BLIP と OpenVINO を使用した視覚的な質疑応答と画像キャプション#
この Jupyter ノートブックは、ローカルへのインストール後にのみ起動できます。
人間は視覚と言語を通じて世界を認識します。AI の長年の目標は、視覚と言語入力を通じて世界を理解し、自然言語を通じて人間と対話できる知的なエージェントを構築することです。この目標を達成するため、視覚言語事前トレーニングが効果的なアプローチとして登場しました。これは、大規模な画像テキスト・データセットでディープ・ニューラル・ネットワーク・モデルを事前トレーニングし、画像テキスト検索、画像キャプション作成、視覚的な質問への回答などの下流の視覚言語タスクのパフォーマンスを向上させるものです。
BLIP は、統合された視覚と言語の理解と生成のための言語と画像の事前トレーニング・フレームワークです。BLIP は、幅広い視覚言語タスクで最先端の結果を実現します。このチュートリアルでは、視覚的な質問回答と画像キャプションの作成に BLIP を使用する方法を説明します。チュートリアルの追加部分では、8 ビットのトレーニング後の量子化と NNCF (ニューラル・ネットワーク圧縮フレームワーク) のデータフリー int8 重み圧縮を OpenVINO IR モデルに適用してモデルを高速化し、OpenVINO™ ツールキットによって最適化された BLIP モデルを推論する方法を示します。
チュートリアルは以下で構成されています:
BLIP モデルをインスタンス化します。
BLIP モデルを OpenVINO IR に変換します。
OpenVINO を使用して、視覚的な質疑応答と画像キャプションを実行します。
NNCF を使用して BLIP モデルを最適化
オリジナルモデルと最適化モデルを比較
インタラクティブなデモを起動
目次:
背景#
視覚言語処理は、コンピューターが画像とその内容をより正確に理解できるように設計されたアルゴリズムに重点を置いた人工知能の分野です。
次のタスクが良く利用されます:
テキストからの画像検索 - 与えられたテキストの説明に最も関連性の高い画像を見つけることを目的としたセマンティック・タスク。
画像キャプション - 画像コンテンツにテキストによる説明を提供することを目的としたセマンティック・タスク。
視覚的な質問回答 - 画像コンテンツに基づいて質問に答えることを目的としたセマンティック・タスク。
下の図に示すように、これら 3 つのタスクは AI システムに提供される入力が異なります。テキストから画像への検索では、検索用に事前定義された画像ギャラリーと、ユーザーが要求したテキストの記述 (クエリー) があります。画像キャプションは、視覚的な質問応答の特殊なケースとして表すことができます。ここでは、“画像には何がありますか?” という事前定義された質問と、ユーザーが提供するさまざまな画像があります。視覚的な質問応答の場合、テキストベースの質問と画像のコンテキストの両方がユーザーによって要求される変数です。
このノートブックはテキストから画像への検索に焦点を当てていません。代わりに、画像キャプションと視覚的な質問回答を考慮します。
画像のキャプション#
画像キャプションとは、画像の内容を言葉で説明する作業です。このタスクは、コンピューター・ビジョンと自然言語処理の交差点にあります。ほとんどの画像キャプション・システムでは、エンコーダー/デコーダー・フレームワークが使用されています。このフレームワークでは、入力画像が画像内の情報の中間表現にエンコードされ、その後、説明的なテキストシーケンスにデコードされます。
視覚的な質問への回答#
Visual Question Answering (VQA) は、画像コンテンツに関するテキストベースの質問に答えるタスクです。
VQA の仕組みをより理解するために、与えられたテキスト入力から質問に対する回答を取得することを目的とする、質問応答などの従来の NLP タスクを考えてみましょう。通常、質問応答パイプラインは次の 3 つのステップで構成されます:
質問分析 - 質問内のオブジェクトと追加のコンテキストを理解するため、自然言語形式で提供された質問を分析します。例えば、“パリには橋がいくつありますか?” という質問の場合、“いくつ” という疑問語は答えが数字である可能性が高いというヒントを与え、“橋” は質問の対象オブジェクトであり、”パリには” は検索の追加コンテキストとして機能します。
検索用のクエリーを構築します - 分析された結果を使用して、最も関連性の高い情報を見つけるためのクエリーを形式化します。
ナレッジベースで検索を実行します。クエリーをナレッジベースに送信します。通常、テキスト・ドキュメントまたはデータベースが知識のソースとして提供されます。
テキストベースの質問応答とビジュアル質問応答の違いは、画像がコンテキストと知識ベースとして使用されることです。
画像に関する任意の質問に答えることは、多くのコンピューター・ビジョンのサブタスクを必要とするため、複雑な問題です。以下の表に、質問の例と、回答を見つけるために必要なコンピューター・ビジョンのスキルを示します。
コンピューター・ビジョン・タスク |
質問例 |
---|---|
物体認識 |
写真に何が写っていますか? それは何ですか? |
物体検出 |
画像内に何か物体 (犬、男性、本) はありますか? どこに位置していますか? |
物体と画像の属性認識 |
傘は何色ですか? この男性は眼鏡をかけていますか? 画像に色はありますか? |
シーン認識 |
雨ですか? 描かれているのはどんなお祝いですか? |
物体カウント |
サッカー場には何人の選手がいますか? 階段は何段ありますか? |
アクティビティー認識 |
赤ちゃんは泣いていますか? その女性は何を料理していますか? 彼らは何をしていますか? |
物体間の空間関係 |
ソファとアームチェアの間には何がありますか? 左下隅には何がありますか? |
常識的な推論 |
彼女の視力は 100% ですか? この人には子供がいますか? |
知識ベースの推論 |
ベジタリアン向けのピザですか? |
テキスト認識 |
その本のタイトルは何ですか? 画面には何が映っていますか? |
視覚的な質問回答には多くのアプリケーションがあります:
視覚障害者の支援: VQA モデルは、視覚障害者がウェブや現実世界から画像に関する情報を取得できるようにすることで、視覚障害者の障壁を軽減するのに使用できます。
教育: VQA モデルを使用すると、観察者が興味のある質問を直接できるようにすることで博物館での訪問者の体験を向上させたり、特定の知識を習得することに興味のある子供たちのために教科書の対話性を高めたりすることができます。
