), (0, pad_height), (0, pad_width), (0, 0))
image = np.pad(image, pad, mode="constant")
image = image.astype(np.float32) / 255.0
image = 2.0 * image - 1.0
image = image.transpose(0, 3, 1, 2)
return image, pad
def randn_tensor(
shape: Union[Tuple, List],
dtype: Optional[np.dtype] = np.float32,
):
"""
Helper function for generation random values tensor with given shape and data type
Parameters:
shape (Union[Tuple, List]): shape for filling random values
dtype (np.dtype, *optiona*, np.float32): data type for result
Returns:
latents (np.ndarray): tensor with random values with given data type and shape (usually represents noise in latent space)
"""
latents = np.random.randn(*shape).astype(dtype)
return latents
class OVInstructPix2PixPipeline(DiffusionPipeline):
"""
OpenVINO inference pipeline for InstructPix2Pix
"""
def __init__(
self,
tokenizer: CLIPTokenizer,
scheduler: EulerAncestralDiscreteScheduler,
core: ov.Core,
text_encoder: ov.Model,
vae_encoder: ov.Model,
unet: ov.Model,
vae_decoder: ov.Model,
device: str = "AUTO"
):
super().__init__()
self.tokenizer = tokenizer
self.vae_scale_factor = 8
self.scheduler = scheduler
self.load_models(core, device, text_encoder,
vae_encoder, unet, vae_decoder)
def load_models(self, core: ov.Core, device: str, text_encoder: ov.Model, vae_encoder: ov.Model, unet: ov.Model, vae_decoder: ov.Model):
"""
Function for loading models on device using OpenVINO
Parameters:
core (Core): OpenVINO runtime Core class instance
device (str): inference device
text_encoder (Model): OpenVINO Model object represents text encoder
vae_encoder (Model): OpenVINO Model object represents vae encoder
unet (Model): OpenVINO Model object represents unet
vae_decoder (Model): OpenVINO Model object represents vae decoder
Returns
None
"""
self.text_encoder = core.compile_model(text_encoder, device)
self.text_encoder_out = self.text_encoder.output(0)
ov_config = {"INFERENCE_PRECISION_HINT": "f32"} if device != "CPU" else {}
self.vae_encoder = core.compile_model(vae_encoder, device, ov_config)
self.vae_encoder_out = self.vae_encoder.output(0)
# We have to register UNet in config to be able to change it externally to collect calibration data
self.register_to_config(unet=core.compile_model(unet, device))
self.unet_out = self.unet.output(0)
self.vae_decoder = core.compile_model(vae_decoder, device, ov_config)
self.vae_decoder_out = self.vae_decoder.output(0)
def __call__(
self,
prompt: Union[str, List[str]],
image: PIL.Image.Image,
num_inference_steps: int = 10,
guidance_scale: float = 7.5,
image_guidance_scale: float = 1.5,
eta: float = 0.0,
latents: Optional[np.array] = None,
output_type: Optional[str] = "pil",
):
"""
Function invoked when calling the pipeline for generation.
Parameters:
prompt (`str` or `List[str]`):
The prompt or prompts to guide the image generation.
image (`PIL.Image.Image`):
`Image`, or tensor representing an image batch which will be repainted according to `prompt`.
num_inference_steps (`int`, *optional*, defaults to 100):
The number of denoising steps. More denoising steps usually lead to a higher quality image at the
expense of slower inference.
guidance_scale (`float`, *optional*, defaults to 7.5):
Guidance scale as defined in [Classifier-Free Diffusion Guidance](https://arxiv.org/abs/2207.12598).
`guidance_scale` is defined as `w` of equation 2. of [Imagen
Paper](https://arxiv.org/pdf/2205.11487.pdf). Guidance scale is enabled by setting `guidance_scale >
1`. Higher guidance scale encourages to generate images that are closely linked to the text `prompt`,
usually at the expense of lower image quality. This pipeline requires a value of at least `1`.
image_guidance_scale (`float`, *optional*, defaults to 1.5):
Image guidance scale is to push the generated image towards the inital image `image`. Image guidance
scale is enabled by setting `image_guidance_scale > 1`. Higher image guidance scale encourages to
generate images that are closely linked to the source image `image`, usually at the expense of lower
image quality. This pipeline requires a value of at least `1`.
latents (`torch.FloatTensor`, *optional*):
Pre-generated noisy latents, sampled from a Gaussian distribution, to be used as inputs for image
generation. Can be used to tweak the same generation with different prompts. If not provided, a latents
tensor will ge generated by sampling using the supplied random `generator`.
output_type (`str`, *optional*, defaults to `"pil"`):
The output format of the generate image. Choose between
[PIL](https://pillow.readthedocs.io/en/stable/): `PIL.Image.Image` or `np.array`.
