OpenVINO™ を使用した YOLOv9 の変換と最適化#
この Jupyter ノートブックは、ローカルへのインストール後にのみ起動できます。
YOLOv9 は、プログラマブル勾配情報 (PGI) や一般化効率レイヤー集約ネットワーク (GELAN) などの画期的な技術を導入し、リアルタイムの物体検出に大きな進歩をもたらしました。このモデルは、効率、精度、適応性において顕著な改善を示し、MS COCO データセットに新たなベンチマークを設定しました。モデルの詳細については、論文と元のリポジトリーを参照してください。このチュートリアルでは、OpenVINO を使用して PyTorch YOLO V9 を実行および最適化する方法について、手順を追って説明します。
このチュートリアルは次のステップで構成されます:
PyTorch モデルの準備
PyTorch モデルを OpenVINO IR に変換
OpenVINO でモデル推論を実行
最適化パイプラインの準備と実行
FP32 モデルと量子化モデルのパフォーマンスを比較します。
ビデオで最適化されたモデル推論を実行
目次:
必要条件#
import platform
%pip install -q "openvino>=2023.3.0" "nncf>=2.8.1" "opencv-python" "seaborn" "pandas" "scikit-learn" "torch" "torchvision" "tqdm" --extra-index-url https://download.pytorch.org/whl/cpu
if platform.system() != "Windows":
%pip install -q "matplotlib>=3.4"
else:
%pip install -q "matplotlib>=3.4,<3.7"
Note: you may need to restart the kernel to use updated packages.
Note: you may need to restart the kernel to use updated packages.
from pathlib import Path
# `notebook_utils` モジュールを取得
import requests
r = requests.get(
url="https://raw.githubusercontent.com/openvinotoolkit/openvino_notebooks/latest/utils/notebook_utils.py",
)
open("notebook_utils.py", "w").write(r.text)
from notebook_utils import download_file, VideoPlayer
if not Path("yolov9").exists(): !git clone https://github.com/WongKinYiu/yolov9 %cd yolov9
Cloning into 'yolov9'...
remote: Enumerating objects: 781, done.[K
remote: Counting objects: 100% (407/407), done.[K
remote: Compressing objects: 100% (168/168), done.[K
remote: Total 781 (delta 280), reused 279 (delta 227), pack-reused 374[K
Receiving objects: 100% (781/781), 3.30 MiB | 7.49 MiB/s, done.
Resolving deltas: 100% (325/325), done.
/opt/home/k8sworker/ci-ai/cibuilds/ov-notebook/OVNotebookOps-727/.workspace/scm/ov-notebook/notebooks/yolov9-optimization/yolov9
PyTorch モデルを取得#
一般に、PyTorch モデルは、モデルの重みを含む状態辞書によって初期化された torch.nn.Module クラスのインスタンスを表します。このリポジトリーで入手可能な COCO データセットで事前トレーニングされた gelan-c
(yolov9 の軽量バージョン) モデルを使用しますが、同じ手順は YOLO V9 ファミリーの他のモデルにも適用できます。
# 事前トレーニング済みモデルの重みをダウンロード
MODEL_LINK = "https://github.com/WongKinYiu/yolov9/releases/download/v0.1/gelan-c.pt"
DATA_DIR = Path("data/")
MODEL_DIR = Path("model/")
MODEL_DIR.mkdir(exist_ok=True)
DATA_DIR.mkdir(exist_ok=True)
download_file(MODEL_LINK, directory=MODEL_DIR, show_progress=True)
model/gelan-c.pt: 0%| | 0.00/49.1M [00:00<?, ?B/s]
PosixPath('/opt/home/k8sworker/ci-ai/cibuilds/ov-notebook/OVNotebookOps-727/.workspace/scm/ov-notebook/notebooks/yolov9-optimization/yolov9/model/gelan-c.pt')
PyTorch モデルを OpenVINO IR に変換#
OpenVINO はモデル・トランスフォーメーション API を介して PyTorch モデル変換をサポートします。ov.convert_model
関数は、モデルをトレースするためモデル・オブジェクトとサンプル入力を受け入れ、このモデルを OpenVINO 形式で表す ov.Model
のインスタンスを返します。取得したモデルは、特定のデバイスに読み込む準備が整っており、ov.save_model
を使用して以降のデプロイのためディスクに保存することもできます。
from models.experimental import attempt_load
import torch
import openvino as ov
from models.yolo import Detect, DualDDetect
from utils.general import yaml_save, yaml_load
weights = MODEL_DIR / "gelan-c.pt"
ov_model_path = MODEL_DIR / weights.name.replace(".pt", "_openvino_model") / weights.name.replace(".pt", ".xml")
if not ov_model_path.exists():
model = attempt_load(weights, device="cpu", inplace=True, fuse=True)
metadata = {"stride": int(max(model.stride)), "names": model.names}
model.eval()
for k, m in model.named_modules():
if isinstance(m, (Detect, DualDDetect)):
m.inplace = False
m.dynamic = True
m.export = True
example_input = torch.zeros((1, 3, 640, 640))
model(example_input)
ov_model = ov.convert_model(model, example_input=example_input)
# yolov9 リポジトリー・インターフェイスとの互換性のために入力名と出力名を指定
ov_model.outputs[0].get_tensor().set_names({"output0"})
ov_model.inputs[0].get_tensor().set_names({"images"})
ov.save_model(ov_model, ov_model_path)
# メタデータを保存
yaml_save(ov_model_path.parent / weights.name.replace(".pt", ".yaml"), metadata)
else:
metadata = yaml_load(ov_model_path.parent / weights.name.replace(".pt", ".yaml"))
Fusing layers...
