Bark と OpenVINO を使用したテキスト読み上げの生成#
この Jupyter ノートブックは、ローカルへのインストール後にのみ起動できます。
🐶 Bark は、Suno によって作成されたトランスフォーマー・ベースのテキストから音声へのモデルです。Bark は、音楽、バックグラウンド・ノイズ、シンプルなサウンド効果など、リアルな多言語音声やその他のオーディオを生成できます。このモデルは、笑ったり、ため息をついたり、泣いたりといった非言語的なコミュニケーションも生み出すことができます。
Bark を使用すると、ユーザーは笑ったり、ため息をついたり、泣いたりといった非言語的なコミュニケーションも行えるため、さまざまな用途に利用可能な多目的ツールになります。
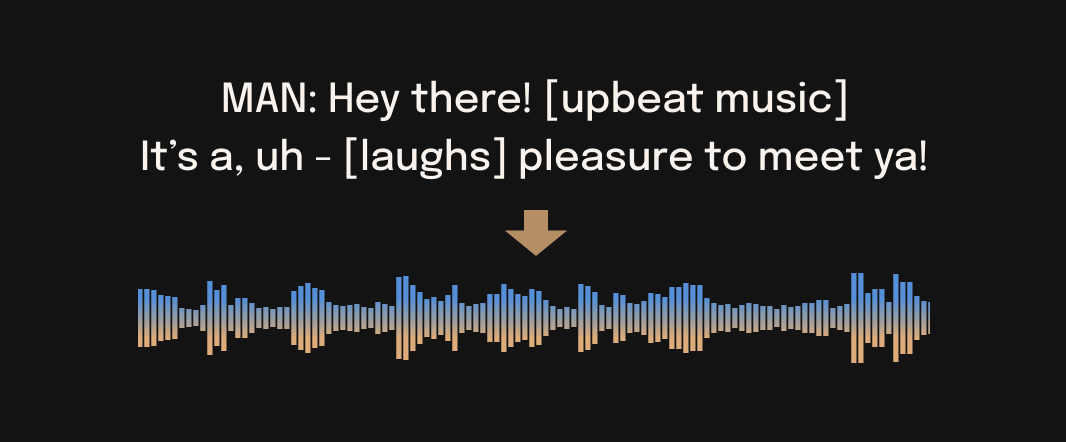
image.png#
Bark は、AI の世界に旋風を巻き起こした最先端のテキスト読み上げ (TTS) テクノロジーです。ロボットのような機械的な音声を発する一般的な TTS エンジンとは異なり、Bark は人間のような音声によって非常にリアルで自然な発話を実現します。Bark は GPT スタイルのモデルを使用して、最小限の調整で音声を生成し、トーン、ピッチ、リズムなどのニュアンスを捉えることができる、表現力豊かで感情的な音声を生成します。人間の話を聞いているのか疑問に思うほど素晴らしい体験を提供します。
特に、Bark は複数の言語をサポートしており、中国語、フランス語、イタリア語、スペイン語などの言語で、驚くほど明瞭かつ正確に音声を生成できます。Bark を使用すると、言語を簡単に切り替えながら、高品質のサウンド効果を楽しむことができます。
Bark はインテリジェントであるだけでなく直感的でもあるため、プラットフォーム向けに高品質の音声コンテンツを作成したい個人や企業にとって理想的なツールです。ポッドキャスト、オーディオブック、ビデオゲームのサウンド、またはその他の形式の音声コンテンツの作成にも Bark が対応します。
したがって、音声コンテンツを向上させる革新的なテキスト読み上げテクノロジーを探しているなら、Bark が最適です。このチュートリアルでは、OpenVINO を使用して bark を変換して実行する方法について説明します。
モデルについて#
Bark は GPT スタイルのモデルを使用して最初からオーディオを生成しますが、最初のテキストプロンプトは音素を使用せずに高レベルのセマンティック・トークンに埋め込まれます。これにより、Bark は、音楽の歌詞、効果音、その他の非音声音など、トレーニング・データで発生する音声以外の任意の指示に一般化できるようになります。
後続の 2 番目のモデルは、生成されたセマンティック・トークンをオーディオ・コーデック・トークンに変換して、完全な波形を生成するのに使用されます。コミュニティーがパブリックコード経由で Bark を使用できるようにするため、オーディオ表現として Facebook の EnCodec コーデックが使用されます。
目次:
必要条件#
%pip install -q "torch" "torchvision" "torchaudio" --extra-index-url https://download.pytorch.org/whl/cpu
%pip install -q "openvino>=2023.1.0" "gradio>=4.19"
%pip install -q "git+https://github.com/suno-ai/bark.git" --extra-index-url https://download.pytorch.org/whl/cpu
Note: you may need to restart the kernel to use updated packages.
[notice] A new release of pip is available: 23.3.2 -> 24.0
[notice] To update, run: pip install --upgrade pip
Note: you may need to restart the kernel to use updated packages.
[notice] A new release of pip is available: 23.3.2 -> 24.0
[notice] To update, run: pip install --upgrade pip
Note: you may need to restart the kernel to use updated packages.