Returns:
image ([List[Union[np.ndarray, PIL.Image.Image]]): generaited images
"""
# 1. Define call parameters
batch_size = 1 if isinstance(prompt, str) else len(prompt)
# here `guidance_scale` is defined analog to the guidance weight `w` of equation (2)
# of the Imagen paper: https://arxiv.org/pdf/2205.11487.pdf . `guidance_scale = 1`
# corresponds to doing no classifier free guidance.
do_classifier_free_guidance = guidance_scale > 1.0 and image_guidance_scale >= 1.0
# check if scheduler is in sigmas space
scheduler_is_in_sigma_space = hasattr(self.scheduler, "sigmas")
# 2. Encode input prompt
text_embeddings = self._encode_prompt(prompt)
# 3. Preprocess image
orig_width, orig_height = image.size
image, pad = preprocess(image)
height, width = image.shape[-2:]
# 4. set timesteps
self.scheduler.set_timesteps(num_inference_steps)
timesteps = self.scheduler.timesteps
# 5. Prepare Image latents
image_latents = self.prepare_image_latents(
image,
do_classifier_free_guidance=do_classifier_free_guidance,
)
# 6. Prepare latent variables
num_channels_latents = 4
latents = self.prepare_latents(
batch_size,
num_channels_latents,
height,
width,
text_embeddings.dtype,
latents,
)
# 7. Denoising loop
num_warmup_steps = len(timesteps) - num_inference_steps * self.scheduler.order
with self.progress_bar(total=num_inference_steps) as progress_bar:
for i, t in enumerate(timesteps):
# Expand the latents if we are doing classifier free guidance.
# The latents are expanded 3 times because for pix2pix the guidance\
# is applied for both the text and the input image.
latent_model_input = np.concatenate(
[latents] * 3) if do_classifier_free_guidance else latents
# concat latents, image_latents in the channel dimension
scaled_latent_model_input = self.scheduler.scale_model_input(
latent_model_input, t)
scaled_latent_model_input = np.concatenate(
[scaled_latent_model_input, image_latents], axis=1)
# predict the noise residual
noise_pred = self.unet([scaled_latent_model_input, t, text_embeddings])[
self.unet_out]
# Hack:
# For karras style schedulers the model does classifier free guidance using the
# predicted_original_sample instead of the noise_pred. So we need to compute the
# predicted_original_sample here if we are using a karras style scheduler.
if scheduler_is_in_sigma_space:
step_index = (self.scheduler.timesteps == t).nonzero().item()
sigma = self.scheduler.sigmas[step_index].numpy()
noise_pred = latent_model_input - sigma * noise_pred
# perform guidance
if do_classifier_free_guidance:
noise_pred_text, noise_pred_image, noise_pred_uncond = noise_pred[
0], noise_pred[1], noise_pred[2]
noise_pred = (
noise_pred_uncond + guidance_scale * (noise_pred_text - noise_pred_image) + image_guidance_scale * (noise_pred_image - noise_pred_uncond)
)
# For karras style schedulers the model does classifier free guidance using the
# predicted_original_sample instead of the noise_pred. But the scheduler.step function
# expects the noise_pred and computes the predicted_original_sample internally. So we
# need to overwrite the noise_pred here such that the value of the computed
# predicted_original_sample is correct.
if scheduler_is_in_sigma_space:
noise_pred = (noise_pred - latents) / (-sigma)
# compute the previous noisy sample x_t -> x_t-1
latents = self.scheduler.step(torch.from_numpy(noise_pred), t, torch.from_numpy(latents)).prev_sample.numpy()
# call the callback, if provided
if i == len(timesteps) - 1 or ((i + 1) > num_warmup_steps and (i + 1) % self.scheduler.order == 0):
progress_bar.update()
# 8. Post-processing
image = self.decode_latents(latents, pad)
# 9. Convert to PIL
if output_type == "pil":
image = self.numpy_to_pil(image)
image = [img.resize((orig_width, orig_height),
PIL.Image.Resampling.LANCZOS) for img in image]
else:
image = [cv2.resize(img, (orig_width, orig_width))
for img in image]
return image
def _encode_prompt(self, prompt:Union[str, List[str]], num_images_per_prompt:int = 1, do_classifier_free_guidance:bool = True):
"""
Encodes the prompt into text encoder hidden states.