Model summary: 387 layers, 25288768 parameters, 0 gradients, 102.1 GFLOPs
/opt/home/k8sworker/ci-ai/cibuilds/ov-notebook/OVNotebookOps-727/.workspace/scm/ov-notebook/notebooks/yolov9-optimization/yolov9/models/yolo.py:108: TracerWarning: Converting a tensor to a Python boolean might cause the trace to be incorrect.We can't record the data flow of Python values, so this value will be treated as a constant in the future.This means that the trace might not generalize to other inputs!
elif self.dynamic or self.shape != shape:
['x']
モデルの推論を検証#
モデルの動作をテストするため、detect.py
に似た推論パイプラインを作成します。パイプラインは、前処理ステップ、OpenVINO モデルの推論、および境界ボックスを取得する結果の後処理で構成されます。
前処理#
モデル入力は [1, 3, 640, 640]
の形状を持つ N, C, H, W
形式のテンソルです。ここで:
N
- バッチ内の画像数 (バッチサイズ)C
- 画像チャネルH
- 画像の髙さW
- 画像の幅
モデルは、RGB チャネル形式で [0, 1] の範囲で正規化された画像を想定しています。モデルのサイズに合わせて画像のサイズを変更するには、幅と高さのアスペクト比が維持されるレターボックスのサイズ変更アプローチが使用されます。yolov9 リポジトリーで定義されています。
特定の形状を維持するため、前処理によってパディングが自動的に有効になります。
import numpy as np
import torch
from PIL import Image from utils.augmentations import letterbox
image_url = "https://github.com/openvinotoolkit/openvino_notebooks/assets/29454499/7b6af406-4ccb-4ded-a13d-62b7c0e42e96"
download_file(image_url, directory=DATA_DIR, filename="test_image.jpg", show_progress=True)
def preprocess_image(img0: np.ndarray):
"""
Preprocess image according to YOLOv9 input requirements.Takes image in np.array format, resizes it to specific size using letterbox resize, converts color space from BGR (default in OpenCV) to RGB and changes data layout from HWC to CHW.
Parameters:
img0 (np.ndarray): image for preprocessing
Returns:
img (np.ndarray): image after preprocessing
img0 (np.ndarray): original image
"""
# リサイズ
img = letterbox(img0, auto=False)[0]
# 変換
img = img.transpose(2, 0, 1)
img = np.ascontiguousarray(img)
return img, img0
def prepare_input_tensor(image: np.ndarray):
"""
Converts preprocessed image to tensor format according to YOLOv9 input requirements.
Takes image in np.array format with unit8 data in [0, 255] range and converts it to torch.Tensor object with float data in [0, 1] range
Parameters:
image (np.ndarray): image for conversion to tensor
Returns:
input_tensor (torch.Tensor): float tensor ready to use for YOLOv9 inference
"""
input_tensor = image.astype(np.float32) # uint8 から fp16/32
input_tensor /= 255.0 # 0 - 255 から 0.0 - 1.0
if input_tensor.ndim == 3:
input_tensor = np.expand_dims(input_tensor, 0)
return input_tensor
NAMES = metadata["names"]
data/test_image.jpg: 0%| | 0.00/101k [00:00<?, ?B/s]
後処理#
モデル出力には検出ボックスの候補が含まれます。これは、B, N, 85
形式の [1,25200,85]
の形状を持つテンソルです。ここで:
B
- バッチサイズN
- 検出ボックスの数
検出ボックスの形式は [x
, y
, h
, w
, box_score
,
class_no_1
, …, class_no_80
] です。ここで:
(
x
,y
) - ボックス中心の生座標h
,w
- ボックスの生の高さと幅box_score
- 検出ボックスの信頼度class_no_1
, …,class_no_80
- クラス全体の確率分布。
最終的な予測を得るには、非最大抑制アルゴリズムを適用し、ボックスの座標を元の画像サイズに再スケールする必要があります。
from utils.plots import Annotator, colors
from typing import List, Tuple
from utils.general import scale_boxes, non_max_suppression
def detect(
model: ov.Model,
image_path: Path,
conf_thres: float = 0.25,
iou_thres: float = 0.45,
classes: List[int] = None,
agnostic_nms: bool = False,
):
"""
OpenVINO YOLOv9 model inference function.Reads image, preprocess it, runs model inference and postprocess results using NMS.Parameters:
model (Model): OpenVINO compiled model.
image_path (Path): input image path.
conf_thres (float, *optional*, 0.25): minimal accepted confidence for object filtering
iou_thres (float, *optional*, 0.45): minimal overlap score for removing objects duplicates in NMS
classes (List[int], *optional*, None): labels for prediction filtering, if not provided all predicted labels will be used
agnostic_nms (bool, *optional*, False): apply class agnostic NMS approach or not
Returns:
pred (List): list of detections with (n,6) shape, where n - number of detected boxes in format [x1, y1, x2, y2, score, label]
orig_img (np.ndarray): image before preprocessing, can be used for results visualization
inpjut_shape (Tuple[int]): shape of model input tensor, can be used for output rescaling
"""
if isinstance(image_path, np.ndarray):
img = image_path
else:
img = np.array(Image.open(image_path))
preprocessed_img, orig_img = preprocess_image(img)
input_tensor = prepare_input_tensor(preprocessed_img)
predictions = torch.from_numpy(model(input_tensor)[0])
pred = non_max_suppression(predictions, conf_thres, iou_thres, classes=classes, agnostic=agnostic_nms)
return pred, orig_img, input_tensor.shape
def draw_boxes(
predictions: np.ndarray,
input_shape: Tuple[int],
image: np.ndarray,
names: List[str],
):
"""
Utility function for drawing predicted bounding boxes on image
Parameters:
predictions (np.ndarray): list of detections with (n,6) shape, where n - number of detected boxes in format [x1, y1, x2, y2, score, label]
image (np.ndarray): image for boxes visualization
names (List[str]): list of names for each class in dataset
colors (Dict[str, int]): mapping between class name and drawing color
Returns:
image (np.ndarray): box visualization result
"""
if not len(predictions):
