pix2pix-turbo と OpenVINO を使用したワンステップのスケッチから画像への変換#
この Jupyter ノートブックは、ローカルへのインストール後にのみ起動できます。
拡散モデルは画像生成において顕著な結果を達成します。ユーザーの指示に従って高品質の画像を合成できます。同時に、大部分の拡散ベースの画像生成アプローチは、反復的なノイズ除去プロセスに時間がかかります。Pix2Pix-turbo モデルは、画像から画像への変換タスクの拡散プロセスの速度を解決するため、One-Step Image Translation with Text-to-Image Models paper (英語) 論文で提案されました。SD-Turbo は、単一のネットワーク評価でテキストプロンプトから写実的な画像を合成できる、高速な生成テキストから画像へのモデルです。pix2pix-turbo は、単一の推論のみを使用して、Sketch2Photo の ControlNet や 50 ステップの Edge2Image など最近の成果と同等の品質を実現します。
このチュートリアルでは、Pix2Pix-Turbo と OpenVINO を使用してスケッチを画像に変換する方法を学習します。
目次:
必要条件#
モデル・リポジトリーのクローンを作成して必要なパッケージをインストールします。
%pip install -q "openvino>=2024.1.0" "torch>=2.1" torchvision "diffusers==0.25.1" "peft==0.6.2" transformers tqdm pillow opencv-python "gradio==3.43.1" --extra-index-url https://download.pytorch.org/whl/cpu
Note: you may need to restart the kernel to use updated packages.
from pathlib import Path
repo_dir = Path("img2img-turbo")
if not repo_dir.exists():
!git clone https://github.com/GaParmar/img2img-turbo.git
pix2pix_turbo_py_path = repo_dir / "src/pix2pix_turbo.py"
model_py_path = repo_dir / "src/model.py"
orig_pix2pix_turbo_path = pix2pix_turbo_py_path.parent / ("orig_" + pix2pix_turbo_py_path.name)
orig_model_py_path = model_py_path.parent / ("orig_" + model_py_path.name)
if not orig_pix2pix_turbo_path.exists():
pix2pix_turbo_py_path.rename(orig_pix2pix_turbo_path)
with orig_pix2pix_turbo_path.open("r") as f:
data = f.read()
data = data.replace("cuda", "cpu")
with pix2pix_turbo_py_path.open("w") as out_f:
out_f.write(data)
if not orig_model_py_path.exists():
model_py_path.rename(orig_model_py_path)
with orig_model_py_path.open("r") as f:
data = f.read()
data = data.replace("cuda", "cpu")
with model_py_path.open("w") as out_f:
out_f.write(data)
%cd $repo_dir
Cloning into 'img2img-turbo'...
remote: Enumerating objects: 205, done.[K
remote: Counting objects: 100% (70/70), done.[K
remote: Compressing objects: 100% (26/26), done.[K
remote: Total 205 (delta 53), reused 46 (delta 44), pack-reused 135[K
Receiving objects: 100% (205/205), 31.89 MiB | 19.13 MiB/s, done.
Resolving deltas: 100% (96/96), done.
/opt/home/k8sworker/ci-ai/cibuilds/ov-notebook/OVNotebookOps-727/.workspace/scm/ov-notebook/notebooks/sketch-to-image-pix2pix-turbo/img2img-turbo
PyTorch モデルのロード#
Pix2Pix-turbo アーキテクチャーを下の図に示します。このモデルは、元の潜在拡散モデルの 3 つの個別のモジュールを、小さなトレーニング可能な重みを持つ単一のエンドツーエンド・ネットワークに結合します。このアーキテクチャーにより、入力シーンの構造を保持しながら、入力画像 x を出力 y に変換できます。ここでは各モジュールで LoRA アダプターを使用し、入力と出力間にスキップ接続とゼロ畳み込みを導入し、U-Net の最初のレイヤーを再トレーニングします。図の青いボックスはトレーニング可能なレイヤーを示します。半透明のレイヤーはフリーズされます。
import requests
import copy
from tqdm import tqdm
import torch
from transformers import AutoTokenizer, CLIPTextModel
from diffusers import AutoencoderKL, UNet2DConditionModel
from diffusers.models.autoencoders.vae import DiagonalGaussianDistribution
from diffusers.utils.peft_utils import set_weights_and_activate_adapters
from peft import LoraConfig
import types
from src.model import make_1step_sched
from src.pix2pix_turbo import TwinConv
tokenizer = AutoTokenizer.from_pretrained("stabilityai/sd-turbo", subfolder="tokenizer")
def tokenize_prompt(prompt):
caption_tokens = tokenizer(prompt, max_length=tokenizer.model_max_length, padding="max_length", truncation=True, return_tensors="pt").input_ids
return caption_tokens
def _vae_encoder_fwd(self, sample):
sample = self.conv_in(sample)
l_blocks = []
# ダウン
for down_block in self.down_blocks:
l_blocks.append(sample)
sample = down_block(sample)
# ミドル
sample = self.mid_block(sample)
sample = self.conv_norm_out(sample)
sample = self.conv_act(sample)
sample = self.conv_out(sample)
current_down_blocks = l_blocks
return sample, current_down_blocks
def _vae_decoder_fwd(self, sample, incoming_skip_acts, latent_embeds=None):
sample = self.conv_in(sample)
upscale_dtype = next(iter(self.up_blocks.parameters())).dtype
# ミドル
sample = self.mid_block(sample, latent_embeds)
sample = sample.to(upscale_dtype)
if not self.ignore_skip:
skip_convs = [self.skip_conv_1, self.skip_conv_2, self.skip_conv_3, self.skip_conv_4]
# アップ
for idx, up_block in enumerate(self.up_blocks):
skip_in = skip_convs[idx](incoming_skip_acts[::-1][idx] * self.gamma)
# スキップを追加
sample = sample + skip_in
sample = up_block(sample, latent_embeds)
else:
for idx, up_block in enumerate(self.up_blocks):
sample = up_block(sample, latent_embeds)
# 後処理
if latent_embeds is None:
sample = self.conv_norm_out(sample)
else:
sample = self.conv_norm_out(sample, latent_embeds)
sample = self.conv_act(sample)
sample = self.conv_out(sample)
return sample
def vae_encode(self, x: torch.FloatTensor):
"""
Encode a batch of images into latents.