モデルのダウンロードと変換#
from pathlib import Path
from bark.generation import load_model, codec_decode, _flatten_codebooks
models_dir = Path("models")
models_dir.mkdir(exist_ok=True)
テキスト・エンコーダー#
テキスト・エンコーダーは、初期テキストプロンプトを高レベルのセマンティック・トークンに埋め込む役割を担います。トークナイザーを使用して入力テキストをトークン ID に変換し、テキストの意味を捉えるセマンティック・テキスト・トークンを予測します。最初のステップと他のステップでのテキスト・エンコーダーの動作にはいくつかの違いがあります。別々のモデルを使用する必要があるのはそのためです。
text_use_small = True
text_encoder = load_model(model_type="text", use_gpu=False, use_small=text_use_small, force_reload=False)
text_encoder_model = text_encoder["model"]
tokenizer = text_encoder["tokenizer"]
import torch
import openvino as ov
text_model_suffix = "_small" if text_use_small else ""
text_model_dir = models_dir / f"text_encoder{text_model_suffix}"
text_model_dir.mkdir(exist_ok=True)
text_encoder_path1 = text_model_dir / "bark_text_encoder_1.xml"
text_encoder_path0 = text_model_dir / "bark_text_encoder_0.xml"
class TextEncoderModel(torch.nn.Module):
def __init__(self, encoder):
super().__init__()
self.encoder = encoder
def forward(self, idx, past_kv=None):
return self.encoder(idx, merge_context=True, past_kv=past_kv, use_cache=True)
if not text_encoder_path0.exists() or not text_encoder_path1.exists():
text_encoder_exportable = TextEncoderModel(text_encoder_model)
ov_model = ov.convert_model(text_encoder_exportable, example_input=torch.ones((1, 513), dtype=torch.int64))
ov.save_model(ov_model, text_encoder_path0)
logits, kv_cache = text_encoder_exportable(torch.ones((1, 513), dtype=torch.int64))
ov_model = ov.convert_model(
text_encoder_exportable,
example_input=(torch.ones((1, 1), dtype=torch.int64), kv_cache),
)
ov.save_model(ov_model, text_encoder_path1)
del ov_model
del text_encoder_exportable
del text_encoder_model, text_encoder
/home/ea/work/my_optimum_intel/optimum_env/lib/python3.8/site-packages/bark/model.py:176: TracerWarning: Converting a tensor to a Python boolean might cause the trace to be incorrect.We can't record the data flow of Python values, so this value will be treated as a constant in the future.This means that the trace might not generalize to other inputs!
assert(idx.shape[1] >= 256+256+1)
/home/ea/work/my_optimum_intel/optimum_env/lib/python3.8/site-packages/bark/model.py:199: TracerWarning: Converting a tensor to a Python boolean might cause the trace to be incorrect.We can't record the data flow of Python values, so this value will be treated as a constant in the future.This means that the trace might not generalize to other inputs!
assert position_ids.shape == (1, t)
/home/ea/work/my_optimum_intel/optimum_env/lib/python3.8/site-packages/bark/model.py:172: TracerWarning: Converting a tensor to a Python boolean might cause the trace to be incorrect.We can't record the data flow of Python values, so this value will be treated as a constant in the future.This means that the trace might not generalize to other inputs!
assert t == 1
/home/ea/work/my_optimum_intel/optimum_env/lib/python3.8/site-packages/torch/jit/_trace.py:160: UserWarning: The .grad attribute of a Tensor that is not a leaf Tensor is being accessed.Its .grad attribute won't be populated during autograd.backward().If you indeed want the .grad field to be populated for a non-leaf Tensor, use .retain_grad() on the non-leaf Tensor.If you access the non-leaf Tensor by mistake, make sure you access the leaf Tensor instead.See github.com/pytorch/pytorch/pull/30531 for more informations.(Triggered internally at aten/src/ATen/core/TensorBody.h:489.)
if a.grad is not None:
粗粒度エンコーダー#
粗粒度エンコーダーは、テキスト・エンコーダー・モデルの結果を入力として受け取る因果的な自己回帰トランスフォーマーです。EnCodec に必要な最初の 2 つのオーディオ・コードブックを予測することを目的としています。粗粒度エンコーダーは自己回帰モデルです。つまり、次のステップの予測を行うため、前のステップからの独自の出力を使用します。モデルの複雑さと最適化を軽減するため、アテンション・ブロックのキーと値のキャッシュを使用できます。 past_key_values には、前のステップからモデル内の各アテンション・モジュールの事前計算されたアテンション・キーと値のセットが含まれます。これらはステップごとに変更されないため、現在のステップの更新のみを計算して前のステップに結合することができます。最初の推論のためにモデルが分離されることを避けるために、モデルに “過去” がない場合は、最初のステップで空のテンソルを提供します。
coarse_use_small = True
coarse_model = load_model(
model_type="coarse",
use_gpu=False,
use_small=coarse_use_small,
force_reload=False,
)
coarse_model_suffix = "_small" if coarse_use_small else ""
coarse_model_dir = models_dir / f"coarse{coarse_model_suffix}"
coarse_model_dir.mkdir(exist_ok=True)
coarse_encoder_path = coarse_model_dir / "bark_coarse_encoder.xml"
import types
class CoarseEncoderModel(torch.nn.Module):
def __init__(self, encoder):
super().__init__()
self.encoder = encoder
def forward(self, idx, past_kv=None):
