Stable Cascade と OpenVINO による画像生成#
この Jupyter ノートブックは、ローカルへのインストール後にのみ起動できます。
Stable Cascade は Würstchen アーキテクチャーに基づいて構築されており、Stable Diffusion などの他のモデルとの主な違いは、はるかに小さな潜在空間で動作することです。ツールキットの重要性潜在空間が小さいほど、推論の実行速度が速くなり、トレーニングのコストも低くなります。潜在空間はどれほど小さいのでしょう? Stable Diffusion では圧縮係数 8 が使用され、1024x1024 の画像が 128x128 にエンコードされます。Stable Cascade は 42 の圧縮係数を実現します。つまり、鮮明な再構成を維持しながら 1024x1024 の画像を 24x24 にエンコードすることができます。次に、テキスト条件付きモデルは、高度に圧縮された潜在空間でトレーニングされます。
目次:
必要条件#
%pip install -q "diffusers>=0.27.0" accelerate datasets gradio transformers "nncf>=2.10.0" "openvino>=2024.1.0" "torch>=2.1" --extra-index-url https://download.pytorch.org/whl/cpu
Note: you may need to restart the kernel to use updated packages.
元のパイプラインをロードして実行#
import torch
from diffusers import StableCascadeDecoderPipeline, StableCascadePriorPipeline
prompt = "an image of a shiba inu, donning a spacesuit and helmet"
negative_prompt = ""
prior = StableCascadePriorPipeline.from_pretrained("stabilityai/stable-cascade-prior", torch_dtype=torch.float32)
decoder = StableCascadeDecoderPipeline.from_pretrained("stabilityai/stable-cascade", torch_dtype=torch.float32)
2024-07-13 03:34:38.692876: I tensorflow/core/util/port.cc:110] oneDNN custom operations are on.You may see slightly different numerical results due to floating-point round-off errors from different computation orders.To turn them off, set the environment variable TF_ENABLE_ONEDNN_OPTS=0. 2024-07-13 03:34:38.727848: I tensorflow/core/platform/cpu_feature_guard.cc:182] This TensorFlow binary is optimized to use available CPU instructions in performance-critical operations.To enable the following instructions: AVX2 AVX512F AVX512_VNNI FMA, in other operations, rebuild TensorFlow with the appropriate compiler flags.2024-07-13 03:34:39.399629: W tensorflow/compiler/tf2tensorrt/utils/py_utils.cc:38] TF-TRT Warning: Could not find TensorRT /opt/home/k8sworker/ci-ai/cibuilds/ov-notebook/OVNotebookOps-727/.workspace/scm/ov-notebook/.venv/lib/python3.8/site-packages/diffusers/models/vq_model.py:20: FutureWarning: VQEncoderOutput is deprecated and will be removed in version 0.31. Importing VQEncoderOutput from diffusers.models.vq_model is deprecated and this will be removed in a future version. Please use from diffusers.models.autoencoders.vq_model import VQEncoderOutput, instead. deprecate("VQEncoderOutput", "0.31", deprecation_message) /opt/home/k8sworker/ci-ai/cibuilds/ov-notebook/OVNotebookOps-727/.workspace/scm/ov-notebook/.venv/lib/python3.8/site-packages/diffusers/models/vq_model.py:25: FutureWarning: VQModel is deprecated and will be removed in version 0.31. Importing VQModel from diffusers.models.vq_model is deprecated and this will be removed in a future version. Please use from diffusers.models.autoencoders.vq_model import VQModel, instead. deprecate("VQModel", "0.31", deprecation_message)
Loading pipeline components...: 0%| | 0/6 [00:00<?, ?it/s]
The installed version of bitsandbytes was compiled without GPU support.8-bit optimizers, 8-bit multiplication, and GPU quantization are unavailable.
