aMUSEd と OpenVINO による軽量な画像生成#
この Jupyter ノートブックはオンラインで起動でき、ブラウザーのウィンドウで対話型環境を開きます。ローカルにインストールすることもできます。次のオプションのいずれかを選択します:
Amused は、muse アーキテクチャーに基づいた軽量のテキストから画像へのモデルです。Amused は、一度に大量の画像を素早く生成するなど、軽量で高速なモデルを必要とするアプリケーションに有効です。
Amused は、他の拡散モデルよりも少ない順方向パスでイメージを生成できる VQVAE トークンベースのトランスフォーマーです。Muse とは対照的に、t5-xxl の代わりに小型のテキスト・エンコーダー CLIP-L/14 を使用します。Ammused はパラメーター数が少なく、フォワードパス生成プロセスが少ないため、多くの画像を迅速に生成できます。この利点は、特に大きなバッチサイズで顕著です。
目次:
必要条件#
%pip install -q transformers "diffusers>=0.25.0" "openvino>=2023.2.0" "accelerate>=0.20.3" "gradio>=4.19" "torch>=2.1" "pillow" "torchmetrics" "torch-fidelity" --extra-index-url https://download.pytorch.org/whl/cpu
%pip install -q "nncf>=2.9.0" datasets
Note: you may need to restart the kernel to use updated packages.
Note: you may need to restart the kernel to use updated packages.
元のパイプラインをロードして実行#
import torch
from diffusers import AmusedPipeline
pipe = AmusedPipeline.from_pretrained(
"amused/amused-256",
)
prompt = "kind smiling ghost"
image = pipe(prompt, generator=torch.Generator("cpu").manual_seed(8)).images[0]
image.save("text2image_256.png")
Loading pipeline components...: 0%| | 0/5 [00:00<?, ?it/s]
0%| | 0/12 [00:00<?, ?it/s]
image
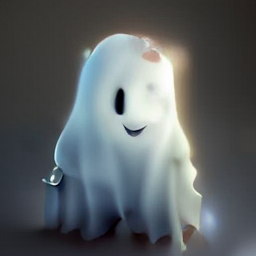
モデルを OpenVINO IR に変換#
aMUSEd は、事前トレーニングされた CLIP-L/14 テキスト・エンコーダー、VQ-GAN、および U-ViT の 3 つのコンポーネントで構成されます。

image_png#
推論では、U-ViT はテキスト・エンコーダーの隠れ状態に条件付けされ、すべてのマスクされたトークンの値を繰り返し予測します。コサイン・マスキング・スケジュールにより、反復ごとに修正される最も信頼性の高いトークン予測の割合が決定されます。12 回の反復後、すべてのトークンが予測され、VQ-GAN によって画像ピクセルにデコードされます。
変換されたモデルのパスを定義:
from pathlib import Path
TRANSFORMER_OV_PATH = Path("models/transformer_ir.xml")
TEXT_ENCODER_OV_PATH = Path("models/text_encoder_ir.xml")
VQVAE_OV_PATH = Path("models/vqvae_ir.xml")
PyTorch モジュールの変換関数を定義します。ov.convert_model
関数を使用して OpenVINO 中間表現オブジェクトを取得し、ov.save_model
関数でそれを XML ファイルとして保存します。
import torch
import openvino as ov
def convert(model: torch.nn.Module, xml_path: str, example_input):
xml_path = Path(xml_path)
if not xml_path.exists():
xml_path.parent.mkdir(parents=True, exist_ok=True)
with torch.no_grad():
converted_model = ov.convert_model(model, example_input=example_input)
ov.save_model(converted_model, xml_path, compress_to_fp16=False)
# メモリーをクリーンアップ
torch._C._jit_clear_class_registry()
torch.jit._recursive.concrete_type_store = torch.jit._recursive.ConcreteTypeStore()
torch.jit._state._clear_class_state()
テキスト・エンコーダーを変換#
class TextEncoderWrapper(torch.nn.Module):
def __init__(self, text_encoder):
super().__init__()
self.text_encoder = text_encoder
def forward(self, input_ids=None, return_dict=None, output_hidden_states=None):
outputs = self.text_encoder(
input_ids=input_ids,
return_dict=return_dict,
output_hidden_states=output_hidden_states,
)
return outputs.text_embeds, outputs.last_hidden_state, outputs.hidden_states
input_ids = pipe.tokenizer(
prompt,
return_tensors="pt",
padding="max_length",
truncation=True,
max_length=pipe.tokenizer.model_max_length,
)
input_example = {
"input_ids": input_ids.input_ids,
"return_dict": torch.tensor(True),
"output_hidden_states": torch.tensor(True),
}
convert(TextEncoderWrapper(pipe.text_encoder), TEXT_ENCODER_OV_PATH, input_example)
/opt/home/k8sworker/ci-ai/cibuilds/ov-notebook/OVNotebookOps-727/.workspace/scm/ov-notebook/.venv/lib/python3.8/site-packages/transformers/modeling_utils.py:4565: FutureWarning: _is_quantized_training_enabled is going to be deprecated in transformers 4.39.0.Please use model.hf_quantizer.is_trainable instead warnings.warn( /opt/home/k8sworker/ci-ai/cibuilds/ov-notebook/OVNotebookOps-727/.workspace/scm/ov-notebook/.venv/lib/python3.8/site-packages/transformers/models/distilbert/modeling_distilbert.py:86: TracerWarning: Converting a tensor to a Python boolean might cause the trace to be incorrect.We