Parameters:
prompt (str or list(str)): prompt to be encoded
num_images_per_prompt (int): number of images that should be generated per prompt
do_classifier_free_guidance (bool): whether to use classifier free guidance or not
Returns:
text_embeddings (np.ndarray): text encoder hidden states
"""
batch_size = len(prompt) if isinstance(prompt, list) else 1
# tokenize input prompts
text_inputs = self.tokenizer(
prompt,
padding="max_length",
max_length=self.tokenizer.model_max_length,
truncation=True,
return_tensors="np",
)
text_input_ids = text_inputs.input_ids
text_embeddings = self.text_encoder(
text_input_ids)[self.text_encoder_out]
# duplicate text embeddings for each generation per prompt, using mps friendly method
if num_images_per_prompt != 1:
bs_embed, seq_len, _ = text_embeddings.shape
text_embeddings = np.tile(
text_embeddings, (1, num_images_per_prompt, 1))
text_embeddings = np.reshape(
text_embeddings, (bs_embed * num_images_per_prompt, seq_len, -1))
# get unconditional embeddings for classifier free guidance
if do_classifier_free_guidance:
uncond_tokens: List[str]
uncond_tokens = [""] * batch_size
max_length = text_input_ids.shape[-1]
uncond_input = self.tokenizer(
uncond_tokens,
padding="max_length",
max_length=max_length,
truncation=True,
return_tensors="np",
)
uncond_embeddings = self.text_encoder(uncond_input.input_ids)[
self.text_encoder_out]
# duplicate unconditional embeddings for each generation per prompt, using mps friendly method
seq_len = uncond_embeddings.shape[1]
uncond_embeddings = np.tile(
uncond_embeddings, (1, num_images_per_prompt, 1))
uncond_embeddings = np.reshape(
uncond_embeddings, (batch_size * num_images_per_prompt, seq_len, -1))
# For classifier free guidance, you need to do two forward passes.
# Here, you concatenate the unconditional and text embeddings into a single batch
# to avoid doing two forward passes
text_embeddings = np.concatenate(
[text_embeddings, uncond_embeddings, uncond_embeddings])
return text_embeddings
def prepare_image_latents(
self, image, batch_size=1, num_images_per_prompt=1, do_classifier_free_guidance=True
):
"""
Encodes input image to latent space using VAE Encoder
Parameters:
image (np.ndarray): input image tensor
num_image_per_prompt (int, *optional*, 1): number of image generated for promt
do_classifier_free_guidance (bool): whether to use classifier free guidance or not
Returns:
image_latents: image encoded to latent space
"""
image = image.astype(np.float32)
batch_size = batch_size * num_images_per_prompt
image_latents = self.vae_encoder(image)[self.vae_encoder_out]
if batch_size > image_latents.shape[0] and batch_size % image_latents.shape[0] == 0:
# expand image_latents for batch_size
additional_image_per_prompt = batch_size // image_latents.shape[0]
image_latents = np.concatenate(
[image_latents] * additional_image_per_prompt, axis=0)
elif batch_size > image_latents.shape[0] and batch_size % image_latents.shape[0] != 0:
raise ValueError(
f"Cannot duplicate `image` of batch size {image_latents.shape[0]} to {batch_size} text prompts."
)
else:
image_latents = np.concatenate([image_latents], axis=0)
if do_classifier_free_guidance:
uncond_image_latents = np.zeros_like(image_latents)
image_latents = np.concatenate([image_latents, image_latents, uncond_image_latents], axis=0)
return image_latents
def prepare_latents(self, batch_size:int, num_channels_latents:int, height:int, width:int, dtype:np.dtype = np.float32, latents:np.ndarray = None):
"""
Preparing noise to image generation. If initial latents are not provided, they will be generated randomly,
then prepared latents scaled by the standard deviation required by the scheduler
Parameters:
batch_size (int): input batch size
num_channels_latents (int): number of channels for noise generation
height (int): image height
width (int): image width
dtype (np.dtype, *optional*, np.float32): dtype for latents generation
latents (np.ndarray, *optional*, None): initial latent noise tensor, if not provided will be generated
Returns:
latents (np.ndarray): scaled initial noise for diffusion
"""
shape = (batch_size, num_channels_latents, height // self.vae_scale_factor, width // self.vae_scale_factor)