return self.encoder(idx, past_kv=past_kv, use_cache=True)
def casual_self_attention_forward(self, x, past_kv=None, use_cache=False):
B, T, C = x.size() # バッチサイズ、シーケンスの長さ、埋め込み次元数 (n_embd)
# バッチ内のすべてのヘッドのクエリー、キー、値を計算し、ヘッドをバッチ dim まで前方に移動
q, k, v = self.c_attn(x).split(self.n_embd, dim=2)
k = k.view(B, T, self.n_head, C // self.n_head).transpose(1, 2) # (B, nh, T, hs)
q = q.view(B, T, self.n_head, C // self.n_head).transpose(1, 2) # (B, nh, T, hs)
v = v.view(B, T, self.n_head, C // self.n_head).transpose(1, 2) # (B, nh, T, hs)
past_len = 0
if past_kv is not None:
past_key = past_kv[0]
past_value = past_kv[1]
k = torch.cat((past_key, k), dim=-2)
v = torch.cat((past_value, v), dim=-2)
past_len = past_key.shape[-2]
FULL_T = k.shape[-2]
if use_cache is True:
present = (k, v)
else:
present = None
# 因果的自己注意; 自己注意: (B, nh, T, hs) x (B, nh, hs, T) -> (B, nh, T, T)
if self.flash:
# フラッシュ・アテンションを使用した効率的な注意
full_attention_mask = torch.ones(
B,
T,
T,
device=x.device,
dtype=torch.float,
) * float("-inf")
full_attention_mask.triu_(diagonal=1)
if past_len > 0:
full_attention_mask = torch.cat(
(
torch.zeros(B, T, past_len, device=x.device),
full_attention_mask,
),
dim=-1,
)
y = torch.nn.functional.scaled_dot_product_attention(q, k, v, dropout_p=self.dropout, attn_mask=full_attention_mask)
else:
# アテンションの手動実装
att = (q @ k.transpose(-2, -1)) * (1.0 / math.sqrt(k.size(-1)))
att = att.masked_fill(self.bias[:, :, FULL_T - T : FULL_T, :FULL_T] == 0, float("-inf"))
att = F.softmax(att, dim=-1)
att = self.attn_dropout(att)
y = att @ v # (B, nh, T, T) x (B, nh, T, hs) -> (B, nh, T, hs)
y = y.transpose(1, 2).contiguous().view(B, T, C) # すべてのヘッド出力を並べて再組み立て
# 出力投影
y = self.resid_dropout(self.c_proj(y))
return (y, present)
if not coarse_encoder_path.exists():
coarse_encoder_exportable = CoarseEncoderModel(coarse_model)
for block in coarse_encoder_exportable.encoder.transformer.h:
block.attn.forward = types.MethodType(casual_self_attention_forward, block.attn)
logits, kv_cache = coarse_encoder_exportable(torch.ones((1, 886), dtype=torch.int64))
ov_model = ov.convert_model(
coarse_encoder_exportable,
example_input=(torch.ones((1, 1), dtype=torch.int64), kv_cache),
)
ov.save_model(ov_model, coarse_encoder_path)
del ov_model
del coarse_encoder_exportable
del coarse_model
/tmp/ipykernel_1046533/1432960018.py:47: TracerWarning: Converting a tensor to a Python boolean might cause the trace to be incorrect.We can't record the data flow of Python values, so this value will be treated as a constant in the future.This means that the trace might not generalize to other inputs!
if past_len > 0:
fine_use_small = False
fine_model = load_model(model_type="fine", use_gpu=False, use_small=fine_use_small, force_reload=False)
fine_model_suffix = "_small" if fine_use_small else ""
fine_model_dir = models_dir / f"fine_model{fine_model_suffix}"
fine_model_dir.mkdir(exist_ok=True)
class FineModel(torch.nn.Module):
def __init__(self, model):
super().__init__()
self.model = model
def forward(self, pred_idx, idx):
b, t, codes = idx.size()
pos = torch.arange(0, t, dtype=torch.long).unsqueeze(0) # 形状 (1, t)
# GPT モデル自体を転送
tok_embs = [wte(idx[:, :, i]).unsqueeze(-1) for i, wte in enumerate(self.model.transformer.wtes)] # 形状 (b, t, n_embd) のトークン埋め込み
tok_emb = torch.cat(tok_embs, dim=-1)
pos_emb = self.model.transformer.wpe(pos) # 形状 (1, t, n_embd) の位置埋め込み
x = tok_emb[:, :, :, : pred_idx + 1].sum(dim=-1)
x = self.model.transformer.drop(x + pos_emb)
for block in self.model.transformer.h:
x = block(x)
x = self.model.transformer.ln_f(x)
return x
fine_feature_extractor_path = fine_model_dir / "bark_fine_feature_extractor.xml"
細粒度エンコーダー#
細粒度エンコーダーは、粗いエンコーダーを使用して取得された以前のコードブックの埋め込みの合計に基づいて、最後のコードブックを反復的に予測する非因果的なオート・エンコーダー・トランスフォーマーです。
if not fine_feature_extractor_path.exists():
lm_heads = fine_model.lm_heads
fine_feature_extractor = FineModel(fine_model)
feature_extractor_out = fine_feature_extractor(3, torch.zeros((1, 1024, 8), dtype=torch.int32))
ov_model = ov.convert_model(
fine_feature_extractor,
example_input=(
torch.ones(1, dtype=torch.long),
torch.zeros((1, 1024, 8), dtype=torch.long),
),
)
ov.save_model(ov_model, fine_feature_extractor_path)
for i, lm_head in enumerate(lm_heads):
ov.save_model(
ov.convert_model(lm_head, example_input=feature_extractor_out),
fine_model_dir / f"bark_fine_lm_{i}.xml",
)
推論パイプラインの準備#
使いやすさを向上させるために、モデルを操作するクラスが提供されています。
class OVBarkTextEncoder:
def __init__(self, core, device, model_path1, model_path2):