can't record the data flow of Python values, so this value will be treated as a constant in the future.This means that the trace might not generalize to other inputs! if input_shape[-1] > 1 or self.sliding_window is not None: /opt/home/k8sworker/ci-ai/cibuilds/ov-notebook/OVNotebookOps-727/.workspace/scm/ov-notebook/.venv/lib/python3.8/site-packages/transformers/modeling_attn_mask_utils.py:162: TracerWarning: Converting a tensor to a Python boolean might cause the trace to be incorrect.We can't record the data flow of Python values, so this value will be treated as a constant in the future.This means that the trace might not generalize to other inputs! if past_key_values_length > 0: /opt/home/k8sworker/ci-ai/cibuilds/ov-notebook/OVNotebookOps-727/.workspace/scm/ov-notebook/.venv/lib/python3.8/site-packages/transformers/models/clip/modeling_clip.py:621: TracerWarning: Converting a tensor to a Python boolean might cause the trace to be incorrect.We can't record the data flow of Python values, so this value will be treated as a constant in the future.This means that the trace might not generalize to other inputs! encoder_states = () if output_hidden_states else None /opt/home/k8sworker/ci-ai/cibuilds/ov-notebook/OVNotebookOps-727/.workspace/scm/ov-notebook/.venv/lib/python3.8/site-packages/transformers/models/clip/modeling_clip.py:626: TracerWarning: Converting a tensor to a Python boolean might cause the trace to be incorrect.We can't record the data flow of Python values, so this value will be treated as a constant in the future.This means that the trace might not generalize to other inputs! if output_hidden_states: /opt/home/k8sworker/ci-ai/cibuilds/ov-notebook/OVNotebookOps-727/.workspace/scm/ov-notebook/.venv/lib/python3.8/site-packages/transformers/models/clip/modeling_clip.py:275: TracerWarning: Converting a tensor to a Python boolean might cause the trace to be incorrect.We can't record the data flow of Python values, so this value will be treated as a constant in the future.This means that the trace might not generalize to other inputs! if attn_weights.size() != (bsz * self.num_heads, tgt_len, src_len): /opt/home/k8sworker/ci-ai/cibuilds/ov-notebook/OVNotebookOps-727/.workspace/scm/ov-notebook/.venv/lib/python3.8/site-packages/transformers/models/clip/modeling_clip.py:283: TracerWarning: Converting a tensor to a Python boolean might cause the trace to be incorrect.We can't record the data flow of Python values, so this value will be treated as a constant in the future.This means that the trace might not generalize to other inputs! if causal_attention_mask.size() != (bsz, 1, tgt_len, src_len): /opt/home/k8sworker/ci-ai/cibuilds/ov-notebook/OVNotebookOps-727/.workspace/scm/ov-notebook/.venv/lib/python3.8/site-packages/transformers/models/clip/modeling_clip.py:315: TracerWarning: Converting a tensor to a Python boolean might cause the trace to be incorrect.We can't record the data flow of Python values, so this value will be treated as a constant in the future.This means that the trace might not generalize to other inputs! if attn_output.size() != (bsz * self.num_heads, tgt_len, self.head_dim): /opt/home/k8sworker/ci-ai/cibuilds/ov-notebook/OVNotebookOps-727/.workspace/scm/ov-notebook/.venv/lib/python3.8/site-packages/transformers/models/clip/modeling_clip.py:649: TracerWarning: Converting a tensor to a Python boolean might cause the trace to be incorrect.We can't record the data flow of Python values, so this value will be treated as a constant in the future.This means that the trace might not generalize to other inputs! if output_hidden_states: /opt/home/k8sworker/ci-ai/cibuilds/ov-notebook/OVNotebookOps-727/.workspace/scm/ov-notebook/.venv/lib/python3.8/site-packages/transformers/models/clip/modeling_clip.py:652: TracerWarning: Converting a tensor to a Python boolean might cause the trace to be incorrect.We can't record the data flow of Python values, so this value will be treated as a constant in the future.This means that the trace might not generalize to other inputs! if not return_dict: /opt/home/k8sworker/ci-ai/cibuilds/ov-notebook/OVNotebookOps-727/.workspace/scm/ov-notebook/.venv/lib/python3.8/site-packages/transformers/models/clip/modeling_clip.py:744: TracerWarning: Converting a tensor to a Python boolean might cause the trace to be incorrect.We can't record the data flow of Python values, so this value will be treated as a constant in the future.This means that the trace might not generalize to other inputs! if not return_dict: /opt/home/k8sworker/ci-ai/cibuilds/ov-notebook/OVNotebookOps-727/.workspace/scm/ov-notebook/.venv/lib/python3.8/site-packages/transformers/models/clip/modeling_clip.py:1231: TracerWarning: Converting a tensor to a Python boolean might cause the trace to be incorrect.We can't record the data flow of Python values, so this value will be treated as a constant in the future.This means that the trace might not generalize to other inputs! if not return_dict:
U-ViT トランスフォーマーを変換#
class TransformerWrapper(torch.nn.Module):
def __init__(self, transformer):
super().__init__()
self.transformer = transformer
def forward(
self, latents=None,
micro_conds=None,
pooled_text_emb=None,
encoder_hidden_states=None,
):
return self.transformer(
latents,
micro_conds=micro_conds,
pooled_text_emb=pooled_text_emb,
encoder_hidden_states=encoder_hidden_states,
)
shape = (1, 16, 16)
latents = torch.full(shape, pipe.scheduler.config.mask_token_id, dtype=torch.long)
latents = torch.cat([latents] * 2)
example_input = {
"latents": latents,
"micro_conds": torch.rand([2, 5], dtype=torch.float32),
"pooled_text_emb": torch.rand([2, 768], dtype=torch.float32),
"encoder_hidden_states": torch.rand([2, 77, 768], dtype=torch.float32),
}
pipe.transformer.eval()
w_transformer = TransformerWrapper(pipe.transformer)
convert(w_transformer, TRANSFORMER_OV_PATH, example_input)
VQ-GAN デコーダー (VQVAE) を変換#
get_latents
関数は、変換用の実際の潜在を返すのに必要です。VQVAE の実装により自動生成された必要な形状のテンソルは、適切ではありません。この関数は AmusedPipeline
を部分的に繰り返します。
def get_latents():
shape = (1, 16, 16)
latents = torch.full(shape, pipe.scheduler.config.mask_token_id, dtype=torch.long)
model_input = torch.cat([latents] * 2)
model_output = pipe.transformer(
model_input,
micro_conds=torch.rand([2, 5], dtype=torch.float32),
pooled_text_emb=torch.rand([2, 768], dtype=torch.float32),
encoder_hidden_states=torch.rand([2, 77, 768], dtype=torch.float32),
)
guidance_scale = 10.0
uncond_logits, cond_logits = model_output.chunk(2)
model_output = uncond_logits + guidance_scale * (cond_logits - uncond_logits)
latents = pipe.scheduler.step(
model_output=model_output,
timestep=torch.tensor(0),
sample=latents,
).prev_sample
return latents
class VQVAEWrapper(torch.nn.Module):
def __init__(self, vqvae):
super().__init__()
self.vqvae = vqvae
def forward(self, latents=None, force_not_quantize=True, shape=None):
outputs = self.vqvae.decode(
latents,
force_not_quantize=force_not_quantize,
shape=shape.tolist(),
)
return outputs
latents = get_latents()
example_vqvae_input = {
"latents": latents,
"force_not_quantize": torch.tensor(True),
"shape": torch.tensor((1, 16, 16, 64)),
}
convert(VQVAEWrapper(pipe.vqvae), VQVAE_OV_PATH, example_vqvae_input)
/tmp/ipykernel_114139/3779428577.py:34: TracerWarning: Converting a tensor to a Python list might cause the trace to be incorrect. We can't record the data flow of Python values, so this value will be treated as a constant in the future.This means that the trace might not generalize to other inputs!