Loading pipeline components...: 0%| | 0/5 [00:00<?, ?it/s]
メモリー使用量を削減するために、元の推論をスキップします。必要であれば、スキップを無効にできます。
import ipywidgets as widgets
run_original_inference = widgets.Checkbox(
value=False,
description="Run original inference",
disabled=False,
)
run_original_inference
Checkbox(value=False, description='Run original inference')
if run_original_inference.value:
prior.to(torch.device("cpu"))
prior_output = prior(
prompt=prompt,
height=1024,
width=1024,
negative_prompt=negative_prompt,
guidance_scale=4.0,
num_images_per_prompt=1,
num_inference_steps=20,
)
decoder_output = decoder(
image_embeddings=prior_output.
image_embeddings, prompt=prompt,
negative_prompt=negative_prompt,
guidance_scale=0.0,
output_type="pil",
num_inference_steps=10,
).images[0]
display(decoder_output)
モデルを OpenVINO IR に変換#
主なモデル・コンポーネント: - 事前ステージ prior
: テキスト条件付き LDM を使用して画像の低次元潜在空間表現を作成します
- デコーダーステージ decoder
: 事前ステージからの表現を使用して、別の LDM を使用して高次元の潜在空間に潜在画像を生成し、VQGAN デコーダーを使用して潜在画像をデコードしてフル解像度の出力画像を生成します
PyTorch モジュールの変換関数を定義します。ov.convert_model
関数を使用して OpenVINO 中間表現オブジェクトを取得し、ov.save_model
関数でそれを XML ファイルとして保存します。モデルのサイズを縮小するため、nncf.compress_weights
を使用してモデルの重みを 8 ビットに圧縮します。
import gc
from pathlib import Path
import openvino as ov
import nncf
MODELS_DIR = Path("models")
def convert(model: torch.nn.Module, xml_path: str, example_input, input_shape=None):
xml_path = Path(xml_path)
if not xml_path.exists():
model.eval()
xml_path.parent.mkdir(parents=True, exist_ok=True)
with torch.no_grad():
if not input_shape:
converted_model = ov.convert_model(model, example_input=example_input)
else:
converted_model = ov.convert_model(model, example_input=example_input, input=input_shape)
converted_model = nncf.compress_weights(converted_model)
ov.save_model(converted_model, xml_path)
del converted_model
# メモリーをクリーンアップ
torch._C._jit_clear_class_registry()
torch.jit._recursive.concrete_type_store = torch.jit._recursive.ConcreteTypeStore()
torch.jit._state._clear_class_state()
gc.collect()
INFO:nncf:NNCF initialized successfully.Supported frameworks detected: torch, tensorflow, onnx, openvino
以前のパイプライン#
このパイプラインは、テキスト・エンコーダーと事前拡散モデルで構成されています。ここからは、input_shape
パラメーターを使用して変換時に常に固定形状を使用し、メモリーをあまり消費しないモデルを生成します。
PRIOR_TEXT_ENCODER_OV_PATH = MODELS_DIR / "prior_text_encoder_model.xml"
prior.text_encoder.config.output_hidden_states = True
class TextEncoderWrapper(torch.nn.Module):
def __init__(self, text_encoder):
super().__init__()
self.text_encoder = text_encoder
def forward(self, input_ids, attention_mask):
outputs = self.text_encoder(input_ids=input_ids, attention_mask=attention_mask, output_hidden_states=True)
return outputs["text_embeds"], outputs["last_hidden_state"], outputs["hidden_states"]
convert(
TextEncoderWrapper(prior.text_encoder),
PRIOR_TEXT_ENCODER_OV_PATH,
example_input={
"input_ids": torch.zeros(1, 77, dtype=torch.int32),
"attention_mask": torch.zeros(1, 77),
},
input_shape={"input_ids": ((1, 77),),"attention_mask": ((1, 77),)},
)
del prior.text_encoder
gc.collect();
WARNING:tensorflow:Please fix your imports.Module tensorflow.python.training.tracking.base has been moved to tensorflow.python.trackable.base.The old module will be deleted in version 2.11.