return image
annotator = Annotator(image, line_width=1, example=str(names))
# 入力サイズから元の画像サイズにボックスのサイズを変更
predictions[:, :4] = scale_boxes(input_shape[2:], predictions[:, :4], image.shape).round()
# 結果を書き込み
for *xyxy, conf, cls in reversed(predictions):
label = f"{names[int(cls)]} {conf:.2f}"
annotator.box_label(xyxy, label, color=colors(int(cls), True))
return image
core = ov.Core()
# 変換されたモデルを読み込み
ov_model = core.read_model(ov_model_path)
推論デバイスの選択#
OpenVINO を使用して推論を実行するためにドロップダウン・リストからデバイスを選択します
import ipywidgets as widgets
device = widgets.Dropdown(
options=core.available_devices + ["AUTO"],
value="AUTO",
description="Device:",
disabled=False,
)
device
Dropdown(description='Device:', index=1, options=('CPU', 'AUTO'), value='AUTO')
# 選択したデバイスにモデルをロード
if device.value != "CPU":
ov_model.reshape({0: [1, 3, 640, 640]})
compiled_model = core.compile_model(ov_model, device.value)
boxes, image, input_shape = detect(compiled_model, DATA_DIR / "test_image.jpg")
image_with_boxes = draw_boxes(boxes[0], input_shape, image, NAMES)
# 結果を視覚化
Image.fromarray(image_with_boxes)
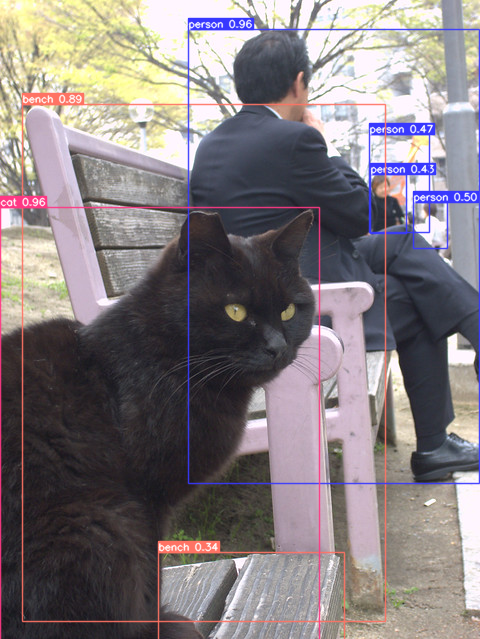
NNCF トレーニング後の量子化 API を使用してモデルを最適化#
NNCF は、精度の低下を最小限に抑えながら、OpenVINO でニューラル・ネットワーク推論を最適化する一連の高度なアルゴリズムを提供します。YOLOv9 を最適化するため、ポストトレーニング・モード (微調整パイプラインなし) で 8 ビット量子化を使用します。最適化プロセスには次の手順が含まれます:
量子化用のデータセットを作成します。
nncf.quantize
を実行して、最適化されたモデルを取得します。ov.save_model
関数を使用して、OpenVINO IR モデルをシリアル化します。
データセットの準備#
以下のコードは、COCO データセットをダウンロードし、yolov9 モデルの精度を評価するために使用されるデータローダーを準備します。そのサブセットを量子化に再利用します。
from zipfile import ZipFile
DATA_URL = "http://images.cocodataset.org/zips/val2017.zip"
LABELS_URL = "https://github.com/ultralytics/yolov5/releases/download/v1.0/coco2017labels-segments.zip"
OUT_DIR = Path(".")
download_file(DATA_URL, directory=OUT_DIR, show_progress=True)
download_file(LABELS_URL, directory=OUT_DIR, show_progress=True)
if not (OUT_DIR / "coco/labels").exists():
with ZipFile("coco2017labels-segments.zip", "r") as zip_ref:
zip_ref.extractall(OUT_DIR)
with ZipFile("val2017.zip", "r") as zip_ref:
zip_ref.extractall(OUT_DIR / "coco/images")
val2017.zip: 0%| | 0.00/778M [00:00<?, ?B/s]
coco2017labels-segments.zip: 0%| | 0.00/169M [00:00<?, ?B/s]
from collections import namedtuple
import yaml
from utils.dataloaders import create_dataloader
from utils.general import colorstr
# データセット構成の読み取り
DATA_CONFIG = "data/coco.yaml"
with open(DATA_CONFIG) as f:
data = yaml.load(f, Loader=yaml.SafeLoader)
# データローダー
TASK = "val" # トレーニング/検証/テスト画像へのパス
Option = namedtuple("Options", ["single_cls"]) # 単一クラス評価のためのコマンドライン・オプションの模倣
opt = Option(False)
dataloader = create_dataloader(
str(Path("coco") / data[TASK]),
640,
1,
32,
opt,
pad=0.5,
prefix=colorstr(f"{TASK}: "),
)[0]
val: Scanning coco/val2017... 4952 images, 48 backgrounds, 0 corrupt: 100%|██████████| 5000/5000 00:00
val: New cache created: coco/val2017.cache
NNCF は、量子化パイプラインでネイティブ・フレームワーク・データローダーを使用する nncf.Dataset
ラッパーを提供します。さらに、モデルが期待する形式で入力データを準備する変換関数を指定します。
import nncf
def transform_fn(data_item):
"""
Quantization transform function.Extracts and preprocess input data from dataloader item for quantization.Parameters:
data_item: Tuple with data item produced by DataLoader during iteration
Returns:
input_tensor: Input data for quantization
"""
img = data_item[0].numpy()
input_tensor = prepare_input_tensor(img)
return input_tensor
quantization_dataset = nncf.Dataset(dataloader, transform_fn)
INFO:nncf:NNCF initialized successfully.Supported frameworks detected: torch, tensorflow, onnx, openvino
モデル量子化の実行#
nncf.quantize
関数は、モデル量子化のインターフェイスを提供します。OpenVINO モデルのインスタンスと量子化データセットが必要です。オプションで、量子化プロセスの追加パラメーター (量子化のサンプル数、プリセット、無視される範囲など) を提供できます。YOLOv9 モデルには、活性化の非対称量子化を必要とする非 ReLU 活性化関数が含まれています。さらに良い結果を得るため、混合量子化プリセットを使用します。これは、重みの対称量子化と活性化の非対称量子化を提供します。
ov_int8_model_path = MODEL_DIR / weights.name.replace(".pt", "_int8_openvino_model") / weights.name.replace(".pt", "_int8.xml")
if not ov_int8_model_path.exists():
quantized_model = nncf.quantize(ov_model, quantization_dataset, preset=nncf.QuantizationPreset.MIXED)
ov.save_model(quantized_model, ov_int8_model_path)
yaml_save(ov_int8_model_path.parent / weights.name.replace(".pt", "_int8.yaml"), metadata)
2024-07-13 04:25:19.627535: I tensorflow/core/util/port.cc:110] oneDNN custom operations are on.You may see slightly different numerical results due to floating-point round-off errors from different computation orders.To turn them off, set the environment variable TF_ENABLE_ONEDNN_OPTS=0. 2024-07-13 04:25:19.663330: I tensorflow/core/platform/cpu_feature_guard.cc:182] This TensorFlow binary is optimized to use available CPU instructions in performance-critical operations.To enable the following instructions: AVX2 AVX512F AVX512_VNNI FMA, in other operations, rebuild TensorFlow with the appropriate compiler flags. 2024-07-13 04:25:20.258143: W tensorflow/compiler/tf2tensorrt/utils/py_utils.cc:38] TF-TRT Warning: Could not find TensorRT
Output()
Output()
Converting value of float32 to float16. Memory sharing is disabled by default.Set shared_memory=False to hide this warning.