E コマース: VQA モデルは、オンラインストアの写真を使用して製品に関する情報を取得できます。
独立した専門家による評価: VQA モデルは、スポーツ競技、医療診断、法医学的検査において客観的な評価を提供できます。
モデルのインスタンス化#
BLIP モデルは、BLIP: Bootstrapping Language-Image Pre-training for Unified Vision-Language Understanding and Generation (英語) という論文で提案されました。

blip.gif#
理解と生成の両方の機能を備えた統合視覚言語モデルを事前トレーニングするため、BLIP は、次の 3 つのモードのいずれかで動作できるエンコーダーとデコーダーのマルチモーダル混合とマルチタスク・モデルを導入します:
画像とテキストを別々にエンコードするユニモーダル・エンコーダー。画像エンコーダーはビジョン・トランスフォーマーです。テキスト・エンコーダーは BERT と同じです。
Image-grounded テキスト・エンコーダーは、テキスト・エンコーダーの各トランスフォーマー・ブロックの自己注意層とフィードフォワード・ネットワークの間にクロス注意レイヤーを挿入することで視覚情報を注入します。
Image-grounded テキストデコーダーは、テキスト・エンコーダーの双方向自己注意レイヤーを因果自己注意レイヤーに置き換えます。
モデルの詳細については、研究論文、Salesforce ブログ、GitHub リポジトリー、Hugging Face モデルのドキュメントをご覧ください。
このチュートリアルでは、Hugging Face からダウンロードできる blip-vqa-base モデルを使用します。同じアクションは、BLIP ファミリーの他の同様なモデルにも適用できます。このモデルクラスは質問への回答を実行するように設計されていますが、そのコンポーネントは画像のキャプション作成にも再利用できます。
モデルの操作を開始するには、from_pretrained
メソッドを使用して BlipForQuestionAnswering
クラスをインスタンス化する必要があります。BlipProcessor
は、テキストとビジョンの両方のモダリティーの入力データを準備し、生成結果を後処理するためのヘルパークラスです。
import platform
%pip install -q --extra-index-url https://download.pytorch.org/whl/cpu "torch>=2.1.0" torchvision "transformers>=4.26.0" "gradio>=4.19" "openvino>=2023.3.0" "datasets>=2.14.6" "nncf>=2.8.1" "tqdm"
if platform.system() != "Windows":
%pip install -q "matplotlib>=3.4"
else:
%pip install -q "matplotlib>=3.4,<3.7"
import time
from PIL import Image
from transformers import BlipProcessor, BlipForQuestionAnswering
# `notebook_utils` モジュールを取得
import requests
r = requests.get(
url="https://raw.githubusercontent.com/openvinotoolkit/openvino_notebooks/latest/utils/notebook_utils.py",
)
open("notebook_utils.py", "w").write(r.text)
from notebook_utils import download_file
# モデルとプロセッサーを取得
processor = BlipProcessor.from_pretrained("Salesforce/blip-vqa-base")
model = BlipForQuestionAnswering.from_pretrained("Salesforce/blip-vqa-base")
# テスト入力の設定: 画像をダウンロードして読み取り質問を準備
img_url = "https://storage.googleapis.com/sfr-vision-language-research/BLIP/demo.jpg"
download_file(img_url, "demo.jpg")
raw_image = Image.open("demo.jpg").convert("RGB")
question = "how many dogs are in the picture?"# 入力データを前処理
inputs = processor(raw_image, question, return_tensors="pt")
start = time.perf_counter()
# 生成を実行
out = model.generate(**inputs)
end = time.perf_counter() - start
# 後処理結果
answer = processor.decode(out[0], skip_special_tokens=True)
print(f"Processing time: {end:.4f} s")
Processing time: 0.3707 s
from pathlib import Path
if not Path("./utils.py").exists():
download_file(url="https://raw.githubusercontent.com/openvinotoolkit/openvino_notebooks/latest/notebooks/blip-visual-language-processing/utils.py")
from utils import visualize_results
fig = visualize_results(raw_image, answer, question)
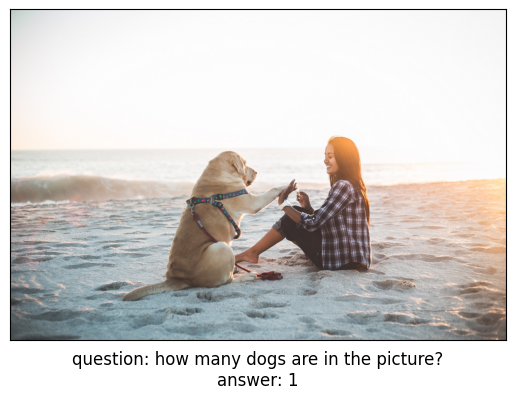
モデルを OpenVINO IR に変換#
