ControlNet QR Code Monster と OpenVINO™ を使用してクリエイティブな QR コードを生成#
この Jupyter ノートブックは、ローカルへのインストール後にのみ起動できます。
最先端の画像生成技術である Stable Diffusion は、広く使用されている制御ネットワーク・アプローチである ControlNet と組み合わせることで、さらに強化することができます。この組み合わせにより、Stable Diffusion は条件入力を使用して画像生成プロセスをガイドできるため、正確で視覚的に魅力的な画像が生成されます。条件入力は、落書き、エッジマップ、ポーズ・キー・ポイント、深度マップ、セグメント化マップ、法線マップ、または生成された画像コンテンツをガイドするのに役立つ関連情報 (QR コードなど) など、さまざまなデータ形式にすることができます。この方法は、目的の結果を得るため正確な制御と微調整が必要となる複雑な画像生成シナリオで特に役立ちます。
このチュートリアルでは、monster-labs の Controlnet QR Code Monster For SD-1.5 を変換して実行する方法を学びます。追加部分では、パイプラインを高速化するため NNCF を使用して量子化を実行する方法を示します。
ControlNet、特にポーズによる条件について詳しく知りたい場合は、このチュートリアルを参照してください
目次:
必要条件#
%pip install -q accelerate diffusers transformers "torch>=2.1" "gradio>=4.19" qrcode opencv-python "peft==0.6.2" --extra-index-url https://download.pytorch.org/whl/cpu
%pip install -q "openvino>=2023.1.0" "nncf>=2.7.0"
生成パイプラインのインスタンス化#
Diffusers ライブラリーの ControlNet#
安定拡散モデルと ControlNet モデルを操作するには、Hugging Face Diffusers ライブラリーを使用します。ControlNet を実験するため、Diffuser は他の Diffuser パイプラインと同様に StableDiffusionControlNetPipeline を公開します。StableDiffusionControlNetPipeline
の中核となるのは、事前トレーニングされた拡散モデルの重みを同じに保ちながら、独自にトレーニングされた ControlNetModel インスタンスを提供できるようにする controlnet
引数です。以下のコードは、controlnet-openpose
controlnet モデルと stable-diffusion-v1-5
を使用して StableDiffusionControlNetPipeline
を作成する方法を示しています:
from diffusers import (
StableDiffusionControlNetPipeline,
ControlNetModel,
)
controlnet = ControlNetModel.from_pretrained("monster-labs/control_v1p_sd15_qrcode_monster")
pipe = StableDiffusionControlNetPipeline.from_pretrained(
"runwayml/stable-diffusion-v1-5",
controlnet=controlnet,
)
モデルを OpenVINO 中間表現 (IR) 形式に変換#
OpenVINO ov.Model
オブジェクト・インスタンスを取得するには、モデル・オブジェクト、モデルトレース用の入力データを ov.convert_model
関数に提供する必要があります。ov.save_model
関数を使用して、次回のデプロイのためにモデルをディスクに保存できます。
パイプラインは 4 つの重要なパーツで構成されます:
画像アノテーションによる調整のための ControlNet。
テキストプロンプトから画像を生成する作成条件のテキスト・エンコーダー。
段階的にノイズを除去する潜像表現のための Unet。
潜在空間を画像にデコードするオート・エンコーダー (VAE)。
import gc
from functools import partial
from pathlib import Path
from PIL import Image
import openvino as ov
import torch
def cleanup_torchscript_cache():
"""
Helper for removing cached model representation
"""
torch._C._jit_clear_class_registry()
torch.jit._recursive.concrete_type_store = torch.jit._recursive.ConcreteTypeStore()
torch.jit._state._clear_class_state()
ControlNet 変換#
ControlNet モデルは、安定拡散パイプラインの UNet と同じ入力、および追加の条件サンプル (姿勢推定器によって予測されたスケルトン・キー・ポイント・マップ) を受け入れます:
sample
- 前のステップの潜像サンプル、生成プロセスがまだ開始されていないため、ランダムノイズを使用します、timestep
- 現在のスケジューラー・ステップ、encoder_hidden_state
- テキスト・エンコーダーの非表示状態、controlnet_cond
- 条件入力アノテーション。
モデルの出力は、下および中央のブロックからのアテンションの隠れ状態であり、UNet モデルの追加コンテキストとして機能します。
controlnet_ir_path = Path("./controlnet.xml")
controlnet_inputs = {
"sample": torch.randn((2, 4, 96, 96)),
"timestep": torch.tensor(1),
"encoder_hidden_states": torch.randn((2, 77, 768)),
"controlnet_cond": torch.randn((2, 3, 768, 768)),
}
with torch.no_grad():
down_block_res_samples, mid_block_res_sample = controlnet(**controlnet_inputs, return_dict=False)
if not controlnet_ir_path.exists():
controlnet.forward = partial(controlnet.forward, return_dict=False)
with torch.no_grad():
ov_model = ov.convert_model(controlnet, example_input=controlnet_inputs)
ov.save_model(ov_model, controlnet_ir_path)
del ov_model
del pipe.controlnet, controlnet
cleanup_torchscript_cache()
print("ControlNet successfully converted to IR")
else:
del pipe.controlnet, controlnet
print(f"ControlNet will be loaded from {controlnet_ir_path}")
ControlNet will be loaded from controlnet.xml
テキスト・エンコーダー#
テキスト・エンコーダーは、入力プロンプト (例えば、“馬に乗った宇宙飛行士の写真”) を、U-Net が理解できる埋め込みスペースに変換する役割を果たします。これは通常、入力トークンのシーケンスを潜在テキスト埋め込みのシーケンスにマッピングする単純なトランスフォーマー・ベースのエンコーダーです。
テキスト・エンコーダーの入力はテンソル input_ids
です。これには、トークナイザーによって処理され、モデルによって受け入れられる最大長までパディングされたテキストからのトークン・インデックスが含まれます。モデルの出力は第 2 章テンソルです: モデルの出力は 2 つのテンソルです:
last_hidden_state
- モデル内の最後の MultiHeadtention レイヤーからの非表示状態、および pooler_out
- モデル全体の非表示状態のプールされた出力。
text_encoder_ir_path = Path("./text_encoder.xml")
if not text_encoder_ir_path.exists():
pipe.text_encoder.eval()
with torch.no_grad():
ov_model = ov.convert_model(
pipe.text_encoder, # model instance
example_input=torch.ones((1, 77), dtype=torch.long), # モデルトレースの入力
)
ov.save_model(ov_model, text_encoder_ir_path)
del ov_model
del pipe.text_encoder
cleanup_torchscript_cache()
print("Text Encoder successfully converted to IR")
else:
del pipe.text_encoder
print(f"Text Encoder will be loaded from {controlnet_ir_path}")
Text Encoder will be loaded from controlnet.xml
UNet 変換#
UNet モデル変換のプロセスは、元の安定拡散モデルと同じですが、ControlNet によって生成された新しい入力を尊重します。
from typing import Tuple
unet_ir_path = Path("./unet.xml")
dtype_mapping = {
torch.float32: ov.Type.f32,
torch.float64: ov.Type.f64,
torch.int32: ov.Type.i32,
torch.int64: ov.Type.i64,
}
def flattenize_inputs(inputs):
flatten_inputs = []
for input_data in inputs:
if input_data is None:
continue
if isinstance(input_data, (list, tuple)):