Converting value of float32 to float16.Memory sharing is disabled by default.Set shared_memory=False to hide this warning.
Converting value of float32 to float16.Memory sharing is disabled by default.Set shared_memory=False to hide this warning.
Converting value of float32 to float16.Memory sharing is disabled by default.Set shared_memory=False to hide this warning.
Converting value of float32 to float16.Memory sharing is disabled by default.Set shared_memory=False to hide this warning.
Converting value of float32 to float16.Memory sharing is disabled by default.Set shared_memory=False to hide this warning.
Converting value of float32 to float16.Memory sharing is disabled by default.Set shared_memory=False to hide this warning.
Converting value of float32 to float16.Memory sharing is disabled by default.Set shared_memory=False to hide this warning.
Converting value of float32 to float16.Memory sharing is disabled by default.Set shared_memory=False to hide this warning.
Converting value of float32 to float16.Memory sharing is disabled by default.Set shared_memory=False to hide this warning.
Converting value of float32 to float16.Memory sharing is disabled by default.Set shared_memory=False to hide this warning.
Converting value of float32 to float16.Memory sharing is disabled by default.Set shared_memory=False to hide this warning.
Converting value of float32 to float16.Memory sharing is disabled by default.Set shared_memory=False to hide this warning.
Converting value of float32 to float16.Memory sharing is disabled by default.Set shared_memory=False to hide this warning.
Converting value of float32 to float16.Memory sharing is disabled by default.Set shared_memory=False to hide this warning.
Converting value of float32 to float16.Memory sharing is disabled by default.Set shared_memory=False to hide this warning.
Converting value of float32 to float16.Memory sharing is disabled by default.Set shared_memory=False to hide this warning.
Converting value of float32 to float16.Memory sharing is disabled by default.Set shared_memory=False to hide this warning.
Converting value of float32 to float16.Memory sharing is disabled by default.Set shared_memory=False to hide this warning.
Converting value of float32 to float16.Memory sharing is disabled by default.Set shared_memory=False to hide this warning.
Converting value of float32 to float16.Memory sharing is disabled by default.Set shared_memory=False to hide this warning.
Converting value of float32 to float16.Memory sharing is disabled by default.Set shared_memory=False to hide this warning.
Converting value of float32 to float16.Memory sharing is disabled by default.Set shared_memory=False to hide this warning.
Converting value of float32 to float16.Memory sharing is disabled by default.Set shared_memory=False to hide this warning.
Converting value of float32 to float16.Memory sharing is disabled by default.Set shared_memory=False to hide this warning.
Converting value of float32 to float16.Memory sharing is disabled by default.Set shared_memory=False to hide this warning.
Converting value of float32 to float16.Memory sharing is disabled by default.Set shared_memory=False to hide this warning.
Converting value of float32 to float16.Memory sharing is disabled by default.Set shared_memory=False to hide this warning.
Converting value of float32 to float16.Memory sharing is disabled by default.Set shared_memory=False to hide this warning.
Converting value of float32 to float16.Memory sharing is disabled by default.Set shared_memory=False to hide this warning.
Converting value of float32 to float16.Memory sharing is disabled by default.Set shared_memory=False to hide this warning.
Converting value of float32 to float16.Memory sharing is disabled by default.Set shared_memory=False to hide this warning.
Converting value of float32 to float16.Memory sharing is disabled by default.Set shared_memory=False to hide this warning.
Converting value of float32 to float16.Memory sharing is disabled by default.Set shared_memory=False to hide this warning.
Converting value of float32 to float16.Memory sharing is disabled by default.Set shared_memory=False to hide this warning.
Converting value of float32 to float16.Memory sharing is disabled by default.Set shared_memory=False to hide this warning.
Converting value of float32 to float16.Memory sharing is disabled by default.Set shared_memory=False to hide this warning.
Converting value of float32 to float16.Memory sharing is disabled by default.Set shared_memory=False to hide this warning.
Converting value of float32 to float16.Memory sharing is disabled by default.Set shared_memory=False to hide this warning.
Converting value of float32 to float16.Memory sharing is disabled by default.Set shared_memory=False to hide this warning.
Converting value of float32 to float16.Memory sharing is disabled by default.Set shared_memory=False to hide this warning.
Converting value of float32 to float16.Memory sharing is disabled by default.Set shared_memory=False to hide this warning.
Converting value of float32 to float16.Memory sharing is disabled by default.Set shared_memory=False to hide this warning.
Converting value of float32 to float16.Memory sharing is disabled by default.Set shared_memory=False to hide this warning.
Converting value of float32 to float16.Memory sharing is disabled by default.Set shared_memory=False to hide this warning.
Converting value of float32 to float16.Memory sharing is disabled by default.Set shared_memory=False to hide this warning.
Converting value of float32 to float16.Memory sharing is disabled by default.Set shared_memory=False to hide this warning.
Converting value of float32 to float16.Memory sharing is disabled by default.Set shared_memory=False to hide this warning.
Converting value of float32 to float16.Memory sharing is disabled by default.Set shared_memory=False to hide this warning.
Converting value of float32 to float16.Memory sharing is disabled by default.Set shared_memory=False to hide this warning.
Converting value of float32 to float16.Memory sharing is disabled by default.Set shared_memory=False to hide this warning.
Converting value of float32 to float16.Memory sharing is disabled by default.Set shared_memory=False to hide this warning.
Converting value of float32 to float16.Memory sharing is disabled by default.Set shared_memory=False to hide this warning.
Converting value of float32 to float16.Memory sharing is disabled by default.Set shared_memory=False to hide this warning.
