nanoLLaVA と OpenVINO による視覚言語アシスタント#
この Jupyter ノートブックはオンラインで起動でき、ブラウザーのウィンドウで対話型環境を開きます。ローカルにインストールすることもできます。次のオプションのいずれかを選択します:
nanoLLaVA は、エッジデバイス上で効率よく実行できるように設計された “小さいながらも強力な” 1B 視覚言語モデルです。画像エンコーダーとして SigLIP-400m を、LLM として Qwen1.5-0.5B を使用します。このチュートリアルでは、OpenVINO を使用して nanoLLaVA モデルを変換して実行する方法について説明します。また、NNCF を使用してモデルを最適化します。
目次:
必要条件#
%pip install -q "torch>=2.1" "transformers>=4.40" "accelerate" "pillow" "gradio>=4.26" "openvino>=2024.1.0" "tqdm" "nncf>=2.10" --extra-index-url https://download.pytorch.org/whl/cpu
ERROR: pip's dependency resolver does not currently take into account all the packages that are installed.This behaviour is the source of the following dependency conflicts.
mobileclip 0.1.0 requires torch==1.13.1, but you have torch 2.3.1+cpu which is incompatible.
mobileclip 0.1.0 requires torchvision==0.14.1, but you have torchvision 0.18.1+cpu which is incompatible. Note: you may need to restart the kernel to use updated packages.
from huggingface_hub import snapshot_download
from pathlib import Path
model_local_dir = Path("nanoLLaVA")
if not model_local_dir.exists():
snapshot_download(repo_id="qnguyen3/nanoLLaVA", local_dir=model_local_dir)
modeling_file = model_local_dir / "modeling_llava_qwen2.py"
orig_modeling_file = model_local_dir / f"orig_{modeling_file.name}"
# モデルコードは flash_attn パッケージに依存しており、読み込みに問題が発生する可能性があります。このパッケージのインポートを回避するためのパッチ・モデル・コード
if not orig_modeling_file.exists():
modeling_file.rename(orig_modeling_file)
with orig_modeling_file.open("r") as f:
content = f.read()
replacement_lines = [
("from flash_attn import flash_attn_func, flash_attn_varlen_func", ""),
("from flash_attn.bert_padding import index_first_axis, pad_input, unpad_input", ""),
(' _flash_supports_window_size = "window_size" in list(inspect.signature(flash_attn_func).parameters)', "pass"),
]
for replace_pair in replacement_lines:
content = content.replace(*replace_pair)
with modeling_file.open("w") as f:
f.write(content)
Fetching 14 files: 0%| | 0/14 [00:00<?, ?it/s]
README.md: 0%| | 0.00/3.47k [00:00<?, ?B/s]
generation_config.json: 0%| | 0.00/172 [00:00<?, ?B/s]
example_1.png: 0%| | 0.00/200k [00:00<?, ?B/s]
config.json: 0%| | 0.00/1.28k [00:00<?, ?B/s]
configuration_llava_qwen2.py: 0%| | 0.00/8.87k [00:00<?, ?B/s]
.gitattributes: 0%| | 0.00/1.52k [00:00<?, ?B/s]
added_tokens.json: 0%| | 0.00/80.0 [00:00<?, ?B/s]
modeling_llava_qwen2.py: 0%| | 0.00/103k [00:00<?, ?B/s]
special_tokens_map.json: 0%| | 0.00/510 [00:00<?, ?B/s]
tokenizer_config.json: 0%| | 0.00/1.32k [00:00<?, ?B/s]
vocab.json: 0%| | 0.00/2.78M [00:00<?, ?B/s]
tokenizer.json: 0%| | 0.00/7.03M [00:00<?, ?B/s]
merges.txt: 0%| | 0.00/1.67M [00:00<?, ?B/s]
model.safetensors: 0%| | 0.00/2.10G [00:00<?, ?B/s]
PyTorch モデルのロード#
PyTorch モデルを作成するには、AutoModelForCausalLM
モデルクラスの from_pretrained
メソッドを使用する必要があります。モデルの重みは、前の手順で snapshot_downloadsnapshot_download
関数を使用して HuggingFace ハブからすでにダウンロードされています。
import transformers
from transformers import AutoModelForCausalLM, AutoTokenizer
from PIL import Image
import warnings
transformers.logging.set_verbosity_error()
warnings.filterwarnings("ignore")
model = AutoModelForCausalLM.from_pretrained(model_local_dir, trust_remote_code=True)
tokenizer = AutoTokenizer.from_pretrained(model_local_dir, trust_remote_code=True)