flatten_inputs.extend(flattenize_inputs(input_data))
else:
flatten_inputs.append(input_data)
return flatten_inputs
class UnetWrapper(torch.nn.Module):
def __init__(
self,
unet,
sample_dtype=torch.float32,
timestep_dtype=torch.int64,
encoder_hidden_states=torch.float32,
down_block_additional_residuals=torch.float32,
mid_block_additional_residual=torch.float32,
):
super().__init__()
self.unet = unet
self.sample_dtype = sample_dtype
self.timestep_dtype = timestep_dtype
self.encoder_hidden_states_dtype = encoder_hidden_states
self.down_block_additional_residuals_dtype = down_block_additional_residuals
self.mid_block_additional_residual_dtype = mid_block_additional_residual
def forward(
self,
sample: torch.Tensor,
timestep: torch.Tensor,
encoder_hidden_states: torch.Tensor,
down_block_additional_residuals: Tuple[torch.Tensor],
mid_block_additional_residual: torch.Tensor,
):
sample.to(self.sample_dtype)
timestep.to(self.timestep_dtype)
encoder_hidden_states.to(self.encoder_hidden_states_dtype)
down_block_additional_residuals = [res.to(self.down_block_additional_residuals_dtype) for res in down_block_additional_residuals]
mid_block_additional_residual.to(self.mid_block_additional_residual_dtype)
return self.unet(
sample,
timestep,
encoder_hidden_states,
down_block_additional_residuals=down_block_additional_residuals,
mid_block_additional_residual=mid_block_additional_residual,
)
pipe.unet.eval()
unet_inputs = {
"sample": torch.randn((2, 4, 96, 96)),
"timestep": torch.tensor(1),
"encoder_hidden_states": torch.randn((2, 77, 768)),
"down_block_additional_residuals": down_block_res_samples,
"mid_block_additional_residual": mid_block_res_sample,
}
if not unet_ir_path.exists():
with torch.no_grad():
ov_model = ov.convert_model(UnetWrapper(pipe.unet), example_input=unet_inputs)
flatten_inputs = flattenize_inputs(unet_inputs.values())
for input_data, input_tensor in zip(flatten_inputs, ov_model.inputs):
input_tensor.get_node().set_partial_shape(ov.PartialShape(input_data.shape))
input_tensor.get_node().set_element_type(dtype_mapping[input_data.dtype])
ov_model.validate_nodes_and_infer_types()
ov.save_model(ov_model, unet_ir_path)
del ov_model
cleanup_torchscript_cache()
del pipe.unet
gc.collect()
print("Unet successfully converted to IR")
else:
del pipe.unet
print(f"Unet will be loaded from {unet_ir_path}")
Unet will be loaded from unet.xml
VAE デコーダー変換#
VAE モデルには、エンコーダーとデコーダーの 2 つのパーツがあります。エンコーダーは、画像を低次元の潜在表現に変換するのに使用され、これが U-Net モデルの入力となります。逆に、デコーダーは潜在表現を変換して画像に戻します。
潜在拡散トレーニング中、エンコーダーは、順拡散プロセス用の画像の潜在表現 (潜在) を取得するために使用され、各ステップでより多くのノイズが適用されます。論中、逆拡散プロセスによって生成されたノイズ除去された潜在は、VAE デコーダーによって画像に変換されます。推論中に、VAE デコーダーのみが必要であることがわかります。エンコーダー部分を変換する方法については、安定拡散のノートブックに記載されています。
vae_ir_path = Path("./vae.xml")
class VAEDecoderWrapper(torch.nn.Module):
def __init__(self, vae):
super().__init__()
vae.eval()
self.vae = vae
def forward(self, latents):
return self.vae.decode(latents)
if not vae_ir_path.exists():
vae_decoder = VAEDecoderWrapper(pipe.vae)
latents = torch.zeros((1, 4, 96, 96))
vae_decoder.eval()
with torch.no_grad():
ov_model = ov.convert_model(vae_decoder, example_input=latents)
ov.save_model(ov_model, vae_ir_path)
del ov_model
del pipe.vae
cleanup_torchscript_cache()
print("VAE decoder successfully converted to IR")
else:
del pipe.vae
print(f"VAE decoder will be loaded from {vae_ir_path}")
VAE decoder will be loaded from vae.xml
Stable Diffusion パイプライン用の推論デバイスの選択#
OpenVINO を使用して推論を実行するためにドロップダウン・リストからデバイスを選択します
import ipywidgets as widgets
core = ov.Core()
device = widgets.Dropdown(
options=core.available_devices + ["AUTO"],
value="CPU",
description="Device:",
disabled=False,
)
device
Dropdown(description='Device:', options=('CPU', 'GPU.0', 'GPU.1', 'GPU.2', 'AUTO'), value='CPU')
推論パイプラインの準備#
Stable diffusion モデルは、潜在シードとテキストプロンプトの両方を入力として受け取ります。次に、潜在シードを使用して、サイズ
次に、U-Net モデルは、テキスト埋め込みを条件として、ランダムな潜在画像表現を繰り返しノイズ除去します。中間ブロックと下流ブロックの注意パラメーターを取得するため、各ノイズ除去ステップで ControlNet を介して渡される元の stable-diffusion パイプライン、潜在画像表現、エンコーダーの隠れ状態、および制御条件注釈と比較して、これらの注意ブロックの結果は、制御生成プロセス向けに UNet モデルに追加で提供されます。U-Net の出力はノイズ残差であり、スケジューラー・アルゴリズムを介してノイズ除去された潜在画像表現を計算するために使用されます。この計算にはさまざまなスケジューラー・アルゴリズムを使用できますが、それぞれに長所と短所があります。Stable Diffusion の場合、次のいずれかを使用することを推奨します:
スケジューラーのアルゴリズム関数が動作する理論は、このノートブックの範囲外ですが、簡単に言うと、以前のノイズ表現と予測されたノイズ残差から、予測されたノイズ除去画像表現を計算することを覚えてください。詳細については、拡散ベースの生成モデルの設計空間の解明を参照することを推奨します。
このチュートリアルでは、Stable Diffusion のデフォルトの PNDMScheduler を使用する代わりに、推奨される EulerAncestralDiscreteScheduler を使用します。スケジューラーに関する詳細は、こちらを参照してください。
ノイズ除去プロセスは、指定された回数 (デフォルトでは 50 回) 繰り返され、段階的に潜在画像表現の改善が図られます。完了すると、潜在画像表現は変分オート・エンコーダーのデコーダー部によってデコードされます。
ディフューザーの StableDiffusionControlNetPipeline
と同様に、OpenVINO に基づいて独自の OVContrlNetStableDiffusionPipeline
推論パイプラインを定義します。
from diffusers import DiffusionPipeline
from transformers import CLIPTokenizer
from typing import Union, List, Optional, Tuple
import cv2
import numpy as np