OpenVINO 2023.0 リリース以降、OpenVINO は、高度な OpenVINO 最適化ツールと機能を活用できるように、PyTorch モデルを OpenVINO 中間表現 (IR) 形式に直接変換するのをサポートしています。OpenVINO モデル・トランスフォーメーション API にモデル・オブジェクトとモデルトレースの入力データを提供する必要があります。ov.convert_model
関数は、PyTorch モデル・インスタンスを、デバイス上でコンパイルするために使用したり、ov.save_model
を使用して FP16 形式に圧縮してディスクに保存できる ov.Model
オブジェクトに変換します。
モデルは 3 つのパーツで構成されます:
vision_model - 画像表現用のエンコーダー。
text_encoder - 入力クエリー用のエンコーダーであり、質問への回答とテキストから画像への検索にのみ使用されます。
text_decoder - 出力回答用のデコーダー。
同じモデル・コンポーネントを使用して複数のタスクを実行できるようにするには、各パーツを個別に変換する必要があります。
ビジョンモデル#
ビジョンモデルは、[0,1] の範囲で正規化された RGB 画像ピクセル値を含む、[1,3,384,384] 形状の浮動小数点入力テンソルを受け入れます。
import torch
from pathlib import Path
import openvino as ov
VISION_MODEL_OV = Path("blip_vision_model.xml")
vision_model = model.vision_model
vision_model.eval()
# モデルが機能することを確認し、テキスト・エンコーダーの入力として再利用するため出力を保存
with torch.no_grad():
vision_outputs = vision_model(inputs["pixel_values"])
# openvino モデルが存在しない場合は IR に変換
if not VISION_MODEL_OV.exists():
# PyTorch モデルを ov.Model にエクスポート
with torch.no_grad():
ov_vision_model = ov.convert_model(vision_model, example_input=inputs["pixel_values"])
# 次回に備えてモデルをディスクに保存
ov.save_model(ov_vision_model, VISION_MODEL_OV)
print(f"Vision model successfuly converted and saved to {VISION_MODEL_OV}")
else:
print(f"Vision model will be loaded from {VISION_MODEL_OV}")
/home/ltalamanova/tmp_venv/lib/python3.11/site-packages/transformers/modeling_utils.py:4225: FutureWarning: _is_quantized_training_enabled is going to be deprecated in transformers 4.39.0.Please use model.hf_quantizer.is_trainable instead warnings.warn(
Vision model successfuly converted and saved to blip_vision_model.xml
テキスト・エンコーダー#
テキスト・エンコーダーは、視覚的な質問応答タスクによって質問埋め込み表現を構築するために使用されます。トークン化された質問を含む input_ids
を受け取り、ビジョンモデルから取得された画像埋め込みとそれらの注意マスクを出力します。
TEXT_ENCODER_OV = Path("blip_text_encoder.xml")
text_encoder = model.text_encoder
text_encoder.eval()
# openvino モデルが存在しない場合は IR に変換
if not TEXT_ENCODER_OV.exists():
# サンプル入力を準備
image_embeds = vision_outputs[0]
image_attention_mask = torch.ones(image_embeds.size()[:-1], dtype=torch.long)
input_dict = {
"input_ids": inputs["input_ids"],
"attention_mask": inputs["attention_mask"],
"encoder_hidden_states": image_embeds,
"encoder_attention_mask": image_attention_mask,
}
# PyTorch モデルをエクスポート
with torch.no_grad():
ov_text_encoder = ov.convert_model(text_encoder, example_input=input_dict)
} # 次回に備えてモデルをディスクに保存
ov.save_model(ov_text_encoder, TEXT_ENCODER_OV)
print(f"Text encoder successfuly converted and saved to {TEXT_ENCODER_OV}")
else:
print(f"Text encoder will be loaded from {TEXT_ENCODER_OV}")
Text encoder successfuly converted and saved to blip_text_encoder.xml
テキストデコーダー#
テキストデコーダーは、画像 (および必要に応じて質問) 表現を使用して、モデル出力 (質問への回答またはキャプション) を表すトークンのシーケンスを生成する役割を担います。生成アプローチは、単語シーケンスの確率分布を条件付きの次の単語分布の積に分解できるという仮定に基づいています。言い換えると、モデルは、停止条件 (最大長の生成されたシーケンスまたは文字列トークンの終了が取得される) に達するまで、以前に生成されたトークンに基づいてループ内の次のトークンを予測します。予測される確率に基づいて次のトークンが選択される方法は、選択されたデコード方法によって決まります。最も一般的なデコード方法の詳細については、このブログをご覧ください。Hugging Face Transformers ライブラリーのモデル生成プロセスのエントリーポイントは、generate