if latents is None:
latents = randn_tensor(shape, dtype=dtype)
else:
latents = latents
# scale the initial noise by the standard deviation required by the scheduler
latents = latents * self.scheduler.init_noise_sigma.numpy()
return latents
def decode_latents(self, latents:np.array, pad:Tuple[int]):
"""
Decode predicted image from latent space using VAE Decoder and unpad image result
Parameters:
latents (np.ndarray): image encoded in diffusion latent space
pad (Tuple[int]): each side padding sizes obtained on preprocessing step
Returns:
image: decoded by VAE decoder image
"""
latents = 1 / 0.18215 * latents
image = self.vae_decoder(latents)[self.vae_decoder_out]
(_, end_h), (_, end_w) = pad[1:3]
h, w = image.shape[2:]
unpad_h = h - end_h
unpad_w = w - end_w
image = image[:, :, :unpad_h, :unpad_w]
image = np.clip(image / 2 + 0.5, 0, 1)
image = np.transpose(image, (0, 2, 3, 1))
return image
import matplotlib.pyplot as plt
def visualize_results(orig_img:PIL.Image.Image, processed_img:PIL.Image.Image, img1_title:str, img2_title:str):
"""
Helper function for results visualization
Parameters:
orig_img (PIL.Image.Image): original image
processed_img (PIL.Image.Image): processed image after editing
img1_title (str): title for the image on the left
img2_title (str): title for the image on the right
Returns:
fig (matplotlib.pyplot.Figure): matplotlib generated figure contains drawing result
"""
im_w, im_h = orig_img.size
is_horizontal = im_h <= im_w
figsize = (20, 30) if is_horizontal else (30, 20)
fig, axs = plt.subplots(1 if is_horizontal else 2, 2 if is_horizontal else 1, figsize=figsize, sharex='all', sharey='all')
fig.patch.set_facecolor('white')
list_axes = list(axs.flat)
for a in list_axes:
a.set_xticklabels([])
a.set_yticklabels([])
a.get_xaxis().set_visible(False)
a.get_yaxis().set_visible(False)
a.grid(False)
list_axes[0].imshow(np.array(orig_img))
list_axes[1].imshow(np.array(processed_img))
list_axes[0].set_title(img1_title, fontsize=20)
list_axes[1].set_title(img2_title, fontsize=20)
fig.subplots_adjust(wspace=0.0 if is_horizontal else 0.01 , hspace=0.01 if is_horizontal else 0.0)
fig.tight_layout()
fig.savefig("result.png", bbox_inches='tight')
return fig
モデル・トークナイザーとスケジューラーもパイプラインの重要なパーツです。それらを定義して、すべてのコンポーネントをまとめてみましょう。さらに、ドロップダウン・リストからデバイスを選択して提供することもできます。
import ipywidgets as widgets
device = widgets.Dropdown(
options=core.available_devices + ["AUTO"],
value='AUTO',
description='Device:',
disabled=False,
)
device
Dropdown(description='Device:', index=1, options=('CPU', 'AUTO'), value='AUTO')
from transformers import CLIPTokenizer
tokenizer = CLIPTokenizer.from_pretrained('openai/clip-vit-large-patch14')
scheduler = EulerAncestralDiscreteScheduler.from_config(scheduler_config)
ov_pipe = OVInstructPix2PixPipeline(tokenizer, scheduler, core, TEXT_ENCODER_OV_PATH, VAE_ENCODER_OV_PATH, UNET_OV_PATH, VAE_DECODER_OV_PATH, device=device.value)
/home/ltalamanova/env_ci/lib/python3.8/site-packages/diffusers/configuration_utils.py:134: FutureWarning: Accessing config attribute unet directly via 'OVInstructPix2PixPipeline' object attribute is deprecated. Please access 'unet' over 'OVInstructPix2PixPipeline's config object instead, e.g. 'scheduler.config.unet'. deprecate("direct config name access", "1.0.0", deprecation_message, standard_warn=False)
これで、推論パイプラインを実行する編集手順とイメージを定義する準備が整いました。インスピレーションが必要な場合、このページでモデルによって生成されたサンプル結果を確認できます。オプションで、潜在状態の初期化とステップ数に対するランダム・ジェネレーターのシード値を変更することもできます。
注: より正確な結果を得るため、ステップを増やすことを検討してください。推奨値は
100
ですが、処理に時間がかかります。
style = {'description_width': 'initial'}
text_prompt = widgets.Text(value=" Make it in galaxy", description='your text')
num_steps = widgets.IntSlider(min=1, max=100, value=10, description='steps:')
seed = widgets.IntSlider(min=0, max=1024, description='seed: ', value=42)
image_widget = widgets.FileUpload(
accept='',
multiple=False,
description='Upload image',
style=style
)
widgets.VBox([text_prompt, seed, num_steps, image_widget])
VBox(children=(Text(value=' Make it in galaxy', description='your text'), IntSlider(value=42, description='see… NOTE: Diffusion process can take some time, depending on what hardware you select.