def scale_fit_to_window(dst_width: int, dst_height: int, image_width: int, image_height: int):
"""
Preprocessing helper function for calculating image size for resize with peserving original aspect ratio
and fitting image to specific window size
Parameters:
dst_width (int): destination window width
dst_height (int): destination window height
image_width (int): source image width
image_height (int): source image height
Returns:
result_width (int): calculated width for resize
result_height (int): calculated height for resize
"""
im_scale = min(dst_height / image_height, dst_width / image_width)
return int(im_scale * image_width), int(im_scale * image_height)
def preprocess(image: Image.Image):
"""
Image preprocessing function.Takes image in PIL.Image format, resizes it to keep aspect ration and fits to model input window 768x768,
then converts it to np.ndarray and adds padding with zeros on right or bottom side of image (depends from aspect ratio), after that
converts data to float32 data type and change range of values from [0, 255] to [-1, 1], finally, converts data layout from planar NHWC to NCHW.
The function returns preprocessed input tensor and padding size, which can be used in postprocessing.
Parameters:
image (Image.Image): input image
Returns:
image (np.ndarray): preprocessed image tensor
pad (Tuple[int]): pading size for each dimension for restoring image size in postprocessing
"""
src_width, src_height = image.size
dst_width, dst_height = scale_fit_to_window(768, 768, src_width, src_height)
image = image.convert("RGB")
image = np.array(image.resize((dst_width, dst_height), resample=Image.Resampling.LANCZOS))[None, :]
pad_width = 768 - dst_width
pad_height = 768 - dst_height
pad = ((0, 0), (0, pad_height), (0, pad_width), (0, 0))
image = np.pad(image, pad, mode="constant")
image = image.astype(np.float32) / 255.0
image = image.transpose(0, 3, 1, 2)
return image, pad
def randn_tensor( shape: Union[Tuple, List], dtype: Optional[np.dtype] = np.float32, ):
"""
Helper function for generation random values tensor with given shape and data type
Parameters:
shape (Union[Tuple, List]): shape for filling random values
dtype (np.dtype, *optiona*, np.float32): data type for result
Returns:
latents (np.ndarray): tensor with random values with given data type and shape (usually represents noise in latent space)
"""
latents = np.random.randn(*shape).astype(dtype)
return latents
class OVContrlNetStableDiffusionPipeline(DiffusionPipeline):
"""
OpenVINO inference pipeline for Stable Diffusion with ControlNet guidence
"""
def __init__(
self,
tokenizer: CLIPTokenizer,
scheduler,
core: ov.Core,
controlnet: ov.Model,
text_encoder: ov.Model,
unet: ov.Model,
vae_decoder: ov.Model,
device: str = "AUTO",
):
super().__init__()
self.tokenizer = tokenizer
self.vae_scale_factor = 8
self.scheduler = scheduler
self.load_models(core, device, controlnet, text_encoder, unet, vae_decoder)
self.set_progress_bar_config(disable=True)
def load_models(
self,
core: ov.Core, device: str,
controlnet: ov.Model,
text_encoder: ov.Model,
unet: ov.Model,
vae_decoder: ov.Model,
):
"""
Function for loading models on device using OpenVINO
Parameters:
core (Core): OpenVINO runtime Core class instance
device (str): inference device
controlnet (Model): OpenVINO Model object represents ControlNet
text_encoder (Model): OpenVINO Model object represents text encoder
unet (Model): OpenVINO Model object represents UNet
vae_decoder (Model): OpenVINO Model object represents vae decoder
Returns
None
"""
self.text_encoder = core.compile_model(text_encoder, device)
self.text_encoder_out = self.text_encoder.output(0)
self.register_to_config(controlnet=core.compile_model(controlnet, device))
self.register_to_config(unet=core.compile_model(unet, device))
self.unet_out = self.unet.output(0)
self.vae_decoder = core.compile_model(vae_decoder, device)
self.vae_decoder_out = self.vae_decoder.output(0)
def __call__(
self,
prompt: Union[str, List[str]],
image: Image.Image,
num_inference_steps: int = 10,
negative_prompt: Union[str, List[str]] = None,
guidance_scale: float = 7.5,
controlnet_conditioning_scale: float = 1.0,
eta: float = 0.0,
latents: Optional[np.array] = None,
output_type: Optional[str] = "pil",
):
"""
Function invoked when calling the pipeline for generation.
Parameters:
prompt (`str` or `List[str]`):
The prompt or prompts to guide the image generation.
image (`Image.Image`):
`Image`, or tensor representing an image batch which will be repainted according to `prompt`.
num_inference_steps (`int`, *optional*, defaults to 100):
The number of denoising steps.More denoising steps usually lead to a higher quality image at the
expense of slower inference.
negative_prompt (`str` or `List[str]`):
negative prompt or prompts for generation
guidance_scale (`float`, *optional*, defaults to 7.5):
Guidance scale as defined in [Classifier-Free Diffusion Guidance](https://arxiv.org/abs/2207.12598).