self.compiled_model1 = core.compile_model(model_path1, device)
self.compiled_model2 = core.compile_model(model_path2, device)
def __call__(self, input_ids, past_kv=None):
if past_kv is None:
outputs = self.compiled_model1(input_ids, share_outputs=True)
else:
outputs = self.compiled_model2([input_ids, *past_kv], share_outputs=True)
logits, kv_cache = self.postprocess_outputs(outputs, past_kv is None)
return logits, kv_cache
def postprocess_outputs(self, outs, is_first_stage):
net_outs = self.compiled_model1.outputs if is_first_stage else self.compiled_model2.outputs
logits = outs[net_outs[0]]
kv_cache = []
for out_tensor in net_outs[1:]:
kv_cache.append(outs[out_tensor])
return logits, kv_cache
class OVBarkEncoder:
def __init__(self, core, device, model_path):
self.compiled_model = core.compile_model(model_path, device)
def __call__(self, idx, past_kv=None):
if past_kv is None:
past_kv = self._init_past_kv()
outs = self.compiled_model([idx, *past_kv], share_outputs=True)
return self.postprocess_outputs(outs)
def postprocess_outputs(self, outs):
net_outs = self.compiled_model.outputs
logits = outs[net_outs[0]]
kv_cache = []
for out_tensor in net_outs[1:]:
kv_cache.append(outs[out_tensor])
return logits, kv_cache
def _init_past_kv(self):
inputs = []
for input_t in self.compiled_model.inputs[1:]:
input_shape = input_t.partial_shape
input_shape[0] = 1
input_shape[2] = 0
inputs.append(ov.Tensor(ov.Type.f32, input_shape.get_shape()))
return inputs
class OVBarkFineEncoder:
def __init__(self, core, device, model_dir, num_lm_heads=7):
self.feats_compiled_model = core.compile_model(model_dir / "bark_fine_feature_extractor.xml", device)
self.feats_out = self.feats_compiled_model.output(0)
lm_heads = []
for i in range(num_lm_heads):
lm_heads.append(core.compile_model(model_dir / f"bark_fine_lm_{i}.xml", device))
self.lm_heads = lm_heads
def __call__(self, pred_idx, idx):
feats = self.feats_compiled_model([ov.Tensor(pred_idx), ov.Tensor(idx)])[self.feats_out]
lm_id = pred_idx - 1
logits = self.lm_heads[int(lm_id)](feats)[0]
return logits
generate_audio
関数は、オーディオ生成プロセスを開始するメイン関数です。ユーザーが提供する入力テキストとオプションの履歴プロンプトを受け入れ、推論パイプラインを実行します。推論パイプラインは、以下の図に示すように、いくつかのステップで構成されています:
bark_pipeline#
テキスト・エンコーダーを使用して入力テキストからセマンティック・トークンを生成します
粗粒度エンコーダーを使用して意味トークンから粗い音響コードブックを生成します
細粒度エンコーダーを使用して粗いコードブックから音響コードブックを生成します
コードブックをオーディオ波形にデコードします
from typing import Optional, Union, Dict
import tqdm
import numpy as np
def generate_audio(
text: str,
history_prompt: Optional[Union[Dict, str]] = None,
text_temp: float = 0.7, waveform_temp: float = 0.7,
silent: bool = False,
):
"""Generate audio array from input text.
Args:
text: text to be turned into audio
history_prompt: history choice for audio cloning
text_temp: generation temperature (1.0 more diverse, 0.0 more conservative)
waveform_temp: generation temperature (1.0 more diverse, 0.0 more conservative)
silent: disable progress bar
Returns:
numpy audio array at sample frequency 24khz
"""
semantic_tokens = text_to_semantic(
text,
history_prompt=history_prompt,
temp=text_temp,
silent=silent,
)
out = semantic_to_waveform(
semantic_tokens,
history_prompt=history_prompt,
temp=waveform_temp,
silent=silent,
)
return out
def text_to_semantic(
text: str,
history_prompt: Optional[Union[Dict, str]] = None,
temp: float = 0.7,
silent: bool = False,
):
"""Generate semantic array from text.
Args:
text: text to be turned into audio
history_prompt: history choice for audio cloning
temp: generation temperature (1.0 more diverse, 0.0 more conservative)
silent: disable progress bar
Returns:
numpy semantic array to be fed into `semantic_to_waveform`
"""
x_semantic = generate_text_semantic(
text,
history_prompt=history_prompt,
temp=temp,
silent=silent,
)
return x_semantic
from bark.generation import (
_load_history_prompt,
_tokenize,
_normalize_whitespace,
TEXT_PAD_TOKEN,
TEXT_ENCODING_OFFSET,
SEMANTIC_VOCAB_SIZE,
SEMANTIC_PAD_TOKEN,
SEMANTIC_INFER_TOKEN,
COARSE_RATE_HZ,
SEMANTIC_RATE_HZ,
N_COARSE_CODEBOOKS,
COARSE_INFER_TOKEN,
CODEBOOK_SIZE,
N_FINE_CODEBOOKS,
COARSE_SEMANTIC_PAD_TOKEN,
)
import torch.nn.functional as F
from typing import List, Optional, Union, Dict
def generate_text_semantic(
text: str,
history_prompt: List[str] = None,
temp: float = 0.7,
top_k: int = None,
top_p: float = None,
silent: bool = False,
min_eos_p: float = 0.2,
max_gen_duration_s: int = None,
allow_early_stop: bool = True,
):
"""
Generate semantic tokens from text.