Args:
x (`torch.FloatTensor`): Input batch of images.
Returns:
The latent representations of the encoded images.If `return_dict` is True, a
[`~models.autoencoder_kl.AutoencoderKLOutput`] is returned, otherwise a plain `tuple` is returned.
"""
h, down_blocks = self.encoder(x)
moments = self.quant_conv(h)
posterior = DiagonalGaussianDistribution(moments)
return (posterior, down_blocks)
def vae_decode(self, z: torch.FloatTensor, skip_acts):
decoded = self._decode(z, skip_acts)[0]
return (decoded,)
def vae__decode(self, z: torch.FloatTensor, skip_acts):
z = self.post_quant_conv(z)
dec = self.decoder(z, skip_acts)
return (dec,)
class Pix2PixTurbo(torch.nn.Module):
def __init__(self, pretrained_name=None, pretrained_path=None, ckpt_folder="checkpoints", lora_rank_unet=8, lora_rank_vae=4):
super().__init__()
self.text_encoder = CLIPTextModel.from_pretrained("stabilityai/sd-turbo", subfolder="text_encoder").cpu()
self.sched = make_1step_sched()
vae = AutoencoderKL.from_pretrained("stabilityai/sd-turbo", subfolder="vae")
vae.encoder.forward = types.MethodType(_vae_encoder_fwd, vae.encoder)
vae.decoder.forward = types.MethodType(_vae_decoder_fwd, vae.decoder)
vae.encode = types.MethodType(vae_encode, vae)
vae.decode = types.MethodType(vae_decode, vae) vae._decode = types.MethodType(vae__decode, vae)
# スキップ接続変換を追加
vae.decoder.skip_conv_1 = torch.nn.Conv2d(512, 512, kernel_size=(1, 1), stride=(1, 1), bias=False).cpu()
vae.decoder.skip_conv_2 = torch.nn.Conv2d(256, 512, kernel_size=(1, 1), stride=(1, 1), bias=False).cpu()
vae.decoder.skip_conv_3 = torch.nn.Conv2d(128, 512, kernel_size=(1, 1), stride=(1, 1), bias=False).cpu()
vae.decoder.skip_conv_4 = torch.nn.Conv2d(128, 256, kernel_size=(1, 1), stride=(1, 1), bias=False).cpu()
vae.decoder.ignore_skip = False
unet = UNet2DConditionModel.from_pretrained("stabilityai/sd-turbo", subfolder="unet")
ckpt_folder = Path(ckpt_folder)
if pretrained_name == "edge_to_image":
url = "https://www.cs.cmu.edu/~img2img-turbo/models/edge_to_image_loras.pkl"
ckpt_folder.mkdir(exist_ok=True)
outf = ckpt_folder / "edge_to_image_loras.pkl"
if not outf:
print(f"Downloading checkpoint to {outf}")
response = requests.get(url, stream=True)
total_size_in_bytes = int(response.headers.get("content-length", 0))
block_size = 1024 # 1 Kibibyte
progress_bar = tqdm(total=total_size_in_bytes, unit="iB", unit_scale=True)
with open(outf, "wb") as file:
for data in response.iter_content(block_size):
progress_bar.update(len(data))
file.write(data)
progress_bar.close()
if total_size_in_bytes != 0 and progress_bar.n != total_size_in_bytes:
print("ERROR, something went wrong")
print(f"Downloaded successfully to {outf}")
p_ckpt = outf
sd = torch.load(p_ckpt, map_location="cpu")
unet_lora_config = LoraConfig(r=sd["rank_unet"], init_lora_weights="gaussian", target_modules=sd["unet_lora_target_modules"])
vae_lora_config = LoraConfig(r=sd["rank_vae"], init_lora_weights="gaussian", target_modules=sd["vae_lora_target_modules"])
vae.add_adapter(vae_lora_config, adapter_name="vae_skip")
_sd_vae = vae.state_dict()
for k in sd["state_dict_vae"]:
_sd_vae[k] = sd["state_dict_vae"][k]
vae.load_state_dict(_sd_vae)
unet.add_adapter(unet_lora_config)
_sd_unet = unet.state_dict()
for k in sd["state_dict_unet"]:
_sd_unet[k] = sd["state_dict_unet"][k]
unet.load_state_dict(_sd_unet)
elif pretrained_name == "sketch_to_image_stochastic":
# URL からダウンロード
url = "https://www.cs.cmu.edu/~img2img-turbo/models/sketch_to_image_stochastic_lora.pkl"
ckpt_folder.mkdir(exist_ok=True)
outf = ckpt_folder / "sketch_to_image_stochastic_lora.pkl"
if not outf.exists():
print(f"Downloading checkpoint to {outf}")
response = requests.get(url, stream=True)
total_size_in_bytes = int(response.headers.get("content-length", 0))
block_size = 1024 # 1 Kibibyte
progress_bar = tqdm(total=total_size_in_bytes, unit="iB", unit_scale=True)
with open(outf, "wb") as file:
for data in response.iter_content(block_size):
progress_bar.update(len(data))
file.write(data)
progress_bar.close()
if total_size_in_bytes != 0 and progress_bar.n != total_size_in_bytes:
print("ERROR, something went wrong")
print(f"Downloaded successfully to {outf}")
p_ckpt = outf
convin_pretrained = copy.deepcopy(unet.conv_in)
unet.conv_in = TwinConv(convin_pretrained, unet.conv_in)
sd = torch.load(p_ckpt, map_location="cpu")
unet_lora_config = LoraConfig(r=sd["rank_unet"], init_lora_weights="gaussian", target_modules=sd["unet_lora_target_modules"])