`guidance_scale` is defined as `w` of equation 2. of [Imagen
Paper](https://arxiv.org/pdf/2205.11487.pdf).Guidance scale is enabled by setting `guidance_scale >
1`. Higher guidance scale encourages to generate images that are closely linked to the text `prompt`,
usually at the expense of lower image quality.This pipeline requires a value of at least `1`.
latents (`np.ndarray`, *optional*):
Pre-generated noisy latents, sampled from a Gaussian distribution, to be used as inputs for image
generation. Can be used to tweak the same generation with different prompts. If not provided, a latents
tensor will ge generated by sampling using the supplied random `generator`.
output_type (`str`, *optional*, defaults to `"pil"`):
The output format of the generate image.Choose between
[PIL](https://pillow.readthedocs.io/en/stable/): `Image.Image` or `np.array`.
Returns:
image ([List[Union[np.ndarray, Image.Image]]): generaited images
"""
# 1. 呼び出しパラメーターを定義
batch_size = 1 if isinstance(prompt, str) else len(prompt)
# ここで `guidance_scale` は Imagen 論文の式 (2)
# のガイダンス重み`w`と同様に定義されます: https://arxiv.org/pdf/2205.11487.pdf`guidance_scale = 1`
# は、分類器フリーのガイダンスを行わないことに相当
do_classifier_free_guidance = guidance_scale > 1.0
# 2. 入力プロンプトをエンコード
text_embeddings = self._encode_prompt(prompt, negative_prompt=negative_prompt)
# 3. 画像の前処理
orig_width, orig_height = image.size
image, pad = preprocess(image)
height, width = image.shape[-2:]
if do_classifier_free_guidance:
image = np.concatenate(([image] * 2))
# 4. タイムステップを設定
self.scheduler.set_timesteps(num_inference_steps)
timesteps = self.scheduler.timesteps
# 6. 潜在変数を準備
num_channels_latents = 4
latents = self.prepare_latents(
batch_size,
num_channels_latents,
height,
width,
text_embeddings.dtype,
latents,
)
# 7. ノイズ除去ループ
num_warmup_steps = len(timesteps) - num_inference_steps * self.scheduler.order
with self.progress_bar(total=num_inference_steps) as progress_bar:
for i, t in enumerate(timesteps):
# 分類器フリーのガイダンスを実行する場合は潜在変数を拡張
# pix2pix ではテキストと入力画像の両方にガイダンスが適用されるため、
# 潜在変数は 3 倍に拡張されます
latent_model_input = np.concatenate([latents] * 2) if do_classifier_free_guidance else latents
latent_model_input = self.scheduler.scale_model_input(latent_model_input, t)
result = self.controlnet([latent_model_input, t, text_embeddings, image])
down_and_mid_blok_samples = [sample * controlnet_conditioning_scale for _, sample in result.items()]
# ノイズ残留を予測
noise_pred = self.unet([latent_model_input, t, text_embeddings, *down_and_mid_blok_samples])[self.unet_out]
# ガイダンスを実行
if do_classifier_free_guidance:
noise_pred_uncond, noise_pred_text = noise_pred[0], noise_pred[1]
noise_pred = noise_pred_uncond + guidance_scale * (noise_pred_text - noise_pred_uncond)
# 前のノイズサンプル x_t -> x_t-1 を計算
latents = self.scheduler.step(torch.from_numpy(noise_pred), t, torch.from_numpy(latents)).prev_sample.numpy()
# 進捗状況の更新
if i == len(timesteps) - 1 or ((i + 1) > num_warmup_steps and (i + 1) % self.scheduler.order == 0):
progress_bar.update()
# 8. 後処理
image = self.decode_latents(latents, pad)
# 9. PIL に変換
if output_type == "pil":
image = self.numpy_to_pil(image)
image = [img.resize((orig_width, orig_height), Image.Resampling.LANCZOS) for img in image]
else:
image = [cv2.resize(img, (orig_width, orig_width)) for img in image]
return image
def _encode_prompt(
self,
prompt: Union[str, List[str]],
num_images_per_prompt: int = 1,
do_classifier_free_guidance: bool = True,
negative_prompt: Union[str, List[str]] = None,
):
"""
Encodes the prompt into text encoder hidden states.
Parameters:
prompt (str or list(str)): prompt to be encoded
num_images_per_prompt (int): number of images that should be generated per prompt
do_classifier_free_guidance (bool): whether to use classifier free guidance or not
negative_prompt (str or list(str)): negative prompt to be encoded
Returns:
text_embeddings (np.ndarray): text encoder hidden states
"""
batch_size = len(prompt) if isinstance(prompt, list) else 1
# 入力プロンプトをトークン化
text_inputs = self.tokenizer(
prompt,
padding="max_length",
max_length=self.tokenizer.model_max_length,
truncation=True,
return_tensors="np",
)
text_input_ids = text_inputs.input_ids
text_embeddings = self.text_encoder(text_input_ids)[self.text_encoder_out]