2024-07-13 01:15:06.266352: I tensorflow/core/util/port.cc:110] oneDNN custom operations are on.You may see slightly different numerical results due to floating-point round-off errors from different computation orders.To turn them off, set the environment variable TF_ENABLE_ONEDNN_OPTS=0. 2024-07-13 01:15:06.301452: I tensorflow/core/platform/cpu_feature_guard.cc:182] This TensorFlow binary is optimized to use available CPU instructions in performance-critical operations. To enable the following instructions: AVX2 AVX512F AVX512_VNNI FMA, in other operations, rebuild TensorFlow with the appropriate compiler flags. 2024-07-13 01:15:06.954075: W tensorflow/compiler/tf2tensorrt/utils/py_utils.cc:38] TF-TRT Warning: Could not find TensorRT
PyTorch モデル推論を実行#
import torch
import requests
prompt = "Describe this image in detail"
messages = [{"role": "user", "content": f"<image>\n{prompt}"}]
text = tokenizer.apply_chat_template(messages, tokenize=False, add_generation_prompt=True)
text_chunks = [tokenizer(chunk).input_ids for chunk in text.split("<image>")]
input_ids = torch.tensor(text_chunks[0] + [-200] + text_chunks[1], dtype=torch.long).unsqueeze(0)
url = "https://github.com/openvinotoolkit/openvino_notebooks/assets/29454499/8bf7d9f2-018a-4498-bec4-55f17c273ecc"
image = Image.open(requests.get(url, stream=True).raw)
image_tensor = model.process_images([image], model.config)
print(prompt)
image
Describe this image in detail
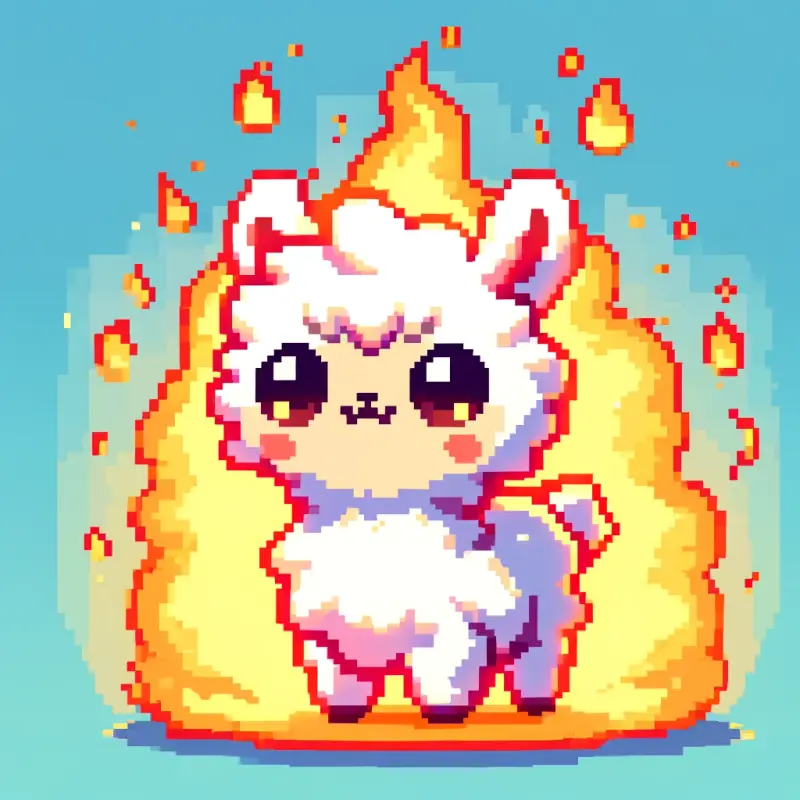
from transformers import TextStreamer
streamer = TextStreamer(tokenizer, skip_prompt=True, skip_special_tokens=True)
output_ids = model.generate(input_ids, images=image_tensor, max_new_tokens=128, use_cache=True, streamer=streamer)
This image captures a delightful scene featuring a white, fluffy lamb with a playful expression. The lamb is positioned towards the center of the image, its body filling most of the frame from left to right. The lamb has a charmingly expressive face, with a pair of black eyes that appear to be squinting slightly. It has a small, round, pink nose, and its ears are also pink, which contrasts with the rest of its white fur. The lamb's legs are white, and the lower part of its body is fluffy, adding to its adorable appearance. The lamb's face is quite expressive, with its eyes looking down and
モデルの変換と最適化#
当社のモデル変換と最適化は、次の手順で構成されます。
- モデルを OpenVINO 形式に変換し、ディスクに保存します。
- NNCF を使用してモデルの重みを圧縮します。
それぞれのステップをさらに深く考えてみましょう。
モデルを OpenVINO IR 形式に変換#
以下で定義する変換ヘルパー関数を使用して、モデルを OpenVINO 形式に変換します。PyTorch モデルを OpenVINO 中間表現形式に変換するには、OpenVINO モデル・トランスフォーメーション API を使用します。ov.convert_model
関数は、トレース用の PyTorch モデル・インスタンスとサンプル入力を受け入れ、core.compile_model
を使用してデバイス上でコンパイルしたり、ov.save_model
関数を使用して次回使用するためディスクに保存したりできる、OpenVINO モデル・オブジェクトを返します。生成ステップに応じて、モデルは各種入力を受け入れ、パイプラインのさまざまな部分をアクティブにします。同じレベルの柔軟性を維持するため、モデルをパーツごとに分割します: 画像エンコーダー、テキスト埋め込み、言語モデルを各パーツごとに変換します。
NNCF を使用してモデルの重みを 4 ビットと 8 ビットに圧縮#
メモリー消費を削減するため、NNCF を使用して重み圧縮を最適化できます。重み圧縮は、モデルのメモリー使用量を削減することを目的としています。また、大規模言語モデル (LLM) など、メモリーに依存する大規模なモデルのパフォーマンスが大幅に向上する可能性もあります。LLM やその他のモデルは、推論中に重みを保存する大量のメモリーを必要とするため、次の方法で重み圧縮の利点を得られます:
デバイスのメモリーに格納できない大規模なモデルの推論を可能にします。
線形レイヤーなどの重みを使用した演算を行う際のメモリーアクセス・レイテンシーを短縮することで、モデルの推論パフォーマンスを向上させます。
ニューラル・ネットワーク圧縮フレームワーク (NNCF) は、主に LLM の最適化向けに設計された圧縮方法として、4 ビット / 8 ビット混合重み量子化を提供します。重み圧縮とフルモデル量子化 (トレーニング後の量子化) 違いは、重み圧縮のでは、活性化が浮動小数点のままであるため、精度が向上することです。LLM の重み圧縮は、完全なモデル量子化のパフォーマンスに匹敵する推論パフォーマンスの向上をもたらします。さらに、重み圧縮はデータに依存せず、キャリブレーション・データセットも必要としないため、容易に利用できます。
nncf.compress_weights
関数は重み圧縮の実行に使用できます。この関数は、OpenVINO モデルとその他の圧縮パラメーターを受け入れます。INT8 圧縮と比較して、INT4 圧縮はパフォーマンスをさらに向上させますが、予測品質は若干低下します。
重み圧縮の詳細については、OpenVINO のドキュメントを参照してください。
注: dGPU 上の INT4 圧縮モデルでは高速化は行われません。
INT8 重み圧縮の代わりに INT4 重み圧縮を実行するかどうかは、以下で選択してください。
import ipywidgets as widgets
compression_mode = widgets.Dropdown(
options=["INT4", "INT8"],
value="INT4",
description="Compression mode:",