メソッドです。パラメーターと構成の詳細については、ドキュメントを参照してください。選択デコード方法論の柔軟性を維持するため、1 つのステップでモデル推論のみを変換します。
生成プロセスを最適化し、メモリーをより効率的に使用するには、use_cache=True
オプションを有効にします。出力側は自動回帰であるため、出力トークンの非表示状態は、その後の生成ステップごとに計算されると同じままになります。したがって、新しいトークンを生成するたびに再計算するのは無駄であるように思えます。キャッシュを使用すると、モデルは計算後に非表示の状態を保存します。モデルは各タイムステップで最後に生成された出力トークンのみを計算し、保存された出力トークンを非表示のトークンに再利用します。これにより、変圧器モデルの生成の複雑さが O(n^3) から O(n^2) に軽減されます。仕組みの詳細については、この記事を参照してください。このオプションを使用すると、モデルは前のステップの非表示状態を入力として取得し、さらに現在のステップの非表示状態を出力として提供します。最初は、前のステップの隠し状態がないため、最初のステップでは隠し状態を提供する必要はありませんが、デフォルト値で初期化する必要があります。PyTorch では、過去の隠し状態の出力は、モデル内の各トランスフォーマー・レイヤーのペアのリスト (キーの隠し状態、値の隠し状態) として表現されます。OpenVINO モデルはネストされた出力をサポートしていないため、出力はフラット化されます。
text_encoder
と同様に、text_decoder
はさまざまな長さの入力シーケンスを処理でき、動的な入力形状を保持する必要があります。
text_decoder = model.text_decoder
text_decoder.eval()
TEXT_DECODER_OV = Path("blip_text_decoder_with_past.xml")
# サンプル入力を準備
input_ids = torch.tensor([[30522]]) # シーケンストークン ID の開始
attention_mask = torch.tensor([[1]]) # input_ids のアテンション・マスク
encoder_hidden_states = torch.rand((1, 10, 768)) # text_encoder からのエンコーダーの最後の非表示状態
encoder_attention_mask = torch.ones((1, 10), dtype=torch.long) # エンコーダーの隠れた状態のアテンション・マスク
input_dict = {
"input_ids": input_ids,
"attention_mask": attention_mask,
"encoder_hidden_states": encoder_hidden_states,
"encoder_attention_mask": encoder_attention_mask,
}
text_decoder_outs = text_decoder(**input_dict)
# 前のステップの隠し状態を使用して入力辞書を拡張
input_dict["past_key_values"] = text_decoder_outs["past_key_values"]
text_decoder.config.torchscript = True
if not TEXT_DECODER_OV.exists():
# PyTorch モデルをエクスポート
with torch.no_grad():
ov_text_decoder = ov.convert_model(text_decoder, example_input=input_dict)
# 次回に備えてモデルをディスクに保存
ov.save_model(ov_text_decoder, TEXT_DECODER_OV)
print(f"Text decoder successfuly converted and saved to {TEXT_DECODER_OV}")
else:
print(f"Text decoder will be loaded from {TEXT_DECODER_OV}")
/home/ltalamanova/tmp_venv/lib/python3.11/site-packages/transformers/models/blip/modeling_blip_text.py:635: TracerWarning: Converting a tensor to a Python boolean might cause the trace to be incorrect.We can't record the data flow of Python values, so this value will be treated as a constant in the future.This means that the trace might not generalize to other inputs!
if causal_mask.shape[1] < attention_mask.shape[1]: /home/ltalamanova/tmp_venv/lib/python3.11/site-packages/torch/jit/_trace.py:165: UserWarning: The .grad attribute of a Tensor that is not a leaf Tensor is being accessed.Its .grad attribute won't be populated during autograd.backward().If you indeed want the .grad field to be populated for a non-leaf Tensor, use .retain_grad() on the non-leaf Tensor.If you access the non-leaf Tensor by mistake, make sure you access the leaf Tensor instead.See github.com/pytorch/pytorch/pull/30531 for more informations.(Triggered internally at aten/src/ATen/core/TensorBody.h:489.)