# プロンプトごとに各世代のテキスト埋め込みを複製
if num_images_per_prompt != 1:
bs_embed, seq_len, _ = text_embeddings.shape
text_embeddings = np.tile(text_embeddings, (1, num_images_per_prompt, 1))
text_embeddings = np.reshape(text_embeddings, (bs_embed * num_images_per_prompt, seq_len, -1))
# 分類器の無条件埋め込みを取得する無料ガイダンス
if do_classifier_free_guidance:
uncond_tokens: List[str]
max_length = text_input_ids.shape[-1]
if negative_prompt is None:
uncond_tokens = [""] * batch_size
elif isinstance(negative_prompt, str):
uncond_tokens = [negative_prompt]
else:
uncond_tokens = negative_prompt
uncond_input = self.tokenizer(
uncond_tokens,
padding="max_length",
max_length=max_length,
truncation=True,
return_tensors="np",
)
uncond_embeddings = self.text_encoder(uncond_input.input_ids)[self.text_encoder_out]
# mps フレンドリーな方法を使用して、プロンプトごとに各世代の無条件埋め込みを複製
seq_len = uncond_embeddings.shape[1]
uncond_embeddings = np.tile(uncond_embeddings, (1, num_images_per_prompt, 1))
uncond_embeddings = np.reshape(uncond_embeddings, (batch_size * num_images_per_prompt, seq_len, -1))
# 分類器フリーのガイダンスでは、2 回のフォワードパスを実行する必要がある
# ここでは、無条件埋め込みとテキスト埋め込みを 1 つのバッチに連結して、
# 2 回のフォワードパスの実行を回避
text_embeddings = np.concatenate([uncond_embeddings, text_embeddings])
return text_embeddings
def prepare_latents(
self,
batch_size: int,
num_channels_latents: int,
height: int,
width: int,
dtype: np.dtype = np.float32,
latents: np.ndarray = None,
):
"""
Preparing noise to image generation.If initial latents are not provided, they will be generated randomly,
then prepared latents scaled by the standard deviation required by the scheduler
Parameters:
batch_size (int): input batch size
num_channels_latents (int): number of channels for noise generation
height (int): image height
width (int): image width
dtype (np.dtype, *optional*, np.float32): dtype for latents generation
latents (np.ndarray, *optional*, None): initial latent noise tensor, if not provided will be generated
Returns:
latents (np.ndarray): scaled initial noise for diffusion
"""
shape = (
batch_size,
num_channels_latents,
height // self.vae_scale_factor,
width // self.vae_scale_factor,
)
if latents is None:
latents = randn_tensor(shape, dtype=dtype)
else:
latents = latents
# スケジューラーが要求する標準偏差で初期ノイズをスケール
latents = latents * np.array(self.scheduler.init_noise_sigma)
return latents
def decode_latents(self, latents: np.array, pad: Tuple[int]):
"""
Decode predicted image from latent space using VAE Decoder and unpad image result
Parameters:
latents (np.ndarray): image encoded in diffusion latent space
pad (Tuple[int]): each side padding sizes obtained on preprocessing step
Returns:
image: decoded by VAE decoder image
"""
latents = 1 / 0.18215 * latents
image = self.vae_decoder(latents)[self.vae_decoder_out]
(_, end_h), (_, end_w) = pad[1:3]
h, w = image.shape[2:]
unpad_h = h - end_h
unpad_w = w - end_w
image = image[:, :, :unpad_h, :unpad_w]
image = np.clip(image / 2 + 0.5, 0, 1)
image = np.transpose(image, (0, 2, 3, 1))
return image
import qrcode
def create_code(content: str):
"""Creates QR codes with provided content."""
qr = qrcode.QRCode(
version=1,
error_correction=qrcode.constants.ERROR_CORRECT_H,
box_size=16,
border=0,
)
qr.add_data(content)
qr.make(fit=True)
img = qr.make_image(fill_color="black", back_color="white")
# QR に適合する 256 の倍数の最小画像サイズを検出
offset_min = 8 * 16
w, h = img.size
w = (w + 255 + offset_min) // 256 * 256
h = (h + 255 + offset_min) // 256 * 256
if w > 1024:
raise RuntimeError("QR code is too large, please use a shorter content")
bg = Image.new("L", (w, h), 128)
# 16 ピクセルのグリッドに合わせる
coords = ((w - img.size[0]) // 2 // 16 * 16, (h - img.size[1]) // 2 // 16 * 16)
bg.paste(img, coords)
return bg
from transformers import CLIPTokenizer
from diffusers import EulerAncestralDiscreteScheduler
tokenizer = CLIPTokenizer.from_pretrained("openai/clip-vit-large-patch14")
scheduler = EulerAncestralDiscreteScheduler.from_config(pipe.scheduler.config)
ov_pipe = OVContrlNetStableDiffusionPipeline(
tokenizer,
scheduler,
core,
controlnet_ir_path,
text_encoder_ir_path,
unet_ir_path,
vae_ir_path,
device=device.value,
)
実際のモデルを見てみましょう
np.random.seed(42)
qrcode_image = create_code("Hi OpenVINO")
image = ov_pipe(
"cozy town on snowy mountain slope 8k",
qrcode_image,