disabled=False,
)
compression_mode
Dropdown(description='Compression mode:', options=('INT4', 'INT8'), value='INT4')
import gc
import warnings
import torch
import openvino as ov
import nncf
from typing import Optional, Tuple
warnings.filterwarnings("ignore")
def flattenize_inputs(inputs):
"""
Helper function for making nested inputs flattens
"""
flatten_inputs = []
for input_data in inputs:
if input_data is None:
continue
if isinstance(input_data, (list, tuple)):
flatten_inputs.extend(flattenize_inputs(input_data))
else:
flatten_inputs.append(input_data)
return flatten_inputs
def cleanup_torchscript_cache():
"""
Helper for removing cached model representation
"""
torch._C._jit_clear_class_registry()
torch.jit._recursive.concrete_type_store = torch.jit._recursive.ConcreteTypeStore()
torch.jit._state._clear_class_state()
def postprocess_converted_model(
ov_model,
example_input=None,
input_names=None,
output_names=None,
dynamic_shapes=None,
):
"""
Helper function for appling postprocessing on converted model with updating input names, shapes and output names
acording to requested specification
"""
flatten_example_inputs = flattenize_inputs(example_input) if example_input else []
if input_names:
for inp_name, m_input, input_data in zip(input_names, ov_model.inputs, flatten_example_inputs):
input_node = m_input.get_node()
if input_node.element_type == ov.Type.dynamic:
m_input.get_node().set_element_type(ov.Type.f32)
shape = list(input_data.shape)
if dynamic_shapes is not None and inp_name in dynamic_shapes:
for k in dynamic_shapes[inp_name]:
shape[k] = -1
input_node.set_partial_shape(ov.PartialShape(shape))
m_input.get_tensor().set_names({inp_name})
if output_names:
for out, out_name in zip(ov_model.outputs, output_names):
out.get_tensor().set_names({out_name})
ov_model.validate_nodes_and_infer_types()
return ov_model
INFO:nncf:NNCF initialized successfully.Supported frameworks detected: torch, tensorflow, onnx, openvino
if compression_mode.value == "INT4":
ov_out_path = Path("ov_nanollava/INT4_compressed_weights")
llava_wc_parameters = dict(mode=nncf.CompressWeightsMode.INT4_ASYM, group_size=128, ratio=0.8)
else:
ov_out_path = Path("ov_nanollava/INT8_compressed_weights")
llava_wc_parameters = dict(mode=nncf.CompressWeightsMode.INT8)
image_encoder_wc_parameters = dict(mode=nncf.CompressWeightsMode.INT8)
ov_out_path.mkdir(exist_ok=True, parents=True)
model.config.save_pretrained(ov_out_path)
vision_tower = model.get_vision_tower()
if not vision_tower.is_loaded:
vision_tower.load_model()
image_encoder_path = ov_out_path / "image_encoder.xml"
token_embedding_model_path = ov_out_path / "token_embed.xml"
model_path = ov_out_path / "llava_with_past.xml"
model.eval()
model.config.use_cache = True
model.config.torchscript = True
画像エンコーダー#
画像エンコーダーは、事前トレーニング済みの SigLIP モデルによって nanoLLaVA で表現されます。入力画像を埋め込み空間にエンコードする画像エンコーダー。
if not image_encoder_path.exists():
model.forward = model.encode_images
with torch.no_grad():
ov_model = ov.convert_model(
model,
example_input=torch.zeros((1, 3, 384, 384)),
input=[(-1, 3, 384, 384)],
)
if image_encoder_wc_parameters is not None:
print("Applying weight compression to image encoder")
ov_model = nncf.compress_weights(ov_model, **image_encoder_wc_parameters)
ov.save_model(ov_model, image_encoder_path)
cleanup_torchscript_cache()
del ov_model
gc.collect()
print("Image Encoder model successfully converted")
WARNING:tensorflow:Please fix your imports.Module tensorflow.python.training.tracking.base has been moved to tensorflow.python.trackable.base.The old module will be deleted in version 2.11.