if a.grad is not None:
Text decoder successfuly converted and saved to blip_text_decoder_with_past.xml
OpenVINO モデルの実行#
推論パイプラインの準備#
前述したように、このモデルは、さまざまなタスクのパイプラインを構築するのに再利用できる複数のブロックで構成されています。下の図で、画像キャプションの仕組みがわかります:
ビジュアルモデルは、BlipProcessor
によって前処理された画像を入力として受け入れ、画像埋め込みを生成します。この画像埋め込みは、キャプション・トークンを生成するためテキストデコーダーに直接渡されます。生成が完了すると、トークンの出力シーケンスが BlipProcessor
に提供され、トークナイザーを使用してテキストにデコードされます。
質疑応答のパイプラインは似ていますが、質問処理が追加されています。この場合、BlipProcessor
によってトークン化された画像埋め込みと質問がテキスト・エンコーダーに提供され、次にマルチモーダル質問埋め込みがテキストデコーダーに渡されて回答の生成が行われます。
次のステップは、OpenVINO モデルを使用して両方のパイプラインを実装することです。
# OpenVINO Core オブジェクト・インスタンスを作成
core = ov.Core()
推論デバイスの選択#
OpenVINO を使用して推論を実行するためにドロップダウン・リストからデバイスを選択します
import ipywidgets as widgets
device = widgets.Dropdown(
options=core.available_devices + ["AUTO"],
value="AUTO",
description="Device:",
disabled=False,
)
device
huggingface/tokenizers: The current process just got forked, after parallelism has already been used.Disabling parallelism to avoid deadlocks...To disable this warning, you can either: - Avoid using tokenizers before the fork if possible - Explicitly set the environment variable TOKENIZERS_PARALLELISM=(true | false)
Dropdown(description='Device:', index=4, options=('CPU', 'GPU.0', 'GPU.1', 'GPU.2', 'AUTO'), value='AUTO')
# デバイスにモデルをロード
ov_vision_model = core.compile_model(VISION_MODEL_OV, device.value)
ov_text_encoder = core.compile_model(TEXT_ENCODER_OV, device.value)
ov_text_decoder_with_past = core.compile_model(TEXT_DECODER_OV, device.value)
from functools import partial
from blip_model import text_decoder_forward
text_decoder.forward = partial(text_decoder_forward, ov_text_decoder_with_past=ov_text_decoder_with_past)
モデルのヘルパークラスには、生成用の 2 つのメソッドがあります: generate_answer - 視覚的な質問回答に使用、 generate_caption - キャプション生成に使用。初期化では、モデルクラスは、テキスト・エンコーダー、ビジョンモデル、テキストデコーダー用のコンパイル済み OpenVINO モデルを受け入れ、デコーダー作業用の生成および初期トークンの構成も受け入れます。
if not Path("./blip_model.py").exists():
download_file(url="https://raw.githubusercontent.com/openvinotoolkit/openvino_notebooks/latest/notebooks/blip-visual-language-processing/blip_model.py")
from blip_model import OVBlipModel
ov_model = OVBlipModel(model.config, model.decoder_start_token_id, ov_vision_model, ov_text_encoder, text_decoder)
out = ov_model.generate_answer(**inputs, max_length=20)
これで、モデルを生成する準備が整いました。
画像のキャプション#
out = ov_model.generate_caption(inputs["pixel_values"], max_length=20)
caption = processor.decode(out[0], skip_special_tokens=True)
fig = visualize_results(raw_image, caption)
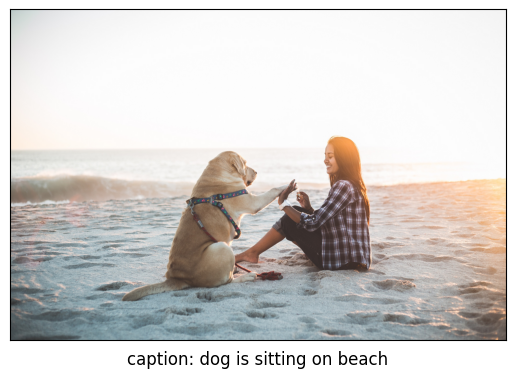
質問への回答#
start = time.perf_counter()
out = ov_model.generate_answer(**inputs, max_length=20)
end = time.perf_counter() - start
answer = processor.decode(out[0], skip_special_tokens=True)
fig = visualize_results(raw_image, answer, question)
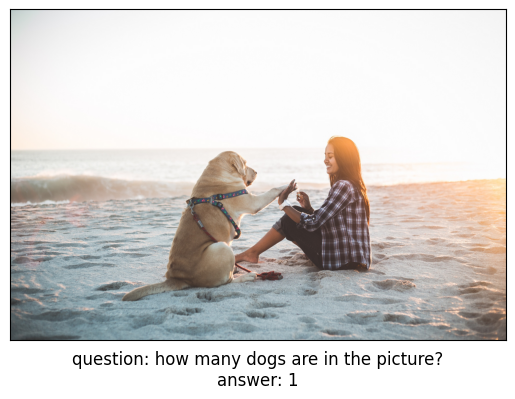
print(f"Processing time: {end:.4f}")
Processing time: 0.1186
NNCF を使用してモデルを最適化#
NNCF は、モデルグラフに量子化レイヤーを追加し、トレーニング・データセットのサブセットを使用してこれらの追加の量子化レイヤーのパラメーターを初期化することで、トレーニング後の量子化を可能にします。このフレームワークは、元のトレーニング・コードへの変更が最小限になるように設計されています。
最適化プロセスには次の手順が含まれます:
-
量子化用のデータセットを作成します。
nncf.quantize
を実行して、事前トレーニングされたFP16
モデルから量子化されたモデルを取得します。openvino.save_model
関数を使用してINT8
モデルをシリアル化します。
注: 量子化は時間とメモリーを消費する操作です。以下の量子化コードの実行には時間がかかる場合があります。以下のウィジェットで無効化できます:
to_quantize = widgets.Checkbox(
value=True,
description="Quantization",
disabled=False,
)
to_quantize
Checkbox(value=True, description='Quantization')
VISION_MODEL_OV_INT8 = Path(str(VISION_MODEL_OV).replace(".xml", "_int8.xml"))
TEXT_ENCODER_OV_INT8 = Path(str(TEXT_ENCODER_OV).replace(".xml", "_int8.xml"))
TEXT_DECODER_OV_INT8 = Path(str(TEXT_DECODER_OV).replace(".xml", "_int8.xml"))
int8_model = None
# skip_kernel_extension モジュールを取得
r = requests.get(
url="https://raw.githubusercontent.com/openvinotoolkit/openvino_notebooks/latest/utils/skip_kernel_extension.py",
)
open("skip_kernel_extension.py", "w").write(r.text)
%load_ext skip_kernel_extension
データセットの準備#
VQAv2 は、画像に関する自由形式の質問を含むデータセットです。これらの問に答えるには、視覚、言語、常識的な知識の理解が必要です。
%%skip not $to_quantize.value
import numpy as np
from datasets import load_dataset
from tqdm.notebook import tqdm
def preprocess_batch(batch, vision_model, inputs_info):
"""
Preprocesses a dataset batch by loading and transforming image and text data.