Converting value of float32 to float16.Memory sharing is disabled by default.Set shared_memory=False to hide this warning.
Converting value of float32 to float16.Memory sharing is disabled by default.Set shared_memory=False to hide this warning.
Converting value of float32 to float16.Memory sharing is disabled by default.Set shared_memory=False to hide this warning.
Converting value of float32 to float16.Memory sharing is disabled by default.Set shared_memory=False to hide this warning.
Converting value of float32 to float16.Memory sharing is disabled by default.Set shared_memory=False to hide this warning.
Converting value of float32 to float16.Memory sharing is disabled by default.Set shared_memory=False to hide this warning.
Converting value of float32 to float16.Memory sharing is disabled by default.Set shared_memory=False to hide this warning.
Converting value of float32 to float16.Memory sharing is disabled by default.Set shared_memory=False to hide this warning.
Converting value of float32 to float16.Memory sharing is disabled by default.Set shared_memory=False to hide this warning.
Converting value of float32 to float16.Memory sharing is disabled by default.Set shared_memory=False to hide this warning.
Converting value of float32 to float16.Memory sharing is disabled by default.Set shared_memory=False to hide this warning.
Converting value of float32 to float16.Memory sharing is disabled by default.Set shared_memory=False to hide this warning.
Converting value of float32 to float16.Memory sharing is disabled by default.Set shared_memory=False to hide this warning.
Converting value of float32 to float16.Memory sharing is disabled by default.Set shared_memory=False to hide this warning.
Converting value of float32 to float16.Memory sharing is disabled by default.Set shared_memory=False to hide this warning.
Converting value of float32 to float16.Memory sharing is disabled by default.Set shared_memory=False to hide this warning.
Converting value of float32 to float16.Memory sharing is disabled by default.Set shared_memory=False to hide this warning.
Converting value of float32 to float16.Memory sharing is disabled by default.Set shared_memory=False to hide this warning.
Converting value of float32 to float16.Memory sharing is disabled by default.Set shared_memory=False to hide this warning.
Converting value of float32 to float16.Memory sharing is disabled by default.Set shared_memory=False to hide this warning.
Converting value of float32 to float16.Memory sharing is disabled by default.Set shared_memory=False to hide this warning.
Converting value of float32 to float16.Memory sharing is disabled by default.Set shared_memory=False to hide this warning.
Converting value of float32 to float16.Memory sharing is disabled by default.Set shared_memory=False to hide this warning.
Converting value of float32 to float16.Memory sharing is disabled by default.Set shared_memory=False to hide this warning.
Converting value of float32 to float16.Memory sharing is disabled by default.Set shared_memory=False to hide this warning.
Converting value of float32 to float16.Memory sharing is disabled by default.Set shared_memory=False to hide this warning.
Converting value of float32 to float16.Memory sharing is disabled by default.Set shared_memory=False to hide this warning.
Converting value of float32 to float16.Memory sharing is disabled by default.Set shared_memory=False to hide this warning.
Converting value of float32 to float16.Memory sharing is disabled by default.Set shared_memory=False to hide this warning.
Converting value of float32 to float16.Memory sharing is disabled by default.Set shared_memory=False to hide this warning.
Converting value of float32 to float16.Memory sharing is disabled by default.Set shared_memory=False to hide this warning.
Converting value of float32 to float16.Memory sharing is disabled by default.Set shared_memory=False to hide this warning.
Converting value of float32 to float16.Memory sharing is disabled by default.Set shared_memory=False to hide this warning.
Converting value of float32 to float16.Memory sharing is disabled by default.Set shared_memory=False to hide this warning.
Converting value of float32 to float16.Memory sharing is disabled by default.Set shared_memory=False to hide this warning.
Converting value of float32 to float16.Memory sharing is disabled by default.Set shared_memory=False to hide this warning.
量子化モデルの推論を実行#
量子化を適用した後、モデルの使用法に変化はありません。以前使用した画像でモデルの動作を確認します。
quantized_model = core.read_model(ov_int8_model_path)
if device.value != "CPU":
quantized_model.reshape({0: [1, 3, 640, 640]})
compiled_model = core.compile_model(quantized_model, device.value)
boxes, image, input_shape = detect(compiled_model, DATA_DIR / "test_image.jpg")
image_with_boxes = draw_boxes(boxes[0], input_shape, image, NAMES)
# 結果を視覚化
Image.fromarray(image_with_boxes)
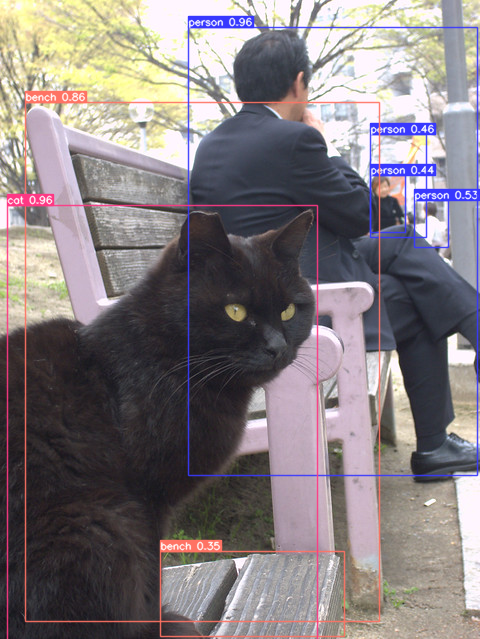
元のモデルと量子化モデルのパフォーマンスを比較#
OpenVINO Benchmark ツールを使用して、FP32
と INT8
モデルの推論パフォーマンスを測定します。
注: より正確なパフォーマンスを得るには、他のアプリケーションを閉じて、ターミナル/コマンドプロンプトで
benchmark_app
を実行することを推奨します。benchmark_app -m model.xml -d CPU
を実行して、CPU で非同期推論のベンチマークを 1 分間実行します。GPU でベンチマークを行うには、CPU
をGPU
に変更します。benchmark_app --help
を実行すると、すべてのコマンドライン・オプションの概要が表示されます。
!benchmark_app -m $ov_model_path -shape "[1,3,640,640]" -d $device.value -api async -t 15
[Step 1/11] Parsing and validating input arguments
[ INFO ] Parsing input parameters
[Step 2/11] Loading OpenVINO Runtime
[ INFO ] OpenVINO:
[ INFO ] Build .................................2024.4.0-16028-fe423b97163
[ INFO ]
[ INFO ] Device info:
[ INFO ] AUTO
[ INFO ] Build .................................2024.4.0-16028-fe423b97163
[ INFO ]
[ INFO ]
[Step 3/11] Setting device configuration
[ WARNING ] Performance hint was not explicitly specified in command line.Device(AUTO) performance hint will be set to PerformanceMode.THROUGHPUT.