import io
import requests
default_url = "https://user-images.githubusercontent.com/29454499/223343459-4ac944f0-502e-4acf-9813-8e9f0abc8a16.jpg"
# read uploaded image
image = PIL.Image.open(io.BytesIO(image_widget.value[-1]['content']) if image_widget.value else requests.get(default_url, stream=True).raw)
image = image.convert("RGB")
print('Pipeline settings')
print(f'Input text: {text_prompt.value}')
print(f'Seed: {seed.value}')
print(f'Number of steps: {num_steps.value}')
np.random.seed(seed.value)
processed_image = ov_pipe(text_prompt.value, image, num_steps.value)
Pipeline settings
Input text: Make it in galaxy
Seed: 42
Number of steps: 10
0%| | 0/10 [00:00<?, ?it/s]
それでは、結果を見てみましょう。上の画像は編集前のオリジナルです。下の画像は編集プロセスの結果です。タイトルの間には、生成に使用されるテキスト指示が含まれています。
fig = visualize_results(image, processed_image[0], img1_title="Original image", img2_title=f"Prompt: {text_prompt.value}")
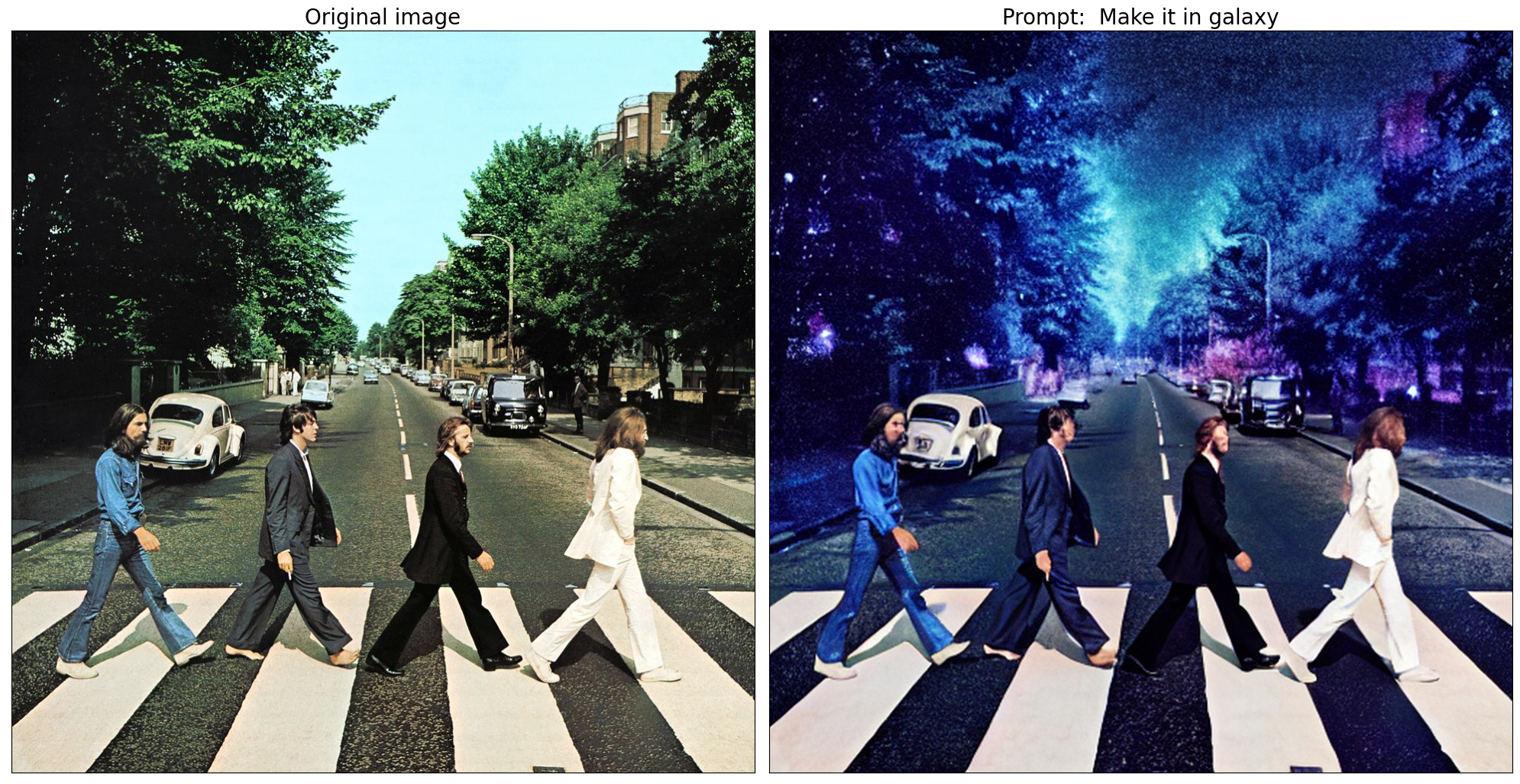
ご覧の通り、画像はかなり高解像度です 🔥。
量子化¶
NNCF は、量子化レイヤーをモデルグラフに追加し、トレーニング・データセットのサブセットを使用してこれらの追加の量子化レイヤーのパラメーターを初期化することで、トレーニング後の量子化を可能にします。量子化操作は FP32
/FP16
ではなく INT8
で実行されるため、モデル推論が高速化されます。
InstructPix2Pix
パイプライン構造に従って、入力の反復的なノイズ除去に UNet が使用されます。これは、モデルが各拡散ステップで推論を繰り返すサイクルで実行され、パイプラインの他の部分は 1 回だけ実行されることを意味します。そのため、UNet ノイズ除去の計算コストと速度がパイプラインのクリティカル・パスになります。
最適化プロセスには次の手順が含まれます。
量子化用のキャリブレーション・データセットを作成します。
nncf.quantize()
を実行して、量子化されたモデルを取得します。openvino.save_model()
関数を使用してINT8
モデルを保存します。
モデルの推論速度を向上させるため量子化を実行するかどうかを以下で選択してください。
to_quantize = widgets.Checkbox(
value=True,
description='Quantization',
disabled=False,
)
to_quantize
Checkbox(value=True, description='Quantization')
to_quantize
が選択されていない場合に量子化をスキップする skip magic
拡張機能をロードします。
import sys
sys.path.append("../utils")
%load_ext skip_kernel_extension
キャリブレーション・データセットの準備¶
Hugging Face の fusing/instructpix2pix-1000-samples データセットの一部をキャリブレーション・データとして使用します。キャリブレーション用の中間モデル入力を収集するには、CompiledModel
をカスタマイズする必要があります。
%%skip not $to_quantize.value
import datasets
from tqdm.notebook import tqdm
from transformers import Pipeline
from typing import Any, Dict, List
class CompiledModelDecorator(ov.CompiledModel):
def __init__(self, compiled_model, prob: float, data_cache: List[Any] = None):