[ WARNING ] Please fix your imports.Module %s has been moved to %s.The old module will be deleted in version %s./opt/home/k8sworker/ci-ai/cibuilds/ov-notebook/OVNotebookOps-727/.workspace/scm/ov-notebook/.venv/lib/python3.8/site-packages/transformers/modeling_utils.py:4371: FutureWarning: _is_quantized_training_enabled is going to be deprecated in transformers 4.39.0.Please use model.hf_quantizer.is_trainable instead warnings.warn( /opt/home/k8sworker/ci-ai/cibuilds/ov-notebook/OVNotebookOps-727/.workspace/scm/ov-notebook/.venv/lib/python3.8/site-packages/transformers/models/distilbert/modeling_distilbert.py:86: TracerWarning: Converting a tensor to a Python boolean might cause the trace to be incorrect. We can't record the data flow of Python values, so this value will be treated as a constant in the future. This means that the trace might not generalize to other inputs! if input_shape[-1] > 1 or self.sliding_window is not None: /opt/home/k8sworker/ci-ai/cibuilds/ov-notebook/OVNotebookOps-727/.workspace/scm/ov-notebook/.venv/lib/python3.8/site-packages/transformers/modeling_attn_mask_utils.py:162: TracerWarning: Converting a tensor to a Python boolean might cause the trace to be incorrect.We can't record the data flow of Python values, so this value will be treated as a constant in the future.This means that the trace might not generalize to other inputs! if past_key_values_length > 0: /opt/home/k8sworker/ci-ai/cibuilds/ov-notebook/OVNotebookOps-727/.workspace/scm/ov-notebook/.venv/lib/python3.8/site-packages/transformers/models/clip/modeling_clip.py:279: TracerWarning: Converting a tensor to a Python boolean might cause the trace to be incorrect.We can't record the data flow of Python values, so this value will be treated as a constant in the future.This means that the trace might not generalize to other inputs! if attn_weights.size() != (bsz * self.num_heads, tgt_len, src_len): /opt/home/k8sworker/ci-ai/cibuilds/ov-notebook/OVNotebookOps-727/.workspace/scm/ov-notebook/.venv/lib/python3.8/site-packages/transformers/models/clip/modeling_clip.py:287: TracerWarning: Converting a tensor to a Python boolean might cause the trace to be incorrect.We can't record the data flow of Python values, so this value will be treated as a constant in the future.This means that the trace might not generalize to other inputs! if causal_attention_mask.size() != (bsz, 1, tgt_len, src_len): /opt/home/k8sworker/ci-ai/cibuilds/ov-notebook/OVNotebookOps-727/.workspace/scm/ov-notebook/.venv/lib/python3.8/site-packages/transformers/models/clip/modeling_clip.py:296: TracerWarning: Converting a tensor to a Python boolean might cause the trace to be incorrect.We can't record the data flow of Python values, so this value will be treated as a constant in the future.This means that the trace might not generalize to other inputs! if attention_mask.size() != (bsz, 1, tgt_len, src_len): /opt/home/k8sworker/ci-ai/cibuilds/ov-notebook/OVNotebookOps-727/.workspace/scm/ov-notebook/.venv/lib/python3.8/site-packages/transformers/models/clip/modeling_clip.py:319: TracerWarning: Converting a tensor to a Python boolean might cause the trace to be incorrect.We can't record the data flow of Python values, so this value will be treated as a constant in the future.This means that the trace might not generalize to other inputs! if attn_output.size() != (bsz * self.num_heads, tgt_len, self.head_dim):
['input_ids', 'attention_mask']
INFO:nncf:Statistics of the bitwidth distribution:
┍━━━━━━━━━━━━━━━━┯━━━━━━━━━━━━━━━━━━━━━━━━━━━━━┯━━━━━━━━━━━━━━━━━━━━━━━━━━━━━━━━━━━━━━━━┑
│ Num bits (N) │ % all parameters (layers) │ % ratio-defining parameters (layers) │
┝━━━━━━━━━━━━━━━━┿━━━━━━━━━━━━━━━━━━━━━━━━━━━━━┿━━━━━━━━━━━━━━━━━━━━━━━━━━━━━━━━━━━━━━━━┥
│ 8 │ 100% (194 / 194) │ 100% (194 / 194) │
┕━━━━━━━━━━━━━━━━┷━━━━━━━━━━━━━━━━━━━━━━━━━━━━━┷━━━━━━━━━━━━━━━━━━━━━━━━━━━━━━━━━━━━━━━━┙
Output()
PRIOR_PRIOR_MODEL_OV_PATH = MODELS_DIR / "prior_prior_model.xml"
convert(
prior.prior,
PRIOR_PRIOR_MODEL_OV_PATH,
example_input={
"sample": torch.zeros(2, 16, 24, 24),
"timestep_ratio": torch.ones(2),
"clip_text_pooled": torch.zeros(2, 1, 1280),
"clip_text": torch.zeros(2, 77, 1280),
"clip_img": torch.zeros(2, 1, 768),
},
input_shape=[((-1, 16, 24, 24),), ((-1),), ((-1, 1, 1280),), ((-1, 77, 1280),), (-1, 1, 768)],
)
del prior.prior
gc.collect();
/opt/home/k8sworker/ci-ai/cibuilds/ov-notebook/OVNotebookOps-727/.workspace/scm/ov-notebook/.venv/lib/python3.8/site-packages/diffusers/models/unets/unet_stable_cascade.py:550: TracerWarning: Converting a tensor to a Python boolean might cause the trace to be incorrect.We can't record the data flow of Python values, so this value will be treated as a constant in the future.This means that the trace might not generalize to other inputs!