shape=shape.tolist(),
/opt/home/k8sworker/ci-ai/cibuilds/ov-notebook/OVNotebookOps-727/.workspace/scm/ov-notebook/.venv/lib/python3.8/site-packages/diffusers/models/autoencoders/vq_model.py:144: TracerWarning: Converting a tensor to a Python boolean might cause the trace to be incorrect.We can't record the data flow of Python values, so this value will be treated as a constant in the future.This means that the trace might not generalize to other inputs!
if not force_not_quantize: /opt/home/k8sworker/ci-ai/cibuilds/ov-notebook/OVNotebookOps-727/.workspace/scm/ov-notebook/.venv/lib/python3.8/site-packages/diffusers/models/upsampling.py:146: TracerWarning: Converting a tensor to a Python boolean might cause the trace to be incorrect.We can't record the data flow of Python values, so this value will be treated as a constant in the future.This means that the trace might not generalize to other inputs!
assert hidden_states.shape[1] == self.channels /opt/home/k8sworker/ci-ai/cibuilds/ov-notebook/OVNotebookOps-727/.workspace/scm/ov-notebook/.venv/lib/python3.8/site-packages/diffusers/models/upsampling.py:162: TracerWarning: Converting a tensor to a Python boolean might cause the trace to be incorrect.We can't record the data flow of Python values, so this value will be treated as a constant in the future.This means that the trace might not generalize to other inputs!
if hidden_states.shape[0] >= 64:
モデルをコンパイルしてパイプラインを準備#
OpenVINO を使用して推論を実行するデバイスをドロップダウン・リストから選択します。
import ipywidgets as widgets
core = ov.Core()
device = widgets.Dropdown(
options=core.available_devices + ["AUTO"],
value="AUTO",
description="Device:",
disabled=False,
)
device
Dropdown(description='Device:', index=1, options=('CPU', 'AUTO'), value='AUTO')
ov_text_encoder = core.compile_model(TEXT_ENCODER_OV_PATH, device.value)
ov_transformer = core.compile_model(TRANSFORMER_OV_PATH, device.value)
ov_vqvae = core.compile_model(VQVAE_OV_PATH, device.value)
元の AmusedPipeline
クラスとの対話を可能にするため、コンパイルされたモデルの呼び出し可能なラッパークラスを作成します。すべてのラッパークラスは np.array
ではなく torch.Tensor
を返すことに注意してください。
from collections import namedtuple
class ConvTextEncoderWrapper(torch.nn.Module):
def __init__(self, text_encoder, config):
super().__init__()
self.config = config
self.text_encoder = text_encoder
def forward(self, input_ids=None, return_dict=None, output_hidden_states=None):
inputs = {
"input_ids": input_ids,
"return_dict": return_dict,
"output_hidden_states": output_hidden_states,
}
outs = self.text_encoder(inputs)
outputs = namedtuple("CLIPTextModelOutput", ("text_embeds", "last_hidden_state", "hidden_states"))
text_embeds = torch.from_numpy(outs[0])
last_hidden_state = torch.from_numpy(outs[1])
hidden_states = list(torch.from_numpy(out) for out in outs.values())[2:]
return outputs(text_embeds, last_hidden_state, hidden_states)
class ConvTransformerWrapper(torch.nn.Module):
def __init__(self, transformer, config):
super().__init__()
self.config = config
self.transformer = transformer
def forward(self, latents=None, micro_conds=None, pooled_text_emb=None, encoder_hidden_states=None, **kwargs):
outputs = self.transformer(
{
"latents": latents,
"micro_conds": micro_conds,
"pooled_text_emb": pooled_text_emb,
"encoder_hidden_states": encoder_hidden_states,
},
share_inputs=False, )
return torch.from_numpy(outputs[0])
class ConvVQVAEWrapper(torch.nn.Module):
def __init__(self, vqvae, dtype, config):
super().__init__()
self.vqvae = vqvae
self.dtype = dtype
self.config = config
def decode(self, latents=None, force_not_quantize=True, shape=None):
inputs = {
"latents": latents,
"force_not_quantize": force_not_quantize,
"shape": torch.tensor(shape),
}
outs = self.vqvae(inputs)