Args:
text: text to be turned into audio
history_prompt: history choice for audio cloning
temp: generation temperature (1.0 more diverse, 0.0 more conservative)
top_k: top k number of probabilities for considering during generation
top_p: top probabilities higher than p for considering during generation
silent: disable progress bar
min_eos_p: minimum probability to select end of string token
max_gen_duration_s: maximum duration for generation in seconds
allow_early_stop: allow to stop generation if maximum duration is not reached
Returns:
numpy semantic array to be fed into `semantic_to_waveform`
"""
text = _normalize_whitespace(text)
if history_prompt is not None:
history_prompt = _load_history_prompt(history_prompt)
semantic_history = history_prompt["semantic_prompt"]
else:
semantic_history = None
encoded_text = np.ascontiguousarray(_tokenize(tokenizer, text)) + TEXT_ENCODING_OFFSET
if len(encoded_text) > 256:
p = round((len(encoded_text) - 256) / len(encoded_text) * 100, 1)
logger.warning(f"warning, text too long, lopping of last {p}%")
encoded_text = encoded_text[:256]
encoded_text = np.pad(
encoded_text,
(0, 256 - len(encoded_text)),
constant_values=TEXT_PAD_TOKEN,
mode="constant",
)
if semantic_history is not None:
semantic_history = semantic_history.astype(np.int64)
# 履歴が長すぎる場合は切り取り、必要に応じて追加
semantic_history = semantic_history[-256:]
semantic_history = np.pad(
semantic_history,
(0, 256 - len(semantic_history)),
constant_values=SEMANTIC_PAD_TOKEN,
mode="constant",
)
else:
semantic_history = np.array([SEMANTIC_PAD_TOKEN] * 256)
x = np.hstack([encoded_text, semantic_history, np.array([SEMANTIC_INFER_TOKEN])]).astype(np.int64)[None]
assert x.shape[1] == 256 + 256 + 1
n_tot_steps = 768
# EOS がいつ発生するかわからないため、カスタム tqdm 更新
pbar = tqdm.tqdm(disable=silent, total=100)
pbar_state = 0
tot_generated_duration_s = 0
kv_cache = None
for n in range(n_tot_steps):
if kv_cache is not None:
x_input = x[:, [-1]]
else:
x_input = x
logits, kv_cache = ov_text_model(ov.Tensor(x_input), kv_cache)
relevant_logits = logits[0, 0, :SEMANTIC_VOCAB_SIZE]
if allow_early_stop:
relevant_logits = np.hstack((relevant_logits, logits[0, 0, [SEMANTIC_PAD_TOKEN]])) # eos
if top_p is not None:
sorted_indices = np.argsort(relevant_logits)[::-1]
sorted_logits = relevant_logits[sorted_indices]
cumulative_probs = np.cumsum(F.softmax(sorted_logits))
sorted_indices_to_remove = cumulative_probs > top_p
sorted_indices_to_remove[1:]= sorted_indices_to_remove[:-1].copy()
sorted_indices_to_remove[0] = False
relevant_logits[sorted_indices[sorted_indices_to_remove]] = -np.inf
relevant_logits = torch.from_numpy(relevant_logits)
if top_k is not None:
relevant_logits = torch.from_numpy(relevant_logits)
v, _ = torch.topk(relevant_logits, min(top_k, relevant_logits.size(-1)))
relevant_logits[relevant_logits < v[-1]] = -float("Inf")
probs = F.softmax(torch.from_numpy(relevant_logits) / temp, dim=-1)
item_next = torch.multinomial(probs, num_samples=1)
if allow_early_stop and (item_next == SEMANTIC_VOCAB_SIZE or (min_eos_p is not None and probs[-1] >= min_eos_p)): # eos found, so break
pbar.update(100 - pbar_state)
break
x = torch.cat((torch.from_numpy(x), item_next[None]), dim=1).numpy()
tot_generated_duration_s += 1 / SEMANTIC_RATE_HZ
if max_gen_duration_s is not None and tot_generated_duration_s > max_gen_duration_s:
pbar.update(100 - pbar_state)
break
if n == n_tot_steps - 1:
pbar.update(100 - pbar_state)
break
del logits, relevant_logits, probs, item_next
req_pbar_state = np.min([100, int(round(100 * n / n_tot_steps))])
if req_pbar_state > pbar_state:
pbar.update(req_pbar_state - pbar_state)
pbar_state = req_pbar_state
pbar.close()
out = x.squeeze()[256 + 256 + 1 :]
return out
def semantic_to_waveform(
semantic_tokens: np.ndarray,
history_prompt: Optional[Union[Dict, str]] = None,
temp: float = 0.7,
silent: bool = False,
):
"""Generate audio array from semantic input.
Args:
semantic_tokens: semantic token output from `text_to_semantic`
history_prompt: history choice for audio cloning
temp: generation temperature (1.0 more diverse, 0.0 more conservative)
silent: disable progress bar
Returns:
numpy audio array at sample frequency 24khz
"""
coarse_tokens = generate_coarse(
semantic_tokens,
history_prompt=history_prompt,
temp=temp,
silent=silent,
)
fine_tokens = generate_fine(
coarse_tokens,
history_prompt=history_prompt,
temp=0.5,
)
audio_arr = codec_decode(fine_tokens)
return audio_arr
def generate_coarse(
x_semantic: np.ndarray,
history_prompt: Optional[Union[Dict, str]] = None,
temp: float = 0.7,
top_k: int = None,
top_p: float = None,
silent: bool = False,
max_coarse_history: int = 630, # 最小 60 (高速)、最大 630 (より多くのコンテキスト)
sliding_window_len: int = 60,
):
"""
Generate coarse audio codes from semantic tokens.