vae_lora_config = LoraConfig(r=sd["rank_vae"], init_lora_weights="gaussian", target_modules=sd["vae_lora_target_modules"])
vae.add_adapter(vae_lora_config, adapter_name="vae_skip")
_sd_vae = vae.state_dict()
for k in sd["state_dict_vae"]:
if k not in _sd_vae:
continue
_sd_vae[k] = sd["state_dict_vae"][k]
vae.load_state_dict(_sd_vae)
unet.add_adapter(unet_lora_config)
_sd_unet = unet.state_dict()
for k in sd["state_dict_unet"]:
_sd_unet[k] = sd["state_dict_unet"][k]
unet.load_state_dict(_sd_unet)
elif pretrained_path is not None:
sd = torch.load(pretrained_path, map_location="cpu")
unet_lora_config = LoraConfig(r=sd["rank_unet"], init_lora_weights="gaussian", target_modules=sd["unet_lora_target_modules"])
vae_lora_config = LoraConfig(r=sd["rank_vae"], init_lora_weights="gaussian", target_modules=sd["vae_lora_target_modules"])
vae.add_adapter(vae_lora_config, adapter_name="vae_skip")
_sd_vae = vae.state_dict()
for k in sd["state_dict_vae"]:
_sd_vae[k] = sd["state_dict_vae"][k]
vae.load_state_dict(_sd_vae)
unet.add_adapter(unet_lora_config)
_sd_unet = unet.state_dict()
for k in sd["state_dict_unet"]:
_sd_unet[k] = sd["state_dict_unet"][k]
unet.load_state_dict(_sd_unet)
# unet.enable_xformers_memory_efficient_attention()
unet.to("cpu")
vae.to("cpu")
self.unet, self.vae = unet, vae
self.vae.decoder.gamma = 1
self.timesteps = torch.tensor([999], device="cpu").long()
self.text_encoder.requires_grad_(False)
def set_r(self, r):
self.unet.set_adapters(["default"], weights=[r])
set_weights_and_activate_adapters(self.vae, ["vae_skip"], [r])
self.r = r
self.unet.conv_in.r = r
self.vae.decoder.gamma = r
def forward(self, c_t, prompt_tokens, noise_map):
caption_enc = self.text_encoder(prompt_tokens)[0]
# r 値に基づいて lora の重みをスケール
sample, current_down_blocks = self.vae.encode(c_t)
encoded_control = sample.sample() * self.vae.config.scaling_factor
# 入力とノイズを組み合わせ
unet_input = encoded_control * self.r + noise_map * (1 - self.r)
unet_output = self.unet(
unet_input,
self.timesteps,
encoder_hidden_states=caption_enc,
).sample
x_denoised = self.sched.step(unet_output, self.timesteps, unet_input, return_dict=True).prev_sample
output_image = (self.vae.decode(x_denoised / self.vae.config.scaling_factor, current_down_blocks)[0]).clamp(-1, 1)
return output_image
/opt/home/k8sworker/ci-ai/cibuilds/ov-notebook/OVNotebookOps-727/.workspace/scm/ov-notebook/.venv/lib/python3.8/site-packages/diffusers/utils/outputs.py:63: UserWarning: torch.utils._pytree._register_pytree_node is deprecated.Please use torch.utils._pytree.register_pytree_node instead. torch.utils._pytree._register_pytree_node( The installed version of bitsandbytes was compiled without GPU support.8-bit optimizers, 8-bit multiplication, and GPU quantization are unavailable./opt/home/k8sworker/ci-ai/cibuilds/ov-notebook/OVNotebookOps-727/.workspace/scm/ov-notebook/.venv/lib/python3.8/site-packages/huggingface_hub/file_download.py:1132: FutureWarning: resume_download is deprecated and will be removed in version 1.0.0.Downloads always resume when possible.If you want to force a new download, use force_download=True. warnings.warn(
ov_model_path = Path("model/pix2pix-turbo.xml")
pt_model = None
if not ov_model_path.exists():
pt_model = Pix2PixTurbo("sketch_to_image_stochastic")
pt_model.set_r(0.4)
pt_model.eval()
/opt/home/k8sworker/ci-ai/cibuilds/ov-notebook/OVNotebookOps-727/.workspace/scm/ov-notebook/.venv/lib/python3.8/site-packages/diffusers/utils/outputs.py:63: UserWarning: torch.utils._pytree._register_pytree_node is deprecated.Please use torch.utils._pytree.register_pytree_node instead. torch.utils._pytree._register_pytree_node( /opt/home/k8sworker/ci-ai/cibuilds/ov-notebook/OVNotebookOps-727/.workspace/scm/ov-notebook/.venv/lib/python3.8/site-packages/huggingface_hub/file_download.py:1132: FutureWarning: resume_download is deprecated and will be removed in version 1.0.0.Downloads always resume when possible.If you want to force a new download, use force_download=True. warnings.warn(
Downloading checkpoint to checkpoints/sketch_to_image_stochastic_lora.pkl
100%|██████████| 525M/525M [33:51<00:00, 258kiB/s]
Downloaded successfully to checkpoints/sketch_to_image_stochastic_lora.pkl
PyTorch モデルを OpenVINO 中間表現に変換#
OpenVINO 2023.0 リリース以降、OpenVINO は、高度な OpenVINO 最適化ツールと機能を活用できるように、PyTorch モデルを OpenVINO 中間表現 (IR) 形式に直接変換するのをサポートしています。OpenVINO モデル・トランスフォーメーション API にモデル・オブジェクトとモデルトレースの入力データを提供する必要があります。ov.convert_model