outs = namedtuple("VQVAE", "sample")(torch.from_numpy(outs[0]))
return outs
パイプラインにラッパー・インスタンスを挿入:
prompt = "kind smiling ghost"
transformer = pipe.transformer
vqvae = pipe.vqvae
text_encoder = pipe.text_encoder
pipe.__dict__["_internal_dict"]["_execution_device"] = pipe._execution_device # this is to avoid some problem that can occur in the pipeline
pipe.register_modules(
text_encoder=ConvTextEncoderWrapper(ov_text_encoder, text_encoder.config),
transformer=ConvTransformerWrapper(ov_transformer, transformer.config),
vqvae=ConvVQVAEWrapper(ov_vqvae, vqvae.dtype, vqvae.config),
)
image = pipe(prompt, generator=torch.Generator("cpu").manual_seed(8)).images[0]
image.save("text2image_256.png")
/opt/home/k8sworker/ci-ai/cibuilds/ov-notebook/OVNotebookOps-727/.workspace/scm/ov-notebook/.venv/lib/python3.8/site-packages/diffusers/configuration_utils.py:140: FutureWarning: Accessing config attribute _execution_device directly via 'AmusedPipeline' object attribute is deprecated. Please access '_execution_device' over 'AmusedPipeline's config object instead, e.g. 'scheduler.config._execution_device'. deprecate("direct config name access", "1.0.0", deprecation_message, standard_warn=False)
0%| | 0/12 [00:00<?, ?it/s]
image
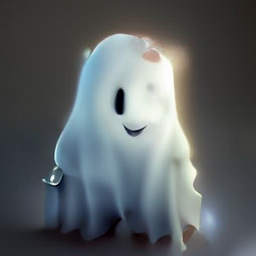
量子化#
NNCF は、量子化レイヤーをモデルグラフに追加し、トレーニング・データセットのサブセットを使用してこれらの追加の量子化レイヤーのパラメーターを初期化することで、トレーニング後の量子化を可能にします。量子化操作は FP32
/FP16
ではなく INT8
で実行されるため、モデル推論が高速化されます。
Amused
パイプライン構造によれば、ビジョン・トランスフォーマー・モデルはパイプライン全体の実行時間のほとんどを占めます。ここでは、NNCFを使用して UNet 部分を最適化し、計算コストを削減してパイプラインを高速化する方法を説明します。パイプラインの残りの部分を量子化しても、推論パフォーマンスは大幅に向上せず、生成品質が大幅に低下する可能性があります。
また、テキストから画像への生成システムの品質を測定するために用入れられる Inception スコア を使用して、最適化されたパイプラインによる生成の品質も評価します。
手順は次のとおりです:
量子化用のキャリブレーション・データセットを作成します。
モデルに対して
nncf.quantize()
を実行します。openvino.save_model()
関数で量子化されたモデルを保存します。元のパイプラインと量子化されたパイプラインの推論時間と Inception スコアを比較します。
モデルの推論速度を向上させるため量子化を実行するかどうかを以下で選択してください。
注: 量子化は時間とメモリーを消費する操作です。以下の量子化コードの実行には時間がかかる場合があります。
QUANTIZED_TRANSFORMER_OV_PATH = Path(str(TRANSFORMER_OV_PATH).replace(".xml", "_quantized.xml"))
skip_for_device = "GPU" in device.value
to_quantize = widgets.Checkbox(value=not skip_for_device, description="Quantization",
disabled=skip_for_device)
to_quantize
Checkbox(value=True, description='Quantization')
import requests
r = requests.get(
url="https://raw.githubusercontent.com/openvinotoolkit/openvino_notebooks/latest/utils/skip_kernel_extension.py",
)
open("skip_kernel_extension.py", "w").write(r.text)
%load_ext skip_kernel_extension
キャリブレーション・データセットの準備#
Hugging Face の検証 conceptual_captions データセットの一部をキャリブレーション・データとして使用します。キャリブレーション用の中間モデル入力を収集するには、CompiledModel
をカスタマイズする必要があります。
%%skip not $to_quantize.value
import datasets
from tqdm.auto import tqdm
from typing import Any, Dict, List
import pickle
import numpy as np
def disable_progress_bar(pipeline, disable=True):
if not hasattr(pipeline, "_progress_bar_config"):
pipeline._progress_bar_config = {'disable': disable}
else:
pipeline._progress_bar_config['disable'] = disable
class CompiledModelDecorator(ov.CompiledModel):
def __init__(self, compiled_model: ov.CompiledModel, data_cache: List[Any] = None, keep_prob: float = 0.5):
super().__init__(compiled_model)
self.data_cache = data_cache if data_cache is not None else []
self.keep_prob = keep_prob
def __call__(self, *args, **kwargs):
if np.random.rand() <= self.keep_prob:
self.data_cache.append(*args)
return super().__call__(*args, **kwargs)
def collect_calibration_data(ov_transformer_model, calibration_dataset_size: int) -> List[Dict]:
calibration_dataset_filepath = Path(f"calibration_data/{calibration_dataset_size}.pkl")
if not calibration_dataset_filepath.exists():
calibration_data = []