VQAv2 dataset contains multiple questions to image.
To reduce dataset preparation time we will store preprocessed images in `inputs_info`.
"""
image_id = batch["image_id"]
if image_id in inputs_info:
inputs = processor(text=batch['question'], return_tensors="np")
pixel_values = inputs_info[image_id]["pixel_values"]
encoder_hidden_states = inputs_info[image_id]["encoder_hidden_states"]
else:
inputs = processor(images=batch["image"], text=batch["question"], return_tensors="np")
pixel_values = inputs["pixel_values"]
encoder_hidden_states = vision_model(pixel_values)[vision_model.output(0)]
inputs_info[image_id] = {
"pixel_values": pixel_values,
"encoder_hidden_states": encoder_hidden_states,
"text_encoder_inputs": []
}
text_encoder_inputs = {
"input_ids": inputs["input_ids"],
"attention_mask": inputs["attention_mask"]
}
inputs_info[image_id]["text_encoder_inputs"].append(text_encoder_inputs)
def prepare_input_data(dataloader, vision_model, opt_init_steps):
"""
Store calibration subset in List to reduce quantization time.
"""
inputs_info = {}
for idx, batch in enumerate(tqdm(dataloader, total=opt_init_steps, desc="Prepare calibration data")):
preprocess_batch(batch, vision_model, inputs_info)
calibration_subset = []
for image_id in inputs_info:
pixel_values = inputs_info[image_id]["pixel_values"]
encoder_hidden_states = inputs_info[image_id]["encoder_hidden_states"]
encoder_attention_mask = np.ones(encoder_hidden_states.shape[:-1], dtype=int)
for text_encoder_inputs in inputs_info[image_id]["text_encoder_inputs"]:
text_encoder_inputs["encoder_hidden_states"] = encoder_hidden_states
text_encoder_inputs["encoder_attention_mask"] = encoder_attention_mask
blip_inputs = {
"vision_model_inputs": {"pixel_values": pixel_values},
"text_encoder_inputs": text_encoder_inputs,
}
calibration_subset.append(blip_inputs)
return calibration_subset
def prepare_dataset(vision_model, opt_init_steps=300, streaming=False):
"""
Prepares a vision-text dataset for quantization.
"""
split = f"train[:{opt_init_steps}]" if not streaming else "train"
dataset = load_dataset("HuggingFaceM4/VQAv2", split=split, streaming=streaming, trust_remote_code=True)
dataset = dataset.shuffle(seed=42)
if streaming:
dataset = dataset.take(opt_init_steps)
calibration_subset = prepare_input_data(dataset, vision_model, opt_init_steps)
return calibration_subset
ストリーミング・モードでのデータセットの読み込みと処理には時間がかかりますが、インターネットの接続環境によって異なります。
%%skip not $to_quantize.value
import nncf
comp_vision_model = core.compile_model(VISION_MODEL_OV, device.value)
calibration_data = prepare_dataset(comp_vision_model)
量子化ビジョンモデル#
%%skip not $to_quantize.value
vision_dataset = nncf.Dataset(calibration_data, lambda x: x["vision_model_inputs"])
vision_model = core.read_model(VISION_MODEL_OV)
quantized_model = nncf.quantize(
model=vision_model,
calibration_dataset=vision_dataset,
model_type=nncf.ModelType.TRANSFORMER
)
ov.save_model(quantized_model, VISION_MODEL_OV_INT8)
Output()
Output()
INFO:nncf:36 ignored nodes were found by name in the NNCFGraph
INFO:nncf:48 ignored nodes were found by name in the NNCFGraph
Output()
Output()
量子化テキスト・エンコーダー#
%%skip not $to_quantize.value
text_encoder_dataset = nncf.Dataset(calibration_data, lambda x: x["text_encoder_inputs"])
text_encoder_model = core.read_model(TEXT_ENCODER_OV)
quantized_model = nncf.quantize(
model=text_encoder_model,
calibration_dataset=text_encoder_dataset,
model_type=nncf.ModelType.TRANSFORMER
)
ov.save_model(quantized_model, TEXT_ENCODER_OV_INT8)
Output()
Output()
INFO:nncf:72 ignored nodes were found by name in the NNCFGraph
INFO:nncf:73 ignored nodes were found by name in the NNCFGraph
Output()
Output()
テキストデコーダーの圧縮重み#