[Step 4/11] Reading model files
[ INFO ] Loading model files
[ INFO ] Read model took 26.21 ms
[ INFO ] Original model I/O parameters:
[ INFO ] Model inputs:
[ INFO ] images (node: x) : f32 / [...] / [?,3,?,?]
[ INFO ] Model outputs:
[ INFO ] output0 (node: __module.model.22/aten::cat/Concat_5) : f32 / [...]/ [?,84,8400]
[ INFO ] xi.1 (node: __module.model.22/aten::cat/Concat_2) : f32 / [...]/ [?,144,4..,4..]
[ INFO ] xi.3 (node: __module.model.22/aten::cat/Concat_1) : f32 / [...]/ [?,144,2..,2..]
[ INFO ] xi (node: __module.model.22/aten::cat/Concat) : f32 / [...] / [?,144,1..,1..]
[Step 5/11] Resizing model to match image sizes and given batch
[ INFO ] Model batch size: 1
[ INFO ] Reshaping model: 'images': [1,3,640,640]
[ INFO ] Reshape model took 7.85 ms
[Step 6/11] Configuring input of the model
[ INFO ] Model inputs:
[ INFO ] images (node: x) : u8 / [N,C,H,W] / [1,3,640,640]
[ INFO ] Model outputs:
[ INFO ] output0 (node: __module.model.22/aten::cat/Concat_5) : f32 / [...]/ [1,84,8400]
[ INFO ] xi.1 (node: __module.model.22/aten::cat/Concat_2) : f32 / [...]/ [1,144,80,80]
[ INFO ] xi.3 (node: __module.model.22/aten::cat/Concat_1) : f32 / [...]/ [1,144,40,40]
[ INFO ] xi (node: __module.model.22/aten::cat/Concat) : f32 / [...]/ [1,144,20.20]
[Step 7/11] Loading the model to the device
[ INFO ] Compile model took 490.27 ms
[Step 8/11] Querying optimal runtime parameters
[ INFO ] Model:
[ INFO ] NETWORK_NAME: Model0
[ INFO ] EXECUTION_DEVICES: ['CPU']
[ INFO ] PERFORMANCE_HINT: PerformanceMode.THROUGHPUT
[ INFO ] OPTIMAL_NUMBER_OF_INFER_REQUESTS: 6
[ INFO ] MULTI_DEVICE_PRIORITIES: CPU
[ INFO ] CPU:
[ INFO ] AFFINITY: Affinity.CORE
[ INFO ] CPU_DENORMALS_OPTIMIZATION: False
[ INFO ] CPU_SPARSE_WEIGHTS_DECOMPRESSION_RATE: 1.0
[ INFO ] DYNAMIC_QUANTIZATION_GROUP_SIZE: 32
[ INFO ] ENABLE_CPU_PINNING: True
[ INFO ] ENABLE_HYPER_THREADING: True
[ INFO ] EXECUTION_DEVICES: ['CPU']
[ INFO ] EXECUTION_MODE_HINT: ExecutionMode.PERFORMANCE
[ INFO ] INFERENCE_NUM_THREADS: 24
[ INFO ] INFERENCE_PRECISION_HINT: <Type: 'float32'>
[ INFO ] KV_CACHE_PRECISION: <Type: 'float16'>
[ INFO ] LOG_LEVEL: Level.NO
[ INFO ] MODEL_DISTRIBUTION_POLICY: set()
[ INFO ] NETWORK_NAME: Model0
[ INFO ] NUM_STREAMS: 6
[ INFO ] OPTIMAL_NUMBER_OF_INFER_REQUESTS: 6
[ INFO ] PERFORMANCE_HINT: THROUGHPUT
[ INFO ] PERFORMANCE_HINT_NUM_REQUESTS: 0
[ INFO ] PERF_COUNT: NO
[ INFO ] SCHEDULING_CORE_TYPE: SchedulingCoreType.ANY_CORE
[ INFO ] MODEL_PRIORITY: Priority.MEDIUM
[ INFO ] LOADED_FROM_CACHE: False
[ INFO ] PERF_COUNT: False
[Step 9/11] Creating infer requests and preparing input tensors
[ WARNING ] No input files were given for input 'images'!.This input will be filled with random values!
[ INFO ] Fill input 'images' with random values
[Step 10/11] Measuring performance (Start inference asynchronously, 6 inference requests, limits: 15000 ms duration)
[ INFO ] Benchmarking in inference only mode (inputs filling are not included in measurement loop).
[ INFO ] First inference took 186.95 ms
[Step 11/11] Dumping statistics report
[ INFO ] Execution Devices:['CPU']
[ INFO ] Count: 228 iterations
[ INFO ] Duration: 15678.96 ms
[ INFO ] Latency:
[ INFO ] Median: 413.56 ms
[ INFO ] Average: 411.44 ms
[ INFO ] Min: 338.36 ms
[ INFO ] Max: 431.50 ms
[ INFO ] Throughput: 14.54 FPS
!benchmark_app -m $ov_int8_model_path -shape "[1,3,640,640]" -d $device.value -api async -t 15
[Step 1/11] Parsing and validating input arguments
[ INFO ] Parsing input parameters
[Step 2/11] Loading OpenVINO Runtime
[ INFO ] OpenVINO:
[ INFO ] Build .................................2024.4.0-16028-fe423b97163
[ INFO ]
[ INFO ] Device info:
[ INFO ] AUTO
[ INFO ] Build .................................2024.4.0-16028-fe423b97163
[ INFO ]
[ INFO ]
[Step 3/11] Setting device configuration
[ WARNING ] Performance hint was not explicitly specified in command line.Device(AUTO) performance hint will be set to PerformanceMode.THROUGHPUT.