negative_prompt="blurry unreal occluded",
num_inference_steps=25,
guidance_scale=7.7,
controlnet_conditioning_scale=1.4,
)[0]
image
/home/ltalamanova/omz/lib/python3.8/site-packages/diffusers/configuration_utils.py:135: FutureWarning: Accessing config attribute controlnet directly via 'OVContrlNetStableDiffusionPipeline' object attribute is deprecated. Please access 'controlnet' over 'OVContrlNetStableDiffusionPipeline's config object instead, e.g. 'scheduler.config.controlnet'. deprecate("direct config name access", "1.0.0", deprecation_message, standard_warn=False)
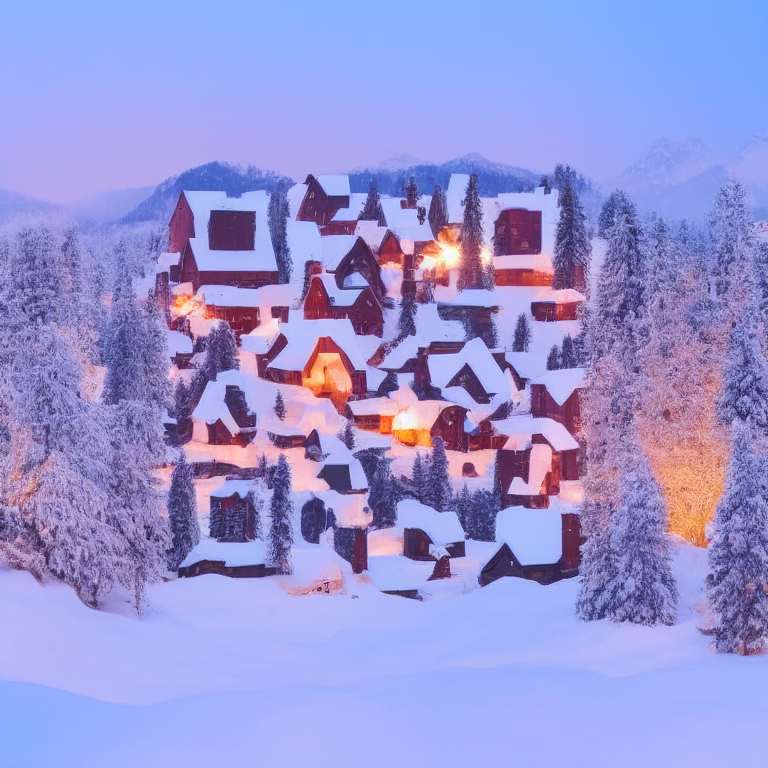
量子化#
NNCF は、量子化レイヤーをモデルグラフに追加し、トレーニング・データセットのサブセットを使用してこれらの追加の量子化レイヤーのパラメーターを初期化することで、トレーニング後の量子化を可能にします。量子化操作は FP32
/FP16
ではなく INT8
で実行されるため、モデル推論が高速化されます。
OVContrlNetStableDiffusionPipeline
構造によれば、ControlNet と UNet は各拡散ステップで推論を繰り返すサイクルで使用されますが、パイプラインの他のパーツは 1 回だけ参加します。そのため、ControlNet と UNet の計算コストと速度がパイプラインのクリティカル・パスになります。SD パイプラインの残りのパーツを量子化しても、推論パフォーマンスは大幅に向上せず、精度が大幅に低下する可能性があります。
最適化プロセスには次の手順が含まれます:
量子化用のキャリブレーション・データセットを作成します。
nncf.quantize()
を実行して、量子化されたモデルを取得します。openvino.save_model()
関数を使用してINT8
モデルを保存します。
モデルの推論速度を向上させるため量子化を実行するかどうかを以下で選択してください。
skip_for_device = "GPU" in device.value
to_quantize = widgets.Checkbox(value=not skip_for_device, description="Quantization", disabled=skip_for_device)
to_quantize
Checkbox(value=True, description='Quantization')
to_quantize
が選択されていない場合に量子化をスキップする skip magic
拡張機能をロードします
# `skip_kernel_extension` モジュールを取得
import requests
r = requests.get(
url="https://raw.githubusercontent.com/openvinotoolkit/openvino_notebooks/latest/utils/skip_kernel_extension.py",
)
open("skip_kernel_extension.py", "w").write(r.text)
int8_pipe = None
%load_ext skip_kernel_extension
キャリブレーション・データセットの準備#
ControlNet および UNet のキャリブレーション・データとして、以下のプロンプトを使用します。キャリブレーション用の中間モデル入力を収集するには、CompiledModel
をカスタマイズする必要があります。
%%skip not $to_quantize.value
text_prompts = [
"a bilboard in NYC with a qrcode",
"a samurai side profile, realistic, 8K, fantasy",
"A sky view of a colorful lakes and rivers flowing through the desert",
"Bright sunshine coming through the cracks of a wet, cave wall of big rocks",
"A city view with clouds",
"A forest overlooking a mountain",
"Sky view of highly aesthetic, ancient greek thermal baths in beautiful nature",
"A dream-like futuristic city with the light trails of cars zipping through it's many streets",
]
negative_prompts = [
"blurry unreal occluded",
"low contrast disfigured uncentered mangled",
"amateur out of frame low quality nsfw",
"ugly underexposed jpeg artifacts",
"low saturation disturbing content",
"overexposed severe distortion",
"amateur NSFW",
"ugly mutilated out of frame disfigured.",
]
qr_code_contents = [
"Hugging Face",
"pre-trained diffusion model",
"image generation technique",
"control network",
"AI QR Code Generator",
"Explore NNCF today!",
"Join OpenVINO community",
"network compression",
]
qrcode_images = [create_code(content) for content in qr_code_contents]
%%skip not $to_quantize.value
from tqdm.notebook import tqdm
from transformers import set_seed
from typing import Any, Dict, List
set_seed(1)
num_inference_steps = 25
class CompiledModelDecorator(ov.CompiledModel):
def __init__(self, compiled_model, prob: float):
super().__init__(compiled_model)
self.data_cache = [] self.prob = np.clip(prob, 0, 1)
def __call__(self, *args, **kwargs):
if np.random.rand() >= self.prob:
self.data_cache.append(*args)
return super().__call__(*args, **kwargs)
def collect_calibration_data(pipeline: OVContrlNetStableDiffusionPipeline, subset_size: int) -> List[Dict]:
original_unet = pipeline.unet
pipeline.unet = CompiledModelDecorator(original_unet, prob=0)
pipeline.set_progress_bar_config(disable=True)
pbar = tqdm(total=subset_size)
diff = 0
for prompt, qrcode_image, negative_prompt in zip(text_prompts, qrcode_images, negative_prompts): _ = pipeline(
prompt,
qrcode_image,
negative_prompt=negative_prompt,
num_inference_steps=num_inference_steps,
)
collected_subset_size = len(pipeline.unet.data_cache)
pbar.update(collected_subset_size - diff)
if collected_subset_size >= subset_size:
break
diff = collected_subset_size
calibration_dataset = pipeline.unet.data_cache