if skip is not None and (x.size(-1) != skip.size(-1) or x.size(-2) != skip.size(-2)):
['sample', 'timestep_ratio', 'clip_text_pooled', 'clip_text', 'clip_img']
INFO:nncf:Statistics of the bitwidth distribution:
┍━━━━━━━━━━━━━━━━┯━━━━━━━━━━━━━━━━━━━━━━━━━━━━━┯━━━━━━━━━━━━━━━━━━━━━━━━━━━━━━━━━━━━━━━━┑
│ Num bits (N) │ % all parameters (layers) │ % ratio-defining parameters (layers) │
┝━━━━━━━━━━━━━━━━┿━━━━━━━━━━━━━━━━━━━━━━━━━━━━━┿━━━━━━━━━━━━━━━━━━━━━━━━━━━━━━━━━━━━━━━━┥
│ 8 │ 100% (711 / 711) │ 100% (711 / 711) │
┕━━━━━━━━━━━━━━━━┷━━━━━━━━━━━━━━━━━━━━━━━━━━━━━┷━━━━━━━━━━━━━━━━━━━━━━━━━━━━━━━━━━━━━━━━┙
Output()
デコーダー・パイプライン#
デコーダー・パイプラインは、デコーダー、テキスト・エンコーダー、VQGAN の 3 つの部分で構成されます。
DECODER_TEXT_ENCODER_MODEL_OV_PATH = MODELS_DIR / "decoder_text_encoder_model.xml"
convert(
TextEncoderWrapper(decoder.text_encoder),
DECODER_TEXT_ENCODER_MODEL_OV_PATH,
example_input={
"input_ids": torch.zeros(1, 77, dtype=torch.int32),
"attention_mask": torch.zeros(1, 77),
},
input_shape={"input_ids": ((1, 77),),"attention_mask": ((1, 77),)},
)
del decoder.text_encoder
gc.collect();
['input_ids', 'attention_mask']
INFO:nncf:Statistics of the bitwidth distribution:
┍━━━━━━━━━━━━━━━━┯━━━━━━━━━━━━━━━━━━━━━━━━━━━━━┯━━━━━━━━━━━━━━━━━━━━━━━━━━━━━━━━━━━━━━━━┑
│ Num bits (N) │ % all parameters (layers) │ % ratio-defining parameters (layers) │
┝━━━━━━━━━━━━━━━━┿━━━━━━━━━━━━━━━━━━━━━━━━━━━━━┿━━━━━━━━━━━━━━━━━━━━━━━━━━━━━━━━━━━━━━━━┥
│ 8 │ 100% (194 / 194) │ 100% (194 / 194) │
┕━━━━━━━━━━━━━━━━┷━━━━━━━━━━━━━━━━━━━━━━━━━━━━━┷━━━━━━━━━━━━━━━━━━━━━━━━━━━━━━━━━━━━━━━━┙
Output()
DECODER_DECODER_MODEL_OV_PATH = MODELS_DIR / "decoder_decoder_model.xml"
convert(
decoder.decoder,
DECODER_DECODER_MODEL_OV_PATH,
example_input={
"sample": torch.zeros(1, 4, 256, 256),
"timestep_ratio": torch.ones(1),
"clip_text_pooled": torch.zeros(1, 1, 1280),
"effnet": torch.zeros(1, 16, 24, 24),
},
input_shape=[((-1, 4, 256, 256),), ((-1),), ((-1, 1, 1280),), ((-1, 16, 24, 24),)],
)
del decoder.decoder
gc.collect();
['sample', 'timestep_ratio', 'clip_text_pooled', 'effnet']
INFO:nncf:Statistics of the bitwidth distribution:
┍━━━━━━━━━━━━━━━━┯━━━━━━━━━━━━━━━━━━━━━━━━━━━━━┯━━━━━━━━━━━━━━━━━━━━━━━━━━━━━━━━━━━━━━━━┑