super().__init__(compiled_model)
self.data_cache = data_cache if data_cache else []
self.prob = np.clip(prob, 0, 1)
def __call__(self, *args, **kwargs):
if np.random.rand() >= self.prob:
self.data_cache.append(*args)
return super().__call__(*args, **kwargs)
def collect_calibration_data(pix2pix_pipeline: Pipeline, subset_size: int) -> List[Dict]:
original_unet = pix2pix_pipeline.unet
pix2pix_pipeline.unet = CompiledModelDecorator(original_unet, prob=0.3)
dataset = datasets.load_dataset("fusing/instructpix2pix-1000-samples", split="train", streaming=True).shuffle(seed=42)
pix2pix_pipeline.set_progress_bar_config(disable=True)
# Run inference for data collection
pbar = tqdm(total=subset_size)
diff = 0
for batch in dataset:
prompt = batch["edit_prompt"]
image = batch["input_image"].convert("RGB")
_ = pix2pix_pipeline(prompt, image)
collected_subset_size = len(pix2pix_pipeline.unet.data_cache)
if collected_subset_size >= subset_size:
pbar.update(subset_size - pbar.n)
break
pbar.update(collected_subset_size - diff)
diff = collected_subset_size
calibration_dataset = pix2pix_pipeline.unet.data_cache
pix2pix_pipeline.set_progress_bar_config(disable=False)
pix2pix_pipeline.unet = original_unet
return calibration_dataset
%%skip not $to_quantize.value
UNET_INT8_OV_PATH = Path("unet_int8.xml")
if not UNET_INT8_OV_PATH.exists():
subset_size = 300
unet_calibration_data = collect_calibration_data(ov_pipe, subset_size=subset_size)
/home/ltalamanova/env_ci/lib/python3.8/site-packages/diffusers/configuration_utils.py:134: FutureWarning: Accessing config attribute unet directly via 'OVInstructPix2PixPipeline' object attribute is deprecated. Please access 'unet' over 'OVInstructPix2PixPipeline's config object instead, e.g. 'scheduler.config.unet'. deprecate("direct config name access", "1.0.0", deprecation_message, standard_warn=False)
0%| | 0/300 [00:00<?, ?it/s]
量子化を実行¶
事前トレーニング済みの変換済み OpenVINO モデルから量子化モデルを作成します。
注: 量子化は時間とメモリーを消費する操作です。以下の量子化コードの実行には時間がかかる場合があります。
%%skip not $to_quantize.value
import nncf
if UNET_INT8_OV_PATH.exists():
print("Loading quantized model")
quantized_unet = core.read_model(UNET_INT8_OV_PATH)
else:
unet = core.read_model(UNET_OV_PATH)
quantized_unet = nncf.quantize(
model=unet,
subset_size=subset_size,
calibration_dataset=nncf.Dataset(unet_calibration_data),
model_type=nncf.ModelType.TRANSFORMER
)
ov.save_model(quantized_unet, UNET_INT8_OV_PATH)
INFO:nncf:NNCF initialized successfully. Supported frameworks detected: torch, tensorflow, onnx, openvino
Statistics collection: 100%|██████████| 300/300 [06:48<00:00, 1.36s/it]
Applying Smooth Quant: 100%|██████████| 100/100 [00:07<00:00, 13.51it/s]
INFO:nncf:96 ignored nodes was found by name in the NNCFGraph
Statistics collection: 100%|██████████| 300/300 [14:34<00:00, 2.91s/it]
Applying Fast Bias correction: 100%|██████████| 186/186 [05:31<00:00, 1.78s/it]
同じ入力データを使用して、量子化された UNet で予測を確認してみましょう。
%%skip not $to_quantize.value
print('Pipeline settings')
print(f'Input text: {text_prompt.value}')
print(f'Seed: {seed.value}')
print(f'Number of steps: {num_steps.value}')
np.random.seed(seed.value)
int8_pipe = OVInstructPix2PixPipeline(tokenizer, scheduler, core, TEXT_ENCODER_OV_PATH, VAE_ENCODER_OV_PATH, UNET_INT8_OV_PATH, VAE_DECODER_OV_PATH, device=device.value)
int8_processed_image = int8_pipe(text_prompt.value, image, num_steps.value)
fig = visualize_results(processed_image[0], int8_processed_image[0], img1_title="FP16 result", img2_title="INT8 result")
Pipeline settings
Input text: Make it in galaxy
Seed: 42
Number of steps: 10
0%| | 0/10 [00:00<?, ?it/s]
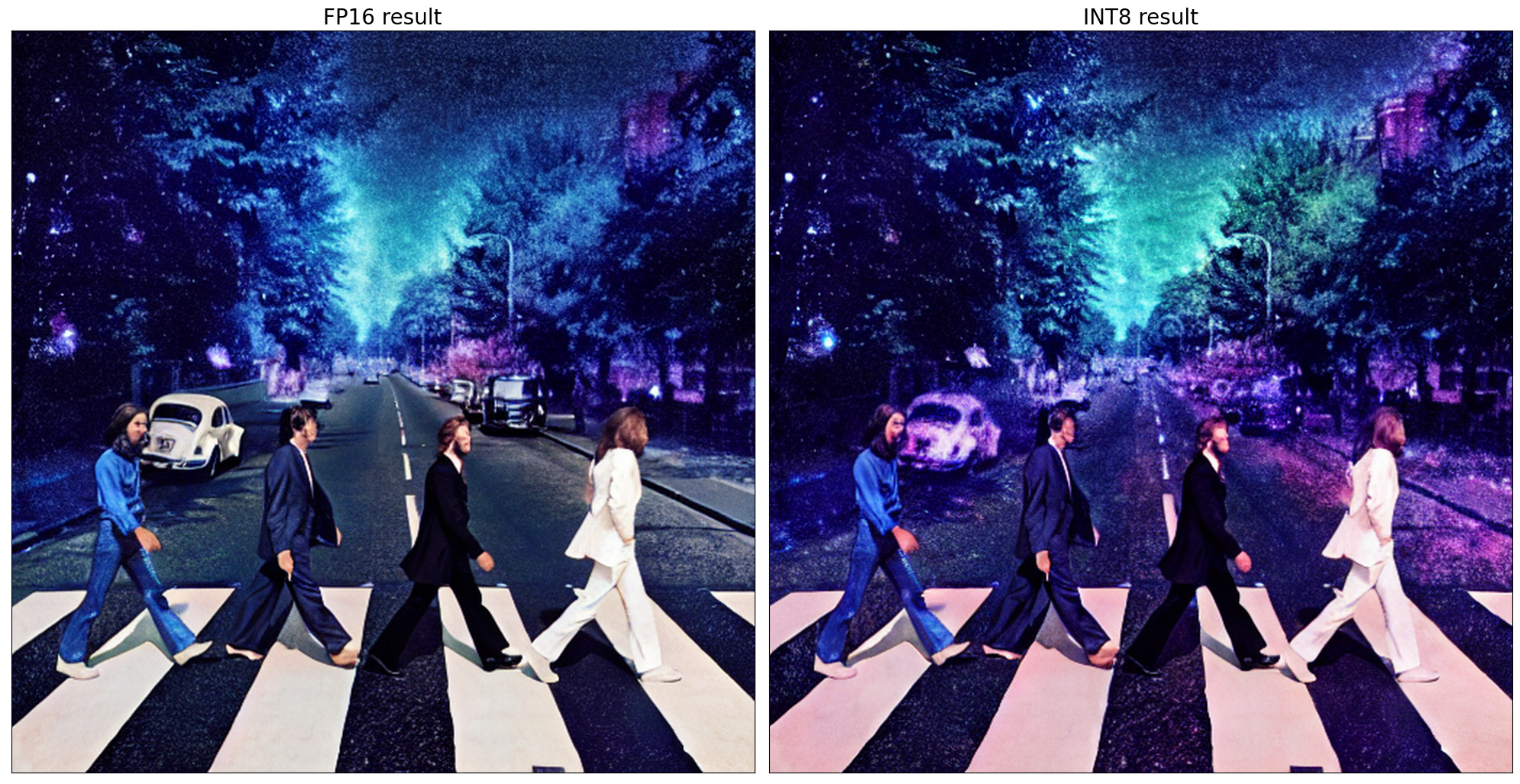
FP16 モデルと INT8 モデルの推論時間を比較¶
FP16
と INT8
モデルの推論パフォーマンスを測定するには、キャリブレーション・サブセットの推論時間の中央値を使用します。
注: 最も正確なパフォーマンス推定を行うには、他のアプリケーションを閉じた後、ターミナル/コマンドプロンプトで
benchmark_app
を実行することを推奨します。
%%skip not $to_quantize.value
import time
calibration_dataset = datasets.load_dataset("fusing/instructpix2pix-1000-samples", split="train", streaming=True)
validation_data = []
validation_size = 10
while len(validation_data) < validation_size:
batch = next(iter(calibration_dataset))
prompt = batch["edit_prompt"]
input_image = batch["input_image"].convert("RGB")
validation_data.append((prompt, input_image))
def calculate_inference_time(pix2pix_pipeline, calibration_dataset, size=10):
inference_time = []