pipe.transformer.transformer = CompiledModelDecorator(ov_transformer_model, calibration_data, keep_prob=1.0)
disable_progress_bar(pipe)
dataset = datasets.load_dataset("google-research-datasets/conceptual_captions", split="train", trust_remote_code=True).shuffle(seed=42)
# データ収集のための推論を実行
pbar = tqdm(total=calibration_dataset_size)
for batch in dataset:
prompt = batch["caption"]
if len(prompt) > pipe.tokenizer.model_max_length:
continue
pipe(prompt, generator=torch.Generator('cpu').manual_seed(0))
pbar.update(len(calibration_data) - pbar.n)
if pbar.n >= calibration_dataset_size:
break
pipe.transformer.transformer = ov_transformer_model
disable_progress_bar(pipe, disable=False)
calibration_dataset_filepath.parent.mkdir(exist_ok=True, parents=True)
with open(calibration_dataset_filepath, 'wb') as f:
pickle.dump(calibration_data, f)
with open(calibration_dataset_filepath, 'rb') as f:
calibration_data = pickle.load(f)
return calibration_data
モデル量子化の実行#
キャリブレーション・データ収集を実行し、ビジョン・トランスフォーマー・モデルを量子化します。
%%skip not $to_quantize.value
from nncf.quantization.advanced_parameters import AdvancedSmoothQuantParameters
from nncf.quantization.range_estimator import RangeEstimatorParameters, StatisticsCollectorParameters,
StatisticsType, \
AggregatorType
import nncf
CALIBRATION_DATASET_SIZE = 12 * 25
if not QUANTIZED_TRANSFORMER_OV_PATH.exists():
calibration_data = collect_calibration_data(ov_transformer, CALIBRATION_DATASET_SIZE)
quantized_model = nncf.quantize(
core.read_model(TRANSFORMER_OV_PATH),
nncf.Dataset(calibration_data),
model_type=nncf.ModelType.TRANSFORMER,
subset_size=len(calibration_data),
# 畳み込みを無視することで、推論速度を大幅に低下させることなく生成の品質を向上させる
ignored_scope=nncf.IgnoredScope(types=["Convolution"]),
# 0.85 という値は、以下で計算されたインセプション・スコアに基づくグリッド検索を使用して取得
advanced_parameters=nncf.AdvancedQuantizationParameters(
smooth_quant_alphas=AdvancedSmoothQuantParameters(matmul=0.85),
# 活性化統計収集中に、外れ値の 1 % を無視することで量子化品質が向上
activations_range_estimator_params=RangeEstimatorParameters(
min=StatisticsCollectorParameters(statistics_type=StatisticsType.MIN,
aggregator_type=AggregatorType.MEAN_NO_OUTLIERS,
quantile_outlier_prob=0.01),
max=StatisticsCollectorParameters(statistics_type=StatisticsType.MAX,
aggregator_type=AggregatorType.MEAN_NO_OUTLIERS,
quantile_outlier_prob=0.01)
)
)
)
ov.save_model(quantized_model, QUANTIZED_TRANSFORMER_OV_PATH)
INFO:nncf:NNCF initialized successfully.Supported frameworks detected: torch, onnx, openvino
0%| | 0/300 [00:00<?, ?it/s]
/opt/home/k8sworker/ci-ai/cibuilds/ov-notebook/OVNotebookOps-727/.workspace/scm/ov-notebook/.venv/lib/python3.8/site-packages/diffusers/configuration_utils.py:140: FutureWarning: Accessing config attribute _execution_device directly via 'AmusedPipeline' object attribute is deprecated.Please access '_execution_device' over 'AmusedPipeline's config object instead, e.g. 'scheduler.config._execution_device'. deprecate("direct config name access", "1.0.0", deprecation_message, standard_warn=False)
Output()
Output()
INFO:nncf:3 ignored nodes were found by types in the NNCFGraph
INFO:nncf:182 ignored nodes were found by name in the NNCFGraph
INFO:nncf:Not adding activation input quantizer for operation: 120
__module.transformer.embed.conv/aten::_convolution/Convolution
INFO:nncf:Not adding activation input quantizer for operation: 2154
__module.transformer.mlm_layer.conv1/aten::_convolution/Convolution
INFO:nncf:Not adding activation input quantizer for operation: 2993
__module.transformer.mlm_layer.conv2/aten::_convolution/Convolution
Output()
/opt/home/k8sworker/ci-ai/cibuilds/ov-notebook/OVNotebookOps-727/.workspace/scm/ov-notebook/.venv/lib/python3.8/site-packages/nncf/experimental/tensor/tensor.py:92: RuntimeWarning: invalid value encountered in multiply