│ Num bits (N) │ % all parameters (layers) │ % ratio-defining parameters (layers) │
┝━━━━━━━━━━━━━━━━┿━━━━━━━━━━━━━━━━━━━━━━━━━━━━━┿━━━━━━━━━━━━━━━━━━━━━━━━━━━━━━━━━━━━━━━━┥
│ 8 │ 100% (855 / 855) │ 100% (855 / 855) │
┕━━━━━━━━━━━━━━━━┷━━━━━━━━━━━━━━━━━━━━━━━━━━━━━┷━━━━━━━━━━━━━━━━━━━━━━━━━━━━━━━━━━━━━━━━┙
Output()
VQGAN_PATH = MODELS_DIR / "vqgan_model.xml"
class VqganDecoderWrapper(torch.nn.Module):
def __init__(self, vqgan):
super().__init__()
self.vqgan = vqgan
def forward(self, h):
return self.vqgan.decode(h)
convert(
VqganDecoderWrapper(decoder.vqgan),
VQGAN_PATH,
example_input=torch.zeros(1, 4, 256, 256),
input_shape=(1, 4, 256, 256),
)
del decoder.vqgan
gc.collect();
['h']
INFO:nncf:Statistics of the bitwidth distribution:
┍━━━━━━━━━━━━━━━━┯━━━━━━━━━━━━━━━━━━━━━━━━━━━━━┯━━━━━━━━━━━━━━━━━━━━━━━━━━━━━━━━━━━━━━━━┑
│ Num bits (N) │ % all parameters (layers) │ % ratio-defining parameters (layers) │
┝━━━━━━━━━━━━━━━━┿━━━━━━━━━━━━━━━━━━━━━━━━━━━━━┿━━━━━━━━━━━━━━━━━━━━━━━━━━━━━━━━━━━━━━━━┥
│ 8 │ 100% (42 / 42) │ 100% (42 / 42) │
┕━━━━━━━━━━━━━━━━┷━━━━━━━━━━━━━━━━━━━━━━━━━━━━━┷━━━━━━━━━━━━━━━━━━━━━━━━━━━━━━━━━━━━━━━━┙
Output()
推論デバイスの選択#
OpenVINO を使用して推論を実行するデバイスをドロップダウン・リストから選択します。
core = ov.Core()
device = widgets.Dropdown(
options=core.available_devices + ["AUTO"],
value="AUTO",
description="Device:",
disabled=False,
)
device
Dropdown(description='Device:', index=1, options=('CPU', 'AUTO'), value='AUTO')
パイプラインの構築#
元のパイプラインとの対話を可能にするため、コンパイルされたモデルの呼び出し可能なラッパークラスを作成します。すべてのラッパークラスは np.array
ではなく torch.Tensor
を返すことに注意してください。
from collections import namedtuple
BaseModelOutputWithPooling = namedtuple("BaseModelOutputWithPooling", ["text_embeds", "last_hidden_state", "hidden_states"])
class TextEncoderWrapper:
dtype = torch.float32 # accessed in the original workflow
def __init__(self, text_encoder_path, device):
self.text_encoder = core.compile_model(text_encoder_path, device.value)
def __call__(self, input_ids, attention_mask, output_hidden_states=True):
output = self.text_encoder({"input_ids": input_ids, "attention_mask": attention_mask})
text_embeds = output[0]
last_hidden_state = output[1]