[ WARNING ] Please fix your imports.Module %s has been moved to %s. The old module will be deleted in version %s. huggingface/tokenizers: The current process just got forked, after parallelism has already been used.Disabling parallelism to avoid deadlocks...To disable this warning, you can either: - Avoid using tokenizers before the fork if possible - Explicitly set the environment variable TOKENIZERS_PARALLELISM=(true | false)
['images'] Applying weight compression to image encoder
INFO:nncf:Statistics of the bitwidth distribution:
┍━━━━━━━━━━━━━━━━┯━━━━━━━━━━━━━━━━━━━━━━━━━━━━━┯━━━━━━━━━━━━━━━━━━━━━━━━━━━━━━━━━━━━━━━━┑
│ Num bits (N) │ % all parameters (layers) │ % ratio-defining parameters (layers) │
┝━━━━━━━━━━━━━━━━┿━━━━━━━━━━━━━━━━━━━━━━━━━━━━━┿━━━━━━━━━━━━━━━━━━━━━━━━━━━━━━━━━━━━━━━━┥
│ 8 │ 100% (159 / 159) │ 100% (159 / 159) │
┕━━━━━━━━━━━━━━━━┷━━━━━━━━━━━━━━━━━━━━━━━━━━━━━┷━━━━━━━━━━━━━━━━━━━━━━━━━━━━━━━━━━━━━━━━┙
Output()
Image Encoder model successfully converted
テキスト埋め込み#
LLM では、入力埋め込みは言語モデルの一部ですが、LLaVA の場合、このモデル部分によって生成される最初のステップの隠し状態は、画像埋め込みと共通の埋め込み空間に統合される必要があります。このモデル部分を再利用し、余計な llm モデル・インスタンスの導入を回避するため、個別に使用します。
if not token_embedding_model_path.exists():
with torch.no_grad():
ov_model = ov.convert_model(model.get_model().embed_tokens, example_input=torch.ones((1, 10), dtype=torch.long))
ov.save_model(ov_model, token_embedding_model_path)
cleanup_torchscript_cache()
del ov_model
gc.collect()
print("Token Embedding model successfully converted")
['input']
Token Embedding model successfully converted
言語モデル#
言語モデルは、LLaVA での回答の生成を行います。この部分は、テキスト生成の標準 LLM と非常によく似ています。ここでのモデルでは、ベース LLM として Qwen/Qwen1.5-0.5B を使用します。生成プロセスを最適化し、メモリーをさらに効率良く使用するため、HuggingFace トランスフォーマー API は、入力と出力で use_cache=True
パラメーターと past_key_values
引数を使用してモデル状態を外部にキャッシュするメカニズムを提供します。キャッシュを使用すると、モデルは計算後に非表示の状態を保存します。モデルは各タイムステップで最後に生成された出力トークンのみを計算し、保存された出力トークンを非表示のトークンに再利用します。これにより、変圧器モデルの生成の複雑さが
if not model_path.exists():
model.forward = super(type(model), model).forward
example_input = {"attention_mask": torch.ones([2, 10], dtype=torch.int64), "position_ids": torch.tensor([[8, 9], [8, 9]], dtype=torch.int64)}
dynamic_shapes = {
"input_embeds": {0: "batch_size", 1: "seq_len"},
"attention_mask": {0: "batch_size", 1: "prev_seq_len + seq_len"},
"position_ids": {0: "batch_size", 1: "seq_len"},
}
input_embeds = torch.zeros((2, 2, model.config.hidden_size))
input_names = ["attention_mask", "position_ids"]
output_names = ["logits"]
past_key_values = []
for i in range(model.config.num_hidden_layers):
kv = [torch.randn([2, model.config.num_key_value_heads, 8, model.config.hidden_size // model.config.num_attention_heads]) for _ in range(2)]
past_key_values.append(kv)
input_names.extend([f"past_key_values.{i}.key", f"past_key_values.{i}.value"])
output_names.extend([f"present.{i}.key", f"present.{i}.value"])
dynamic_shapes[input_names[-2]] = {0: "batch_size", 2: "seq_len"}
dynamic_shapes[input_names[-1]] = {0: "batch_size", 2: "seq_len"}
example_input["past_key_values"] = past_key_values
example_input["inputs_embeds"] = input_embeds
input_names.append("inputs_embeds")
dynamic_shapes["inputs_embeds"] = {0: "batch_size", 1: "seq_len"}
ov_model = ov.convert_model(model, example_input=example_input)
ov_model = postprocess_converted_model(
ov_model, example_input=example_input.values(), input_names=input_names, output_names=output_names, dynamic_shapes=dynamic_shapes
)
if llava_wc_parameters is not None:
print("Applying weight compression to second stage LLava model")
ov_model = nncf.compress_weights(ov_model, **llava_wc_parameters)
ov.save_model(ov_model, model_path)
cleanup_torchscript_cache()
del ov_model
gc.collect()
print("LLaVA model successfully converted")
del model
gc.collect();
['attention_mask', 'position_ids', 'past_key_values', 'inputs_embeds']
Applying weight compression to second stage LLava model
Output()
INFO:nncf:Statistics of the bitwidth distribution:
┍━━━━━━━━━━━━━━━━┯━━━━━━━━━━━━━━━━━━━━━━━━━━━━━┯━━━━━━━━━━━━━━━━━━━━━━━━━━━━━━━━━━━━━━━━┑
│ Num bits (N) │ % all parameters (layers) │ % ratio-defining parameters (layers) │
┝━━━━━━━━━━━━━━━━┿━━━━━━━━━━━━━━━━━━━━━━━━━━━━━┿━━━━━━━━━━━━━━━━━━━━━━━━━━━━━━━━━━━━━━━━┥
│ 8 │ 47% (48 / 169) │ 20% (47 / 168) │
├────────────────┼─────────────────────────────┼────────────────────────────────────────┤
│ 4 │ 53% (121 / 169) │ 80% (121 / 168) │
┕━━━━━━━━━━━━━━━━┷━━━━━━━━━━━━━━━━━━━━━━━━━━━━━┷━━━━━━━━━━━━━━━━━━━━━━━━━━━━━━━━━━━━━━━━┙
Output()
LLaVA model successfully converted
モデル推論パイプラインの準備#
クラスは、生成シナリオでモデルを使用するのに使いやすいインターフェイスを提供します。これは、HuggingFace Transformers ライブラリーに実装されている生成のすべてのリーチ機能を再利用する可能性をもたらす、transformers.generation.GenerationMixin
に基づいています。このインターフェイスの詳細については、HuggingFace のドキュメントを参照してください。
from transformers.generation import GenerationConfig, GenerationMixin
from transformers.modeling_outputs import CausalLMOutputWithPast
from transformers import AutoConfig