関数は、PyTorch モデル・インスタンスを、デバイス上でコンパイルするために使用したり、ov.save_model
を使用して FP16 形式に圧縮してディスクに保存できる ov.Model
オブジェクトに変換します。
import gc
import openvino as ov
if not ov_model_path.exists():
example_input = [torch.ones((1, 3, 512, 512)), torch.ones([1, 77], dtype=torch.int64), torch.ones([1, 4, 64, 64])]
with torch.no_grad():
ov_model = ov.convert_model(pt_model, example_input=example_input, input=[[1, 3, 512, 512], [1, 77], [1, 4, 64, 64]])
ov.save_model(ov_model, ov_model_path)
del ov_model
torch._C._jit_clear_class_registry()
torch.jit._recursive.concrete_type_store = torch.jit._recursive.ConcreteTypeStore()
torch.jit._state._clear_class_state()
del pt_model
gc.collect();
/opt/home/k8sworker/ci-ai/cibuilds/ov-notebook/OVNotebookOps-727/.workspace/scm/ov-notebook/.venv/lib/python3.8/site-packages/transformers/modeling_utils.py:4371: FutureWarning: _is_quantized_training_enabled is going to be deprecated in transformers 4.39.0.Please use model.hf_quantizer.is_trainable instead warnings.warn( /opt/home/k8sworker/ci-ai/cibuilds/ov-notebook/OVNotebookOps-727/.workspace/scm/ov-notebook/.venv/lib/python3.8/site-packages/transformers/models/distilbert/modeling_distilbert.py:86: TracerWarning: Converting a tensor to a Python boolean might cause the trace to be incorrect.We can't record the data flow of Python values, so this value will be treated as a constant in the future.This means that the trace might not generalize to other inputs! if input_shape[-1] > 1 or self.sliding_window is not None: /opt/home/k8sworker/ci-ai/cibuilds/ov-notebook/OVNotebookOps-727/.workspace/scm/ov-notebook/.venv/lib/python3.8/site-packages/transformers/modeling_attn_mask_utils.py:162: TracerWarning: Converting a tensor to a Python boolean might cause the trace to be incorrect.We can't record the data flow of Python values, so this value will be treated as a constant in the future.This means that the trace might not generalize to other inputs! if past_key_values_length > 0: /opt/home/k8sworker/ci-ai/cibuilds/ov-notebook/OVNotebookOps-727/.workspace/scm/ov-notebook/.venv/lib/python3.8/site-packages/transformers/models/clip/modeling_clip.py:279: TracerWarning: Converting a tensor to a Python boolean might cause the trace to be incorrect.We can't record the data flow of Python values, so this value will be treated as a constant in the future.This means that the trace might not generalize to other inputs! if attn_weights.size() != (bsz * self.num_heads, tgt_len, src_len): /opt/home/k8sworker/ci-ai/cibuilds/ov-notebook/OVNotebookOps-727/.workspace/scm/ov-notebook/.venv/lib/python3.8/site-packages/transformers/models/clip/modeling_clip.py:287: TracerWarning: Converting a tensor to a Python boolean might cause the trace to be incorrect.We can't record the data flow of Python values, so this value will be treated as a constant in the future.This means that the trace might not generalize to other inputs! if causal_attention_mask.size() != (bsz, 1, tgt_len, src_len): /opt/home/k8sworker/ci-ai/cibuilds/ov-notebook/OVNotebookOps-727/.workspace/scm/ov-notebook/.venv/lib/python3.8/site-packages/transformers/models/clip/modeling_clip.py:319: TracerWarning: Converting a tensor to a Python boolean might cause the trace to be incorrect.We can't record the data flow of Python values, so this value will be treated as a constant in the future.This means that the trace might not generalize to other inputs! if attn_output.size() != (bsz * self.num_heads, tgt_len, self.head_dim): /opt/home/k8sworker/ci-ai/cibuilds/ov-notebook/OVNotebookOps-727/.workspace/scm/ov-notebook/.venv/lib/python3.8/site-packages/diffusers/models/downsampling.py:135: TracerWarning: Converting a tensor to a Python boolean might cause the trace to be incorrect.We can't record the data flow of Python values, so this value will be treated as a constant in the future.This means that the trace might not generalize to other inputs! assert hidden_states.shape[1] == self.channels /opt/home/k8sworker/ci-ai/cibuilds/ov-notebook/OVNotebookOps-727/.workspace/scm/ov-notebook/.venv/lib/python3.8/site-packages/diffusers/models/downsampling.py:144: TracerWarning: Converting a tensor to a Python boolean might cause the trace to be incorrect.We can't record the data flow of Python values, so this value will be treated as a constant in the future.This means that the trace might not generalize to other inputs! assert hidden_states.shape[1] == self.channels /opt/home/k8sworker/ci-ai/cibuilds/ov-notebook/OVNotebookOps-727/.workspace/scm/ov-notebook/.venv/lib/python3.8/site-packages/diffusers/models/unet_2d_condition.py:915: TracerWarning: Converting a tensor to a Python boolean might cause the trace to be incorrect.We can't record the data flow of Python values, so this value will be treated as a constant in the future.This means that the trace might not generalize to other inputs! if dim % default_overall_up_factor != 0: /opt/home/k8sworker/ci-ai/cibuilds/ov-notebook/OVNotebookOps-727/.workspace/scm/ov-notebook/.venv/lib/python3.8/site-packages/diffusers/models/upsampling.py:149: TracerWarning: Converting a tensor to a Python boolean might cause the trace to be incorrect.We can't record the data flow of Python values, so this value will be treated as a constant in the future.This means that the trace might not generalize to other inputs! assert hidden_states.shape[1] == self.channels /opt/home/k8sworker/ci-ai/cibuilds/ov-notebook/OVNotebookOps-727/.workspace/scm/ov-notebook/.venv/lib/python3.8/site-packages/diffusers/models/upsampling.py:165: TracerWarning: Converting a tensor to a Python boolean might cause the trace to be incorrect.We can't record the data flow of Python values, so this value will be treated as a constant in the future.This means that the trace might not generalize to other inputs! if hidden_states.shape[0] >= 64: /opt/home/k8sworker/ci-ai/cibuilds/ov-notebook/OVNotebookOps-727/.workspace/scm/ov-notebook/.venv/lib/python3.8/site-packages/diffusers/schedulers/scheduling_ddpm.py:433: TracerWarning: Converting a tensor to a Python boolean might cause the trace to be incorrect.We can't record the data flow of Python values, so this value will be treated as a constant in the future.This means that the trace might not generalize to other inputs! if model_output.shape[1] == sample.shape[1] * 2 and self.variance_type in ["learned", "learned_range"]: /opt/home/k8sworker/ci-ai/cibuilds/ov-notebook/OVNotebookOps-727/.workspace/scm/ov-notebook/.venv/lib/python3.8/site-packages/diffusers/schedulers/scheduling_ddpm.py:440: TracerWarning: Converting a tensor to a Python boolean might cause the trace to be incorrect.We can't record the data flow of Python values, so this value will be treated as a constant in the future.This means that the trace might not generalize to other inputs! alpha_prod_t_prev = self.alphas_cumprod[prev_t] if prev_t >= 0 else self.one /opt/home/k8sworker/ci-ai/cibuilds/ov-notebook/OVNotebookOps-727/.workspace/scm/ov-notebook/.venv/lib/python3.8/site-packages/diffusers/schedulers/scheduling_ddpm.py:479: TracerWarning: Converting a tensor to a Python boolean might cause the trace to be incorrect.We can't record the data flow of Python values, so this value will be treated as a constant in the future.This means that the trace might not generalize to other inputs! if t > 0: /opt/home/k8sworker/ci-ai/cibuilds/ov-notebook/OVNotebookOps-727/.workspace/scm/ov-notebook/.venv/lib/python3.8/site-packages/diffusers/schedulers/scheduling_ddpm.py:330: TracerWarning: Converting a tensor to a Python boolean might cause the trace to be incorrect.We can't record the data flow of Python values, so this value will be treated as a constant in the future.This means that the trace might not generalize to other inputs! alpha_prod_t_prev = self.alphas_cumprod[prev_t] if prev_t >= 0 else self.one /opt/home/k8sworker/ci-ai/cibuilds/ov-notebook/OVNotebookOps-727/.workspace/scm/ov-notebook/.venv/lib/python3.8/site-packages/torch/jit/_trace.py:1116: TracerWarning: Trace had nondeterministic nodes.Did you forget call .eval() on your model? Nodes: %20785 : Float(1, 4, 64, 64, strides=[16384, 4096, 64, 1], requires_grad=0, device=cpu) = aten::randn(%20779, %20780, %20781, %20782, %20783, %20784) # /opt/home/k8sworker/ci-ai/cibuilds/ov-notebook/OVNotebookOps-727/.workspace/scm/ov-notebook/.venv/lib/python3.8/site-packages/diffusers/utils/torch_utils.py:80:0 %35917 : Float(1, 4, 64, 64, strides=[16384, 4096, 64, 1], requires_grad=0, device=cpu) = aten::randn(%35911, %35912, %35913, %35914, %35915, %35916) # /opt/home/k8sworker/ci-ai/cibuilds/ov-notebook/OVNotebookOps-727/.workspace/scm/ov-notebook/.venv/lib/python3.8/site-packages/diffusers/utils/torch_utils.py:80:0 This may cause errors in trace checking.To disable trace checking, pass check_trace=False to torch.jit.trace() _check_trace( /opt/home/k8sworker/ci-ai/cibuilds/ov-notebook/OVNotebookOps-727/.workspace/scm/ov-notebook/.venv/lib/python3.8/site-packages/torch/jit/_trace.py:1116: TracerWarning: Output nr 1. of the traced function does not match the corresponding output of the Python function.Detailed error: Tensor-likes are not close! Mismatched elements: 35 / 786432 (0.0%) Greatest absolute difference: 1.6555190086364746e-05 at index (0, 2, 421, 41) (up to 1e-05 allowed) Greatest relative difference: 7.15815554884626e-05 at index (0, 2, 421, 41) (up to 1e-05 allowed) _check_trace(