Args:
x_semantic: semantic token output from `text_to_semantic`
history_prompt: history prompt, will be prepened to generated if provided
temp: generation temperature (1.0 more diverse, 0.0 more conservative)
top_k: top k number of probabilities for considering during generation
top_p: top probabilities higher than p for considering during generation
silent: disable progress bar
max_coarse_history: threshold for cutting coarse history (minimum 60 for faster generation, maximum 630 for more context)
sliding_window_len: size of sliding window for generation cycle
Returns:
numpy audio array with coarse audio codes
"""
semantic_to_coarse_ratio = COARSE_RATE_HZ / SEMANTIC_RATE_HZ * N_COARSE_CODEBOOKS
max_semantic_history = int(np.floor(max_coarse_history / semantic_to_coarse_ratio))
if history_prompt is not None:
history_prompt = _load_history_prompt(history_prompt)
x_semantic_history = history_prompt["semantic_prompt"]
x_coarse_history = history_prompt["coarse_prompt"]
x_coarse_history = _flatten_codebooks(x_coarse_history) + SEMANTIC_VOCAB_SIZE
# trim histories correctly
n_semantic_hist_provided = np.min(
[
max_semantic_history,
len(x_semantic_history) - len(x_semantic_history) % 2,
int(np.floor(len(x_coarse_history) / semantic_to_coarse_ratio)),
]
)
n_coarse_hist_provided = int(round(n_semantic_hist_provided * semantic_to_coarse_ratio))
x_semantic_history = x_semantic_history[-n_semantic_hist_provided:].astype(np.int32)
x_coarse_history = x_coarse_history[-n_coarse_hist_provided:].astype(np.int32)
x_coarse_history = x_coarse_history[:-2]
else:
x_semantic_history = np.array([], dtype=np.int32)
x_coarse_history = np.array([], dtype=np.int32)
# start loop
n_steps = int(round(np.floor(len(x_semantic) * semantic_to_coarse_ratio / N_COARSE_CODEBOOKS) * N_COARSE_CODEBOOKS))
x_semantic = np.hstack([x_semantic_history, x_semantic]).astype(np.int32)
x_coarse = x_coarse_history.astype(np.int32)
base_semantic_idx = len(x_semantic_history)
x_semantic_in = x_semantic[None] x_coarse_in = x_coarse[None]
n_window_steps = int(np.ceil(n_steps / sliding_window_len))
n_step = 0
for _ in tqdm.tqdm(range(n_window_steps), total=n_window_steps, disable=silent):
semantic_idx = base_semantic_idx + int(round(n_step / semantic_to_coarse_ratio))
# pad from right side
x_in = x_semantic_in[:, np.max([0, semantic_idx - max_semantic_history]) :]
x_in = x_in[:, :256]
x_in = F.pad(
torch.from_numpy(x_in),
(0, 256 - x_in.shape[-1]),
"constant",
COARSE_SEMANTIC_PAD_TOKEN,
)
x_in = torch.hstack(
[
x_in,
torch.tensor([COARSE_INFER_TOKEN])[None],
torch.from_numpy(x_coarse_in[:, -max_coarse_history:]),
]
).numpy()
kv_cache = None
for _ in range(sliding_window_len):
if n_step >= n_steps:
continue
is_major_step = n_step % N_COARSE_CODEBOOKS == 0
if kv_cache is not None:
x_input = x_in[:, [-1]]
else:
x_input = x_in
logits, kv_cache = ov_coarse_model(x_input, past_kv=kv_cache)
logit_start_idx = SEMANTIC_VOCAB_SIZE + (1 - int(is_major_step)) * CODEBOOK_SIZE
logit_end_idx = SEMANTIC_VOCAB_SIZE + (2 - int(is_major_step)) * CODEBOOK_SIZE
relevant_logits = logits[0, 0, logit_start_idx:logit_end_idx]
if top_p is not None:
sorted_indices = np.argsort(relevant_logits)[::-1]
sorted_logits = relevant_logits[sorted_indices]
cumulative_probs = np.cumsum(F.softmax(sorted_logits))
sorted_indices_to_remove = cumulative_probs > top_p
sorted_indices_to_remove[1:]= sorted_indices_to_remove[:-1].copy()
sorted_indices_to_remove[0] = False
relevant_logits[sorted_indices[sorted_indices_to_remove]] = -np.inf
relevant_logits = torch.from_numpy(relevant_logits)
if top_k is not None:
relevant_logits = torch.from_numpy(relevant_logits)
v, _ = torch.topk(relevant_logits, min(top_k, relevant_logits.size(-1)))
relevant_logits[relevant_logits < v[-1]] = -float("Inf")
probs = F.softmax(torch.from_numpy(relevant_logits) / temp, dim=-1)
item_next = torch.multinomial(probs, num_samples=1)
item_next = item_next
item_next += logit_start_idx
x_coarse_in = torch.cat((torch.from_numpy(x_coarse_in), item_next[None]), dim=1).numpy()
x_in = torch.cat((torch.from_numpy(x_in), item_next[None]), dim=1).numpy()
del logits, relevant_logits, probs, item_next
n_step += 1
del x_in
del x_semantic_in
gen_coarse_arr = x_coarse_in.squeeze()[len(x_coarse_history) :]
del x_coarse_in
gen_coarse_audio_arr = gen_coarse_arr.reshape(-1, N_COARSE_CODEBOOKS).T - SEMANTIC_VOCAB_SIZE
for n in range(1, N_COARSE_CODEBOOKS):
gen_coarse_audio_arr[n, :]-= n * CODEBOOK_SIZE
return gen_coarse_audio_arr
def generate_fine(
x_coarse_gen: np.ndarray,
history_prompt: Optional[Union[Dict, str]] = None,
temp: float = 0.5,
silent: bool = True,
):
"""
Generate full audio codes from coarse audio codes.