from transformers.image_processing_utils import BatchFeature, get_size_dict
from transformers.image_transforms import (
convert_to_rgb,
normalize,
rescale,
resize,
to_channel_dimension_format,
)
from transformers.image_utils import (
ChannelDimension,
PILImageResampling,
to_numpy_array,
)
import numpy as np
import torch
from typing import Dict
from functools import partial, reduce
IGNORE_INDEX = -100
IMAGE_TOKEN_INDEX = -200
class ImageProcessor:
def __init__(
self,
image_mean=(0.5, 0.5, 0.5),
image_std=(0.5, 0.5, 0.5),
size=(384, 384),
crop_size: Dict[str, int] = None,
resample=PILImageResampling.BICUBIC,
rescale_factor=1 / 255,
data_format=ChannelDimension.FIRST,
):
crop_size = crop_size if crop_size is not None else {"height": 384, "width": 384}
crop_size = get_size_dict(crop_size, default_to_square=True, param_name="crop_size")
self.image_mean = image_mean
self.image_std = image_std
self.size = size
self.resample = resample
self.rescale_factor = rescale_factor
self.data_format = data_format
self.crop_size = crop_size
def preprocess(self, images, return_tensors):
if isinstance(images, Image.Image):
images = [images]
else:
assert isinstance(images, list)
transforms = [
convert_to_rgb,
to_numpy_array,
partial(resize, size=self.size, resample=self.resample, data_format=self.data_format),
partial(rescale, scale=self.rescale_factor, data_format=self.data_format),
partial(normalize, mean=self.image_mean, std=self.image_std, data_format=self.data_format),
partial(to_channel_dimension_format, channel_dim=self.data_format, input_channel_dim=self.data_format),
]
images = reduce(lambda x, f: [*map(f, x)], transforms, images)
data = {"pixel_values": images}
return BatchFeature(data=data, tensor_type=return_tensors)
class OVLlavaQwen2ForCausalLM(GenerationMixin):
def __init__(self, core, model_dir, device):
self.image_encoder = core.compile_model(model_dir / "image_encoder.xml", device)
self.embed_tokens = core.compile_model(model_dir / "token_embed.xml", device)
self.model = core.read_model(model_dir / "llava_with_past.xml")
self.input_names = {key.get_any_name(): idx for idx, key in enumerate(self.model.inputs)}
self.output_names = {key.get_any_name(): idx for idx, key in enumerate(self.model.outputs)}
self.key_value_input_names = [key for key in self.input_names if "key_values" in key]
self.key_value_output_names = [key for key in self.output_names if "present" in key]
compiled_model = core.compile_model(self.model, device)
self.request = compiled_model.create_infer_request()
self.config = AutoConfig.from_pretrained(model_dir)
self.generation_config = GenerationConfig.from_model_config(self.config)
self.main_input_name = "input_ids"
self.device = torch.device("cpu")
self.num_pkv = 2
self.image_processor = ImageProcessor()
self._supports_cache_class = False
def can_generate(self):
"""Returns True to validate the check that the model using `GenerationMixin.generate()` can indeed generate."""
return True
def __call__(
self,
input_ids: torch.LongTensor,
images: torch.Tensor,
attention_mask: Optional[torch.LongTensor] = None,
position_ids: Optional[torch.LongTensor] = None,
past_key_values: Optional[Tuple[Tuple[torch.FloatTensor]]] = None,
**kwargs,
) -> CausalLMOutputWithPast:
return self.forward(input_ids, images, attention_mask, position_ids, past_key_values)
def forward(
self,
input_ids: torch.LongTensor,
images: torch.Tensor,
attention_mask: Optional[torch.LongTensor] = None,
position_ids: Optional[torch.LongTensor] = None,
past_key_values: Optional[Tuple[Tuple[torch.FloatTensor]]] = None,
**kwargs,
) -> CausalLMOutputWithPast:
"""General inference method"""
inputs = self.prepare_inputs_for_multimodal(input_ids, position_ids, attention_mask, past_key_values, images)
# Run inference
self.request.start_async(inputs, share_inputs=True)
self.request.wait()
logits = torch.from_numpy(self.request.get_tensor("logits").data)
# 長さが等しいタプル: レイヤー数 * デコーダーレイヤーごとの past_key_value の数 (2 は自己注意レイヤーに対応)
past_key_values = tuple(self.request.get_tensor(key).data for key in self.key_value_output_names)
# 長さが `n_layers` のタプルのタプル。各タプルの長さは 2 (自己注意の k/v) に等しい
past_key_values = tuple(past_key_values[i : i + self.num_pkv] for i in range(0, len(past_key_values), self.num_pkv))
return CausalLMOutputWithPast(logits=logits, past_key_values=past_key_values)
def prepare_inputs_for_multimodal(self, input_ids, position_ids, attention_mask, past_key_values, images):
inputs = {}
if past_key_values is None:
past_key_values = self._dummy_past_key_values(input_ids.shape[0])
else:
past_key_values = tuple(past_key_value for pkv_per_layer in past_key_values for past_key_value in pkv_per_layer)
inputs.update(zip(self.key_value_input_names, past_key_values))
if images is None or input_ids.shape[1] == 1:
target_shape = past_key_values[-1][-1].shape[-2] + 1 if past_key_values is not None else input_ids.shape[1]
attention_mask = torch.cat(