[Step 4/11] Reading model files
[ INFO ] Loading model files
[ INFO ] Read model took 40.98 ms
[ INFO ] Original model I/O parameters:
[ INFO ] Model inputs:
[ INFO ] images (node: x) : f32 / [...]/ [1.3,640,640]
[ INFO ] Model outputs:
[ INFO ] output0 (node: __module.model.22/aten::cat/Concat_5) : f32 / [...]/ [1.84,8400]
[ INFO ] xi.1 (node: __module.model.22/aten::cat/Concat_2) : f32 / [...]/ [1,144,80,80]
[ INFO ] xi.3 (node: __module.model.22/aten::cat/Concat_1) : f32 / [...]/ [1,144,40,40]
[ INFO ] xi (node: __module.model.22/aten::cat/Concat) : f32 / [...]/ [1,144,20.20]
[Step 5/11] Resizing model to match image sizes and given batch
[ INFO ] Model batch size: 1
[ INFO ] Reshaping model: 'images': [1,3,640,640]
[ INFO ] Reshape model took 0.05 ms
[Step 6/11] Configuring input of the model
[ INFO ] Model inputs:
[ INFO ] images (node: x) : u8 / [N,C,H,W] / [1,3,640,640]
[ INFO ] Model outputs:
[ INFO ] output0 (node: __module.model.22/aten::cat/Concat_5) : f32 / [...]/ [1.84,8400]
[ INFO ] xi.1 (node: __module.model.22/aten::cat/Concat_2) : f32 / [...]/ [1,144,80,80]
[ INFO ] xi.3 (node: __module.model.22/aten::cat/Concat_1) : f32 / [...]/ [1,144,40,40]
[ INFO ] xi (node: __module.model.22/aten::cat/Concat) : f32 / [...]/ [1,144,20.20]
[Step 7/11] Loading the model to the device
[ INFO ] Compile model took 964.26 ms
[Step 8/11] Querying optimal runtime parameters
[ INFO ] Model:
[ INFO ] NETWORK_NAME: Model0
[ INFO ] EXECUTION_DEVICES: ['CPU']
[ INFO ] PERFORMANCE_HINT: PerformanceMode.THROUGHPUT
[ INFO ] OPTIMAL_NUMBER_OF_INFER_REQUESTS: 6
[ INFO ] MULTI_DEVICE_PRIORITIES: CPU
[ INFO ] CPU:
[ INFO ] AFFINITY: Affinity.CORE
[ INFO ] CPU_DENORMALS_OPTIMIZATION: False
[ INFO ] CPU_SPARSE_WEIGHTS_DECOMPRESSION_RATE: 1.0
[ INFO ] DYNAMIC_QUANTIZATION_GROUP_SIZE: 32
[ INFO ] ENABLE_CPU_PINNING: True
[ INFO ] ENABLE_HYPER_THREADING: True
[ INFO ] EXECUTION_DEVICES: ['CPU']
[ INFO ] EXECUTION_MODE_HINT: ExecutionMode.PERFORMANCE
[ INFO ] INFERENCE_NUM_THREADS: 24
[ INFO ] INFERENCE_PRECISION_HINT: <Type: 'float32'>
[ INFO ] KV_CACHE_PRECISION: <Type: 'float16'>
[ INFO ] LOG_LEVEL: Level.NO
[ INFO ] MODEL_DISTRIBUTION_POLICY: set()
[ INFO ] NETWORK_NAME: Model0
[ INFO ] NUM_STREAMS: 6
[ INFO ] OPTIMAL_NUMBER_OF_INFER_REQUESTS: 6
[ INFO ] PERFORMANCE_HINT: THROUGHPUT
[ INFO ] PERFORMANCE_HINT_NUM_REQUESTS: 0
[ INFO ] PERF_COUNT: NO
[ INFO ] SCHEDULING_CORE_TYPE: SchedulingCoreType.ANY_CORE
[ INFO ] MODEL_PRIORITY: Priority.MEDIUM
[ INFO ] LOADED_FROM_CACHE: False
[ INFO ] PERF_COUNT: False
[Step 9/11] Creating infer requests and preparing input tensors
[ WARNING ] No input files were given for input 'images'!.This input will be filled with random values!
[ INFO ] Fill input 'images' with random values
[Step 10/11] Measuring performance (Start inference asynchronously, 6 inference requests, limits: 15000 ms duration)
[ INFO ] Benchmarking in inference only mode (inputs filling are not included in measurement loop).