pipeline.set_progress_bar_config(disable=False)
pipeline.unet = original_unet
return calibration_dataset
%%skip not $to_quantize.value
CONTROLNET_INT8_OV_PATH = Path("controlnet_int8.xml")
UNET_INT8_OV_PATH = Path("unet_int8.xml")
if not (CONTROLNET_INT8_OV_PATH.exists() and UNET_INT8_OV_PATH.exists()):
subset_size = 200
unet_calibration_data = collect_calibration_data(ov_pipe, subset_size=subset_size)
0%| | 0/100 [00:00<?, ?it/s]
/home/ltalamanova/omz/lib/python3.8/site-packages/diffusers/configuration_utils.py:135: FutureWarning: Accessing config attribute controlnet directly via 'OVContrlNetStableDiffusionPipeline' object attribute is deprecated.Please access 'controlnet' over 'OVContrlNetStableDiffusionPipeline's config object instead, e.g. 'scheduler.config.controlnet'. deprecate("direct config name access", "1.0.0", deprecation_message, standard_warn=False)
ControlNet の最初の 3 つの入力は UNet の入力と同じで、最後の ControlNet 入力は前処理された qrcode_image
です。
%%skip not $to_quantize.value
if not CONTROLNET_INT8_OV_PATH.exists():
control_calibration_data = []
prev_idx = 0
for qrcode_image in qrcode_images:
preprocessed_image, _ = preprocess(qrcode_image)
for i in range(prev_idx, prev_idx + num_inference_steps):
control_calibration_data.append(unet_calibration_data[i][:3] + [preprocessed_image])
prev_idx += num_inference_steps
量子化を実行#
事前トレーニング済みの変換済み OpenVINO モデルから量子化モデルを作成します。SD モデルの精度向上が最小限であり、量子化時間が増加したため、FastBiasCorrection
アルゴリズムは無効になっています。
注: 量子化は時間とメモリーを消費する操作です。以下の量子化コードの実行には時間がかかる場合があります。
%%skip not $to_quantize.value
import nncf
if not UNET_INT8_OV_PATH.exists():
unet = core.read_model(unet_ir_path)
quantized_unet = nncf.quantize(
model=unet,
calibration_dataset=nncf.Dataset(unet_calibration_data),
subset_size=subset_size,
model_type=nncf.ModelType.TRANSFORMER,
advanced_parameters=nncf.AdvancedQuantizationParameters(
disable_bias_correction=True
)
)
ov.save_model(quantized_unet, UNET_INT8_OV_PATH)
%%skip not $to_quantize.value
if not CONTROLNET_INT8_OV_PATH.exists():
controlnet = core.read_model(controlnet_ir_path)
quantized_controlnet = nncf.quantize(
model=controlnet,
calibration_dataset=nncf.Dataset(control_calibration_data),
subset_size=subset_size,
model_type=nncf.ModelType.TRANSFORMER,
advanced_parameters=nncf.AdvancedQuantizationParameters(
disable_bias_correction=True
)
)
ov.save_model(quantized_controlnet, CONTROLNET_INT8_OV_PATH)
元のパイプラインと最適化されたパイプラインによって生成された画像を比較します。
%%skip not $to_quantize.value
np.random.seed(int(42))
int8_pipe = OVContrlNetStableDiffusionPipeline(tokenizer, scheduler, core, CONTROLNET_INT8_OV_PATH, text_encoder_ir_path, UNET_INT8_OV_PATH, vae_ir_path, device=device.value)
int8_image = int8_pipe(
"cozy town on snowy mountain slope 8k",
qrcode_image,
negative_prompt="blurry unreal occluded",
num_inference_steps=25,
guidance_scale=7.7,
controlnet_conditioning_scale=1.4
)[0]
%%skip not $to_quantize.value
import matplotlib.pyplot as plt
def visualize_results(orig_img:Image.Image, optimized_img:Image.Image):
"""
Helper function for results visualization
Parameters:
orig_img (Image.Image): generated image using FP16 models
optimized_img (Image.Image): generated image using quantized models
Returns:
fig (matplotlib.pyplot.Figure): matplotlib generated figure contains drawing result
"""
orig_title = "FP16 pipeline"
control_title = "INT8 pipeline"
figsize = (20, 20)
fig, axs = plt.subplots(1, 2, figsize=figsize, sharex='all', sharey='all')
list_axes = list(axs.flat)
for a in list_axes:
a.set_xticklabels([])
a.set_yticklabels([])
a.get_xaxis().set_visible(False)
a.get_yaxis().set_visible(False)
a.grid(False)
list_axes[0].imshow(np.array(orig_img))
list_axes[1].imshow(np.array(optimized_img))
list_axes[0].set_title(orig_title, fontsize=15)
list_axes[1].set_title(control_title, fontsize=15)
fig.subplots_adjust(wspace=0.01, hspace=0.01)
fig.tight_layout()
return fig
%%skip not $to_quantize.value
fig = visualize_results(image, int8_image)
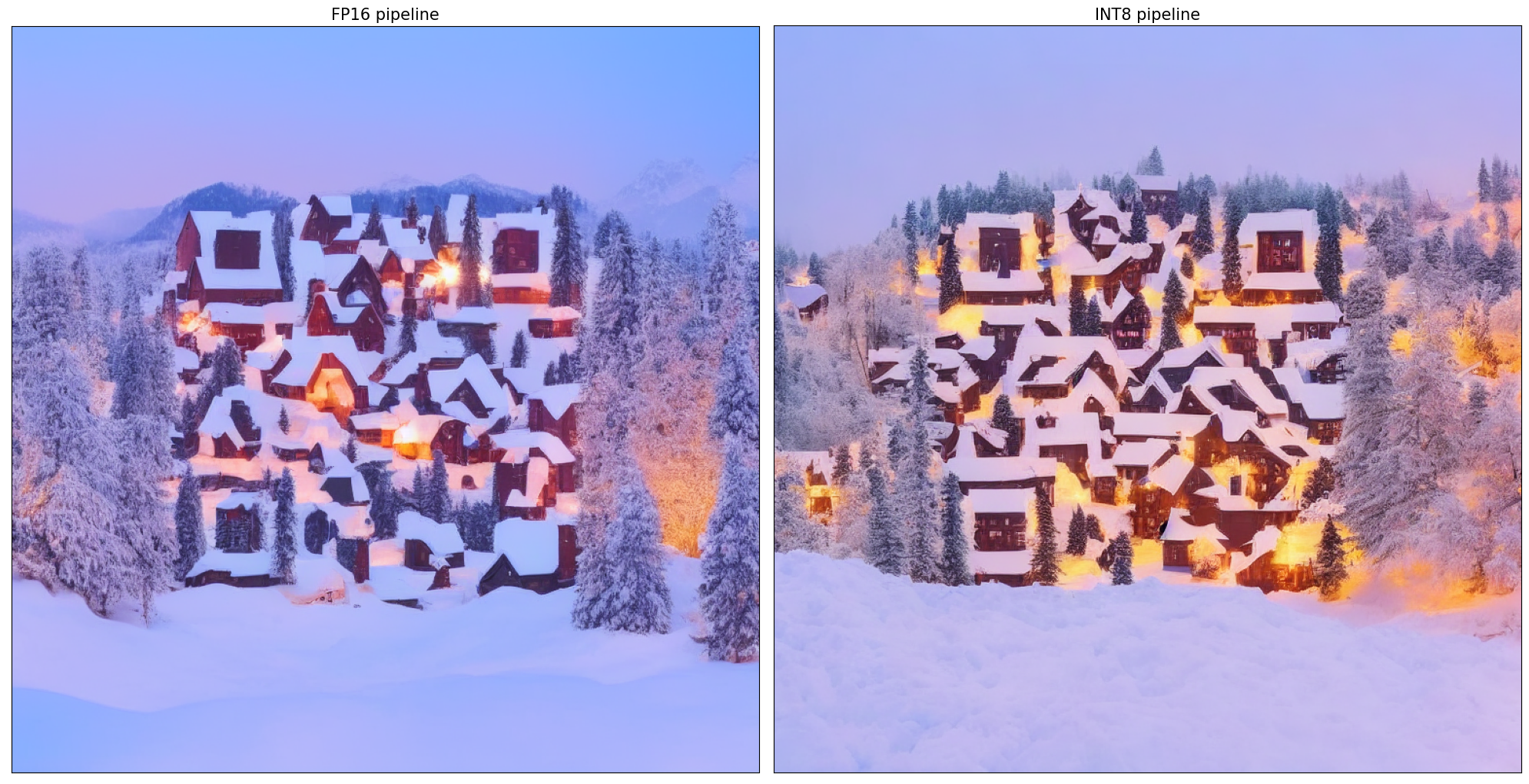
モデルのファイルサイズを比較#
%%skip not $to_quantize.value
fp16_ir_model_size = unet_ir_path.with_suffix(".bin").stat().st_size / 2**20
quantized_model_size = UNET_INT8_OV_PATH.with_suffix(".bin").stat().st_size / 2**20
print(f"FP16 UNet size: {fp16_ir_model_size:.2f} MB")