(
attention_mask,
torch.ones((attention_mask.shape[0], target_shape - attention_mask.shape[1]), dtype=attention_mask.dtype, device=attention_mask.device),
),
dim=1,
)
position_ids = torch.sum(attention_mask, dim=1).unsqueeze(-1) - 1
inputs_embeds = self.embed_tokens(input_ids)[0]
inputs["attention_mask"] = attention_mask.numpy()
inputs["position_ids"] = position_ids.numpy()
inputs["inputs_embeds"] = inputs_embeds
return inputs
if type(images) is list or images.ndim == 5:
concat_images = torch.cat([image for image in images], dim=0)
image_features = self.encode_images(concat_images)
split_sizes = [image.shape[0] for image in images]
image_features = torch.split(image_features, split_sizes, dim=0)
image_features = [x.flatten(0, 1).to(self.device) for x in image_features]
else:
image_features = self.encode_images(images).to(self.device)
# ダミーテンソルが存在しない場合は追加するだけにします
# 常に None を処理するのは面倒です
# しかし、これは理想的ではありません。良いアイデアがある場合は、
# 問題を提起するか PR を送信してください。お願いします。
labels = None
_attention_mask = attention_mask
if attention_mask is None:
attention_mask = torch.ones_like(input_ids, dtype=torch.bool)
else:
attention_mask = attention_mask.bool()
if position_ids is None:
position_ids = torch.arange(0, input_ids.shape[1], dtype=torch.long, device=input_ids.device)
if labels is None:
labels = torch.full_like(input_ids, IGNORE_INDEX)
# attention_mask を使用してパディングを削除 -- TODO: 再確認
input_ids = [cur_input_ids[cur_attention_mask] for cur_input_ids, cur_attention_mask in zip(input_ids, attention_mask)]
labels = [cur_labels[cur_attention_mask] for cur_labels, cur_attention_mask in zip(labels, attention_mask)]
new_input_embeds = []
new_labels = []
cur_image_idx = 0
for batch_idx, cur_input_ids in enumerate(input_ids):
num_images = (cur_input_ids == IMAGE_TOKEN_INDEX).sum()
if num_images == 0:
cur_image_features = image_features[cur_image_idx]
cur_input_embeds_1 = self.embed_tokens(cur_input_ids)
cur_input_embeds = torch.cat([cur_input_embeds_1, cur_image_features[0:0]], dim=0)
new_input_embeds.append(cur_input_embeds)
new_labels.append(labels[batch_idx])
cur_image_idx += 1
continue
image_token_indices = [-1] + torch.where(cur_input_ids == IMAGE_TOKEN_INDEX)[0].tolist() + [cur_input_ids.shape[0]]
cur_input_ids_noim = []
cur_labels = labels[batch_idx]
cur_labels_noim = []
for i in range(len(image_token_indices) - 1):
cur_input_ids_noim.append(cur_input_ids[image_token_indices[i] + 1 : image_token_indices[i + 1]])
cur_labels_noim.append(cur_labels[image_token_indices[i] + 1 : image_token_indices[i + 1]])
split_sizes = [x.shape[0] for x in cur_labels_noim]
cur_input_embeds = torch.from_numpy(self.embed_tokens(torch.cat(cur_input_ids_noim).unsqueeze(0))[0])[0]
cur_input_embeds_no_im = torch.split(cur_input_embeds, split_sizes, dim=0)
cur_new_input_embeds = []
cur_new_labels = []
for i in range(num_images + 1):
cur_new_input_embeds.append(cur_input_embeds_no_im[i])
cur_new_labels.append(cur_labels_noim[i])
if i < num_images:
cur_image_features = image_features[cur_image_idx]
cur_image_idx += 1
cur_new_input_embeds.append(cur_image_features)
cur_new_labels.append(torch.full((cur_image_features.shape[0],), IGNORE_INDEX, device=cur_labels.device, dtype=cur_labels.dtype))
cur_new_input_embeds = torch.cat(cur_new_input_embeds)
cur_new_labels = torch.cat(cur_new_labels)
new_input_embeds.append(cur_new_input_embeds)
new_labels.append(cur_new_labels)
# 画像の埋め込みによりシーケンスが長くなる可能性があるため、シーケンスを最大長に切り捨て
tokenizer_model_max_length = getattr(self.config, "tokenizer_model_max_length", None)
if tokenizer_model_max_length is not None:
new_input_embeds = [x[:tokenizer_model_max_length] for x in new_input_embeds]
new_labels = [x[:tokenizer_model_max_length] for x in new_labels]
# 組み合わせる
max_len = max(x.shape[0] for x in new_input_embeds)
batch_size = len(new_input_embeds)
new_input_embeds_padded = []
new_labels_padded = torch.full((batch_size, max_len), IGNORE_INDEX, dtype=new_labels[0].dtype, device=new_labels[0].device)
attention_mask = torch.zeros((batch_size, max_len), dtype=attention_mask.dtype, device=attention_mask.device)
position_ids = torch.zeros((batch_size, max_len), dtype=position_ids.dtype, device=position_ids.device)
for i, (cur_new_embed, cur_new_labels) in enumerate(zip(new_input_embeds, new_labels)):
cur_len = cur_new_embed.shape[0]
if getattr(self.config, "tokenizer_padding_side", "right") == "left":
new_input_embeds_padded.append(
torch.cat(
(torch.zeros((max_len - cur_len, cur_new_embed.shape[1]), dtype=cur_new_embed.dtype, device=cur_new_embed.device), cur_new_embed), dim=0
)
)
if cur_len > 0:
new_labels_padded[i, -cur_len:]= cur_new_labels
attention_mask[i, -cur_len:]= True
position_ids[i, -cur_len:]= torch.arange(0, cur_len, dtype=position_ids.dtype, device=position_ids.device)
else:
new_input_embeds_padded.append(
torch.cat(
(cur_new_embed, torch.zeros((max_len - cur_len, cur_new_embed.shape[1]), dtype=cur_new_embed.dtype, device=cur_new_embed.device)), dim=0