['c_t', 'prompt_tokens', 'noise_map']
推論デバイスの選択#
import ipywidgets as widgets
core = ov.Core()
device = widgets.Dropdown(
options=core.available_devices + ["AUTO"],
value="AUTO",
description="Device:",
disabled=False,
)
device
Dropdown(description='Device:', index=1, options=('CPU', 'AUTO'), value='AUTO')
モデルのコンパイル#
compiled_model = core.compile_model(ov_model_path, device.value)
モデルの推論を実行#
それでは、実際にモデルを試して、シンプルな猫のスケッチをプロフェッショナルなアートワークに変えてみます。
from diffusers.utils import load_image
sketch_image =
load_image("https://github.com/openvinotoolkit/openvino_notebooks/assets/29454499/f964a51d-34e8-411a-98f4-5f97a28f56b0")
sketch_image
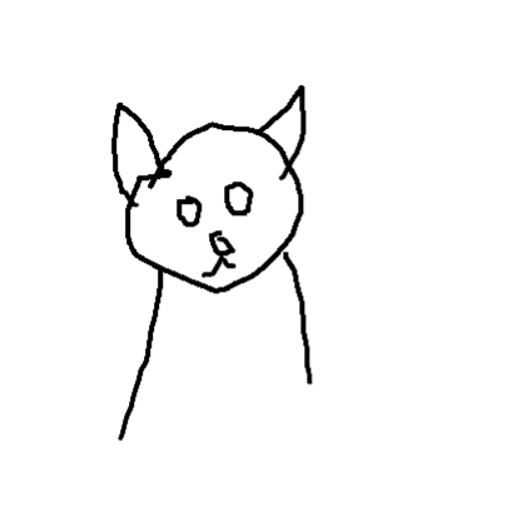
import torchvision.transforms.functional as F
torch.manual_seed(145)
c_t = torch.unsqueeze(F.to_tensor(sketch_image) > 0.5, 0)
noise = torch.randn((1, 4, 512 // 8, 512 // 8))
prompt_template = "anime artwork {prompt} . anime style, key visual, vibrant, studio anime, highly detailed"
prompt = prompt_template.replace("{prompt}", "fluffy magic cat")
prompt_tokens = tokenize_prompt(prompt)
result = compiled_model([1 - c_t.to(torch.float32), prompt_tokens, noise])[0]
from PIL import Image
import numpy as np
image_tensor = (result[0] * 0.5 + 0.5) * 255
image = np.transpose(image_tensor, (1, 2, 0)).astype(np.uint8)
Image.fromarray(image)
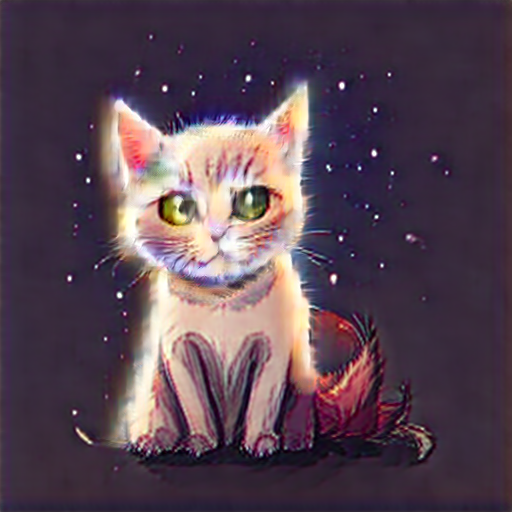
インタラクティブなデモ#
ここでは、自身の絵でモデルを試すことができます。
指示: * テキストプロンプトを入力します (例: cat) * 鉛筆と消しゴムボタンを使用してスケッチを開始します * スタイル・テンプレートを使用して画像のスタイルを変更します * さまざまなシードで結果を生成します * ダウンロード・ボタンを使用して結果をダウンロードします
import random
import base64
from io import BytesIO
import gradio as gr
style_list = [
{
"name": "Cinematic",
"prompt": "cinematic still {prompt} . emotional, harmonious, vignette, highly detailed, high budget, bokeh, cinemascope, moody, epic, gorgeous, film grain, grainy",
},
{
"name": "3D Model",
"prompt": "professional 3d model {prompt} . octane render, highly detailed, volumetric, dramatic lighting",
},
{
"name": "Anime",
"prompt": "anime artwork {prompt} . anime style, key visual, vibrant, studio anime, highly detailed",
},
{
"name": "Digital Art",
"prompt": "concept art {prompt} . digital artwork, illustrative, painterly, matte painting, highly detailed",
},
{
"name": "Photographic",
"prompt": "cinematic photo {prompt} . 35mm photograph, film, bokeh, professional, 4k, highly detailed",
},
{
"name": "Pixel art",
"prompt": "pixel-art {prompt} . low-res, blocky, pixel art style, 8-bit graphics",
},
{
"name": "Fantasy art",
"prompt": "ethereal fantasy concept art of {prompt} . magnificent, celestial, ethereal, painterly, epic, majestic, magical, fantasy art, cover art, dreamy",
},
{
"name": "Neonpunk",
"prompt": "neonpunk style {prompt} . cyberpunk, vaporwave, neon, vibes, vibrant, stunningly beautiful, crisp, detailed, sleek, ultramodern, magenta highlights, dark purple shadows, high contrast, cinematic, ultra detailed, intricate, professional",
},
{
"name": "Manga",
"prompt": "manga style {prompt} . vibrant, high-energy, detailed, iconic, Japanese comic style",
},
]
styles = {k["name"]: k["prompt"] for k in style_list}
STYLE_NAMES = list(styles.keys())
DEFAULT_STYLE_NAME = "Fantasy art"
MAX_SEED = np.iinfo(np.int32).max
def pil_image_to_data_uri(img, format="PNG"):
buffered = BytesIO()
img.save(buffered, format=format)
img_str = base64.b64encode(buffered.getvalue()).decode()
return f"data:image/{format.lower()};base64,{img_str}"
def run(image, prompt, prompt_template, style_name, seed):
print(f"prompt: {prompt}")
print("sketch updated")
if image is None:
ones = Image.new("L", (512, 512), 255)
temp_uri = pil_image_to_data_uri(ones)
return ones, gr.update(link=temp_uri), gr.update(link=temp_uri)
prompt = prompt_template.replace("{prompt}", prompt)
image = image.convert("RGB")
image_t = F.to_tensor(image) > 0.5
print(f"seed={seed}")