pix2pix_pipeline.set_progress_bar_config(disable=True)
for (prompt, image) in calibration_dataset:
start = time.perf_counter()
_ = pix2pix_pipeline(prompt, image)
end = time.perf_counter()
delta = end - start
inference_time.append(delta)
return np.median(inference_time)
%%skip not $to_quantize.value
fp_latency = calculate_inference_time(ov_pipe, validation_data)
int8_latency = calculate_inference_time(int8_pipe, validation_data)
print(f"Performance speed up: {fp_latency / int8_latency:.3f}")
Performance speed up: 1.437
Gradio によるインタラクティブなデモ¶
注: 選択したハードウェアによっては、拡散プロセスに時間がかかる場合があります。
pipe_precision = widgets.Dropdown(
options=["FP16"] if not to_quantize.value else ["FP16", "INT8"],
value="FP16",
description='Precision:',
disabled=False,
)
pipe_precision
Dropdown(description='Precision:', options=('FP16', 'INT8'), value='FP16')
import gradio as gr
from urllib.request import urlretrieve
from pathlib import Path
import numpy as np
default_url = "https://user-images.githubusercontent.com/29454499/223343459-4ac944f0-502e-4acf-9813-8e9f0abc8a16.jpg"
path = Path("data/example.jpg")
path.parent.mkdir(parents=True, exist_ok=True)
urlretrieve(default_url, path)
pipeline = int8_pipe if pipe_precision.value == "INT8" else ov_pipe
def generate(img, text, seed, num_steps, _=gr.Progress(track_tqdm=True)):
if img is None:
raise gr.Error("Please upload an image or choose one from the examples list")
np.random.seed(seed)
result = pipeline(text, img, num_steps)[0]
return result
demo = gr.Interface(
generate,
[
gr.Image(label="Image", type="pil"),
gr.Textbox(label="Text"),
gr.Slider(0, 1024, label="Seed", value=42),
gr.Slider(
1,
100,
label="Steps",
value=10,
info="Consider increasing the value to get more precise results. A suggested value is 100, but it will take more time to process.",
),
],
gr.Image(label="Result"),
examples=[[path, "Make it in galaxy"]],
)
try:
demo.queue().launch(debug=False)
except Exception:
demo.queue().launch(share=True, debug=False)
# if you are launching remotely, specify server_name and server_port
# demo.launch(server_name='your server name', server_port='server port in int')
# Read more in the docs: https://gradio.app/docs/