return Tensor(self.data * unwrap_tensor_data(other))
/opt/home/k8sworker/ci-ai/cibuilds/ov-notebook/OVNotebookOps-727/.workspace/scm/ov-notebook/.venv/lib/python3.8/site-packages/nncf/experimental/tensor/tensor.py:92: RuntimeWarning: invalid value encountered in multiply
return Tensor(self.data * unwrap_tensor_data(other))
/opt/home/k8sworker/ci-ai/cibuilds/ov-notebook/OVNotebookOps-727/.workspace/scm/ov-notebook/.venv/lib/python3.8/site-packages/nncf/experimental/tensor/tensor.py:92: RuntimeWarning: invalid value encountered in multiply
return Tensor(self.data * unwrap_tensor_data(other))
/opt/home/k8sworker/ci-ai/cibuilds/ov-notebook/OVNotebookOps-727/.workspace/scm/ov-notebook/.venv/lib/python3.8/site-packages/nncf/experimental/tensor/tensor.py:92: RuntimeWarning: invalid value encountered in multiply
return Tensor(self.data * unwrap_tensor_data(other))
/opt/home/k8sworker/ci-ai/cibuilds/ov-notebook/OVNotebookOps-727/.workspace/scm/ov-notebook/.venv/lib/python3.8/site-packages/nncf/experimental/tensor/tensor.py:92: RuntimeWarning: invalid value encountered in multiply
return Tensor(self.data * unwrap_tensor_data(other))
/opt/home/k8sworker/ci-ai/cibuilds/ov-notebook/OVNotebookOps-727/.workspace/scm/ov-notebook/.venv/lib/python3.8/site-packages/nncf/experimental/tensor/tensor.py:92: RuntimeWarning: invalid value encountered in multiply
return Tensor(self.data * unwrap_tensor_data(other))
量子化パイプラインによるデモ生成#
%%skip not $to_quantize.value
original_ov_transformer_model = pipe.transformer.transformer
pipe.transformer.transformer = core.compile_model(QUANTIZED_TRANSFORMER_OV_PATH, device.value)
image = pipe(prompt, generator=torch.Generator('cpu').manual_seed(8)).images[0]
image.save('text2image_256_quantized.png')
pipe.transformer.transformer = original_ov_transformer_model
display(image)
/opt/home/k8sworker/ci-ai/cibuilds/ov-notebook/OVNotebookOps-727/.workspace/scm/ov-notebook/.venv/lib/python3.8/site-packages/diffusers/configuration_utils.py:140: FutureWarning: Accessing config attribute _execution_device directly via 'AmusedPipeline' object attribute is deprecated.Please access '_execution_device' over 'AmusedPipeline's config object instead, e.g. 'scheduler.config._execution_device'. deprecate("direct config name access", "1.0.0", deprecation_message, standard_warn=False)
0%| | 0/12 [00:00<?, ?it/s]
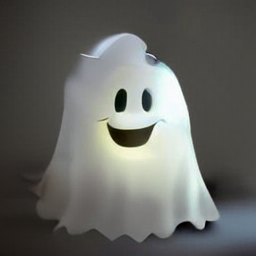
インセプション・スコアと推論時間を計算#
以下では、小さな画像のサブセットに対して、元のパイプラインと量子化されたパイプラインのインセプション・スコアを計算します。画像は、conceptual_captions
検証セットのプロンプトから生成されます。比較のため、画像の生成にかかった時間も測定します。
検証データセットのサイズは小さく、生成品質の見積もりとしてのみ機能することに注意してください。
%%skip not $to_quantize.value
from torchmetrics.image.inception import InceptionScore
from torchvision import transforms as transforms
from itertools import islice
import time
VALIDATION_DATASET_SIZE = 100
def compute_inception_score(ov_transformer_model_path, validation_set_size, batch_size=100):
original_ov_transformer_model = pipe.transformer.transformer
pipe.transformer.transformer = core.compile_model(ov_transformer_model_path, device.value)
disable_progress_bar(pipe)
dataset = datasets.load_dataset("google-research-datasets/conceptual_captions", "unlabeled", split="validation", trust_remote_code=True).shuffle(seed=42)
dataset = islice(dataset, validation_set_size)
inception_score = InceptionScore(normalize=True, splits=1)
images = []
infer_times = []
for batch in tqdm(dataset, total=validation_set_size, desc="Computing Inception Score"):
prompt = batch["caption"]
if len(prompt) > pipe.tokenizer.model_max_length:
continue
start_time = time.perf_counter()
image = pipe(prompt, generator=torch.Generator('cpu').manual_seed(0)).images[0]
infer_times.append(time.perf_counter() - start_time)