)
)
if cur_len > 0:
new_labels_padded[i, :cur_len] = cur_new_labels
attention_mask[i, :cur_len] = True
position_ids[i, :cur_len] = torch.arange(0, cur_len, dtype=position_ids.dtype, device=position_ids.device)
new_input_embeds = torch.stack(new_input_embeds_padded, dim=0)
attention_mask = attention_mask.to(dtype=_attention_mask.dtype)
inputs["inputs_embeds"] = new_input_embeds.numpy()
inputs["attention_mask"] = attention_mask.numpy()
inputs["position_ids"] = position_ids.numpy()
return inputs
def prepare_inputs_for_generation(self, input_ids, past_key_values=None, **kwargs):
"""
This function is used during running GenerationMixin.generate for preparing model specific inputs for
each generation step
"""
past_len = 0
if past_key_values is not None:
input_ids = input_ids[:, -1].unsqueeze(-1)
past_len = past_key_values[-1][-1].shape[-2]
attention_mask = kwargs.get(
"attention_mask",
torch.ones(input_ids.shape[0], input_ids.shape[1] + past_len),
)
return {
"input_ids": input_ids,
"attention_mask": attention_mask,
"position_ids": kwargs.get("position_ids", None),
"past_key_values": past_key_values,
"images": kwargs.get("images", None),
}
def _reorder_cache(self, past_key_values: Tuple[Tuple[torch.Tensor]], beam_idx: torch.Tensor) -> Tuple[Tuple[torch.Tensor]]:
"""
This function is used to re-order the `past_key_values` cache if [`~PreTrainedModel.beam_search`] or
[`~PreTrainedModel.beam_sample`] is called.
This is required to match `past_key_values` with the correct beam_idx at every generation step.
"""
# from transformers.models.gpt2.modeling_gpt2.GPT2LMHeadModel._reorder_cache
return tuple(tuple(np.take(past_state, beam_idx, 0) for past_state in layer_past) for layer_past in past_key_values)
def _dummy_past_key_values(self, batch_size):
pkv = []
for input_name in self.key_value_input_names:
input_t = self.model.input(input_name)
input_shape = self.model.input(input_name).get_partial_shape()
input_shape[0] = batch_size
input_shape[2] = 0
pkv.append(ov.Tensor(input_t.get_element_type(), input_shape.get_shape()))
return pkv
def encode_images(self, images):
return torch.from_numpy(self.image_encoder(images)[0])
def expand2square(self, pil_img, background_color):
width, height = pil_img.size
if width == height:
return pil_img
elif width > height:
result = Image.new(pil_img.mode, (width, width), background_color)
result.paste(pil_img, (0, (width - height) // 2)) return result
else:
result = Image.new(pil_img.mode, (height, height), background_color)
result.paste(pil_img, ((height - width) // 2, 0))
return result
def process_images(self, images, model_cfg):
image_aspect_ratio = getattr(model_cfg, "image_aspect_ratio", None)
new_images = []
if image_aspect_ratio == "pad":
for image in images:
image = self.expand2square(image, tuple(int(x * 255) for x in self.image_processor.image_mean))
image = self.image_processor.preprocess(image, return_tensors="pt")["pixel_values"][0]
new_images.append(image)
else:
return self.image_processor(images, return_tensors="pt")["pixel_values"]
if all(x.shape == new_images[0].shape for x in new_images):
new_images = torch.stack(new_images, dim=0)
return new_images
OpenVINO モデル推論を実行#
import ipywidgets as widgets
core = ov.Core()
support_devices = core.available_devices
if "NPU" in support_devices:
support_devices.remove("NPU")
device = widgets.Dropdown(
options=support_devices + ["AUTO"],
value="AUTO",
description="Device:",
disabled=False,
)
device
Dropdown(description='Device:', index=1, options=('CPU', 'AUTO'), value='AUTO')
ov_model = OVLlavaQwen2ForCausalLM(core, ov_out_path, device.value)
streamer = TextStreamer(tokenizer, skip_prompt=True, skip_special_tokens=True)
output_ids = ov_model.generate(input_ids, images=image_tensor, max_new_tokens=128, use_cache=True, streamer=streamer)
The image features a white, fluffy lamb with a playful expression. The lamb is positioned in the center of the image, and it appears to be in motion, as if it's running. The lamb's fur is fluffy and white, and it has a cute, adorable appearance. The lamb's eyes are wide open, and it has a big, black nose. The lamb's ears are also visible, and it has a cute, adorable expression. The lamb's mouth is open, and it seems to be smiling. The lamb's legs are also visible, and it appears to be in motion, as if it's running. The lamb
インタラクティブなデモ#
import gradio as gr
import time
from transformers import TextIteratorStreamer, StoppingCriteria
from threading import Thread
import requests
example_image_urls = [
(
"https://github.com/openvinotoolkit/openvino_notebooks/assets/29454499/1d6a0188-5613-418d-a1fd-4560aae1d907",
"bee.jpg",
),
(
"https://github.com/openvinotoolkit/openvino_notebooks/assets/29454499/6cc7feeb-0721-4b5d-8791-2576ed9d2863",
"baklava.png",
),
(
"https://github.com/openvinotoolkit/openvino_notebooks/assets/29454499/dd5105d6-6a64-4935-8a34-3058a82c8d5d",