[ INFO ] First inference took 77.25 ms
[Step 11/11] Dumping statistics report
[ INFO ] Execution Devices:['CPU']
[ INFO ] Count: 750 iterations
[ INFO ] Duration: 15181.84 ms
[ INFO ] Latency:
[ INFO ] Median: 121.39 ms
[ INFO ] Average: 121.02 ms
[ INFO ] Min: 93.56 ms
[ INFO ] Max: 133.28 ms
[ INFO ] Throughput: 49.40 FPS
生物検出の実行#
import collections
import time
from IPython import display
import cv2
# 物体検出を実行するメイン処理関数
def run_object_detection(
source=0,
flip=False,
use_popup=False,
skip_first_frames=0,
model=ov_model,
device=device.value,
):
player = None
compiled_model = core.compile_model(model, device)
try:
# ターゲット fps で再生するビデオプレーヤーを作成
player = VideoPlayer(source=source, flip=flip, fps=30, skip_first_frames=skip_first_frames)
# キャプチャー開始
player.start()
if use_popup:
title = "Press ESC to Exit"
cv2.namedWindow(winname=title, flags=cv2.WINDOW_GUI_NORMAL | cv2.WINDOW_AUTOSIZE)
processing_times = collections.deque()
while True:
# フレームをグラブ
frame = player.next()
if frame is None:
print("Source ended")
break
# フレームがフル HD より大きい場合は、サイズを縮小してパフォーマンスを向上させます
scale = 1280 / max(frame.shape)
if scale < 1:
frame = cv2.resize(
src=frame,
dsize=None,
fx=scale,
fy=scale,
interpolation=cv2.INTER_AREA,
)
# 結果を取得
input_image = np.array(frame)
start_time = time.time()
# モデルは RGB 画像を期待しますが、ビデオは BGR でキャプチャーします
detections, _, input_shape = detect(compiled_model, input_image[:, :, ::-1])
stop_time = time.time()
image_with_boxes = draw_boxes(detections[0], input_shape, input_image, NAMES)
frame = image_with_boxes
processing_times.append(stop_time - start_time)
# 最後の 200 フレームの処理時間を使用
if len(processing_times) > 200:
processing_times.popleft()
_, f_width = frame.shape[:2]
# 平均処理時間 [ms]
processing_time = np.mean(processing_times) * 1000
fps = 1000 / processing_time
cv2.putText(
img=frame,
text=f"Inference time: {processing_time:.1f}ms ({fps:.1f} FPS)",
org=(20, 40),
fontFace=cv2.FONT_HERSHEY_COMPLEX,
fontScale=f_width / 1000,
color=(0, 0, 255),
thickness=1,
lineType=cv2.LINE_AA,
)
# ちらつきがある場合はこの回避策を使用
if use_popup:
cv2.imshow(winname=title, mat=frame)
key = cv2.waitKey(1)
# escape = 27
if key == 27:
break
else:
# numpy 配列を jpg にエンコード
_, encoded_img = cv2.imencode(ext=".jpg", img=frame, params=[cv2.IMWRITE_JPEG_QUALITY, 100])
# IPython イメージを作成 ⬆️
i = display.Image(data=encoded_img)
# このノートブックに画像を表示
display.clear_output(wait=True)
display.display(i)
# ctrl-c
except KeyboardInterrupt:
print("Interrupted")
# 異なるエラー
except RuntimeError as e:
print(e)
finally:
if player is not None:
# キャプチャーを停止
Player.stop().stop()
if use_popup:
cv2.destroyAllWindows()
Web カメラをビデオ入力として使用します。デフォルトでは、プライマリー・ウェブ・カメラは source=0
に設定されます。複数のウェブカメラがある場合、0 から始まる連続した番号が割り当てられます。前面カメラを使用する場合は、flip=True
を設定します。一部のウェブブラウザー、特に Mozilla Firefox ではちらつきが発生する場合があります。ちらつきが発生する場合、use_popup=True
を設定してください。
注: このノートブックをウェブカメラで使用するには、ウェブカメラを備えたコンピューター上でノートブックを実行する必要があります。ノートブックをリモートサーバー (例えば、Binder または Google Colab サービス) で実行する場合、ウェブカメラは動作しません。デフォルトでは、下のセルはビデオファイルに対してモデル推論を実行します。ウェブカメラ・セットでライブ推論を試す場合、
WEBCAM_INFERENCE = True
を設定します
オブジェクト検出を実行します:
WEBCAM_INFERENCE = False
if WEBCAM_INFERENCE:
VIDEO_SOURCE = 0 # ウェブカメラ
else:
VIDEO_SOURCE = "https://storage.openvinotoolkit.org/repositories/openvino_notebooks/data/data/video/people.mp4"
device
Dropdown(description='Device:', index=1, options=('CPU', 'AUTO'), value='AUTO')
quantized_model = core.read_model(ov_int8_model_path)
run_object_detection(
source=VIDEO_SOURCE,
flip=True,
use_popup=False,
model=quantized_model,
device=device.value,
)
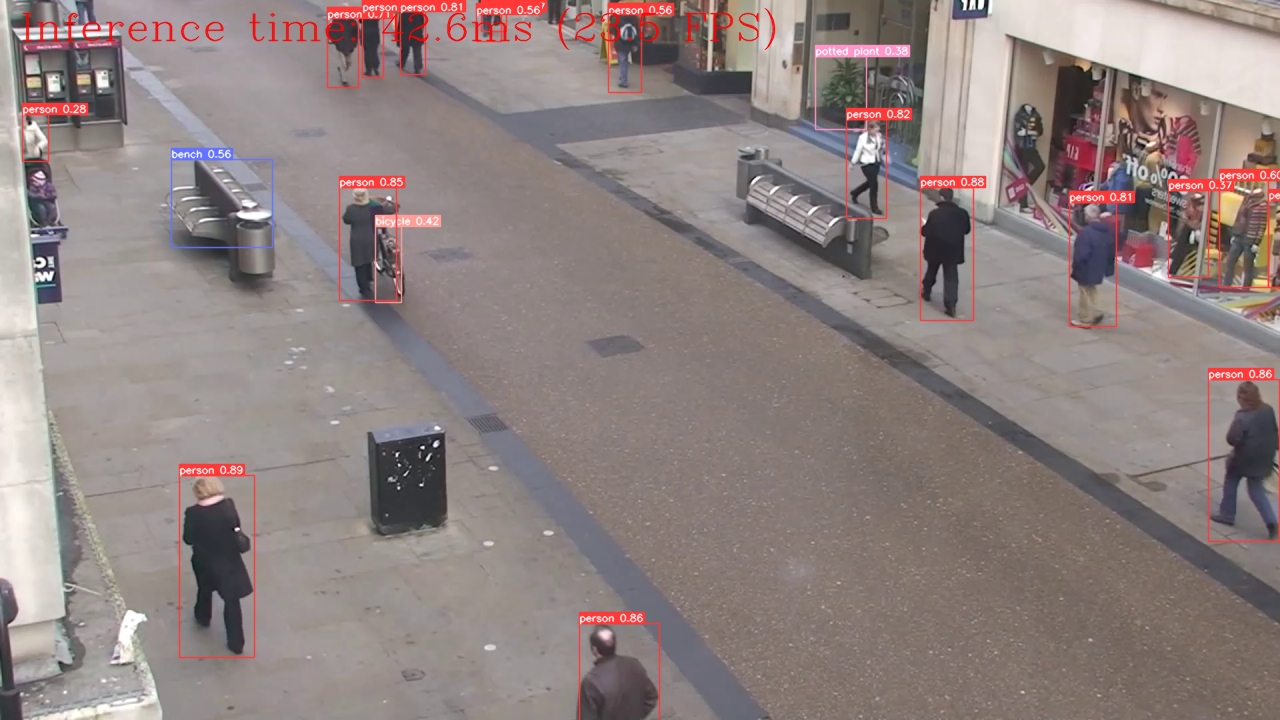
ソースの終わり