caption_tokens = tokenizer(prompt, max_length=tokenizer.model_max_length, padding="max_length", truncation=True, return_tensors="pt").input_ids.cpu()
with torch.no_grad():
c_t = image_t.unsqueeze(0)
torch.manual_seed(seed)
B, C, H, W = c_t.shape
noise = torch.randn((1, 4, H // 8, W // 8))
output_image = torch.from_numpy(compiled_model([c_t.to(torch.float32), caption_tokens, noise])[0])
output_pil = F.to_pil_image(output_image[0].cpu() * 0.5 + 0.5)
input_sketch_uri = pil_image_to_data_uri(Image.fromarray(255 - np.array(image)))
output_image_uri = pil_image_to_data_uri(output_pil)
return (
output_pil,
gr.update(link=input_sketch_uri),
gr.update(link=output_image_uri),
)
def update_canvas(use_line, use_eraser):
if use_eraser:
_color = "#ffffff"
brush_size = 20
if use_line:
_color = "#000000"
brush_size = 4
return gr.update(brush_radius=brush_size, brush_color=_color, interactive=True)
def upload_sketch(file):
_img = Image.open(file.name)
_img = _img.convert("L")
return gr.update(value=_img, source="upload", interactive=True)
scripts = """
async () => {
globalThis.theSketchDownloadFunction = () => {
console.log("test")
var link = document.createElement("a");
dataUri = document.getElementById('download_sketch').href
link.setAttribute("href", dataUri)
link.setAttribute("download", "sketch.png")
document.body.appendChild(link); // Firefox に必要
link.click();
document.body.removeChild(link); // クリーンアップ
// 出力ダウンロード機能も呼び出す
theOutputDownloadFunction();
return false
}
globalThis.theOutputDownloadFunction = () => {
console.log("test output download function")
var link = document.createElement("a");
dataUri = document.getElementById('download_output').href
link.setAttribute("href", dataUri);
link.setAttribute("download", "output.png");
document.body.appendChild(link); // Firefox に必要
link.click();
document.body.removeChild(link); // クリーンアップ
return false
}
globalThis.UNDO_SKETCH_FUNCTION = () => {
console.log("undo sketch function")
var button_undo = document.querySelector('#input_image > div.image-container.svelte-p3y7hu > div.svelte-s6ybro > button:nth-child(1)');
// 新しい 'click' イベントを作成
var event = new MouseEvent('click', {
'view': window,
'bubbles': true,
'cancelable': true
});
button_undo.dispatchEvent(event);
}
globalThis.DELETE_SKETCH_FUNCTION = () => {
console.log("delete sketch function")
var button_del = document.querySelector('#input_image > div.image-container.svelte-p3y7hu > div.svelte-s6ybro > button:nth-child(2)');
// 新しい 'click’ イベントを作成
var event = new MouseEvent('click', {
'view': window,
'bubbles': true,
'cancelable': true
});
button_del.dispatchEvent(event);
}
globalThis.togglePencil = () => {
el_pencil = document.getElementById('my-toggle-pencil');
el_pencil.classList.toggle('clicked');
// グラデーション・ボタンのクリックをシミュレート
btn_gradio = document.querySelector("#cb-line > label > input");
var event = new MouseEvent('click', {
'view': window,
'bubbles': true,
'cancelable': true
});
btn_gradio.dispatchEvent(event);
if (el_pencil.classList.contains('clicked')) {
document.getElementById('my-toggle-eraser').classList.remove('clicked');
document.getElementById('my-div-pencil').style.backgroundColor = "gray";
document.getElementById('my-div-eraser').style.backgroundColor = "white";
}
else {
document.getElementById('my-toggle-eraser').classList.add('clicked');
document.getElementById('my-div-pencil').style.backgroundColor = "white";
document.getElementById('my-div-eraser').style.backgroundColor = "gray";
}
}
globalThis.toggleEraser = () => {
element = document.getElementById('my-toggle-eraser');
element.classList.toggle('clicked');
// gradio ボタンのクリックをシミュレート
btn_gradio = document.querySelector("#cb-eraser > label > input");
var event = new MouseEvent('click', {
'view': window,
'bubbles': true,
'cancelable': true
});
btn_gradio.dispatchEvent(event);
if (element.classList.contains('clicked')) {
document.getElementById('my-toggle-pencil').classList.remove('clicked');
document.getElementById('my-div-pencil').style.backgroundColor = "white";
document.getElementById('my-div-eraser').style.backgroundColor = "gray";
}
else {
document.getElementById('my-toggle-pencil').classList.add('clicked');
document.getElementById('my-div-pencil').style.backgroundColor = "gray";
document.getElementById('my-div-eraser').style.backgroundColor = "white";
}
}
}
"""
with gr.Blocks(css="style.css") as demo:
# これらはキャンバスの変更をトリガーするために使用される隠しボタン
line = gr.Checkbox(label="line", value=False, elem_id="cb-line")