Args:
x_coarse_gen: generated coarse codebooks from `generate_coarse`
history_prompt: history prompt, will be prepended to generated
temp: generation temperature (1.0 more diverse, 0.0 more conservative)
silent: disable progress bar
Returns:
numpy audio array with coarse audio codes
"""
if history_prompt is not None:
history_prompt = _load_history_prompt(history_prompt)
x_fine_history = history_prompt["fine_prompt"]
else:
x_fine_history = None
n_coarse = x_coarse_gen.shape[0]
# 入力 arr を作成
in_arr = np.vstack(
[
x_coarse_gen,
np.zeros((N_FINE_CODEBOOKS - n_coarse, x_coarse_gen.shape[1])) + CODEBOOK_SIZE,
]
).astype(
np.int32
) # パディング
# 履歴がある場合は先頭に追加 (最大 512)
if x_fine_history is not None:
x_fine_history = x_fine_history.astype(np.int32)
in_arr = np.hstack([x_fine_history[:, -512:].astype(np.int32), in_arr])
n_history = x_fine_history[:, -512:].shape[1]
else:
n_history = 0
n_remove_from_end = 0
# 短すぎる場合はパディングが必要 (非因果モデルのため)
if in_arr.shape[1] < 1024:
n_remove_from_end = 1024 - in_arr.shape[1]
in_arr = np.hstack(
[
in_arr,
np.zeros((N_FINE_CODEBOOKS, n_remove_from_end), dtype=np.int32) + CODEBOOK_SIZE,
]
)
n_loops = np.max([0, int(np.ceil((x_coarse_gen.shape[1] - (1024 - n_history)) / 512))]) + 1
in_arr = in_arr.T
for n in tqdm.tqdm(range(n_loops), disable=silent):
start_idx = np.min([n * 512, in_arr.shape[0] - 1024])
start_fill_idx = np.min([n_history + n * 512, in_arr.shape[0] - 512])
rel_start_fill_idx = start_fill_idx - start_idx
in_buffer = in_arr[start_idx : start_idx + 1024, :][None]
for nn in range(n_coarse, N_FINE_CODEBOOKS):
logits = ov_fine_model(np.array([nn]).astype(np.int64), in_buffer.astype(np.int64))
if temp is None:
relevant_logits = logits[0, rel_start_fill_idx:, :CODEBOOK_SIZE]
codebook_preds = torch.argmax(relevant_logits, -1)
else:
relevant_logits = logits[0, :, :CODEBOOK_SIZE] / temp
probs = F.softmax(torch.from_numpy(relevant_logits), dim=-1)
codebook_preds = torch.hstack([torch.multinomial(probs[nnn], num_samples=1) for nnn in range(rel_start_fill_idx, 1024)])
in_buffer[0, rel_start_fill_idx:, nn] = codebook_preds.numpy()
del logits, codebook_preds
for nn in range(n_coarse, N_FINE_CODEBOOKS):
in_arr[start_fill_idx : start_fill_idx + (1024 - rel_start_fill_idx), nn] = in_buffer[0, rel_start_fill_idx:, nn]
del in_buffer
gen_fine_arr = in_arr.squeeze().T
del in_arr
gen_fine_arr = gen_fine_arr[:, n_history:]
if n_remove_from_end > 0:
gen_fine_arr = gen_fine_arr[:, :-n_remove_from_end]
return gen_fine_arr
モデルの推論を実行#
次は、モデルの動作を見てみましょう。モデルをクラスにラップし、generate_audio
関数を実行するだけです。
推論デバイスの選択#
OpenVINO を使用して推論を実行するためにドロップダウン・リストからデバイスを選択します
import ipywidgets as widgets
import openvino as ov
core = ov.Core()
device = widgets.Dropdown(
options=core.available_devices + ["AUTO"],
value="AUTO",
description="Device:",
disabled=False,
)
device
Dropdown(description='Device:', index=1, options=('CPU', 'AUTO'), value='AUTO')
core = ov.Core()
ov_text_model = OVBarkTextEncoder(core, device.value, text_encoder_path0, text_encoder_path1)
ov_coarse_model = OVBarkEncoder(core, device.value, coarse_encoder_path)
ov_fine_model = OVBarkFineEncoder(core, device.value, fine_model_dir)
import time
from bark import SAMPLE_RATE
torch.manual_seed(42)
t0 = time.time()
text = "Hello, my name is Suno. And, uh — and I like banana and apples. [laughs] But I also have other interests such as playing tic tac toe."
audio_array = generate_audio(text)
generation_duration_s = time.time() - t0
audio_duration_s = audio_array.shape[0] / SAMPLE_RATE
print(f"took {generation_duration_s:.0f}s to generate {audio_duration_s:.0f}s of audio")
100%|
███████████████████████████████████████████████████████████████████████████████████████████████████████████████████████████████████████████████████████████████████████████████████████████████████████████████████████████████████████████████| 100/100 [00:10<00:00, 9.63it/s]
100%|
█████████████████████████████████████████████████████████████████████████████████████████████████████████████████████████████████████████████████████████████████████████████████████████████████████████████████████████████████████████████████| 32/32 [00:37<00:00, 1.17s/it]
/home/ea/work/my_optimum_intel/optimum_env/lib/python3.8/site-packages/torch/nn/utils/weight_norm.py:30: UserWarning: torch.nn.utils.weight_norm is deprecated in favor of torch.nn.utils.parametrizations.weight_norm.