"small.png"
),
(
"https://github.com/openvinotoolkit/openvino_notebooks/assets/29454499/1221e2a8-a6da-413a-9af6-f04d56af3754",
"chart.png"
),
]
for url, file_name in example_image_urls:
if not Path(file_name).exists(): Image.open(requests.get(url, stream=True).raw).save(file_name)
class KeywordsStoppingCriteria(StoppingCriteria):
def __init__(self, keywords, tokenizer, input_ids):
self.keywords = keywords
self.keyword_ids = []
self.max_keyword_len = 0
for keyword in keywords:
cur_keyword_ids = tokenizer(keyword).input_ids
if len(cur_keyword_ids) > 1 and cur_keyword_ids[0] == tokenizer.bos_token_id:
cur_keyword_ids = cur_keyword_ids[1:]
if len(cur_keyword_ids) > self.max_keyword_len:
self.max_keyword_len = len(cur_keyword_ids)
self.keyword_ids.append(torch.tensor(cur_keyword_ids))
self.tokenizer = tokenizer self.start_len = input_ids.shape[1]
def call_for_batch(self, output_ids: torch.LongTensor, scores: torch.FloatTensor, **kwargs) -> bool:
offset = min(output_ids.shape[1] - self.start_len, self.max_keyword_len)
self.keyword_ids = [keyword_id.to(output_ids.device)
for keyword_id in self.keyword_ids] for keyword_id in self.keyword_ids:
truncated_output_ids = output_ids[0, -keyword_id.shape[0] :]
if torch.equal(truncated_output_ids, keyword_id):
return True
outputs = self.tokenizer.batch_decode(output_ids[:, -offset:], skip_special_tokens=True)[0]
for keyword in self.keywords:
if keyword in outputs:
return True
return False
def __call__(self, output_ids: torch.LongTensor, scores: torch.FloatTensor, **kwargs) -> bool:
outputs = []
for i in range(output_ids.shape[0]):
outputs.append(self.call_for_batch(output_ids[i].unsqueeze(0), scores))
return all(outputs)
def bot_streaming(message, history):
messages = []
if message["files"]:
image = message["files"][-1]["path"] if isinstance(message["files"][-1], dict) else message["files"][-1]
else:
for _, hist in enumerate(history):
if isinstance(hist[0], tuple):
image = hist[0][0]
if len(history) > 0 and image is not None:
messages.append({"role": "user", "content": f"<image>\n{history[1][0]}"})
messages.append({"role": "assistant", "content": history[1][1]})
for human, assistant in history[2:]:
if assistant is None:
continue
messages.append({"role": "user", "content": human})
messages.append({"role": "assistant", "content": assistant})
messages.append({"role": "user", "content": message["text"]})
elif len(history) > 0 and image is None:
for human, assistant in history:
if assistant is None:
continue
messages.append({"role": "user", "content": human})
messages.append({"role": "assistant", "content": assistant})
messages.append({"role": "user", "content": message["text"]})
elif len(history) == 0 and image is not None:
messages.append({"role": "user", "content": f"<image>\n{message['text']}"})
elif len(history) == 0 and image is None:
messages.append({"role": "user", "content": message["text"]})
print(messages)
image = Image.open(image).convert("RGB")
text = tokenizer.apply_chat_template(messages, tokenize=False, add_generation_prompt=True)
text_chunks = [tokenizer(chunk).input_ids for chunk in text.split("<image>")]
input_ids = torch.tensor(text_chunks[0] + [-200] + text_chunks[1], dtype=torch.long).unsqueeze(0)
stop_str = "<|im_end|>"
keywords = [stop_str]
stopping_criteria = KeywordsStoppingCriteria(keywords, tokenizer, input_ids)
streamer = TextIteratorStreamer(tokenizer, skip_prompt=True, skip_special_tokens=True)
image_tensor = ov_model.process_images([image], ov_model.config)
generation_kwargs = dict(
input_ids=input_ids, images=image_tensor, streamer=streamer, max_new_tokens=128, stopping_criteria=[stopping_criteria], temperature=0.01
)
thread = Thread(target=ov_model.generate, kwargs=generation_kwargs)
thread.start()
buffer = ""
for new_text in streamer:
buffer += new_text
generated_text_without_prompt = buffer[:]
time.sleep(0.04)
yield generated_text_without_prompt
demo = gr.ChatInterface(
fn=bot_streaming,
title="🚀nanoLLaVA",
examples=[
{"text": "What is on the flower?", "files": ["./bee.jpg"]},
{"text": "How to make this pastry?", "files": ["./baklava.png"]},
{"text": "What is the text saying?", "files": ["./small.png"]},
{"text": "What does the chart display?", "files": ["./chart.png"]},
],
description="Try [nanoLLaVA](https://huggingface.co/qnguyen3/nanoLLaVA) using OpenVINO in this demo.Upload an image and start chatting about it, or simply try one of the examples below.If you don't upload an image, you will receive an error.",
stop_btn="Stop Generation",
multimodal=True,
)
# リモートで起動する場合は、server_name と server_port を指定
# demo.launch(server_name='your server name', server_port='server port in int')
# 詳細については、ドキュメントをご覧ください: https://gradio.app/docs/
try:
demo.launch(debug=False)
except Exception:
demo.launch(share=True, debug=False)
ローカル URL で実行中: http://127.0.0.1:7860 パブリックリンクを作成するには、launch() で share=True を設定します。