テキストデコーダーの量子化により、精度が大幅に低下します。トレーニング後の量子化の代わりに、データのフリーウェイト圧縮を使用してモデルのフットプリントを削減できます。
最適化プロセスには次の手順が含まれます:
nncf.compress_weights
を実行して、圧縮された重みを持つモデルを取得します。openvino.save_model
関数を使用してOpenVINO
モデルをシリアル化します。
%%skip not $to_quantize.value
text_decoder_model = core.read_model(TEXT_DECODER_OV)
compressed_text_decoder = nncf.compress_weights(text_decoder_model)
ov.save_model(compressed_text_decoder, str(TEXT_DECODER_OV_INT8))
INFO:nncf:Statistics of the bitwidth distribution:
+--------------+---------------------------+-----------------------------------+
| Num bits (N) | % all parameters (layers) | % ratio-defining parameters |
| | | (layers) |
+==============+===========================+===================================+
| 8 | 100% (124 / 124) | 100% (124 / 124) |
+--------------+---------------------------+-----------------------------------+
Output()
最適化された OpenVINO モデルを実行#
最適化された OpenVINO BLIP モデルを使用して予測を行う手順は、PyTorch モデルと似ています。量子化前のモデルと同じ入力データを使用してモデルの結果を確認してみます。
%%skip not $to_quantize.value
q_ov_vision_model = core.compile_model(VISION_MODEL_OV_INT8, device.value)
q_ov_text_encoder = core.compile_model(TEXT_ENCODER_OV_INT8, device.value)
q_ov_text_decoder_with_past = core.compile_model(TEXT_DECODER_OV_INT8, device.value)
%%skip not $to_quantize.value
from functools import partial
from transformers import BlipForQuestionAnswering
from blip_model import OVBlipModel, text_decoder_forward
model = BlipForQuestionAnswering.from_pretrained("Salesforce/blip-vqa-base")
text_decoder = model.text_decoder
text_decoder.eval()
text_decoder.forward = partial(text_decoder_forward, ov_text_decoder_with_past=q_ov_text_decoder_with_past)
int8_model = OVBlipModel(model.config, model.decoder_start_token_id, q_ov_vision_model, q_ov_text_encoder, text_decoder)
%%skip not $to_quantize.value
raw_image = Image.open("demo.jpg").convert('RGB')
question = "how many dogs are in the picture?"
# 入力データを前処理
inputs = processor(raw_image, question, return_tensors="pt")
画像のキャプション#
%%skip not $to_quantize.value
out = int8_model.generate_caption(inputs["pixel_values"], max_length=20)
caption = processor.decode(out[0], skip_special_tokens=True)
fig = visualize_results(raw_image, caption)
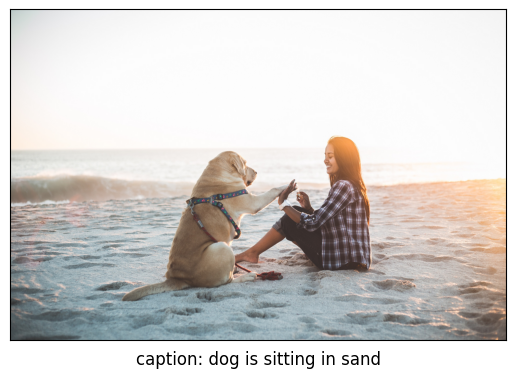
質問への回答#
%%skip not $to_quantize.value
out = int8_model.generate_answer(**inputs, max_length=20)
answer = processor.decode(out[0], skip_special_tokens=True)
fig = visualize_results(raw_image, answer, question)
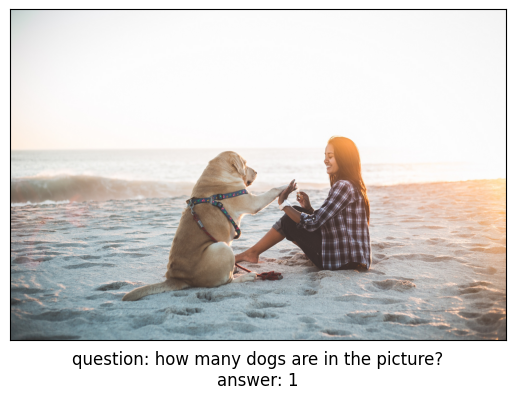
ファイルサイズを比較#
%%skip not $to_quantize.value
def calculate_compression_rate(ov_model_path):
fp16_ir_model_size = Path(ov_model_path).with_suffix(".bin").stat().st_size / 1024
int8_model_path = str(ov_model_path).replace(".xml", "_int8.xml")
quantized_model_size = Path(int8_model_path).with_suffix(".bin").stat().st_size / 1024
print(f'{ov_model_path.as_posix().split(".")[0]}')
print(f" * FP16 IR model size: {fp16_ir_model_size:.2f} KB")
print(f" * INT8 model size: {quantized_model_size:.2f} KB")
print(f" * Model compression rate: {fp16_ir_model_size / quantized_model_size:.3f}")
%%skip not $to_quantize.value
for fp16_path in [VISION_MODEL_OV, TEXT_ENCODER_OV, TEXT_DECODER_OV]:
calculate_compression_rate(fp16_path)
blip_vision_model
* FP16 IR model size: 168145.70 KB
* INT8 model size: 84915.48 KB
* Model compression rate: 1.980
blip_text_encoder
* FP16 IR model size: 268087.16 KB
* INT8 model size: 134676.75 KB
* Model compression rate: 1.991
blip_text_decoder_with_past
* FP16 IR model size: 269303.35 KB
* INT8 model size: 135302.53 KB
* Model compression rate: 1.990
FP16 と最適化モデルの推論時間を比較#
FP16
と INT8
モデルの推論パフォーマンスを測定するには、キャリブレーション・データセットの 100 サンプルの推論時間の中央値を使用します。したがって、動的量子化モデルの速度向上を見積もることができます。