hidden_states = list(output.values())[1:]
return BaseModelOutputWithPooling(torch.from_numpy(text_embeds), torch.from_numpy(last_hidden_state), [torch.from_numpy(hs) for hs in hidden_states])
class PriorPriorWrapper:
def __init__(self, prior_path, device):
self.prior = core.compile_model(prior_path, device.value)
self.config = namedtuple("PriorWrapperConfig", ["clip_image_in_channels", "in_channels"])(768, 16) # 元のワークフローでアクセス
self.parameters = lambda: (torch.zeros(i, dtype=torch.float32) for i in range(1)) # 元のワークフローでアクセス
def __call__(self, sample, timestep_ratio, clip_text_pooled, clip_text=None, clip_img=None, **kwargs):
inputs = {
"sample": sample,
"timestep_ratio": timestep_ratio,
"clip_text_pooled": clip_text_pooled,
"clip_text": clip_text,
"clip_img": clip_img,
}
output = self.prior(inputs)
return [torch.from_numpy(output[0])]
class DecoderWrapper:
dtype = torch.float32 # 元のワークフローでアクセス
def __init__(self, decoder_path, device):
self.decoder = core.compile_model(decoder_path, device.value)
def __call__(self, sample, timestep_ratio, clip_text_pooled, effnet, **kwargs):
inputs = {"sample": sample, "timestep_ratio": timestep_ratio, "clip_text_pooled": clip_text_pooled, "effnet": effnet}
output = self.decoder(inputs)
return [torch.from_numpy(output[0])]
VqganOutput = namedtuple("VqganOutput", "sample")
class VqganWrapper:
config = namedtuple("VqganWrapperConfig", "scale_factor")(0.3764) # 元のワークフローでアクセス
def __init__(self, vqgan_path, device):
self.vqgan = core.compile_model(vqgan_path, device.value)
def decode(self, h):
output = self.vqgan(h)[0]
output = torch.tensor(output)
return VqganOutput(output)
パイプラインにラッパー・インスタンスを挿入:
prior.text_encoder = TextEncoderWrapper(PRIOR_TEXT_ENCODER_OV_PATH, device)
prior.prior = PriorPriorWrapper(PRIOR_PRIOR_MODEL_OV_PATH, device)
decoder.decoder = DecoderWrapper(DECODER_DECODER_MODEL_OV_PATH, device)
decoder.text_encoder = TextEncoderWrapper(DECODER_TEXT_ENCODER_MODEL_OV_PATH, device)
decoder.vqgan = VqganWrapper(VQGAN_PATH, device)
推論#
prior_output = prior(
prompt=prompt,
height=1024,
width=1024,
negative_prompt=negative_prompt,
guidance_scale=4.0,
num_images_per_prompt=1,
num_inference_steps=20,