image = transforms.ToTensor()(image)
images.append(image)
mean_perf_time = sum(infer_times) / len(infer_times)
while len(images) > 0:
images_batch = torch.stack(images[-batch_size:])
images = images[:-batch_size]
inception_score.update(images_batch)
kl_mean, kl_std = inception_score.compute()
pipe.transformer.transformer = original_ov_transformer_model
disable_progress_bar(pipe, disable=False)
return kl_mean, mean_perf_time
original_inception_score, original_time = compute_inception_score(TRANSFORMER_OV_PATH, VALIDATION_DATASET_SIZE)
print(f"Original pipeline Inception Score: {original_inception_score}")
quantized_inception_score, quantized_time = compute_inception_score(QUANTIZED_TRANSFORMER_OV_PATH, VALIDATION_DATASET_SIZE)
print(f"Quantized pipeline Inception Score: {quantized_inception_score}")
print(f"Quantization speed-up: {original_time / quantized_time:.2f}x")
/opt/home/k8sworker/ci-ai/cibuilds/ov-notebook/OVNotebookOps-727/.workspace/scm/ov-notebook/.venv/lib/python3.8/site-packages/torchmetrics/utilities/prints.py:43: UserWarning: Metric InceptionScore will save all extracted features in buffer. For large datasets this may lead to large memory footprint. warnings.warn(*args, **kwargs) # noqa: B028
Computing Inception Score: 0%| | 0/100 [00:00<?, ?it/s]
/opt/home/k8sworker/ci-ai/cibuilds/ov-notebook/OVNotebookOps-727/.workspace/scm/ov-notebook/.venv/lib/python3.8/site-packages/diffusers/configuration_utils.py:140: FutureWarning: Accessing config attribute _execution_device directly via 'AmusedPipeline' object attribute is deprecated.Please access '_execution_device' over 'AmusedPipeline's config object instead, e.g. 'scheduler.config._execution_device'. deprecate("direct config name access", "1.0.0", deprecation_message, standard_warn=False) /opt/home/k8sworker/ci-ai/cibuilds/ov-notebook/OVNotebookOps-727/.workspace/scm/ov-notebook/.venv/lib/python3.8/site-packages/torchmetrics/image/inception.py:176: UserWarning: std(): degrees of freedom is <= 0. Correction should be strictly less than the reduction factor (input numel divided by output numel). (Triggered internally at ../aten/src/ATen/native/ReduceOps.cpp:1807.) return kl.mean(), kl.std()
Original pipeline Inception Score: 11.146076202392578
Computing Inception Score: 0%| | 0/100 [00:00<?, ?it/s]
Quantized pipeline Inception Score: 9.630992889404297
Quantization speed-up: 2.09x
インタラクティブな推論#
以下では、実行するパイプライン (オリジナルまたは量子化) を選択できます。
quantized_model_present = QUANTIZED_TRANSFORMER_OV_PATH.exists()
use_quantized_model = widgets.Checkbox(
value=True if quantized_model_present else False,
description="Use quantized pipeline",
disabled=not quantized_model_present,
)
use_quantized_model
Checkbox(value=True, description='Use quantized pipeline')
import gradio as gr
import numpy as np
pipe.transformer.transformer = core.compile_model(
QUANTIZED_TRANSFORMER_OV_PATH if use_quantized_model.value else TRANSFORMER_OV_PATH,
device.value,
)
def generate(prompt, seed, _=gr.Progress(track_tqdm=True)):
image = pipe(prompt, generator=torch.Generator("cpu").manual_seed(seed)).images[0]
return image
demo = gr.Interface(
generate,
[
gr.Textbox(label="Prompt"),
gr.Slider(0, np.iinfo(np.int32).max, label="Seed", step=1),
],
"image",
examples=[
["happy snowman", 88],
["green ghost rider", 0],
["kind smiling ghost", 8],
], allow_flagging="never",
)
try:
demo.queue().launch(debug=False)
except Exception:
demo.queue().launch(debug=False, share=True)
# リモートで起動する場合は、server_name と server_port を指定
# demo.launch(server_name='your server name', server_port='server port in int')
# 詳細はドキュメントをご覧ください: https://gradio.app/docs/
ローカル URL で実行中: http://127.0.0.1:7860 パブリックリンクを作成するには、launch() で share=True を設定します。