注: 最も正確なパフォーマンス推定を行うには、他のアプリケーションを閉じた後、ターミナル/コマンドプロンプトで
benchmark_app
を実行することを推奨します。
%%skip not $to_quantize.value
import time
import torch
def calculate_inference_time(blip_model, calibration_data, generate_caption):
inference_time = []
for inputs in calibration_data:
pixel_values = torch.from_numpy(inputs["vision_model_inputs"]["pixel_values"])
input_ids = torch.from_numpy(inputs["text_encoder_inputs"]["input_ids"])
attention_mask = torch.from_numpy(inputs["text_encoder_inputs"]["attention_mask"])
start = time.perf_counter()
if generate_caption: _ = blip_model.generate_caption(pixel_values, max_length=20)
else: _ = blip_model.generate_answer(pixel_values=pixel_values, input_ids=input_ids, attention_mask=attention_mask, max_length=20)
end = time.perf_counter()
delta = end - start
inference_time.append(delta)
return np.median(inference_time)
%%skip not $to_quantize.value
fp_original_model = BlipForQuestionAnswering.from_pretrained("Salesforce/blip-vqa-base")
fp_text_decoder = fp_original_model.text_decoder
fp_text_decoder.eval()
comp_text_encoder = core.compile_model(TEXT_ENCODER_OV, device.value)
comp_text_decoder_with_past = core.compile_model(TEXT_DECODER_OV, device.value)
fp_text_decoder.forward = partial(text_decoder_forward,
ov_text_decoder_with_past=comp_text_decoder_with_past)
fp16_model = OVBlipModel(model.config, model.decoder_start_token_id, comp_vision_model,
comp_text_encoder, fp_text_decoder)
%%skip not $to_quantize.value
validation_data = calibration_data[:100]
int8_caption_latency = calculate_inference_time(int8_model, validation_data, generate_caption=True)
fp16_caption_latency = calculate_inference_time(fp16_model, validation_data, generate_caption=True)
print(f"Image Captioning speed up: {fp16_caption_latency / int8_caption_latency:.3f}")
Image Captioning speed up: 1.254
%%skip not $to_quantize.value
int8_generate_answer_latency = calculate_inference_time(int8_model, validation_data, generate_caption=False)
fp16_generate_answer_latency = calculate_inference_time(fp16_model, validation_data, generate_caption=False)
print(f"Question Answering speed up: {fp16_generate_answer_latency / int8_generate_answer_latency:.3f}")
Question Answering speed up: 1.715
インタラクティブなデモ#
インタラクティブなデモを起動するため量子化モデルを使用するかどうか以下で選択してください。
use_quantized_model = widgets.Checkbox(
description="Use quantized model",
value=int8_model is not None,
disabled=int8_model is None,
)
use_quantized_model
Checkbox(value=True, description='Use quantized model')
import gradio as gr
ov_model = int8_model if use_quantized_model.value else ov_model
def generate_answer(img, question):
if img is None:
raise gr.Error("Please upload an image or choose one from the examples list")
start = time.perf_counter()
inputs = processor(img, question, return_tensors="pt")
output = ov_model.generate_answer(**inputs, max_length=20) if len(question) else ov_model.generate_caption(inputs["pixel_values"], max_length=20)
answer = processor.decode(output[0], skip_special_tokens=True)
elapsed = time.perf_counter() - start
html = f"<p>Processing time: {elapsed:.4f}</p>"
return answer, html
demo = gr.Interface(
generate_answer,
[
gr.Image(label="Image"),
gr.Textbox(
label="Question",
info="If this field is empty, an image caption will be generated",
),
],
[gr.Text(label="Answer"), gr.HTML()],
examples=[["demo.jpg", ""], ["demo.jpg", question]],
allow_flagging="never",
)
try:
demo.launch(debug=False)
except Exception:
demo.launch(share=True, debug=False)
# リモートで起動する場合は、server_name と server_port を指定
# demo.launch(server_name='your server name', server_port='server port in int')
# 詳細はドキュメントをご覧ください: https://gradio.app/docs/