)
decoder_output = decoder(
image_embeddings=prior_output.image_embeddings,
prompt=prompt,
negative_prompt=negative_prompt,
guidance_scale=0.0,
output_type="pil",
num_inference_steps=10,
).images[0]
display(decoder_output)
0%| | 0/20 [00:00<?, ?it/s]
0%| | 0/10 [00:00<?, ?it/s]
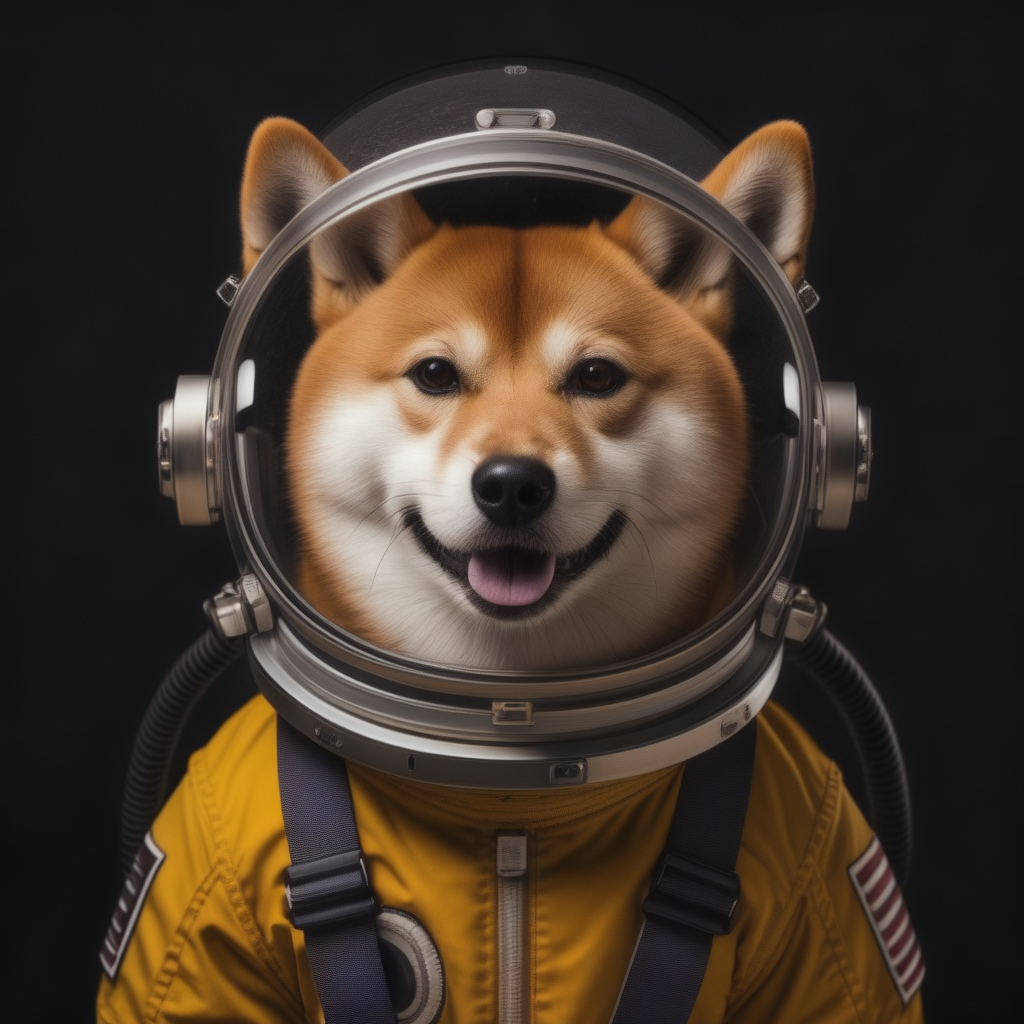
インタラクティブな推論#
def generate(prompt, negative_prompt, prior_guidance_scale, decoder_guidance_scale, seed):
generator = torch.Generator().manual_seed(seed)
prior_output = prior(
prompt=prompt,
height=1024,
width=1024,
negative_prompt=negative_prompt,
guidance_scale=prior_guidance_scale,
num_images_per_prompt=1,
num_inference_steps=20,
generator=generator,
)
decoder_output = decoder(
image_embeddings=prior_output.image_embeddings,
prompt=prompt,
negative_prompt=negative_prompt,
guidance_scale=decoder_guidance_scale,
output_type="pil",
num_inference_steps=10,
generator=generator,
).images[0]
return decoder_output
import gradio as gr
import numpy as np
demo = gr.Interface(
generate,
[
gr.Textbox(label="Prompt"),
gr.Textbox(label="Negative prompt"),
gr.Slider(
0,
20,
step=1,
label="Prior guidance scale",
info="Higher guidance scale encourages to generate images that are closely "
"linked to the text `prompt`, usually at the expense of lower image quality.Applies to the prior pipeline",
),
gr.Slider(
0,
20,
step=1,
label="Decoder guidance scale",
info="Higher guidance scale encourages to generate images that are closely "
"linked to the text `prompt`, usually at the expense of lower image quality.Applies to the decoder pipeline",
),
gr.Slider(0, np.iinfo(np.int32).max, label="Seed", step=1),
],
"image",
examples=[["An image of a shiba inu, donning a spacesuit and helmet", "", 4, 0, 0], ["An armchair in the shape of an avocado", "", 4, 0, 0]],
allow_flagging="never",
)
try:
demo.queue().launch(debug=False)
except Exception:
demo.queue().launch(debug=False, share=True)
# リモートで起動する場合は、server_name と server_port を指定
# demo.launch(server_name='your server name', server_port='server port in int')
# 詳細はドキュメントをご覧ください: https://gradio.app/docs/
ローカル URL で実行中: http://127.0.0.1:7860 パブリックリンクを作成するには、launch() で share=True を設定します。