warnings.warn("torch.nn.utils.weight_norm is deprecated in favor of torch.nn.utils.parametrizations.weight_norm.")
took 53s to generate 13s of audio
from IPython.display import Audio
from bark import SAMPLE_RATE
Audio(audio_array, rate=SAMPLE_RATE)
インタラクティブなデモ#
import numpy as np
import gradio as gr
from bark import SAMPLE_RATE
from bark.generation import SUPPORTED_LANGS
AVAILABLE_PROMPTS = ["Unconditional", "Announcer"]
PROMPT_LOOKUP = {}
for _, lang in SUPPORTED_LANGS:
for n in range(10):
label = f"Speaker {n} ({lang})"
AVAILABLE_PROMPTS.append(label)
PROMPT_LOOKUP[label] = f"{lang}_speaker_{n}"
PROMPT_LOOKUP["Unconditional"] = None
PROMPT_LOOKUP["Announcer"] = "announcer"
default_text = "Hello, my name is Suno. And, uh — and I like pizza. [laughs]\nBut I also have other interests such as playing tic tac toe."
title = "# 🐶 Bark: Text-to-Speech using OpenVINO</div>"
description = """
Bark is a universal text-to-audio model created by [Suno](http://suno.ai).\
Bark can generate highly realistic, multilingual speech as well as other audio - including music, background noise and simple sound effects.\
The model output is not censored and the authors do not endorse the opinions in the generated content.\
Use at your own risk."""
article = """
## 🌎 Foreign Language
Bark supports various languages out-of-the-box and automatically determines language from input text.\
When prompted with code-switched text, Bark will even attempt to employ the native accent for the respective languages in the same voice.
Try the prompt:
```
Buenos días Miguel. Tu colega piensa que tu alemán es extremadamente malo. But I suppose your english isn't terrible. ```
## 🤭 Non-Speech Sounds
Below is a list of some known non-speech sounds, but we are finding more every day.\
Please let us know if you find patterns that work particularly well on Discord!
* [laughter]
* [laughs]
* [sighs]
* [music]
* [gasps]
* [clears throat]
* — or ... for hesitations
* ♪ for song lyrics
* capitalization for emphasis of a word
* MAN/WOMAN: for bias towards speaker
Try the prompt:
```
" [clears throat] Hello, my name is Suno. And, uh — and I like pizza. [laughs] But I also have other interests such as... ♪ singing ♪." ```
## 🎶 Music
Bark can generate all types of audio, and, in principle, doesn't see a difference between speech and music.\
Sometimes Bark chooses to generate text as music, but you can help it out by adding music notes around your lyrics.
Try the prompt:
```
♪ In the jungle, the mighty jungle, the lion barks tonight ♪
```
## 🧬 Voice Cloning
Bark has the capability to fully clone voices - including tone, pitch, emotion and prosody.\
The model also attempts to preserve music, ambient noise, etc. from input audio.\
However, to mitigate misuse of this technology, we limit the audio history prompts to a limited set of Suno-provided, fully synthetic options to choose from.
## 👥 Speaker Prompts
You can provide certain speaker prompts such as NARRATOR, MAN, WOMAN, etc. \
Please note that these are not always respected, especially if a conflicting audio history prompt is given.
Try the prompt:
```
WOMAN: I would like an oatmilk latte please.
MAN: Wow, that's expensive!
```
"""
examples = [
[
"Please surprise me and speak in whatever voice you enjoy.Vielen Dank und Gesundheit!",
"Unconditional",
],
[
"Hello, my name is Suno. And, uh — and I like pizza. [laughs] But I also have other interests such as playing tic tac toe.",
"Speaker 1 (en)",
],
[
"Buenos días Miguel. Tu colega piensa que tu alemán es extremadamente malo. But I suppose your english isn't terrible.",
"Speaker 0 (es)",
],
]
def gen_tts(text, history_prompt):
history_prompt = PROMPT_LOOKUP[history_prompt]
audio_arr = generate_audio(text, history_prompt=history_prompt)
audio_arr = (audio_arr * 32767).astype(np.int16)
return (SAMPLE_RATE, audio_arr)
with gr.Blocks() as block:
gr.Markdown(title)
gr.Markdown(description)
with gr.Row():
with gr.Column():
input_text = gr.Textbox(label="Input Text", lines=2, value=default_text)
options = gr.Dropdown(AVAILABLE_PROMPTS, value="Speaker 1 (en)", label="Acoustic Prompt")
run_button = gr.Button()
with gr.Column():
audio_out = gr.Audio(label="Generated Audio", type="numpy")
inputs = [input_text, options]
outputs = [audio_out]
gr.Examples(examples=examples, fn=gen_tts, inputs=inputs, outputs=outputs)
gr.Markdown(article)
run_button.click(fn=gen_tts, inputs=inputs, outputs=outputs, queue=True)
try:
block.launch(debug=False)
except Exception:
block.launch(share=True, debug=False)
# リモートで起動する場合は、server_name と server_port を指定
# demo.launch(server_name='your server name', server_port='server port in int')
# 詳細はドキュメントをご覧ください: https://gradio.app/docs/