eraser = gr.Checkbox(label="eraser", value=False, elem_id="cb-eraser")
with gr.Row(elem_id="main_row"):
with gr.Column(elem_id="column_input"):
gr.Markdown("## INPUT", elem_id="input_header")
image = gr.Image(
source="canvas",
tool="color-sketch",
type="pil",
image_mode="L",
invert_colors=True,
shape=(512, 512),
brush_radius=4,
height=440,
width=440,
brush_color="#000000",
interactive=True,
show_download_button=True,
elem_id="input_image",
show_label=False,
)
download_sketch = gr.Button("Download sketch", scale=1, elem_id="download_sketch")
gr.HTML(
"""
<div class="button-row">
<div id="my-div-pencil" class="pad2"> <button id="my-toggle-pencil" onclick="return togglePencil(this)"></button> </div>
<div id="my-div-eraser" class="pad2"> <button id="my-toggle-eraser" onclick="return toggleEraser(this)"></button> </div>
<div class="pad2"> <button id="my-button-undo" onclick="return UNDO_SKETCH_FUNCTION(this)"></button> </div>
<div class="pad2"> <button id="my-button-clear" onclick="return DELETE_SKETCH_FUNCTION(this)"></button> </div>
<div class="pad2"> <button href="TODO" download="image" id="my-button-down" onclick='return theSketchDownloadFunction()'></button> </div>
</div>
"""
)
# gr.Markdown("## Prompt", elem_id="tools_header")
prompt = gr.Textbox(label="Prompt", value="", show_label=True)
with gr.Row():
style = gr.Dropdown(
label="Style",
choices=STYLE_NAMES,
value=DEFAULT_STYLE_NAME,
scale=1,
)
prompt_temp = gr.Textbox(
label="Prompt Style Template",
value=styles[DEFAULT_STYLE_NAME],
scale=2,
max_lines=1,
)
with gr.Row():
seed = gr.Textbox(label="Seed", value=42, scale=1, min_width=50)
randomize_seed = gr.Button("Random", scale=1, min_width=50)
with gr.Column(elem_id="column_process", min_width=50, scale=0.4):
gr.Markdown("## pix2pix-turbo", elem_id="description")
run_button = gr.Button("Run", min_width=50)
with gr.Column(elem_id="column_output"):
gr.Markdown("## OUTPUT", elem_id="output_header")
result = gr.Image(
label="Result",
height=440,
width=440,
elem_id="output_image",
show_label=False,
show_download_button=True,
)
download_output = gr.Button("Download output", elem_id="download_output")
gr.Markdown("### Instructions")
gr.Markdown("**1**.Enter a text prompt (e.g. cat)")
gr.Markdown("**2**.Start sketching")
gr.Markdown("**3**.Change the image style using a style template")
gr.Markdown("**4**.Try different seeds to generate different results")
eraser.change(
fn=lambda x: gr.update(value=not x),
inputs=[eraser],
outputs=[line],
queue=False,
api_name=False,
).then(update_canvas, [line, eraser], [image])
line.change(
fn=lambda x: gr.update(value=not x),
inputs=[line],
outputs=[eraser],
queue=False,
api_name=False,
).then(update_canvas, [line, eraser], [image])
demo.load(None, None, None, _js=scripts)
randomize_seed.click(
lambda x: random.randint(0, MAX_SEED),
inputs=[],
outputs=seed,
queue=False,
api_name=False,
)
inputs = [image, prompt, prompt_temp, style, seed]
outputs = [result, download_sketch, download_output]
prompt.submit(fn=run, inputs=inputs, outputs=outputs, api_name=False)
style.change(
lambda x: styles[x],
inputs=[style],
outputs=[prompt_temp],
queue=False,
api_name=False,
).then(
fn=run,
inputs=inputs,
outputs=outputs,
api_name=False,
)
run_button.click(fn=run, inputs=inputs, outputs=outputs, api_name=False)
image.change(run, inputs=inputs, outputs=outputs, queue=False, api_name=False)
try:
demo.queue().launch(debug=False)
except Exception:
demo.queue().launch(debug=False, share=True)
# リモートで起動する場合は、server_name と server_port を指定
# demo.launch(server_name='your server name', server_port='server port in int')
# 詳細はドキュメントをご覧ください: https://gradio.app/docs/
/tmp/ipykernel_173952/1555011934.py:259: GradioDeprecationWarning: 'scale' value should be an integer. Using 0.4 will cause issues.
with gr.Column(elem_id="column_process", min_width=50, scale=0.4): /opt/home/k8sworker/ci-ai/cibuilds/ov-notebook/OVNotebookOps-727/.workspace/scm/ov-notebook/.venv/lib/python3.8/site-packages/gradio/utils.py:776: UserWarning: Expected 1 arguments for function <function <lambda> at 0x7fda5d68fca0>, received 0.
warnings.warn(
/opt/home/k8sworker/ci-ai/cibuilds/ov-notebook/OVNotebookOps-727/.workspace/scm/ov-notebook/.venv/lib/python3.8/site-packages/gradio/utils.py:780: UserWarning: Expected at least 1 arguments for function <function <lambda> at 0x7fda5d68fca0>, received 0.
warnings.warn(
ローカル URL で実行中: http://127.0.0.1:7860 パブリックリンクを作成するには、launch() で share=True を設定します。