print(f"INT8 UNet size: {quantized_model_size:.2f} MB")
print(f"UNet compression rate: {fp16_ir_model_size / quantized_model_size:.3f}")
FP16 UNet size: 1639.41 MB
INT8 UNet size: 820.96 MB
UNet compression rate: 1.997
%%skip not $to_quantize.value
fp16_ir_model_size = controlnet_ir_path.with_suffix(".bin").stat().st_size / 2**20
quantized_model_size = CONTROLNET_INT8_OV_PATH.with_suffix(".bin").stat().st_size / 2**20
print(f"FP16 ControlNet size: {fp16_ir_model_size:.2f} MB")
print(f"INT8 ControlNet size: {quantized_model_size:.2f} MB")
print(f"ControlNet compression rate: {fp16_ir_model_size / quantized_model_size:.3f}")
FP16 ControlNet size: 689.09 MB
INT8 ControlNet size: 345.14 MB
ControlNet compression rate: 1.997
FP16 モデルと INT8 パイプラインの推論時間を比較#
FP16
および INT8
パイプラインの推論パフォーマンスを測定するため、3 つのサンプルの平均推論時間を使用します。
注: 最も正確なパフォーマンス推定を行うには、他のアプリケーションを閉じた後、ターミナル/コマンドプロンプトで
benchmark_app
を実行することを推奨します。
%%skip not $to_quantize.value
import time
def calculate_inference_time(pipeline):
inference_time = []
pipeline.set_progress_bar_config(disable=True)
for i in range(3):
prompt, qrcode_image = text_prompts[i], qrcode_images[i]
start = time.perf_counter()
_ = pipeline(prompt, qrcode_image, num_inference_steps=25)
end = time.perf_counter()
delta = end - start
inference_time.append(delta)
pipeline.set_progress_bar_config(disable=False)
return np.mean(inference_time)
%%skip not $to_quantize.value
fp_latency = calculate_inference_time(ov_pipe)
print(f"FP16 pipeline: {fp_latency:.3f} seconds")
int8_latency = calculate_inference_time(int8_pipe)
print(f"INT8 pipeline: {int8_latency:.3f} seconds")
print(f"Performance speed up: {fp_latency / int8_latency:.3f}")
FP16 pipeline: 190.245 seconds
INT8 pipeline: 166.540 seconds
Performance speed up: 1.142
ControlNet コンディショニングと OpenVINO を使用したテキストから画像の生成実行#
これで、生成の準備が整いました。生成プロセスを改善するために、否定プロンプトを提供する可能性も導入します。技術的には、ポジティブなプロンプトはそれに関連付けられた画像に向かって拡散を誘導し、ネガティブなプロンプトは拡散をそこから遠ざけるように誘導します。動作の仕組みの詳細については、この記事を参照してください。否定プロンプトを表示せずに画像を生成したい場合は、このフィールドを空のままにすることができます。
インタラクティブなデモを起動するため量子化モデルを使用するかどうか以下で選択してください。
quantized_model_present = int8_pipe is not None
use_quantized_model = widgets.Checkbox(
value=True if quantized_model_present else False,
description="Use quantized model",
disabled=not quantized_model_present,
)
use_quantized_model
Checkbox(value=True, description='Use quantized model')
import gradio as gr
pipeline = int8_pipe if use_quantized_model.value else ov_pipe
def _generate(
qr_code_content: str,
prompt: str,
negative_prompt: str,
seed: Optional[int] = 42,
guidance_scale: float = 10.0,
controlnet_conditioning_scale: float = 2.0,
num_inference_steps: int = 5,
progress=gr.Progress(track_tqdm=True),
):
if seed is not None:
np.random.seed(int(seed))
qrcode_image = create_code(qr_code_content)
return pipeline(
prompt,
qrcode_image,
negative_prompt=negative_prompt,
num_inference_steps=int(num_inference_steps),
guidance_scale=guidance_scale,
controlnet_conditioning_scale=controlnet_conditioning_scale,
)[0]
demo = gr.Interface(
_generate,
inputs=[
gr.Textbox(label="QR Code content"),
gr.Textbox(label="Text Prompt"),
gr.Textbox(label="Negative Text Prompt"),
gr.Number(
minimum=-1,
maximum=9999999999,
step=1,
value=42,
label="Seed",
info="Seed for the random number generator",
),
gr.Slider(
minimum=0.0,
maximum=25.0,
step=0.25,
value=7,
label="Guidance Scale",
info="Controls the amount of guidance the text prompt guides the image generation",
),
gr.Slider(
minimum=0.5,
maximum=2.5,
step=0.01,
value=1.5,
label="Controlnet Conditioning Scale",
info="""Controls the readability/creativity of the QR code.
High values: The generated QR code will be more readable.
Low values: The generated QR code will be more creative.
""",
),
gr.Slider(label="Steps", step=1, value=5, minimum=1, maximum=50),
],
outputs=["image"],
examples=[
[
"Hi OpenVINO",
"cozy town on snowy mountain slope 8k",
"blurry unreal occluded",
42,
7.7,
1.4,
25,
],
],
)
try:
demo.queue().launch(debug=False)
except Exception:
demo.queue().launch(share=True, debug=False)
# リモートで起動する場合は、server_name と server_port を指定
# 例: `demo.launch(server_name='your server name', server_port='server port in int')`
# 詳細については、Gradio のドキュメントを参照してください: https://gradio.app/docs/